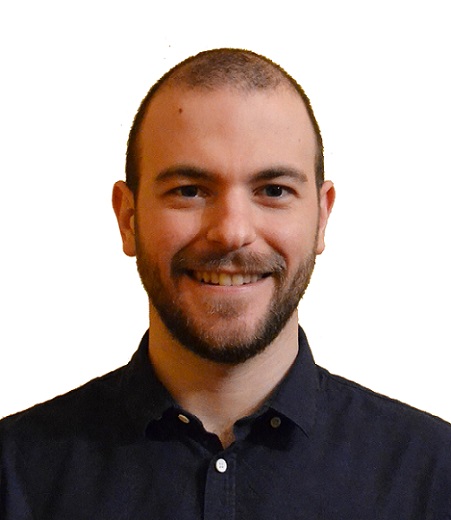 |
TOMMASO ZANOTTI
Ricercatore t.d. art. 24 c. 3 lett. A Dipartimento di Ingegneria "Enzo Ferrari"
|
Home |
Curriculum(pdf) |
Didattica |
Pubblicazioni
2024
- Guidelines for the Design of Random Telegraph Noise-Based True Random Number Generators
[Articolo su rivista]
Zanotti, T.; Ranjan, A.; O'Shea, S. J.; Raghavan, N.; Thamankar, R.; Pey, K. L.; Puglisi, F. M.
abstract
The development of a robust and secure hardware for the Internet of Things (IoT) and edge computing requires improvements in the existing low-power and low-cost hardware security primitives. Among the various available technologies, true random number generators (TRNGs) that leverage random telegraph noise (RTN) from nanoelectronics devices have emerged as effective solutions. However, the temporal instabilities in the RTN signal, such as the DC drift and temporary inhibition, are a few of the key reliability challenges for the TRNG circuits. In this study, we have utilized experimental RTN data collected from the commonly used gate dielectrics, including silicon dioxide (SiO2), hafnium dioxide (HfO2), and 2D crystalline hexagonal boron nitride (h-BN) to identify the crucial reliability challenges for RTN-based TRNG circuits. We have analyzed the impact of RTN instabilities and of circuit parameters on the output randomness and propose reliability aware design guidelines. Finally, we design and simulate an RTN-based TRNG circuit using a 130 nm CMOS technology and evaluate its reliability at the circuit level.
2024
- Information Transfer in Neuronal Circuits: From Biological Neurons to Neuromorphic Electronics
[Articolo su rivista]
Gandolfi, Daniela; Benatti, Lorenzo; Zanotti, Tommaso; M Boiani, Giulia; Bigiani, Albertino; Puglisi, Francesco Maria; Mapelli, Jonathan
abstract
The advent of neuromorphic electronics is increasingly revolutionizing the concept of computation. In the last decade, several studies have shown how materials, architectures, and neuromorphic devices can be leveraged to achieve brain-like computation with limited power consumption and high energy efficiency. Neuromorphic systems have been mainly conceived to support spiking neural networks that embed bioinspired plasticity rules such as spike time-dependent plasticity to potentially support both unsupervised and supervised learning. Despite substantial progress in the field, the information transfer capabilities of biological circuits have not yet been achieved. More importantly, demonstrations of the actual performance of neuromorphic systems in this context have never been presented. In this paper, we report similarities between biological, simulated, and artificially reconstructed microcircuits in terms of information transfer from a computational perspective. Specifically, we extensively analyzed the mutual information transfer at the synapse between mossy fibers and granule cells by measuring the relationship between pre- and post-synaptic variability. We extended this analysis to memristor synapses that embed rate-based learning rules, thus providing quantitative validation for neuromorphic hardware and demonstrating the reliability of brain-inspired applications.
