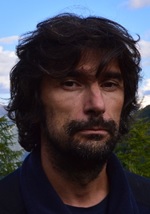 |
SIMONE BENATTI
Ricercatore t.d. art. 24 c. 3 lett. B Dipartimento di Scienze e Metodi dell'Ingegneria
|
Home |
Curriculum(pdf) |
Didattica |
Pubblicazioni
2023
- A Wearable Ultra-Low-Power sEMG-triggered Ultrasound System for Long-term Muscle Activity Monitoring
[Relazione in Atti di Convegno]
Frey, S.; Kartsch, V.; Leitner, C.; Cossettini, A.; Vostrikov, S.; Benatti, S.; Benini, L.
abstract
2023
- ANGELS - Smart Steering Wheel for Driver Safety
[Relazione in Atti di Convegno]
Amidei, A.; Rapa, P. M.; Tagliavini, G.; Rabbeni, R.; Pavan, P.; Benatti, S.
abstract
The automotive industry increasingly recognizes the importance of human-machine interaction in enhancing the driving experience and improving driver safety. Human factors, such as drowsiness and attention deficits, play a primary role in safe driving. There are several research and commercial solutions to address these issues. However, they analyze vehicle behavior and are unable to assess the driver's state in a timely manner. A novel approach to this problem is to monitor the driver's physiological signals. In this context, Photoplethysmography (PPG) is a noninvasive technique that monitors cardiac activity and can provide information regarding the driver's state. This work introduces ANGELS, an embedded system that exploits PPG signals to monitor drivers in a non-invasive way. ANGELS is a low-cost and low-power system that can be integrated into the steering wheel of a car. It acquires and processes the driver's PPG signals in real-time and enables heart rate monitoring without requiring accelerometer data to remove motion artifacts. We perform an experimental assessment using the Maserati driving simulator. ANGELS features a mean absolute error on heart rate detection of 1.19 BPM with a latency of 10 s and power consumption of only 130 mW. These results demonstrate that it is a reliable and promising solution for improving driver safety.
2023
- An Optimized Heart Rate Detection System Based on Low-Power Microcontroller Platforms for Biosignal Processing
[Relazione in Atti di Convegno]
Mazzoni, B.; Tagliavini, G.; Benini, L.; Benatti, S.
abstract
The real-time detection of the R peaks of the ECG signal is crucial to provide information on cardiac functionality, and several strategies have been presented in the past. In this work, we adapt the classical Pan and Tompkins (PT) algorithm for efficient execution on low-power microcontroller (MCU) platforms to design a full-fledged heart rate detection system. We target a commercial MCU based on ARM Cortex-M4 and an ultra-low-power solution based on the RISC-V PULP platform. Experimental results show that our approach achieves an accuracy above 99.5%, comparable to the state-of-the-art solutions, and an energy efficiency that is one order of magnitude better than other software solutions.
2023
- BioGAP: A 10-Core FP-capable Ultra-Low Power IoT Processor, with Medical-Grade AFE and BLE Connectivity for Wearable Biosignal Processing
[Relazione in Atti di Convegno]
Frey, S.; Guermandi, M.; Benatti, S.; Kartsch, V.; Cossettini, A.; Benini, L.
abstract
2023
- Driver Drowsiness Detection: A Machine Learning Approach on Skin Conductance
[Articolo su rivista]
Amidei, A.; Spinsante, S.; Iadarola, G.; Benatti, S.; Tramarin, F.; Pavan, P.; Rovati, L.
abstract
The majority of car accidents worldwide are caused by drowsy drivers. Therefore, it is important to be able to detect when a driver is starting to feel drowsy in order to warn them before a serious accident occurs. Sometimes, drivers are not aware of their own drowsiness, but changes in their body signals can indicate that they are getting tired. Previous studies have used large and intrusive sensor systems that can be worn by the driver or placed in the vehicle to collect information about the driver’s physical status from a variety of signals that are either physiological or vehicle-related. This study focuses on the use of a single wrist device that is comfortable for the driver to wear and appropriate signal processing to detect drowsiness by analyzing only the physiological skin conductance (SC) signal. To determine whether the driver is drowsy, the study tests three ensemble algorithms and finds that the Boosting algorithm is the most effective in detecting drowsiness with an accuracy of 89.4%. The results of this study show that it is possible to identify when a driver is drowsy using only signals from the skin on the wrist, and this encourages further research to develop a real-time warning system for early detection of drowsiness.
2023
- ECHOES: a 200 GOPS/W Frequency Domain SoC with FFT Processor and I2S DSP for Flexible Data Acquisition from Microphone Arrays
[Relazione in Atti di Convegno]
Sinigaglia, M.; Bertaccini, L.; Valente, L.; Garofalo, A.; Benatti, S.; Benini, L.; Conti, F.; Rossi, D.
abstract
Emerging applications in the IoT domain require ultra-low-power and high-performance end-nodes to deal with complex near-sensor-data analytics. Domains such as audio, radar, and Structural Health Monitoring require many computations to be performed in the frequency domain rather than in the time domain. We present Echoes, a System-On-a-Chip (SoC) composed of a RISC-V core enhanced with fixed- and floating-point digital signal processing (DSP) extensions and a Fast-Fourier Transform (FFT) hardware accelerator targeting emerging frequency-domain application. The proposed SoC features an autonomous I/O engine supporting a wide set of peripherals, including Ultra-Low-Power radars, MEMS, and digital microphones over I2S protocol with full-duplex Time Division Multiplexing DSP mode, making Echoes the first open-source SoC which offers this functionality enabling simultaneous communication with up to 16 I/Os devices. Echoes, fabricated with 65nm CMOS technology, reaches a peak performance of 0.16 GFLOPS and a peak energy efficiency of 9.68 GFLOPS/W on a wide range of floating and fixed-point general-purpose DSP kernels. The FFT accelerator achieves performance up to 10.16 GOPS with an efficiency of 199.8 GOPS/W, improving performance and efficiency by up to 41.1× and 11.2×, respectively, over its software implementation of this critical task for frequency domain processing.
2023
- Event-based Low-Power and Low-Latency Regression Method for Hand Kinematics from Surface EMG
[Relazione in Atti di Convegno]
Zanghieri, M.; Benatti, S.; Benini, L.; Donati, E.
abstract
Human-Machine Interfaces (HMIs) are a rapidly progressing field, and gesture recognition is a promising method in industrial, consumer, and health use cases. Surface electromyography (sEMG) is a State-of-the-Art (SoA) pathway for human-to-machine communication. Currently, the research goal is a more intuitive and fluid control, moving from signal classification of discrete positions to continuous control based on regression. The sEMG-based regression is still scarcely explored in research since most approaches have addressed classification. In this work, we propose the first event-based EMG encoding applied to the regression of hand kinematics suitable for working in streaming on a low-power microcontroller (STM32 F401, mounting ARM Cortex-M4). The motivation for event-based encoding is to exploit upcoming neuromorphic hardware to benefit from reduced latency and power consumption. We achieve a Mean Absolute Error of 8.8± 2.3 degrees on 5 degrees of actuation on the public dataset NinaPro DB8, comparable with the SoA Deep Neural Network (DNN). We use 9× less memory and 13× less energy per inference, with 10× shorter latency per inference compared to the SoA deep net, proving suitable for resource-constrained embedded platforms.
2023
- Exploiting Blood Volume Pulse and Skin Conductance for Driver Drowsiness Detection
[Relazione in Atti di Convegno]
Poli, A.; Amidei, A.; Benatti, S.; Iadarola, G.; Tramarin, F.; Rovati, L.; Pavan, P.; Spinsante, S.
abstract
2023
- Long-Term Stable Electromyography Classification Using Canonical Correlation Analysis
[Relazione in Atti di Convegno]
Donati, E.; Benatti, S.; Ceolini, E.; Indiveri, G.
abstract
Discrimination of hand gestures based on the decoding of surface electromyography (sEMG) signals is a well-establish approach for controlling prosthetic devices and for Human-Machine Interfaces (HMI). However, despite the promising results achieved by this approach in well-controlled experimental conditions, its deployment in long-term real-world application scenarios is still hindered by several challenges. One of the most critical challenges is maintaining high EMG data classification performance across multiple days without retraining the decoding system. The drop in performance is mostly due to the high EMG variability caused by electrodes shift, muscle artifacts, fatigue, user adaptation, or skinelectrode interfacing issues. Here we propose a novel statistical method based on canonical correlation analysis (CCA) that stabilizes EMG classification performance across multiple days for long-term control of prosthetic devices. We show how CCA can dramatically decrease the performance drop of standard classifiers observed across days, by maximizing the correlation among multiple-day acquisition data sets. Our results show how the performance of a classifier trained on EMG data acquired only of the first day of the experiment maintains 90% relative accuracy across multiple days, compensating for the EMG data variability that occurs over long-term periods, using the CCA transformation on data obtained from a small number of gestures. This approach eliminates the need for large data sets and multiple or periodic training sessions, which currently hamper the usability of conventional pattern recognition based approaches.
2023
- Motor-Unit Ordering of Blindly-Separated Surface-EMG Signals for Gesture Recognition
[Relazione in Atti di Convegno]
Orlandi, M.; Zanghieri, M.; Schiavone, D.; Donati, E.; Conti, F.; Benatti, S.
abstract
Hand gestures are one of the most natural and expressive way for humans to convey information, and thus hand gesture recognition has become a research hotspot in the human-machine interface (HMI) field. In particular, biological signals such as surface electromyography (sEMG) can be used to recognize hand gestures to implement intuitive control systems, but the decoding from the sEMG signal to actual control signals is non-trivial. Blind source separation (BSS)-based methods, such as convolutive independent component analysis (ICA), can be used to decompose the sEMG signal into its fundamental elements, the motor unit action potential trains (MUAPTs), which can then be processed with a classifier to predict hand gestures. However, ICA does not guarantee a consistent ordering of the extracted motor units (MUs), which poses a problem when considering multiple recording sessions and subjects; therefore, in this work we propose and validate three approaches to address this variability: two ordering criteria based on firing rate and negative entropy, and a re-calibration procedure, which allows the decomposition model to retain information about previous recording sessions when decomposing new data. In particular, we show that re-calibration is the most robust approach, yielding an accuracy up to 99.4%, and always greater than 85% across all the different scenarios that we tested. These results prove that our proposed system, which we publish open-source and which is based on biologically plausible features rather than on data-driven, black-box models, is capable of robust generalization.
2022
- A Low-Power Transprecision Floating-Point Cluster for Efficient Near-Sensor Data Analytics
[Articolo su rivista]
Montagna, F.; Mach, S.; Benatti, S.; Garofalo, A.; Ottavi, G.; Benini, L.; Rossi, D.; Tagliavini, G.
abstract
Recent applications in low-power (1-20 mW) near-sensor computing require the adoption of floating-point arithmetic to reconcile high precision results with a wide dynamic range. In this article, we propose a low-power multi-core computing cluster that leverages the fined-grained tunable principles of transprecision computing to provide support to near-sensor applications at a minimum power budget. Our solution - based on the open-source RISC-V architecture - combines parallelization and sub-word vectorization with a dedicated interconnect design capable of sharing floating-point units (FPUs) among the cores. On top of this architecture, we provide a full-fledged software stack support, including a parallel low-level runtime, a compilation toolchain, and a high-level programming model, with the aim to support the development of end-to-end applications. We performed an exhaustive exploration of the design space of the transprecision cluster on a cycle-accurate FPGA emulator, varying the number of cores and FPUs to maximize performance. Orthogonally, we performed a vertical exploration to identify the most efficient solutions in terms of non-functional requirements (operating frequency, power, and area). We conducted an experimental assessment on a set of benchmarks representative of the near-sensor processing domain, complementing the timing results with a post place - route analysis of the power consumption. A comparison with the state-of-the-art shows that our solution outperforms the competitors in energy efficiency, reaching a peak of 97 Gflop/s/W on single-precision scalars and 162 Gflop/s/W on half-precision vectors. Finally, a real-life use case demonstrates the effectiveness of our approach in fulfilling accuracy constraints.
2022
- A Wireless System for EEG Acquisition and Processing in an Earbud Form Factor with 600 Hours Battery Lifetime
[Relazione in Atti di Convegno]
Guermandi, M.; Cossettini, A.; Benatti, S.; Benini, L.
abstract
In recent years, in-ear electroencephalography (EEG) was demonstrated to record signals of similar quality compared to standard scalp-based EEG, and clinical applications of objective hearing threshold estimations have been reported. Existing devices, however, still lack important features. In fact, most of the available solutions are based on wet electrodes, require to be connected to external acquisition platforms, or do not offer on-board processing capabilities. Here we overcome all these limitations, presenting an ear-EEG system based on dry electrodes that includes all the acquisition, processing, and connectivity electronics directly in the ear bud. The earpiece is equipped with an ultra-low power analog front-end for analog-to-digital conversion, a low-power MEMS microphone, a low-power inertial measurement unit, and an ARM Cortex-M4 based microcontroller enabling on-board processing and Bluetooth Low Energy connectivity. The system can stream raw EEG data or perform data processing directly in-ear. We test the device by analysing its capability to detect brain response to external auditory stimuli, achieving 4 and 1.3 mW power consumption for data streaming or on board processing, respectively. The latter allows for 600 hours operation on a PR44 zinc-air battery. To the best of our knowledge, this is the first wireless and fully self-contained ear-EEG system performing on-board processing, all embedded in a single earbud. Clinical relevance- The proposed ear-EEG system can be employed for diagnostic tasks such as objective hearing threshold estimations, outside of clinical settings, thereby enabling it as a point-of-care solution. The long battery lifetime is also suitable for a continuous monitoring scenario.
2022
- BioWolf16: a 16-channel, 24-bit, 4kSPS Ultra-Low Power Platform for Wearable Clinical-grade Bio-potential Parallel Processing and Streaming
[Relazione in Atti di Convegno]
Donati, R.; Kartsch, V.; Benini, L.; Benatti, S.
abstract
Low-power wearable systems are essential for medical and industrial applications, but they face crucial implementation challenges when providing energy-efficient compact design while increasing the number of available channels, sampling rate and overall processing power. This work presents a small (39×41mm) wireless embedded low-power HMI device for ExG signals, offering up to 16 channels sampled at up to 4kSPS. By virtue of the high sampling rate and medical-grade signal quality (i.e. compliant with the IFCN standards), BioWolf16 is capable of accurate gesture recognition and enables the possibility to acquire data for neural spikes extraction. When employed over an EMG gesture recognition paradigm, the system achieves 90.24% classification accuracy over nine gestures (16 channels@4kSPS) while requiring only 16mW of power (57h of continuous operation) when deployed on Mr. Wolf MCU, part of the system architecture. The system can also provide up to 14h of real-time data streaming (4kSPS), which can further be extended to 23h when reducing the sampling rate to 1kSPS. Our results also demonstrate that this design outperforms many features of current state-of-the-art systems. Clinical Relevance-This work establishes that BioWolf16 is a wearable ultra-low power device enabling advanced multi-channel streaming and processing of medical-grade EMG signal, that can expand research opportunities and applications in healthcare and industrial scenarios.
2022
- Bioformers: Embedding Transformers for Ultra-Low Power sEMG-based Gesture Recognition
[Relazione in Atti di Convegno]
Burrello, A.; Morghet, F. B.; Scherer, M.; Benatti, S.; Benini, L.; Macii, E.; Poncino, M.; Pagliari, D. J.
abstract
2022
- ControlPULP: A RISC-V Power Controller for HPC Processors with Parallel Control-Law Computation Acceleration
[Relazione in Atti di Convegno]
Ottaviano, A.; Balas, R.; Bambini, G.; Bonfanti, C.; Benatti, S.; Rossi, D.; Benini, L.; Bartolini, A.
abstract
High-Performance Computing (HPC) processors are nowadays integrated Cyber-Physical Systems requiring complex and high-performance closed-loop control strategies for efficient power and thermal management. To satisfy high-bandwidth, real-time multi-input multi-output (MIMO) optimal power control requirements, high-end processors integrate on-die Power Controller Systems (PCS). Traditional PCS is based on a simple microcontroller core supported by dedicated interface logic and sequencers. More scalable and flexible PCS architectures are required to support advanced MIMO control algorithms required for managing the ever-increasing number of cores, power states, and process, voltage, temperature (PVT) variability. In this paper, we present ControlPULP, a complete, open-source HW/SW RISC-V parallel PCS platform consisting of a single-core microcontroller coupled with a scalable multi-core cluster system with a specialized DMA engine and a fast multi-core interrupt controller for parallel acceleration of real-time power management policies. ControlPULP relies on a real-time OS (FreeRTOS) to schedule a Power Control Firmware (PCF) software layer. We evaluate ControlPULP design choices in a cycle-accurate, event-based simulation environment and show the benefits of the proposed multi-core acceleration solution. We demonstrate ControlPULP in a PCS use-case targeting a next-generation 72-cores HPC processor. We show that the multi-core cluster accelerates the PCF achieving 4.9x speedup with respect to single-core execution.
2022
- Embedding Temporal Convolutional Networks for Energy-efficient PPG-based Heart Rate Monitoring
[Articolo su rivista]
Burrello, A.; Pagliari, D. J.; Rapa, P. M.; Semilia, M.; Risso, M.; Polonelli, T.; Poncino, M.; Benini, L.; Benatti, S.
abstract
Photoplethysmography (PPG) sensors allow for non-invasive and comfortable heart rate (HR) monitoring, suitable for compact wrist-worn devices. Unfortunately, motion artifacts (MAs) severely impact the monitoring accuracy, causing high variability in the skin-to-sensor interface. Several data fusion techniques have been introduced to cope with this problem, based on combining PPG signals with inertial sensor data. Until now, both commercial and reasearch solutions are computationally efficient but not very robust, or strongly dependent on hand-tuned parameters, which leads to poor generalization performance. In this work, we tackle these limitations by proposing a computationally lightweight yet robust deep learning-based approach for PPG-based HR estimation. Specifically, we derive a diverse set of Temporal Convolutional Networks for HR estimation, leveraging Neural Architecture Search. Moreover, we also introduce ActPPG, an adaptive algorithm that selects among multiple HR estimators depending on the amount of MAs, to improve energy efficiency. We validate our approaches on two benchmark datasets, achieving as low as 3.84 beats per minute of Mean Absolute Error on PPG-Dalia, which outperforms the previous state of the art. Moreover, we deploy our models on a low-power commercial microcontroller (STM32L4), obtaining a rich set of Pareto optimal solutions in the complexity vs. accuracy space.
2022
- Energy-Efficient Tree-Based EEG Artifact Detection
[Relazione in Atti di Convegno]
Ingolfsson, T. M.; Cossettini, A.; Benatti, S.; Benini, L.
abstract
In the context of epilepsy monitoring, EEG artifacts are often mistaken for seizures due to their morphological simi-larity in both amplitude and frequency, making seizure detection systems susceptible to higher false alarm rates. In this work we present the implementation of an artifact detection algorithm based on a minimal number of EEG channels on a parallel ultra-low-power (PULP) embedded platform. The analyses are based on the TUH EEG Artifact Corpus dataset and focus on the temporal electrodes. First, we extract optimal feature models in the frequency domain using an automated machine learning framework, achieving a 93.95% accuracy, with a 0.838 F1 score for a 4 temporal EEG channel setup. The achieved accuracy levels surpass state-of-the-art by nearly 20%. Then, these algorithms are parallelized and optimized for a PULP platform, achieving a 5.21x improvement of energy-efficient compared to state-of-the-art low-power implementations of artifact detection frameworks. Combining this model with a low-power seizure detection algorithm would allow for 300h of continuous monitoring on a 300 mAh battery in a wearable form factor and power budget. These results pave the way for implementing affordable, wearable, long-term epilepsy monitoring solutions with low false-positive rates and high sensitivity, meeting both patients' and caregivers' requirements. Clinical relevance- The proposed EEG artifact detection framework can be employed on wearable EEG recording devices, in combination with EEG-based epilepsy detection algorithms, for improved robustness in epileptic seizure detection scenarios.
2021
- A Fully Integrated 5-mW, 0.8-Gbps Energy-Efficient Chip-to-Chip Data Link for Ultralow-Power IoT End-Nodes in 65-nm CMOS
[Articolo su rivista]
Okuhara, H.; Elnaqib, A.; Dazzi, M.; Palestri, P.; Benatti, S.; Benini, L.; Rossi, D.
abstract
The increasing complexity of Internet-of-Things (IoT) applications and near-sensor processing algorithms is pushing the computational power of low-power, battery-operated end-node systems. This trend also reveals growing demands for high-speed and energy-efficient inter-chip communications to manage the increasing amount of data coming from off-chip sensors and memories. While traditional microcontroller interfaces such as SPIs cannot cope with tight energy and large bandwidth requirements, low-voltage swing transceivers can tackle this challenge, thanks to their capability to achieve several Gbps of the communication speed at milliwatt power levels. However, recent research on high-speed serial links focused on high-performance systems, with a power consumption significantly larger than the one of low-power IoT end-nodes, or on stand-alone designs not integrated at a system level. This article presents a low-swing transceiver for the energy-efficient and low-power chip-to-chip communication fully integrated within an IoT end-node system-on-chip, fabricated in CMOS 65-nm technology. The transceiver can be easily controlled via a software interface; thus, we can consider realistic scenarios for the data communication, which cannot be assessed in stand-alone prototypes. Chip measurements show that the transceiver achieves $8.46 imes $ higher energy efficiency at $15.9 imes $ higher performance than a traditional microcontroller interface such as a single-SPI.