2023
- Biologically Plausible Information Propagation in a CMOS Integrate-and-Fire Artificial Neuron Circuit with Memristive Synapses
[Articolo su rivista]
Benatti, Lorenzo; Zanotti, Tommaso; Gandolfi, Daniela; Mapelli, Jonathan; Puglisi, Francesco Maria
abstract
Neuromorphic circuits based on spikes are currently envisioned as a viable option to achieve brain-like computation capabilities in specific electronic implementations while limiting power dissipation given their ability to mimic energy efficient bio-inspired mechanisms. While several network architectures have been developed to embed in hardware the bio-inspired learning rules found in the biological brain, such as the Spike Timing Dependent Plasticity, it is still unclear if hardware spiking neural network architectures can handle and transfer information akin to biological networks. In this work, we investigate the analogies between an artificial neuron combining memristor synapses and rate-based learning rule with biological neuron response in terms of information propagation from a theoretical perspective. Bio-inspired experiments have been reproduced by linking the biological probability of release with the artificial synapses conductance. Mutual information and surprise have been chosen as metrics to evidence how, for different values of synaptic weights, an artificial neuron allows to develop a reliable and biological resembling neural network in terms of information propagation and analysis
2023
- Study of RRAM-Based Binarized Neural Networks Inference Accelerators Using an RRAM Physics-Based Compact Model
[Capitolo/Saggio]
Zanotti, Tommaso; Pavan, Paolo; Maria Puglisi, Francesco
abstract
In-memory computing hardware accelerators for binarized neural networks based on resistive RAM (RRAM) memory technologies represent a promising solution for enabling the execution of deep neural network algorithms on resource-constrained devices at the edge of the network. However, the intrinsic stochasticity and nonidealities of RRAM devices can easily lead to unreliable circuit operations if not appropriately considered during the design phase. In this chapter, analysis and design methodologies enabled by RRAM physics-based compact models of LIM and mixed-signal BNN inference accelerators are discussed. As a use case example, the UNIMORE RRAM physics-based compact model calibrated on an RRAM technology from the literature, is used to determine the performance vs. reliability trade-offs of different in-memory computing accelerators: i) a logic-in-memory accelerator based on the material implication logic, ii) a mixed-signal BNN accelerator, and iii) a hybrid accelerator enabling both computing paradigms on the same array. Finally, the performance of the three accelerators on a BNN inference task is compared and benchmarked with the state of the art.
2023
- Ultra-low power logic in memory with commercial grade memristors and FPGA-based smart-IMPLY architecture
[Articolo su rivista]
Benatti, L; Zanotti, T; Pavan, P; Puglisi, Fm
abstract
Reducing power consumption in nowadays computer technologies represents an increasingly difficult challenge. Conventional computing architectures suffer from the so-called von Neumann bottleneck (VNB), which consists in the continuous need to exchange data and instructions between the memory and the processing unit, leading to significant and apparently unavoidable power consumption. Even the hardware typically employed to run Artificial Intelligence (AI) algorithms, such as Deep Neural Networks (DNN), suffers from this limitation. A change of paradigm is so needed to comply with the ever-increasing demand for ultra-low power, autonomous, and intelligent systems. From this perspective, emerging memristive non-volatile memories are considered a good candidate to lead this technological transition toward the next-generation hardware platforms, enabling the possibility to store and process information in the same place, therefore bypassing the VNB. To evaluate the state of current public-available devices, in this work commercial-grade packaged Self Directed Channel memristors are thoroughly studied to evaluate their performance in the framework of in-memory computing. Specifically, the operating conditions allowing both analog update of the synaptic weight and stable binary switching are identified, along with the associated issues. To this purpose, a dedicated yet prototypical system based on an FPGA control platform is designed and realized. Then, it is exploited to fully characterize the performance in terms of power consumption of an innovative Smart IMPLY (SIMPLY) Logic-in-Memory (LiM) computing framework that allows reliable in-memory computation of classical Boolean operations. The projection of these results to the nanoseconds regime leads to an estimation of the real potential of this computing paradigm. Although not investigated in this work, the presented platform can also be exploited to test memristor-based SNN and Binarized DNNs (i.e., BNN), that can be combined with LiM to provide the heterogeneous flexible architecture envisioned as the long-term goal for ubiquitous and pervasive AI.
2022
- Comprehensive physics-based RRAM compact model including the effect of variability and multi-level random telegraph noise
[Articolo su rivista]
Zanotti, T; Pavan, P; Puglisi, Fm
abstract
Resistive Random Access Memory (RRAM) technologies are a promising candidate for the development of more energy efficient circuits, for computing, security, and storage applications. However, such devices show stochastic behaviours that not only originate from variations introduced during fabrication, but that are intrinsic to their operation. Specifically, cycle-to-cycle variations cause the programmed resistive state to be randomly distributed, while Random Telegraph Noise (RTN) introduces random current fluctuations over time. These phenomena can easily affect the reliability and performance of RRAM-based circuits. Therefore, designing such circuits requires accurate compact models. Although several RRAM compact models have been proposed in the literature, these are rarely implemented following the programming best-practice for improving the simulator convergence, and a compact model that is able to reproduce the device characteristic including thermal effects, RTN, and variability in multiple operating conditions using a single set of parameters is still missing. Also, only a few works in the literature describe the procedure to calibrate such compact models, and even fewer address the calibration of the variability on experimental data. In this work, we extend the UniMORE RRAM physics-based compact model by developing and validating two variability models, (i) a comprehensive variability model which can reproduce the effect of cycle-to-cycle variability in multiple operating conditions, and (ii) a simplified version that requires fewer calibration data and enables to reproduce cycle-to-cycle variations in specific operating conditions. The model is implemented following Verilog-A programming best-practices and validated on data from three RRAM technologies from the literature and experimentally on TiN/Ti/HfOx/TiN devices, and the relation between experimental data and the variability model parameters is described.
2022
- Hardware implementation of a true random number generator integrating a hexagonal boron nitride memristor with a commercial microcontroller
[Articolo su rivista]
Pazos, S.; Zheng, W.; Zanotti, T.; Aguirre, F.; Becker, T.; Shen, Y.; Zhu, K.; Yuan, Y.; Wirth, G.; Puglisi, F. M.; Roldan, J. B.; Palumbo, F.; Lanza, M.
abstract
The development of the internet-of-things requires cheap, light, small and reliable true random number generator (TRNG) circuits to encrypt the data-generated by objects or humans-before transmitting them. However, all current solutions consume too much power and require a relatively large battery, hindering the integration of TRNG circuits on most objects. Here we fabricated a TRNG circuit by exploiting stable random telegraph noise (RTN) current signals produced by memristors made of two-dimensional (2D) multi-layered hexagonal boron nitride (h-BN) grown by chemical vapor deposition and coupled with inkjet-printed Ag electrodes. When biased at small constant voltages (<= 70 mV), the Ag/h-BN/Ag memristors exhibit RTN signals with very low power consumption (similar to 5.25 nW) and a relatively high current on/off ratio (similar to 2) for long periods (>1 hour). We constructed TRNG circuits connecting an h-BN memristor to a small, light and cheap commercial microcontroller, producing a highly-stochastic, high-throughput signal (up to 7.8 Mbit s(-1)) even if the RTN at the input gets interrupted for long times up to 20 s, and if the stochasticity of the RTN signal is reduced. Our study presents the first full hardware implementation of 2D-material-based TRNGs, enabled by the unique stability and figures of merit of the RTN signals in h-BN based memristors.
2022
- Reliability and Prospects of Logic-in-Memory Circuits
[Relazione in Atti di Convegno]
Zanotti, T
abstract
With the growing demand for more energy efficient computing hardware to support edge computing applications, Logic-in-Memory (LiM) computing architectures are a promising candidate for implementing ultra-low-power in-memory hardware accelerators of logic operations. In this work, future opportunities for LiM solutions and their main reliability challenges are discussed.