2021
- A wearable biosensing system with in-sensor adaptive machine learning for hand gesture recognition
[Articolo su rivista]
Moin, A.; Zhou, A.; Rahimi, A.; Menon, A.; Benatti, S.; Alexandrov, G.; Tamakloe, S.; Ting, J.; Yamamoto, N.; Khan, Y.; Burghardt, F.; Benini, L.; Arias, A. C.; Rabaey, J. M.
abstract
Wearable devices that monitor muscle activity based on surface electromyography could be of use in the development of hand gesture recognition applications. Such devices typically use machine-learning models, either locally or externally, for gesture classification. However, most devices with local processing cannot offer training and updating of the machine-learning model during use, resulting in suboptimal performance under practical conditions. Here we report a wearable surface electromyography biosensing system that is based on a screen-printed, conformal electrode array and has in-sensor adaptive learning capabilities. Our system implements a neuro-inspired hyperdimensional computing algorithm locally for real-time gesture classification, as well as model training and updating under variable conditions such as different arm positions and sensor replacement. The system can classify 13 hand gestures with 97.12% accuracy for two participants when training with a single trial per gesture. A high accuracy (92.87%) is preserved on expanding to 21 gestures, and accuracy is recovered by 9.5% by implementing model updates in response to varying conditions, without additional computation on an external device.
2021
- An Ensemble of Hyperdimensional Classifiers: Hardware-Friendly Short-Latency Seizure Detection with Automatic iEEG Electrode Selection
[Articolo su rivista]
Burrello, A.; Benatti, S.; Schindler, K.; Benini, L.; Rahimi, A.
abstract
We propose a new algorithm for detecting epileptic seizures. Our algorithm first extracts three features, namely mean amplitude, line length, and local binary patterns that are fed to an ensemble of classifiers using hyperdimensional (HD) computing. These features are embedded into prototype vectors representing ictal (during seizures) and interictal (between seizures) brain states are constructed. These vectors can be computed at different spatial scales ranging from a single electrode up to many electrodes. This flexibility allows our algorithm to identify the electrodes that discriminate best between ictal and interictal brain states. We assess our algorithm on the SWEC-ETHZ iEEG dataset that includes 99 short-Time iEEG seizures recorded with 36 to 100 electrodes from 16 drug-resistant epilepsy patients. Using $k$-fold cross-validation and all electrodes, our algorithm surpasses state-of-The-Art algorithms yielding significantly shorter latency (8.81 s vs. 11.57 s) in seizure onset detection, and higher specificity (97.31% vs. 94.84%) and accuracy (96.85% vs. 95.42%). We can further reduce the latency of our algorithm to 3.74 s by allowing a slightly higher percentage of false alarms (2% specificity loss). Using only the top 10% of the electrodes ranked by our algorithm, we still maintain superior latency, sensitivity, and specificity compared to the other algorithms with all the electrodes. We finally demonstrate the suitability of our algorithm to deployment on low-cost embedded hardware platforms, thanks to its robustness to noise/artifacts affecting the signal, its low computational complexity, and the small memory-footprint on a RISC-V microcontroller.
2021
- Efficient Artifact Removal from Low-Density Wearable EEG using Artifacts Subspace Reconstruction
[Relazione in Atti di Convegno]
Kumaravel, V. P.; Kartsch, V.; Benatti, S.; Vallortigara, G.; Farella, E.; Buiatti, M.
abstract
Light-weight, minimally-obtrusive mobile EEG systems with a small number of electrodes (i.e., low-density) allow for convenient monitoring of the brain activity in out-of-the-lab conditions. However, they pose a higher risk for signal contamination with non-stereotypical artifacts due to hardware limitations and the challenging environment where signals are collected. A promising solution is Artifacts Subspace Reconstruction (ASR), a component-based approach that can automatically remove non-stationary transient-like artifacts in EEG data. Since ASR has only been validated with high-density systems, it is unclear whether it is equally efficient on low-density portable EEG. This paper presents a complete analysis of ASR performance based on clean and contaminated datasets acquired with BioWolf, an Ultra-Low-Power system featuring only eight channels, during SSVEP sessions recorded from six adults. Empirical results show that even with such few channels, ASR efficiently corrects artifacts, enabling an overall enhancement of up to 40% in SSVEP response. Furthermore, by choosing the optimal ASR parameters on a single-subject basis, SSVEP response can be further increased to more than 45%. These results suggest that ASR is a viable and robust method for online automatic artifact correction with low-density BCI systems in real-life scenarios.
2021
- Efficient Transform Algorithms for Parallel Ultra-Low-Power IoT End Nodes
[Articolo su rivista]
Mazzoni, B.; Benatti, S.; Benini, L.; Tagliavini, G.
abstract
Modern IoT end nodes must support computational intensive workloads at a limited power-budget. Parallel ultra-low-power architectures are a promising target for this scenario, and the availability of highly optimized software libraries is crucial to exploit parallelism and reduce software development costs. This letter proposes an efficient parallel design of the widely used STFT and DWT transforms targeting ultra-low-power IoT devices. We address key performance challenges related to fine-grained synchronization and banking conflicts in shared memory. We achieve high throughput (50.95 samples/μs, on average), good parallel speedup (up to 6.79×), and high energy efficiency (up to 172.55 GOp/s/W) on a cluster of 8 RISC-V cores optimized for parallel ultra-low-power (PULP) operation.
2021
- Embedded Streaming Principal Components Analysis for Network Load Reduction in Structural Health Monitoring
[Articolo su rivista]
Burrello, A.; Marchioni, A.; Brunelli, D.; Benatti, S.; Mangia, M.; Benini, L.
abstract
Principal component analysis (PCA) is a well-established approach commonly used for dimensionality reduction. However, its computational cost and memory requirements hamper the adoption of PCA in heavily resource-constrained embedded platforms. Streaming approaches have been proposed that may enable embedded implementations of the PCA. Among them, the history PCA (HPCA) algorithm stands out for its robustness to the variability in parameters and accuracy. This article presents a parallel and memory-efficient implementation of HPCA in a structural health monitoring (SHM) application based on a heterogeneous network with sensor nodes measuring three-Axial accelerations and gateways collecting measurements from several nodes and sending them to the cloud storage and analytic facility. In the targeted application, standard PCA reaches 15 imes compression factor with an average reconstruction signal-To-noise ratio of 16 dB and a negligible impact on the accuracy in the tracking of structural modal frequencies. By embedding HPCA on our SHM network gateways, we achieve the same compression factor as standard PCA, with more than 1000 imes reduction in data memory footprint for running the algorithm. Furthermore, we parallelize HPCA on the gateway, and we achieve a speedup of 7.1 imes (on 8 cores). Finally, we explore a fixed-point HPCA implementation on sensors (network end nodes), that maximally distributes compression workload, minimizes required communication bandwidth, and maintains the same quality of reconstruction as HPCA in floating point, with a compression factor of 10 imes .
2021
- Guest Editorial: IEEE TC Special Issue on Smart Edge Computing and IoT
[Abstract in Rivista]
Benini, L.; Benatti, S.; Jang, T.; Rahimi, A.
abstract
2021
- Low-latency detection of epileptic seizures from IEEG with temporal convolutional networks on a low-power parallel MCU
[Relazione in Atti di Convegno]
Zanghieri, M.; Burrello, A.; Benatti, S.; Schindler, K.; Benini, L.
abstract
Epilepsy is a severe neurological disorder that affects about 1% of the world population, and one-third of cases are drug-resistant. Apart from surgery, drug-resistant patients can benefit from closed-loop brain stimulation, eliminating or mitigating the epileptic symptoms. For the closed-loop to be accurate and safe, it is paramount to couple stimulation with a detection system able to recognize seizure onset with high sensitivity and specificity and short latency, while meeting the strict computation and energy constraints of always-on real-time monitoring platforms. We propose a novel setup for iEEG-based epilepsy detection, exploiting a Temporal Convolutional Network (TCN) optimized for deployability on low-power edge devices for real-time monitoring. We test our approach on the Short-Term SWEC-ETHZ iEEG Database, containing a total of 100 epileptic seizures from 16 patients (from 2 to 14 per patient) comparing it with the state-of-the-art (SoA) approach, represented by Hyperdimensional Computing (HD). Our TCN attains a detection delay which is 10 s better than SoA, without performance drop in sensitivity and specificity. Contrary to previous literature, we also enforce a time-consistent setup, where training seizures always precede testing seizures chronologically. When deployed on a commercial low-power parallel microcontroller unit (MCU), each inference with our model has a latency of only 5.68 ms and an energy cost of only 124.5 µJ if executed on 1 core, and latency 1.46 ms and an energy cost 51.2 µJ if parallelized on 8 cores. These latency and energy consumption, lower than the current SoA, demonstrates the suitability of our solution for real-time long-term embedded epilepsy monitoring.
2021
- Q-PPG: Energy-Efficient PPG-Based Heart Rate Monitoring on Wearable Devices
[Articolo su rivista]
Burrello, A.; Pagliari, D. J.; Risso, M.; Benatti, S.; Macii, E.; Benini, L.; Poncino, M.
abstract
Hearth Rate (HR) monitoring is increasingly performed in wrist-worn devices using low-cost photoplethysmography (PPG) sensors. However, Motion Artifacts (MAs) caused by movements of the subject's arm affect the performance of PPG-based HR tracking. This is typically addressed coupling the PPG signal with acceleration measurements from an inertial sensor. Unfortunately, most standard approaches of this kind rely on hand-tuned parameters, which impair their generalization capabilities and their applicability to real data in the field. In contrast, methods based on deep learning, despite their better generalization, are considered to be too complex to deploy on wearable devices. In this work, we tackle these limitations, proposing a design space exploration methodology to automatically generate a rich family of deep Temporal Convolutional Networks (TCNs) for HR monitoring, all derived from a single 'seed' model. Our flow involves a cascade of two Neural Architecture Search (NAS) tools and a hardware-friendly quantizer, whose combination yields both highly accurate and extremely lightweight models. When tested on the PPG-Dalia dataset, our most accurate model sets a new state-of-the-art in Mean Absolute Error. Furthermore, we deploy our TCNs on an embedded platform featuring a STM32WB55 microcontroller, demonstrating their suitability for real-time execution. Our most accurate quantized network achieves 4.41 Beats Per Minute (BPM) of Mean Absolute Error (MAE), with an energy consumption of 47.65 mJ and a memory footprint of 412 kB. At the same time, the smallest network that obtains a MAE < 8 BPM, among those generated by our flow, has a memory footprint of 1.9 kB and consumes just 1.79 mJ per inference.
2021
- Robust and energy-efficient PPG-based heart-rate monitoring
[Articolo su rivista]
Risso, M.; Burrello, A.; Pagliari, D. J.; Benatti, S.; Macii, E.; Benini, L.; Poncino, M.
abstract
A wrist-worn PPG sensor coupled with a lightweight algorithm can run on a MCU to enable non-invasive and comfortable monitoring, but ensuring robust PPG-based heart-rate monitoring in the presence of motion artifacts is still an open challenge. Recent state-of-the-art algorithms combine PPG and inertial signals to mitigate the effect of motion artifacts. However, these approaches suffer from limited generality. Moreover, their deployment on MCU-based edge nodes has not been investigated. In this work, we tackle both the aforementioned problems by proposing the use of hardware-friendly Temporal Convolutional Networks (TCN) for PPG-based heart estimation. Starting from a single “seed” TCN, we leverage an automatic Neural Architecture Search (NAS) approach to derive a rich family of models. Among them, we obtain a TCN that outperforms the previous state-of-the-art on the largest PPG dataset available (PPGDalia), achieving a Mean Absolute Error (MAE) of just 3.84 Beats Per Minute (BPM). Furthermore, we tested also a set of smaller yet still accurate (MAE of 5.64 - 6.29 BPM) networks that can be deployed on a commercial MCU (STM32L4) which require as few as 5k parameters and reach a latency of 17.1 ms consuming just 0.21 mJ per inference.
2021
- SEMG-based Regression of Hand Kinematics with Temporal Convolutional Networks on a Low-Power Edge Microcontroller
[Relazione in Atti di Convegno]
Zanghieri, M.; Benatti, S.; Burrello, A.; Kartsch Morinigo, V. J.; Meattini, R.; Palli, G.; Melchiorri, C.; Benini, L.
abstract
Human-Machine Interfaces based on gesture control are a very active field of research, aiming to enable natural interaction with objects. Nowadays, one of the most promising State-of-The-Art (SoA) methodology for robotic hand control relies on the surface electromyographic (sEMG) signal, a non-invasive approach that can provide accurate and intuitive control when coupled with decoding algorithms based on Deep Learning (DL). However, the vast majority of the approaches so far have focused on sEMG classification, producing control systems that limit gestures to a predefined set of positions. In contrast, sEMG regression is still a new field, providing a more natural and complete control method that returns the complete hand kinematics. This work proposes a regression framework based on TEMPONet, a SoA Temporal Convolutional Network (TCN) for sEMG decoding, which we further optimize for deployment. We test our approach on the NinaPro DB8 dataset, targeting the estimation of 5 continuous degrees of freedom for 12 subjects (10 able-bodied and 2 trans-radial amputees) performing a set of 9 contralateral movements. Our model achieves a Mean Absolute Error of 6.89°, which is 0.15° better than the SoA. Our TCN reaches this accuracy with a memory footprint of only 70.9 kB, thanks to int8 quantization. This is remarkable since high-Accuracy SoA neural networks for sEMG can reach sizes up to tens of MB, if deployment-oriented reductions like quantization or pruning are not applied. We deploy our model on the GAP8 edge microcontroller, obtaining 4.76 ms execution latency and an energy cost per inference of 0.243 mJ, showing that our solution is suitable for implementation on resource-constrained devices for real-Time control.
2021
- Tackling time-variability in semg-based gesture recognition with on-device incremental learning and temporal convolutional networks
[Relazione in Atti di Convegno]
Burrello, A.; Zanghieri, M.; Sarti, C.; Ravaglia, L.; Benatti, S.; Benini, L.
abstract
Human-machine interaction is showing promising results for robotic prosthesis control and rehabilitation. In these fields, hand movement recognition via surface electromyographic (sEMG) signals is one of the most promising approaches. However, it still suffers from the issue of sEMG signal's variability over time, which negatively impacts classification robustness. In particular, the non-stationarity of input signals and the surface electrodes' shift can cause up to 30% degradation in gesture recognition accuracy. This work addresses the temporal variability of the sEMG-based gesture recognition by proposing to train a Temporal Convolutional Network (TCN) incrementally over multiple gesture training sessions. Using incremental learning, we re-train our model on stored latent data spanning multiple sessions. We validate our approach on the UniBo-20-Session dataset, which includes 8 hand gestures from 3 subjects. Our incremental learning framework obtains 18.9% higher accuracy compared to a baseline with a standard single training session. Deploying our TCN on a Parallel, Ultra-Low Power (PULP) microcontroller unit (MCU), GAP8, we achieve an inference latency and energy of 12.9 ms and 0.66 mJ, respectively, with a weight memory footprint of 427 kB and a data memory footprint of 0.5-32 MB.
2021
- Towards Long-term Non-invasive Monitoring for Epilepsy via Wearable EEG Devices
[Relazione in Atti di Convegno]
Ingolfsson, T. M.; Cossettini, A.; Wang, X.; Tabanelli, E.; Tagliavini, G.; Ryvlin, P.; Benini, L.; Benatti, S.
abstract
We present the implementation of seizure detection algorithms based on a minimal number of EEG channels on a parallel ultra-low-power embedded platform. The analyses are based on the CHB-MIT dataset, and include explorations of different classification approaches (Support Vector Machines, Random Forest, Extra Trees, AdaBoost) and different pre/post-processing techniques to maximize sensitivity while guaranteeing no false alarms. We analyze global and subject-specific approaches, considering all 23-electrodes or only 4 temporal channels. For 8 s window size and subject-specific approach, we report zero false positives and 100% sensitivity. These algorithms are parallelized and optimized for a parallel ultra-low power (PULP) platform, enabling 300h of continuous monitoring on a 300 mAh battery, in a wearable form factor and power budget. These results pave the way for the implementation of affordable, wearable, long-term epilepsy monitoring solutions with low false-positive rates and high sensitivity, meeting both patient and caregiver requirements.
2021
- UStEMG: an Ultrasound Transparent Tattoo-based sEMG System for Unobtrusive Parallel Acquisitions of Muscle Electro-mechanics
[Relazione in Atti di Convegno]
Leitner, C.; Benatti, S.; Keller, K.; Cossettini, A.; Kartsch, V.; Penasso, H.; Benini, L.; Greco, F.; Baumgartner, C.
abstract
Human machine interfaces follow machine learning approaches to interpret muscles states, mainly from electrical signals. These signals are easy to collect with tiny devices, on tight power budgets, interfaced closely to the human skin. However, natural movement behavior is not only determined by muscle activation, but it depends on an orchestration of several subsystems, including the instantaneous length of muscle fibers, typically inspected by means of ultrasound (US) imaging systems. This work shows for the first time an ultra-lightweight (7 g) electromyography (sEMG) system transparent to ultrasound, which enables the simultaneous acquisition of sEMG and US signals from the same location. The system is based on ultrathin and skin-conformable temporary tattoo electrodes (TTE) made of printed conducting polymer, connected to a tiny, parallel-ultra-low power acquisition platform (BioWolf). US phantom images recorded with the TTE had mean axial and lateral resolutions of 0.90±0.02 mm and 1.058±0.005 mm, respectively. The root mean squares for sEMG signals recorded with the US during biceps contractions were at 57±10 µV and mean frequencies were at 92±1 Hz. We show that neither ultrasound images nor electromyographic signals are significantly altered during parallel and synchronized operation.Clinical relevance- Modern prosthetic engineering concepts use interfaces connected to muscles or nerves and employ machine learning models to infer on natural movement behavior of amputated limbs. However, relying only on a single data source (e.g., electromyography) reduces the quality of a fine-grained motor control. To address this limitation, we propose a new and unobtrusive device capable of capturing the electrical and mechanical behavior of muscles in a parallel and synchronized fashion. This device can support the development of new prosthetic control and design concepts, further supporting clinical movement science in the configuration of better simulation models.
2020
- A cost-effective embedded platform for scalable multichannel biopotential acquisition
[Relazione in Atti di Convegno]
Benatti, S.; Guermandi, M.; Benini, L.
abstract
2020
- A wearable device for brain–machine interaction with augmented reality head-mounted display
[Relazione in Atti di Convegno]
Salvaro, M.; Benatti, S.; Kartsch, V.; Guermandi, M.; Benini, L.
abstract
2020
- An Open-Source Scalable Thermal and Power Controller for HPC Processors
[Relazione in Atti di Convegno]
Bambini, G.; Balas, R.; Conficoni, C.; Tilli, A.; Benini, L.; Benatti, S.; Bartolini, A.
abstract
In the last decade, high performance multi-core processor designs have followed an increase in number of cores, interfaces, heterogeneity and System-on-chip (SoC) complexity. HPC applications also require tailored chip designs with specific operating points and performance indexes. In this scenario, an advanced and configurable Power Controller System (PCS) is necessary to meet power and thermal constraints, without the necessity of static ultra-conservative margins on the operating points. In this paper, we propose an open-source PCS design, based on a parallel ultra-low power microcontroller with RISC-V cores, and an open-source software environment based on a Real-time operating system (RTOS) with a configurable Power-thermal control algorithm. Considering a 1ms control interval, the overhead of the RTOS is about 6% of the cycles in the nominal case. The control algorithm is able to limit temperature and power consumption within given bounds, while maximizing performance. The PCS is able to control up to 76 different cores/computing units with headroom for larger core counts.