2022
- Self-consistent Automated Parameter Extraction of RRAM Physics-Based Compact Model
[Relazione in Atti di Convegno]
Zanotti, Tommaso; Pavan, Paolo; Puglisi, Francesco Maria
abstract
2022
- Smart Material Implication Using Spin-Transfer Torque Magnetic Tunnel Junctions for Logic-in-Memory Computing
[Articolo su rivista]
De Rose, R.; Zanotti, T.; Puglisi, F. M.; Crupi, F.; Pavan, P.; Lanuzza, M.
abstract
Smart material implication (SIMPLY) logic has been recently proposed for the design of energy-efficient Logic-in-Memory (LIM) architectures based on non-volatile resistive memory devices. The SIMPLY logic is enabled by adding a comparator to the conventional IMPLY scheme. This allows performing a preliminary READ operation and hence the SET operation only in the case it is actually required. This work explores the SIMPLY logic scheme using nanoscale spin-transfer torque magnetic tunnel junction (STT-MTJ) devices. The performance of the STT-MTJ based SIMPLY architecture is analyzed by varying the load resistor and applied voltages to implement both READ and SET operations, while also investigating the effect of temperature on circuit operation. Obtained results show an existing tradeoff between error rate and energy consumption, which can be effectively managed by properly setting the values of load resistor and applied voltages. In addition, our analysis proves that tracking the temperature dependence of the MTJ properties through a proportional to absolute temperature (PTAT) reference voltage at the input of the comparator is beneficial to mitigate the reliability degradation under temperature variations.
2021
- Advanced Data Encryption using 2D Materials
[Articolo su rivista]
Wen, Chao; Li, Xuehua; Zanotti, Tommaso; Puglisi, Francesco Maria; Shi, Yuanyuan; Saiz, Fernan; Antidormi, Aleandro; Roche, Stephan; Zheng, Wenwen; Liang, Xianhu; Hu, Jiaxin; Duhm, Steffen; Roldan, Juan B.; Wu, Tianru; Chen, Victoria; Pop, Eric; Garrido, Blas; Zhu, Kaichen; Hui, Fei; Lanza, Mario
abstract
2021
- Energy-efficient non-von neumann computing architecture supporting multiple computing paradigms for logic and binarized neural networks
[Articolo su rivista]
Zanotti, T.; Puglisi, F. M.; Pavan, P.
abstract
Different in-memory computing paradigms enabled by emerging non-volatile memory technologies are promising solutions for the development of ultra-low-power hardware for edge com-puting. Among these, SIMPLY, a smart logic-in-memory architecture, provides high reconfigurability and enables the in-memory computation of both logic operations and binarized neural networks (BNNs) inference. However, operation-specific hardware accelerators can result in better performance for a particular task, such as the analog computation of the multiply and accumulate operation for BNN inference, but lack reconfigurability. Nonetheless, a solution providing the flexibility of SIMPLY while also achieving the high performance of BNN-specific analog hardware accelerators is missing. In this work, we propose a novel in-memory architecture based on 1T1R crossbar arrays, which enables the coexistence on the same crossbar array of both SIMPLY computing paradigm and the analog acceleration of the multiply and accumulate operation for BNN inference. We also highlight the main design tradeoffs and opportunities enabled by different emerging non-volatile memory technologies. Finally, by using a physics-based Resistive Random Access Memory (RRAM) compact model calibrated on data from the literature, we show that the proposed architecture improves the energy delay product by >103 times when performing a BNN inference task with respect to a SIMPLY implementation.
2021
- Low-Bit Precision Neural Network Architecture with High Immunity to Variability and Random Telegraph Noise based on Resistive Memories
[Relazione in Atti di Convegno]
Zanotti, Tommaso; Puglisi, Francesco Maria; Pavan, Paolo
abstract
In-memory computing architectures based on Resistive random access memory technologies (RRAM) are a promising candidate for the development of ultra-low power hardware accelerators that could enable the deployment of deep neural networks inference algorithms on energy constrained devices at the edge of the communication network. However, the study of the reliability of such circuits is non-trivial due to the intrinsic RRAM devices nonlinearity and stochasticity. For instance, RRAM devices are subject not only to device-to-device and cycle-to-cycle resistance variations but also to Random Telegraph Noise which introduces additional time dependent resistance fluctuations that could result in reduced circuit performance. Previous studies exploited simplified statistical models to show that such device nonidealities may reduce the classification accuracy even when binarized neural networks are employed. However, a circuit reliability analysis based on full circuit-level simulations is still missing. In this work, we develop and train a low-bit precision neural network which employs binary weights and 4-bits activations. We further analyze the impact of RRAM nonidealities (e.g., variability and Random Telegraph Noise) on the classification accuracy by means of full circuit-level simulations enabled by a physics-based RRAM compact model, calibrated on experimental data from the literature. Results show that combining binary weights with low-precision activations allows retaining software-level accuracy even in the presence of Random Telegraph Noise and weight variability.
2021
- Multi-Input Logic-in-Memory for Ultra-Low Power Non-Von Neumann Computing
[Articolo su rivista]
Zanotti, Tommaso; Pavan, Paolo; Puglisi, Francesco Maria
abstract
2021
- Optimized Synthesis Method for Ultra-Low Power Multi-Input Material Implication Logic With Emerging Non-Volatile Memories
[Articolo su rivista]
Puglisi, F. M.; Zanotti, T.; Pavan, P.
abstract
In this paper, we revisit Boole's expansion theorem to propose a new synthesis method for implication logic circuits based on memristors. By rewriting the sum-of-products form of Boole's expansion theorem in terms that are best suited for the implication logic, we develop a generalized rule to derive the sequence of operations needed to realize any logic function written in the classical AND-OR form. The proposed method leverages on multi-input operation, minimizing both the number of steps required to compute a given Boolean function and the number of memristors involved. Moreover, it allows using well-established methods of logic circuit optimization like binary decision diagrams, Karnaugh maps, and heuristic algorithms, that are already implemented in commercial CAD software. The proposed method allows a fair comparison between the performance of CMOS and implication logic implementations of the same logic function under the same degree of optimization, and is shown to outperform existing approaches. Possible device-circuit co-design strategies to optimize circuit performance are finally discussed.
2021
- Performances and Trade-offs of Low-Bit Precision Neural Networks based on Resistive Memories
[Relazione in Atti di Convegno]
Zanotti, T.; Pavan, P.; Puglisi, F. M.
abstract
In this work we devise and train a RRAM-based low-precision neural network with binary weights and 4-bits activations. Full-circuit simulations including the analog neuron peripheral circuitry are run in different conditions, including the effect of RRAM devices nonidealities, to evaluate the reliability and performance of the network when executing a classification task. Results show that the power-throughput trade-off during inference is governed by the neuron circuitry, and that the reset conditions can be tuned to simultaneously maximize energy efficiency and accuracy leading to improved network reliability. Accuracy losses are found to be dominated by the variability of the RRAMs in low resistive state (LRS), which suggests specific strategies for accuracy loss minimization. The network shows excellent performance in terms of accuracy, throughput, and energy efficiency, with robustness to RRAM non-idealities.
2021
- Random Telegraph Noise in Metal-Oxide Memristors for True Random Number Generators: A Materials Study
[Articolo su rivista]
Li, X.; Zanotti, T.; Wang, T.; Zhu, K.; Puglisi, F. M.; Lanza, M.
abstract
Some memristors with metal/insulator/metal (MIM) structure have exhibited random telegraph noise (RTN) current signals, which makes them ideal to build true random number generators (TRNG) for advanced data encryption. However, there is still no clear guide on how essential manufacturing parameters like materials selection, thicknesses, deposition methods, and device lateral size can influence the quality of the RTN signal. In this paper, an exhaustive statistical analysis on the quality of the RTN signals produced by different MIM-like memristors is reported, and straightforward guidelines for the fabrication of memristors with enhanced RTN performance are presented, which are: i) Ni and Ti electrodes show better RTN than Au electrodes, ii) the 50 μm × 50 μm devices show better RTN than the 5 μm × 5 μm ones, iii) TiO2 shows better RTN than HfO2 and Al2O3, iv) sputtered-oxides show better RTN than ALD-oxides, and v) 10 nm thick oxides show better RTN than 5 nm thick oxides. The RTN signals recorded have been used as entropy sources in high-throughput TRNG circuits, which have passed the randomness tests of the National Institute of Standards and Technology. The work can serve as a useful guide for materials scientists and electronic engineers when fabricating MIM-like memristors for RTN applications.