2020
- Modular Design and Optimization of Biomedical Applications for Ultralow Power Heterogeneous Platforms
[Articolo su rivista]
De Giovanni, E.; Montagna, F.; Denkinger, B. W.; Machetti, S.; Peon-Quiros, M.; Benatti, S.; Rossi, D.; Benini, L.; Atienza, D.
abstract
In the last years, remote health monitoring is becoming an essential branch of health care with the rapid development of wearable sensors technology. To meet the demand of new more complex applications and ensuring adequate battery lifetime, wearable sensors have evolved into multicore systems with advanced power-saving capabilities and additional heterogeneous components. In this article, we present an approach that applies optimization and parallelization techniques uncovered by modern ultralow power (ULP) platforms in the SW layers with the goal of improving the mapping and reducing the energy consumption of biomedical applications. Additionally, we investigate the benefit of integrating domain-specific accelerators to further reduce the energy consumption of the most computationally expensive kernels. Using 30-s excerpts of signals from two public databases, we apply the proposed optimization techniques on well-known modules of biomedical benchmarks from the state-of-the-art and two complete applications. We observe speed-ups of $5.17{ imes }$ and energy savings of 41.6% for the multicore implementation using a cluster of 8 cores with respect to single-core wearable sensor designs when processing a standard 12-lead electrocardiogram (ECG) signal analysis. Additionally, we conclude that the minimum workload required to take advantage of parallelization for a heartbeat classifier corresponds to the processing of 3-lead ECG signals, with a speed-up of $2.96{ imes }$ and energy savings of 19.3%. Moreover, we observe additional energy savings of up to 7.75% and 16.8% by applying power management and memory scaling to the multicore implementation of the 3-lead beat classifier and 12-lead ECG analysis, respectively. Finally, by integrating hardware (HW) acceleration we observe overall energy savings of up to 51.3% for the 12-lead ECG analysis.
2020
- Neuro-PULP: A Paradigm Shift Towards Fully Programmable Platforms for Neural Interfaces
[Relazione in Atti di Convegno]
Schiavone, P. D.; Rossi, D.; Liu, Y.; Benatti, S.; Luan, S.; Williams, I.; Benini, L.; Constandinou, T.
abstract
Designing systems with many recording channels is a major challenge in brain-machine interfaces. Power, bandwidth, and size requirements impose tight design constraints for implementing the required processing within an acceptable latency and battery life. Moreover, the variety of brain decoding algorithms require highly versatile systems that can be rapidly adapted to execute different tasks from experiment to experiment as for example microcontrollers (MCUs). However, state of the art MCUs lack of performance and consume too much power to be used as generic platforms for neural decoding applications. To overcome the aforementioned limitations, this paper presents an MCU-based system consisting of a 64-channel event-based neural interface and a Parallel Ultra-Low-Power (PULP) platform that acquires and processes the neural activity. The flexibility of the system (called Neuro-PULP) has been demonstrated through the deployment of two applications: one compressing raw data in streaming mode for wireless transmission, and one generating the cluster and time-stamp of detected spikes, leveraging a low-power event-mode. The event-based approach, coupled with the energy efficiency of the PULP architecture, leads to a more than 4x improvement in energy efficiency with respect to state of the art systems based on FPGAs, leading to the average power consumption of 114μ W /channel, yet retaining the flexibility of fully programmable processor-based architectures.
2020
- Robust Real-Time Embedded EMG Recognition Framework Using Temporal Convolutional Networks on a Multicore IoT Processor
[Articolo su rivista]
Zanghieri, M.; Benatti, S.; Burrello, A.; Kartsch, V.; Conti, F.; Benini, L.
abstract
Hand movement classification via surface electromyographic (sEMG) signal is a well-established approach for advanced Human-Computer Interaction. However, sEMG movement recognition has to deal with the long-Term reliability of sEMG-based control, limited by the variability affecting the sEMG signal. Embedded solutions are affected by a recognition accuracy drop over time that makes them unsuitable for reliable gesture controller design. In this paper, we present a complete wearable-class embedded system for robust sEMG-based gesture recognition, based on Temporal Convolutional Networks (TCNs). Firstly, we developed a novel TCN topology (TEMPONet), and we tested our solution on a benchmark dataset (Ninapro), achieving 49.6% average accuracy, 7.8%, better than current State-Of-The-Art (SoA). Moreover, we designed an energy-efficient embedded platform based on GAP8, a novel 8-core IoT processor. Using our embedded platform, we collected a second 20-sessions dataset to validate the system on a setup which is representative of the final deployment. We obtain 93.7% average accuracy with the TCN, comparable with a SoA SVM approach (91.1%). Finally, we profiled the performance of the network implemented on GAP8 by using an 8-bit quantization strategy to fit the memory constraint of the processor. We reach a mathbf {4 imes } lower memory footprint (460 kB) with a performance degradation of only 3% accuracy. We detailed the execution on the GAP8 platform, showing that the quantized network executes a single classification in 12.84 ms with a power envelope of 0.9 mJ, making it suitable for a long-lifetime wearable deployment.
2020
- Scalable and energy efficient seizure detection based on direct use of compressively-sensed EEG data on an ultra low power multi-core architecture
[Articolo su rivista]
Aghazadeh, R.; Frounchi, J.; Montagna, F.; Benatti, S.
abstract
Extracting information from dense multi-channel neural sensors for accurate diagnosis of brain disorders necessitates computationally expensive and advanced signal processing approaches to analyze the massive volume of recorded data. Compressive Sensing (CS) is an efficient method for reducing the computational complexity and power consumption in the resource-constrained multi-site neural systems. However, reconstructing the signal from compressed measurements is computationally intensive, making it unsuitable for real-time applications such as seizure detection. In this paper, a seizure detection algorithm is proposed to overcome these limitations by circumventing the reconstruction phase and directly processing the compressively sampled EEG signals. The Lomb-Scargle Periodogram (LSP) is used to extract the spectral energy features of the compressed data. Performance of the seizure detector using non-linear support vector machine (SVM) classifier, tested on 24 patients of the CHB-MIT data-set for compression ratios (CR) of 1–64x, is 96–93%, 92-87%, 0.95–0.91, and <1 s for sensitivity, accuracy, the area under the curve, and latency, respectively. A power-efficient classification method based on the utilization of dual linear SVM classifiers is proposed. The proposed classification method based on the dual linear SVM classification achieved better classification performance compared to commonly used classifiers, such as K-nearest neighbor, random forest, artificial neural network, and linear SVM, while consuming low power in comparison to non-linear SVM kernels. The hardware-optimized implementation of this algorithm is proposed on a low-power multi-core SoC for near-sensor data analytics: Mr. Wolf. Optimized implementation of this algorithm on Mr. Wolf platform leads to detecting a seizure with an energy budget of 18.4 μJ and 3.9 μJ for a compression ratio of 24x using non-linear SVM classifier and the dual linear SVM based classification method, respectively.
2020
- Temporal Variability Analysis in sEMG Hand Grasp Recognition using Temporal Convolutional Networks
[Relazione in Atti di Convegno]
Zanghieri, M.; Benatti, S.; Conti, F.; Burrello, A.; Benini, L.
abstract
Hand movement recognition via surface electromyographic (sEMG) signal is a promising approach for the advance in Human-Computer Interaction. However, this field has to deal with two main issues: (1) the long-term reliability of sEMG-based control is limited by the variability affecting the sEMG signal (especially, variability over time); (2) the classification algorithms need to be suitable for implementation on embedded devices, which have strict constraints in terms of power budget and computational resources. Current solutions present a performance over-time drop that makes them unsuitable for reliable gesture controller design. In this paper, we address temporal variability of sEMG-based grasp recognition, proposing a new approach based on Temporal Convolutional Networks, a class of deep learning algorithms particularly suited for time series analysis and temporal pattern recognition. Our approach improves by 7.6% the best results achieved in the literature on the NinaPro DB6, a reference dataset for temporal variability analysis of sEMG. Moreover, when targeting the much more challenging inter-session accuracy objective, our method achieves an accuracy drop of just 4.8% between intra- and inter-session validation. This proves the suitability of our setup for a robust, reliable long-term implementation. Furthermore, we distill the network using deep network quantization and pruning techniques, demonstrating that our approach can use down to 120 lower memory footprint than the initial network and 4 lower memory footprint than a baseline Support Vector Machine, with an inter-session accuracy degradation of only 2.5%, proving that the solution is suitable for embedded resource-constrained implementations.
2020
- Using Low-Power, Low-Cost IoT Processors in Clinical Biosignal Research: An In-depth Feasibility Check
[Relazione in Atti di Convegno]
Kartsch, V.; Artoni, F.; Benatti, S.; Micera, S.; Benini, L.
abstract
Research on biosignal (ExG) analysis is usually performed with expensive systems requiring connection with external computers for data processing. Consumer-grade low-cost wearable systems for bio-potential monitoring and embedded processing have been presented recently, but are not considered suitable for medical-grade analyses. This work presents a detailed quantitative comparative analysis of a recently presented fully-wearable low-power and low-cost platform (BioWolf) for ExG acquisition and embedded processing with two researchgrade acquisition systems, namely, ANTNeuro (EEG) and the Noraxon DTS (EMG). Our preliminary results demonstrate that BioWolf offers competitive performance in terms of electrical properties and classification accuracy. This paper also highlights distinctive features of BioWolf, such as real-time embedded processing, improved wearability, and energy-efficiency, which allows devising new types of experiments and usage scenarios for medical-grade biosignal processing in research and future clinical studies.
2019
- A Minimally Invasive Low-Power Platform for Real-Time Brain Computer Interaction Based on Canonical Correlation Analysis
[Articolo su rivista]
Salvaro, M.; Benatti, S.; Kartsch, V. J.; Guermandi, M.; Benini, L.
abstract
A growing trend in human-computer interaction is to integrate computational capabilities into wearable devices, to enable sophisticated and natural interaction modalities. Acting directly by decoding neural activity is a very natural way of interaction and one of the fundamental paradigms of brain computer interfaces (BCIs) as well. In this paper, we present a wearable Internet of Things node designed for BCI spelling. The system is based on visual evoked potentials detection and runs the canonical correlation analysis on a low power microcontroller. Neural data is acquired by an array of electroencephalography active dry electrodes, suitable for a minimally intrusive interface. To evaluate our solution, we optimized the system on eight subjects and tested it on five different subjects for four and eight stimuli, reaching a peak transfer rate of 1.57 b/s, comparable with those achieved by state-of-the-art nonembedded systems. The power consumption of the device is less than 30 mW, resulting in 122 h of operation with a standard 1000-mAh battery.
2019
- A PULP-based parallel power controller for future exascale systems
[Relazione in Atti di Convegno]
Bartolini, A.; Rossi, D.; Mastrandrea, A.; Conficoni, C.; Benatti, S.; Tilli, A.; Benini, L.
abstract
Power management of digital circuits is raising of importance in a broad spectrum of computing domains. High-performance computing systems as the effect of the stop of Dennard's scaling have become power and thermal limited. In this manuscript, we evaluate the feasibility of using an open-source RISC-V based power controller for the high-performance computing market.