2021
- Reliability and Performance Analysis of Logic-in-Memory Based Binarized Neural Networks
[Articolo su rivista]
Zanotti, T.; Puglisi, F. M.; Pavan, P.
abstract
Resistive Random access memory (RRAM) devices together with the material implication (IMPLY) logic are a promising computing scheme for realizing energy efficient reconfigurable computing hardware for edge computing applications. This approach has been recently shown to enable the in-memory implementation of Binarized Neural Networks. However, an accurate analysis of the performance achieved on a real classification task are still missing. In this work, we train and estimate the performance of an IMPLY-based implementation of a multilayer perceptron (MLP) BNN and highlight its main reliability challenges by using a physics-based RRAM compact model calibrated on three RRAM technologies from the literature. We then show how the smart IMPLY (SIMPLY) architecture solves the reliability issues of conventional IMPLY architectures and compare its performance with respect to conventional solutions considering different parallelization degree. The worst-case energy estimates for an inference task performed on the trained network, show that the SIMPLY implementation results in a >46 energy-delay-product (EDP) improvement with respect to a conventional low-power embedded system implementation.
2021
- STT-MTJ Based Smart Implication for Energy-Efficient Logic-in-Memory Computing
[Articolo su rivista]
De Rose, Raffaele; Zanotti, Tommaso; Maria Puglisi, Francesco; Crupi, Felice; Pavan, Paolo; Lanuzza, Marco
abstract
Spin-transfer torque magnetic tunnel junction (STT-MTJ) technology is an attractive solution for designing non-volatile Logic-in-Memory (LIM) architectures. This work explores a smart material implication (SIMPLY) LIM scheme based on nanoscale STT-MTJs. The SIMPLY architecture is benchmarked against the conventional material implication (IMPLY) logic. Obtained results prove that for similar performance the STT-MTJ based SIMPLY scheme ensures more reliable operation (i.e., lower error rate by more than three orders of magnitude) and an energy saving of -70% than its IMPLY counterpart, at the only cost of minimal area overhead.
2020
- Circuit Reliability Analysis of In-Memory Inference in Binarized Neural Networks
[Relazione in Atti di Convegno]
Zanotti, Tommaso; Puglisi, Francesco Maria; Pavan, Paolo
abstract
2020
- Circuit Reliability Analysis of RRAM-based Logic-in-Memory Crossbar Architectures Including Line Parasitic Effects, Variability, and Random Telegraph Noise
[Relazione in Atti di Convegno]
Zanotti, T.; Puglisi, F. M.; Pavan, P.
abstract
The Logic-in-Memory paradigm is considered a promising solution for improving the energy efficiency and computing power of architectures aimed at low power and/or data-intensive applications. Among in-memory computing enabling technologies, emerging non-volatile memories (e.g., RRAMs) are promising as they offer BEOL integration and small feature size. Several studies have shown that IMPLY architectures based on RRAM devices and the material implication logic enable the efficient computation of logic operations using the RRAM device both as storing and computing element. However, RRAM devices non-idealities introduce important circuit reliability issues, that are frequently neglected, thus undermining the circuit functionality. In this work, we use a physics-based compact model calibrated on experimental data to simulate the IMPLY operation performed on a crossbar array including line parasitic effects and RRAM devices non-idealities. We then introduce a novel smart scheme, SIMPLY, and show the circuit reliability improvement.