2019
- An Energy-Efficient IoT node for HMI applications based on an ultra-low power Multicore Processor
[Relazione in Atti di Convegno]
Kartsch, V.; Guermandi, M.; Benatti, S.; Montagna, F.; Benini, L.
abstract
Developing wearable sensing technologies and unobtrusive devices is paving the way to the design of compelling applications for the next generation of systems for a smart IoT node for Human Machine Interaction (HMI). In this paper we present a smart sensor node for IoT and HMI based on a programmable Parallel Ultra-Low-Power (PULP) platform. We tested the system on a hand gesture recognition application, which is a preferred way of interaction in HMI design. A wearable armband with 8 EMG sensors is controlled by our IoT node, running a machine learning algorithm in real-time, recognizing up to 11 gestures with a power envelope of 11.84 mW. As a result, the proposed approach is capable to 35 hours of continuous operation and 1000 hours in standby. The resulting platform minimizes effectively the power required to run the software application and thus, it allows more power budget for high-quality AFE.
2019
- Analysis of Contraction Effort Level in EMG-Based Gesture Recognition Using Hyperdimensional Computing
[Relazione in Atti di Convegno]
Moin, A.; Zhou, A.; Benatti, S.; Rahimi, A.; Benini, L.; Rabaey, J. M.
abstract
Varying contraction levels of muscles is a big challenge in electromyography-based gesture recognition. Some use cases require the classifier to be robust against varying force changes, while others demand to distinguish between different effort levels of performing the same gesture. We use brain-inspired hyperdimensional computing paradigm to build classification models that are both robust to these variations and able to recognize multiple contraction levels. Experimental results on 5 subjects performing 9 gestures with 3 effort levels show up to 39.17% accuracy drop when training and testing across different effort levels, with up to 30.35% recovery after applying our algorithm.
2019
- BioWolf: A Sub-10-mW 8-Channel Advanced Brain-Computer Interface Platform with a Nine-Core Processor and BLE Connectivity
[Articolo su rivista]
Kartsch, V.; Tagliavini, G.; Guermandi, M.; Benatti, S.; Rossi, D.; Benini, L.
abstract
Advancements in digital signal processing (DSP) and machine learning techniques have boosted the popularity of brain-computer interfaces (BCIs), where electroencephalography is a widely accepted method to enable intuitive human-machine interaction. Nevertheless, the evolution of such interfaces is currently hampered by the unavailability of embedded platforms capable of delivering the required computational power at high energy efficiency and allowing for a small and unobtrusive form factor. To fill this gap, we developed BioWolf, a highly wearable (40 mm × 20 mm × 2 mm) BCI platform based on Mr. Wolf, a parallel ultra low power system-on-chip featuring nine RISC-V cores with DSP-oriented instruction set extensions. BioWolf also integrates a commercial 8-channel medical-grade analog-to-digital converter, and an ARM-Cortex M4 microcontroller unit (MCU) with bluetooth low-energy connectivity. To demonstrate the capabilities of the system, we implemented and tested a BCI featuring canonical correlation analysis (CCA) of steady-state visual evoked potentials. The system achieves an average information transfer rate of 1.46 b/s (aligned with the state-of-the-art of bench-top systems). Thanks to the reduced power envelope of the digital computational platform, which consumes less than the analog front-end, the total power budget is just 6.31 mW, providing up to 38 h operation (65 mAh battery). To our knowledge, our design is the first to explore the significant energy boost of a parallel MCU with respect to single-core MCUs for CCA-based BCI.
2019
- Online Learning and Classification of EMG-Based Gestures on a Parallel Ultra-Low Power Platform Using Hyperdimensional Computing
[Articolo su rivista]
Benatti, S.; Montagna, F.; Kartsch, V.; Rahimi, A.; Rossi, D.; Benini, L.
abstract
This paper presents a wearable electromyographic gesture recognition system based on the hyperdimensional computing paradigm, running on a programmable parallel ultra-low-power (PULP) platform. The processing chain includes efficient on-chip training, which leads to a fully embedded implementation with no need to perform any offline training on a personal computer. The proposed solution has been tested on 10 subjects in a typical gesture recognition scenario achieving 85% average accuracy on 11 gestures recognition, which is aligned with the state-of-the-art, with the unique capability of performing online learning. Furthermore, by virtue of the hardware friendly algorithm and of the efficient PULP system-on-chip (Mr. Wolf) used for prototyping and evaluation, the energy budget required to run the learning part with 11 gestures is 10.04 mJ, and 83.2 muJ per classification. The system works with a average power consumption of 10.4 mW in classification, ensuring around 29 h of autonomy with a 100 mAh battery. Finally, the scalability of the system is explored by increasing the number of channels (up to 256 electrodes), demonstrating the suitability of our approach as universal, energy-efficient biopotential wearable recognition framework.
2019
- Towards a wearable interface for food quality grading through ERP analysis
[Articolo su rivista]
Guermandi, M.; Benatti, S.; Brunelli, D.; Kartsch, V.; Benini, L.
abstract
Sensory evaluation is used to assess the consumer acceptance of foods or other consumer products, so as to improve industrial processes and marketing strategies. The procedures currently involved are time-consuming because they require a statistical approach from measurements and feedback reports from a wide set of evaluators under a well-established measurement setup. In this paper, we propose to collect directly the signal of the perceived quality of the food from Event-related potentials (ERPs) that are the outcome of the processing of visual stimuli. This permits to narrow the number of evaluators since errors related to psychological factors are by-passed. We present the design of a wearable system for ERP measurement and we present preliminary results on the use of ERP to give a quantitative measure to the appearance of a food product. The system is developed to be wearable and our experiments demonstrate that is possible to use it to identify and classify the grade of acceptance of the food.
2019
- Towards versatile fast training for wearable interfaces in prosthetics
[Capitolo/Saggio]
Benatti, S.; Montagna, F.; Kartsch, V.; Rahimi, A.; Benini, L.
abstract
Developing embedded systems tailored for resource-constrained platforms enables the design of robust frameworks for controlling artificial arms in prosthetic applications. This work presents preliminary results of the implementation of a novel platform for EMG-based gesture recognition application based on Hyper dimensional Computing (HDC), a novel brain-inspired classifier. HDC reaches classification accuracy comparable with traditional statistical learning algorithms, while its training phase is one order of magnitude faster, resulting suitable for the implementation on low-power and low-cost digital platforms. The proposed setup acquires EMG data from 8 sensors, performs training in 1.2 s on the embedded microcontroller and classifies 5 gestures with 88% accuracy, a latency of 10ms and energy consumption of just 0.65 mJ per classification.
2019
- Ultra Low-Power Drowsiness Detection System with BioWolf
[Relazione in Atti di Convegno]
Kartsch, V.; Benatti, S.; Guermandi, M.; Montagna, F.; Benini, L.
abstract
Drowsiness is a cause of accidents in industrial and mining activities. A considerable amount of effort has been put into the detection of drowsiness, and since then it has been integrated into a large variety of wearable systems. Nevertheless, the technology still suffers from high intrusiveness, short battery life and lack of generality. An opportunity to address these shortcomings arises from the use of physiological and behavioral features for bio-signals like EEG and IMU sensors. In this work, we propose an energy-efficient wearable platform for drowsiness detection. Our platform features a minimally invasive setup, based on dry EEG sensors to acquire neural data, and Mr. Wolf, an 8-core ultra-low-power digital platform. The system has been validated on three test subjects, achieving detection accuracy of 83%, using a Nearest Centroid Classifier, modeled with a semi-supervised algorithm from previously collected data. This work further extends the capabilities of our previous system, providing a more sophisticated classification mechanism that includes real-time and onboard sensor fusion processing while running into a highly efficient and unobtrusive hardware platform, outperforming the current State of the Art (SoA) in terms of wearability and battery lifetime.
2018
- A Wearable Device for Minimally-Invasive Behind-the-Ear EEG and Evoked Potentials
[Relazione in Atti di Convegno]
Guermandi, M.; Benatti, S.; Kartsch Morinigo, V. J.; Bertini, L.
abstract
We present an unobtrusive and minimally invasive system for acquiring 8-channels of EEG behind the ear, to support brain activity monitoring in a socially acceptable fashion and outside clinical environments. Electrical performance are in line with those of clinical EEG systems (0.48 μV RMS integrated noise in the [0-100] Hz band, 106 dB CMRR) and setup is extremely fast, requiring only a mild cleaning of the skin before applying the device. The system can acquire typical brain activity rhythms and potentials evoked by sensory stimuli, which are at the basis of BCI systems. Current consumption is 6.2 rnA, plus 16 mA for Bluetooth® data transmission (4.5 hours life on a 100 mAh battery). The overall cost of components is below 50 USD.
2018
- A sensor fusion approach for drowsiness detection in wearable ultra-low-power systems
[Articolo su rivista]
Kartsch, V. J.; Benatti, S.; Schiavone, P. D.; Rossi, D.; Benini, L.
abstract
Drowsiness detection mechanisms have been extensively studied in the last years since they are one of the prevalent causes of accidents within the mining, driving and industrial activities. Many research efforts were done to quantify the drowsiness levels using behavioral analyses based on camera eye tracking systems as well as by analyzing physiological features contained in EEG signals. Detection systems typically use specific drowsiness indicators from only one of these methods, leaving a risk of missed detection since not all the population presents same symptoms of drowsiness. Thus, multi-feature systems are preferable even though most of the current State-of-the-Art (SoA) solutions are based on power-hungry platforms and they have meager chance to be used in embedded wearable applications with long battery lifetime. This work presents a drowsiness detection scheme fusing behavioral information coming from user motion through an IMU sensor and physiological information coming from brain activity through a single EEG electrode. The solution is implemented and tested on a low power programmable platform based on an ARM Cortex-M4 microcontroller, resulting in a wearable device capable to detect 5 different levels of drowsiness with an average accuracy of 95.2% and a battery life of 6 hours, using a 200 mAh battery. We also study the energy optimization achievable by accelerating the sensor fusion-based drowsiness detector on a parallel ultra-low power (PULP) platform. Results show that the use of PULP as efficient processing platform provides an energy improvement of 63x with respect to a solution based on a commercial microcontroller. This may extend the battery life of the complete system up to 46 h with a 7x improvement, paving the way for a completely wearable, always-on system.
2018
- An EMG Gesture Recognition System with Flexible High-Density Sensors and Brain-Inspired High-Dimensional Classifier
[Articolo su rivista]
Moin, A.; Zhou, A.; Rahimi, A.; Benatti, S.; Menon, A.; Tamakloe, S.; Ting, J.; Yamamoto, N.; Khan, Y.; Burghardt, F.; Benini, L.; Arias, A. C.; Rabaey, J. M.
abstract
EMG-based gesture recognition shows promise for human-machine interaction. Systems are often afflicted by signal and electrode variability which degrades performance over time. We present an end-to-end system combating this variability using a large-area, high-density sensor array and a robust classification algorithm. EMG electrodes are fabricated on a flexible substrate and interfaced to a custom wireless device for 64-channel signal acquisition and streaming. We use brain-inspired high-dimensional (HD) computing for processing EMG features in one-shot learning. The HD algorithm is tolerant to noise and electrode misplacement and can quickly learn from few gestures without gradient descent or back-propagation. We achieve an average classification accuracy of 96.64% for five gestures, with only 7% degradation when training and testing across different days. Our system maintains this accuracy when trained with only three trials of gestures; it also demonstrates comparable accuracy with the state-of-the-art when trained with one trial.