2020
- Reconfigurable Smart In-Memory Computing Platform Supporting Logic and Binarized Neural Networks for Low-Power Edge Devices
[Articolo su rivista]
Zanotti, T.; Puglisi, F. M.; Pavan, P.
abstract
Edge computing has been shown to be a promising solution that could relax the burden imposed onto the network infrastructure by the increasing amount of data produced by smart devices. However, reconfigurable ultra-low power computing architectures are needed. RRAM devices together with the material implication logic (IMPLY) are a promising solution for the development of low-power reconfigurable logic-in-memory (LiM) hardware. Nevertheless, traditional approaches suffer from several issues introduced by the circuit topology and device non-idealities. Recently, SIMPLY, a smart LiM architecture based on the IMPLY, has been proposed and shown to solve the common issues of traditional architectures. Here, we use a physics-based RRAM compact model calibrated on three RRAM technologies to further analyze the performance of SIMPLY in typical operating conditions, when the repeated execution of logic operation on the same group of devices is considered. The results show that, compared to the conventional IMPLY architecture, SIMPLY spares more than 40% of the high voltage pulses on average even when complex operations are considered (e.g., the 1-bit half adder). We also show how SIMPLY can implement the set of operations required for the implementation of Binarized Neural Networks (BNN) and benchmark its performance against other memristor-based BNN in-memory accelerator from the literature. The results suggest that our approach is more than two orders of magnitude efficient compared to the state of the art reconfigurable in-memory computing approach and could potentially reach the performance of specialized BNN analog hardware accelerators with appropriate device-circuit co-design strategies.
2020
- Reliability of Logic-in-Memory Circuits in Resistive Memory Arrays
[Articolo su rivista]
Zanotti, T.; Zambelli, C.; Puglisi, F. M.; Milo, V.; Perez, E.; Mahadevaiah, M. K.; Ossorio, O. G.; Wenger, C.; Pavan, P.; Olivo, P.; Ielmini, D.
abstract
Logic-in-memory (LiM) circuits based on resistive random access memory (RRAM) devices and the material implication logic are promising candidates for the development of low-power computing devices that could fulfill the growing demand of distributed computing systems. However, these circuits are affected by many reliability challenges that arise from device nonidealities (e.g., variability) and the characteristics of the employed circuit architecture. Thus, an accurate investigation of the variability at the array level is needed to evaluate the reliability and performance of such circuit architectures. In this work, we explore the reliability and performance of smart IMPLY (SIMPLY) (i.e., a recently proposed LiM architecture with improved reliability and performance) on two 4-kb RRAM arrays based on different resistive switching oxides integrated in the back end of line (BEOL) of the 0.25-μm BiCMOS process. We analyze the tradeoff between reliability and energy consumption of SIMPLY architecture by exploiting the results of an extensive array-level variability characterization of the two technologies. Finally, we study the worst case performance of a full adder implemented with the SIMPLY architecture and benchmark it on the analogous CMOS implementation.
2020
- Reliability-Aware Design Strategies for Stateful Logic-in-Memory Architectures
[Articolo su rivista]
Zanotti, Tommaso; Puglisi, Francesco Maria; Pavan, Paolo
abstract
2020
- Smart Logic-in-Memory Architecture For Ultra-Low Power Large Fan-In Operations
[Relazione in Atti di Convegno]
Zanotti, Tommaso; Puglisi, Francesco Maria; Pavan, Paolo
abstract
The need for processing the continuously growing amount of data that is produced every day is promoting research for the development of energy-efficient non-von Neumann computing architectures. Over the last decade, resistive RAM (RRAM) devices together with material implication logic (IMPLY) were proposed as a promising solution for the development of low-power logic-in-memory (LIM) circuits. Still, the high design complexity and the low reliability of these circuits are hindering their practical realization. It is only recently that a new smart IMPLY architecture, named SIMPLY, was proposed and shown to drastically improve circuit reliability and energy efficiency of IMPLY-based LIM circuits. In this work, we introduce a new smart operation, called sFALSE, enabled by the SIMPLY architecture, and verify its feasibility using a physics-based RRAM compact model calibrated on three different technologies. We highlight the significant advantage of the proposed solution vs. ordinary IMPLY architecture in terms of energy reduction, especially for large fan-in logic operations (e.g., n-bits NAND and EXOR).