2018
- An sEMG-Based Human-Robot Interface for Robotic Hands Using Machine Learning and Synergies
[Articolo su rivista]
Meattini, R.; Benatti, S.; Scarcia, U.; De Gregorio, D.; Benini, L.; Melchiorri, C.
abstract
Developing natural control strategies represents an intriguing challenge in the design of human-robot interface (HRI) systems. The teleoperation of robotic grasping devices, especially in industrial, rescue, and aerospace applications, is mostly based on nonintuitive approaches, such as remote controllers. On the other hand, recent research efforts target solutions that mimic the human ability to manage multifinger grasps and finely modulate grasp impedance. Since electromyography (EMG) contains information about human motion control, it is possible to leverage such neuromuscular knowledge to teleoperate robotic hands for grasping tasks. In this paper, we present an HRI system based on eight fully differential EMG sensors connected to a wearable sensor node for acquisition and processing. By virtue of a novel bio-inspired approach, the embedded myocontroller merges pattern recognition and factorization techniques to combine a natural selection of the robotic hand configuration with the proportional control of the related grasps. The HRI system has been fully designed, implemented, and tested on two robotic hands: a dexterous anthropomorphic hand and a three-fingered industrial gripper mounted on a robotic manipulator. The results of the test performed on four able-bodied subjects show success rates greater than 90% reached in grasping objects that require different hand shapes and impedance regulations for the task completion. The outcomes also show that the users modulate the bio-inspired degrees of control in a natural manner, proving the pertinence of the proposed system for an effective human-like control of robotic grasping devices in a wearable form factor.
2018
- Compressed sensing based seizure detection for an ultra low power multi-core architecture
[Relazione in Atti di Convegno]
Aghazadeh, R.; Montagna, F.; Benatti, S.; Rossi, D.; Frounchi, J.
abstract
Extracting information from brain signals in advanced Brain Machine Interfaces (BMI) often requires computationally demanding processing. The complexity of the algorithms traditionally employed to process multi-channel neural data, such as Principal Component Analysis (PCA), dramatically increases while scaling-up the number of channels and requires more power-hungry computational platforms. This could hinder the development of low-cost and low-power interfaces which can be used in wearable or implantable real-Time systems. This work proposes a new algorithm for the detection of epileptic seizure based on compressively sensed EEG information, and its optimization on a low-power multi-core SoC for near-sensor data analytics: Mr. Wolf. With respect to traditional algorithms based on PCA, the proposed approach reduces the computational complexity by 4.4x in ARM Cortex M4-based MCU. Implementing this algorithm on Mr.Wolf platform allows to detect a seizure with 1 ms of latency after acquiring the EEG data for 1 s, within an energy budget of 18.4 μJ. A comparison with the same algorithm on a commercial MCU shows an improvement of 6.9x in performance and up to 18.4x in terms of energy efficiency.
2018
- Exploring Arm Posture and Temporal Variability in Myoelectric Hand Gesture Recognition
[Relazione in Atti di Convegno]
Milosevic, B.; Farella, E.; Benatti, S.
abstract
Hand gesture recognition based on myoelectric (EMG) signals is an innovative approach for the development of intuitive interaction devices, ranging from poliarticulated prosthetic hands to intuitive robot and mobile interfaces. Their study and development in controlled environments provides promising results, but effective real-world adoption is still limited due to reliability problems, such as motion artifacts and arm posture, temporal variability and issues caused by the re-positioning of sensors at each use. In this work, we present an EMG dataset collected with the aim to explore postural and temporal variability in the recognition of arm gestures. Its collection of gestures executed in 4 arm postures over 8 days allows to evaluate the impact of such variability on classification performance. We implemented and tested State-of-the-Art (SoA) recognition approaches analyzing the impact of different training strategies. Moreover, we compared the computational and memory requirements of the considered algorithms, providing an additional evaluation criteria useful for real-time implementation. Results show a decrease in the recognition of inter-posture and inter-day gestures up to 20%. The provided dataset will allow further exploration of such effects and the development of effective training and recognition strategies.
2018
- PULP-HD: Accelerating brain-inspired high-dimensional computing on a parallel ultra-low power platform
[Relazione in Atti di Convegno]
Montagna, F.; Rahimi, A.; Benatti, S.; Rossi, D.; Benini, L.
abstract
Computing with high-dimensional (HD) vectors, also referred to as hypervectors, is a brain-inspired alternative to computing with scalars. Key properties of HD computing include a well-defined set of arithmetic operations on hypervectors, generality, scalability, robustness, fast learning, and ubiquitous parallel operations. HD computing is about manipulating and comparing large patterns-binary hypervectors with 10,000 dimensions-making its efficient realization on minimalistic ultra-low-power platforms challenging. This paper describes HD computing's acceleration and its optimization of memory accesses and operations on a silicon prototype of the PULPv3 4-core platform (1.5mm2, 2 mW), surpassing the stateof-the-art classification accuracy (on average 92.4%) with simultaneous 3.7× end-to-end speed-up and 2× energy saving compared to its single-core execution. We further explore the scalability of our accelerator by increasing the number of inputs and classification window on a new generation of the PULP architecture featuring bitmanipulation instruction extensions and larger number of 8 cores. These together enable a near ideal speed-up of 18.4× compared to the single-core PULPv3.
2018
- Smart Wearable Wristband for EMG based Gesture Recognition Powered by Solar Energy Harvester
[Articolo su rivista]
Kartsch, V.; Benatti, S.; Mancini, M.; Magno, M.; Benini, L.
abstract
With the recent improvement of flexible electronics, wearable systems are becoming more and more unobtrusive and comfortable, pervading fitness and health-care applications. Wearable devices allow non-invasive monitoring of vital signs and physiological parameters, enabling advanced Human Machine Interaction (HMI) as well. On the other hand, battery lifetime remains a challenge especially when they are equipped with bio-medical sensors and not used as simple data logger. In this paper, we present a flexible wristband for EMG gesture recognition, designed on a flexible Printed Circuit Board (PCB) strip and powered by a small form-factor flexible solar energy panel. The proposed wristband executes a Support Vector Machine (SVM) algorithm reaching 94.02 % accuracy in recognition of 5 hand gestures. The system targets healthcare and HMI applications, and can be used to monitor patients during rehabilitation from stroke and neural traumas as well as to enable a simple gesture control interface (e.g. for smart-watches). Experimental results show the accuracy achieved by the algorithm and the lifetime of the device. By virtue of the low power consumption of the proposed solution and the on-board processing that limits the radio activity, the wristband achieves more than 500 hours with a single 200 mAh battery, and perpetual work with a small-form factor flexible solar panel.
2017
- A contactless, energy-neutral power meter for smart city applications
[Relazione in Atti di Convegno]
Villani, C.; Benatti, S.; Brunelli, D.; Benini, L.
abstract
Electrical energy management is fundamental to optimize the generation and usage of power within a Smart Grid; and the measurement of parameters of electrical systems is crucial for achieving efficient control on electric loads. Most of the existing smart metering devices use voltage probes which are invasive, because they need a direct connection to the electric conductor. We present an innovative three-phase clamp-on power meter that can be installed without breaking the circuit under measurement and without temporary interruption of the supply. It is used tight around the cable insulators and it measures both current and voltage of the underneath wire. Moreover, it enables the use of advanced signal processing algorithms such as Non-Intrusive Load Monitoring (NILM), which provides fine grain load detection even if a single point of measurement is used.
2017
- A machine learning approach for automated wide-range frequency tagging analysis in embedded neuromonitoring systems
[Articolo su rivista]
Montagna, F.; Buiatti, M.; Benatti, S.; Rossi, D.; Farella, E.; Benini, L.
abstract
EEG is a standard non-invasive technique used in neural disease diagnostics and neurosciences. Frequency-tagging is an increasingly popular experimental paradigm that efficiently tests brain function by measuring EEG responses to periodic stimulation. Recently, frequency-tagging paradigms have proven successful with low stimulation frequencies (0.5–6 Hz), but the EEG signal is intrinsically noisy in this frequency range, requiring heavy signal processing and significant human intervention for response estimation. This limits the possibility to process the EEG on resource-constrained systems and to design smart EEG based devices for automated diagnostic. We propose an algorithm for artifact removal and automated detection of frequency tagging responses in a wide range of stimulation frequencies, which we test on a visual stimulation protocol. The algorithm is rooted on machine learning based pattern recognition techniques and it is tailored for a new generation parallel ultra low power processing platform (PULP), reaching performance of more that 90% accuracy in the frequency detection even for very low stimulation frequencies (<1 Hz) with a power budget of 56 mW.
2017
- A prosthetic hand body area controller based on efficient pattern recognition control strategies
[Articolo su rivista]
Benatti, S.; Milosevic, B.; Farella, E.; Gruppioni, E.; Benini, L.
abstract
Poliarticulated prosthetic hands represent a powerful tool to restore functionality and improve quality of life for upper limb amputees. Such devices offer, on the same wearable node, sensing and actuation capabilities, which are not equally supported by natural interaction and control strategies. The control in state-of-the-art solutions is still performed mainly through complex encoding of gestures in bursts of contractions of the residual forearm muscles, resulting in a non-intuitive Human-Machine Interface (HMI). Recent research efforts explore the use of myoelectric gesture recognition for innovative interaction solutions, however there persists a considerable gap between research evaluation and implementation into successful complete systems. In this paper, we present the design of a wearable prosthetic hand controller, based on intuitive gesture recognition and a custom control strategy. The wearable node directly actuates a poliarticulated hand and wirelessly interacts with a personal gateway (i.e., a smartphone) for the training and personalization of the recognition algorithm. Through the whole system development, we address the challenge of integrating an efficient embedded gesture classifier with a control strategy tailored for an intuitive interaction between the user and the prosthesis. We demonstrate that this combined approach outperforms systems based on mere pattern recognition, since they target the accuracy of a classification algorithm rather than the control of a gesture. The system was fully implemented, tested on healthy and amputee subjects and compared against benchmark repositories. The proposed approach achieves an error rate of 1.6% in the end-to-end real time control of commonly used hand gestures, while complying with the power and performance budget of a low-cost microcontroller.