2020
- Smart Logic-in-Memory Architecture for Low-Power non-von Neumann Computing
[Articolo su rivista]
Zanotti, Tommaso; Puglisi, Francesco Maria; Pavan, Paolo
abstract
Low-power smart devices are becoming pervasive in our world. Thus, relevant research efforts are directed to the development of innovative low power computing solutions that enable in-memory computations of logic-operations, thus avoiding the von Neumann bottleneck, i.e., the known showstopper of traditional computing architectures. Emerging non-volatile memory technologies, in particular Resistive Random Access memories, have been shown to be particularly suitable to implement logic-in-memory (LIM) circuits based on the material implication logic (IMPLY). However, RRAM devices nonidealities, logic state degradation, and a narrow design space limit the adoption of this logic scheme. In this work, we use a physics-based compact model to study an innovative smart IMPLY (SIMPLY) logic scheme which exploits the peripheral circuitry embedded in ordinary IMPLY architectures to solve the mentioned reliability issues, drastically reducing the energy consumption and setting clear design strategies. We then use SIMPLY to implement a 1-bit full adder and compare the results with other LIM solutions proposed in the literature.
2019
- Circuit reliability of low-power rram-based logic-in-memory architectures
[Relazione in Atti di Convegno]
Zanotti, T.; Puglisi, F. M.; Pavan, P.
abstract
Logic circuits based on Resistive RAM (RRAM) devices and the material implication logic (IMPLY) are promising solutions for low-power logic-in-memory (LiM) architectures. Still, their diffusion is limited by their high design complexity resulting from device and circuit non-idealities. These non-idealities are usually overlooked in the design phase when using simplified RRAM models, thus leading to unreliable designs. In this work, we derive correct design strategies for reliability of RRAM-based LiM circuits and quantitatively evaluate circuit performances using a physics-based compact model.
2019
- METODO DI LETTURA PER CIRCUITI DEL TIPO LOGIC-IN-MEMORY E RELATIVA ARCHITETTURA CIRCUITALE
[Brevetto]
Puglisi, Francesco Maria; Pavan, Paolo; Zanotti, Tommaso
abstract
2019
- SIMPLY: Design of a RRAM-Based Smart Logic-in-Memory Architecture using RRAM Compact Model
[Relazione in Atti di Convegno]
Puglisi, F. M.; Zanotti, T.; Pavan, P.
abstract
In this work, we introduce a new RRAM-based Smart IMPLY (SIMPLY) logic scheme with unique benefits for low-power systems and verify its feasibility and advantages by means of circuit simulations allowing appropriate device/circuit requirements co-design. Differently from previous works, we use a physics-based compact model of RRAM devices able to reproduce both the ultrafast AC and the DC behavior, accounting for the intrinsic variability of the resistive states, the occurrence of Random Telegraph Noise, and the logic state degradation. The proposed scheme strongly alleviates the issue of logic state degradation, breaks the trade-off between the choice of VSET and VCOND, and allows saving energy up to a factor of ~230 requiring minimum area overhead.
2019
- Unimore Resistive Random Access Memory (RRAM) Verilog-A Model 1.0.0
[Software]
Puglisi, Francesco Maria; Zanotti, Tommaso; Pavan, Paolo
abstract
The Unimore RRAM Verilog-A model is a physics-based compact model of bipolar RRAM which includes cycle-to-cycle variability, thermal effects, self-heating, and multilevel Random Telegraph Noise (RTN). The model considers both the quasi-ohmic charge transport along the conductive filament and the trap-assisted tunneling transport in the dielectric barrier. The reset/set operations dynamics is modeled with differential equations considering the field-driven oxygen ions drift and recombination during reset (i.e., barrier growth), and the field accelerated bond breakage during set (i.e., barrier collapse). The temperature dynamics is, likewise, modeled with differential equations that enable accurate predictions also when using very short pulses. Thus, the model enables the advanced design of circuits for many applications such as Memory, Neuromorphic Circuits, RRAM-based Neural Networks, Logic-In-Memory Systems, Physical Unclonable Functions, True Random Number Generators and others.