2017
- A sub-10mW real-Time implementation for EMG hand gesture recognition based on a multi-core biomedical SoC
[Relazione in Atti di Convegno]
Benatti, S.; Rovere, G.; Bosser, J.; Montagna, F.; Farella, E.; Glaser, H.; Schonle, P.; Burger, T.; Fateh, S.; Huang, Q.; Benini, L.
abstract
Real-Time biosignal classification in power-constrained embedded applications is a key step in designing portable e-healtb devices requiring hardware integration along with concurrent signal processing. This paper presents an application based on a novel biomedical System-On-Chip (SoC) for signal acquisition and processing combining a homogeneous multi-core cluster with a versatile bio-potential front-end. The presented implementation acquires raw EMG signals from 3 passive gel-electrodes and classifies 3 hand gestures using a Support Vector Machine (SVM) pattern recognition algorithm. Performance matches state-of-The-Art high-end systems both in terms of recognition accuracy (>S5%) and of real-Time execution (gesture recognition time 300 ms). The power consumption of the employed biomedical SoC is below 10 mW, outperforming implementations on conunercial MCUs by a factor of 10, ensuring a battery life of up to 160 hours with a common Li-ion 1600 mAh battery.
2017
- A wearable EEG-based drowsiness detection system with blink duration and alpha waves analysis
[Relazione in Atti di Convegno]
Kartsch, V.; Benatti, S.; Rossi, D.; Benini, L.
abstract
Drowsiness is one of the most prevalent causes of accidents in mining, driving and industrial activities carrying high personal risks and economic costs. For this reason, automatic detection of drowsiness is becoming an important application, and it is being integrated in a large variety of wearable and deeply embedded systems. Relevant effort has been spent in the past to quantify the drowsiness level from behavioral features exploiting eye tracking systems, dermal sensors or steering wheel movements. On the other hand, all these approaches lack of generality, they are highly intrusive and can only be applied in specific circumstances. A promising alternative approach is based on the extraction and processing of physiological features from the EEG using Brain Computer Interfaces (BCI). This work describes a wearable system capable of detecting drowsiness conditions and emitting alarms using only EEG signals, with three levels of alarm based on the blink duration and on the spectral power of alpha waves. This implementation aims to replace or complement the use of cameras and other sensors, extracting drowsiness information exploiting both behavioral and physiological features from EEG sensors only. The system was validated with 7 test subjects achieving detection accuracy of 85%, while being much more lightweight and compact than other state of the art methods.
2017
- Design challenges for wearable EMG applications
[Relazione in Atti di Convegno]
Milosevic, B.; Benatti, S.; Farella, E.
abstract
Wearable technologies are changing the way we deal with health and fitness in our daily life. Nevertheless, while MEMS-enabled inertial sensors have conquered the consumer market, physiological monitoring has still to face barriers due to the complexity and costs of physical interfaces (e.g. electrodes), the degree of intuitiveness of the interaction and the processing required to reach satisfying performance. These limitations are mitigated by the embedded systems' growing integration of interfacing capabilities and efficient computing power. In this paper, we describe the main applications and the related technologies for the acquisition and processing of myoelectric (EMG) signals. Starting from well established active sensors and bench-top setups, we introduce a recent design based on the combination of an integrated Analog Front End (AFE) and embedded processing. This solution provides high quality signal acquisition and on-board digital processing capabilities with a contained power consumption. The system was tested within the prosthesis control application scenario, one of the most stringent EMG applications, achieving a 90% gesture recognition accuracy with real time on-board processing at a power consumption of 30 mW. Such promising results highlight the current trend in shifting EMG applications from dedicated analog solutions towards integrated digital devices, favouring the development of advanced, modular and low-power wearable solutions.
2017
- Flexible, scalable and energy efficient bio-signals processing on the PULP platform: A case study on seizure detection
[Articolo su rivista]
Montagna, F.; Benatti, S.; Rossi, D.
abstract
Ultra-low power operation and extreme energy efficiency are strong requirements for a number of high-growth application areas requiring near-sensor processing, including elaboration of biosignals. Parallel near-threshold computing is emerging as an approach to achieve significant improvements in energy efficiency while overcoming the performance degradation typical of low-voltage operations. In this paper, we demonstrate the capabilities of the PULP (Parallel Ultra-Low Power) platform on an algorithm for seizure detection, representative of a wide range of EEG signal processing applications. Starting from the 28-nm FD-SOI (Fully Depleted Silicon On Insulator) technology implementation of the third embodiment of the PULP architecture, we analyze the energy-efficient implementation of the seizure detection algorithm on PULP. The proposed parallel implementation exploits the dynamic voltage and frequency scaling capabilities, as well as the embedded power knobs of the PULP platform, reducing energy consumption for a seizure detection by up to 10× with respect to a sequential implementation at the nominal supply voltage and by 4.2× with respect to a sequential implementation with voltage scaling. Moreover, we analyze the trans-precision optimization of the algorithm on PULP, by means of a hybrid fixed-and floating-point implementation. This approach reduces the energy consumption by up to 43% with respect to the plain fixed-point and floating-point implementations, leveraging the requirements in terms of the precision of the kernels composing the processing chain to improve energy efficiency. Thanks to the proposed architecture and system-level approach for optimization, we demonstrate that PULP reduces energy consumption by up to 140× with respect to commercial low-power microcontrollers, being able to satisfy the real-time constraints typical of bio-medical applications, breaking the barrier of microwatts for a 50-ms complete seizure detection and a few milliwatts for a 5-ms detection latency on a fully-programmable architecture.
2017
- Towards a novel HMI paradigm based on mixed EEG and indoor localization platforms
[Relazione in Atti di Convegno]
Salvaro, M.; Kartsch, V.; Benatti, S.; Milano, M.; Benini, L.
abstract
Location Based Services (LBS) have been gaining a great deal of attention thanks to their capability to enhance mobile services with location awareness. While outdoor localization is almost universally achieved via Global Positioning System (GPS), indoor localization is still challenging and a general solution is yet to be found. In a vision where wearable devices are taking over smartphones' leading role as gateway to the cyber world, new paradigms of interactive Human Machine Interfaces (iHMI) are arising. Among others, one of the most intriguing alternative iHMI is based on decoding the brain signals. Combining EEG activity data and indoor localization could dramatically improve the pervasiveness of the interaction between human, devices and environment. For these reasons, we propose a portable Hardware-Software platform that acquires brain EEG signals using a dedicated board along with position information from a cloud service. The positive results of the preliminary analysis successfully show the correlation between EEG signal and motion. Understanding that this is one of the first intents to merge these two sources of information, we intend to share publicly the ever-growing dataset to allow other researchers to investigate better the interaction between subjects and environments, and to lay the foundation of new paradigms in HMI.
2016
- A contactless three-phase autonomous power meter
[Relazione in Atti di Convegno]
Villani, C.; Benatti, S.; Brunelli, D.; Benini, L.
abstract
Electrical energy management is becoming crucial to optimize the generation and usage of power. Therefore, measurement of parameters (such as amplitude and/or phase shift) of electrical systems is of the utmost importance for achieving efficient control on power usage of electric loads in residential and industrial buildings. Most of the existing smart metering devices available on the market need voltage probes which are invasive, because they need a direct connection with the potentials being measured. We present an innovative low-cost clamp-on power meter, designed and optimized for three-phase systems. It can be installed without temporary interruption of the supply directly on the cable insulators and can measure simultaneously both current and voltage of the underneath wires, providing accurate measurements of apparent power, active power, reactive power and power factor. Moreover an energy harvesting unit extracts the necessary energy to supply the meter without electrical contact, permitting to install the device without need of batteries or power plugs. Experimental results show the accuracy of the measurements and the autonomy of the small size and compact power meter.
2016
- Hyperdimensional biosignal processing: A case study for EMG-based hand gesture recognition
[Relazione in Atti di Convegno]
Rahimi, A.; Benatti, S.; Kanerva, P.; Benini, L.; Rabaey, J. M.
abstract
The mathematical properties of high-dimensional spaces seem remarkably suited for describing behaviors produces by brains. Brain-inspired hyperdimensional computing (HDC) explores the emulation of cognition by computing with hypervectors as an alternative to computing with numbers. Hypervectors are high-dimensional, holographic, and (pseudo)random with independent and identically distributed (i.i.d.) components. These features provide an opportunity for energy-efficient computing applied to cyberbiological and cybernetic systems. We describe the use of HDC in a smart prosthetic application, namely hand gesture recognition from a stream of Electromyography (EMG) signals. Our algorithm encodes a stream of analog EMG signals that are simultaneously generated from four channels to a single hypervector. The proposed encoding effectively captures spatial and temporal relations across and within the channels to represent a gesture. This HDC encoder achieves a high level of classification accuracy (97.8%) with only 1/3 the training data required by state-of-the-art SVM on the same task. HDC exhibits fast and accurate learning explicitly allowing online and continuous learning. We further enhance the encoder to adaptively mitigate the effect of gesture-timing uncertainties across different subjects endogenously; further, the encoder inherently maintains the same accuracy when there is up to 30% overlapping between two consecutive gestures in a classification window.
2016
- Power Line Interference Removal for High-Quality Continuous Biosignal Monitoring with Low-Power Wearable Devices
[Articolo su rivista]
Tomasini, M.; Benatti, S.; Milosevic, B.; Farella, E.; Benini, L.
abstract
Mobile and long-term recording of biomedical signals, such as electrocardiogram (ECG), electromyogram (EMG), and EEG, can improve diagnosis and monitor the evolution of several widespread diseases. However, it requires specific solutions, such as wearable devices, that should be particularly comfortable for patients, while at the same time ensuring medical-grade signal acquisition quality, including power line interference (PLI) removal. This paper focuses on the on-board real-time PLI filtering on a low-power biopotential acquisition wearable system. This paper analyzes in depth basic and advanced PLI filtering techniques and evaluates them in a wearable real-time processing scenario, assessing performance on EMG and ECG signals. Our experiments prove that most PLI removal algorithms are not usable in this challenging context, because they lack robustness or they require offline processing and large amounts of available data. On the other hand, adaptive filtering techniques are robust and well suited for lightweight online processing. We substantiate this finding with offline analysis and comparison, as well as with a complete embedded implementation on our low-power low-cost wearable device.
2016
- Sampling modulation: An energy efficient novel feature extraction for biosignal processing
[Relazione in Atti di Convegno]
Causo, M.; Benatti, S.; Frappe, A.; Cathelin, A.; Farella, E.; Kaiser, A.; Benini, L.; Rabaey, J. M.
abstract
Extracting useful information from human bio potentials is an essential component of many wearable health applications. Yet the feature extraction itself can be computationally demanding, and may rapidly exhaust the meager energy supply available to the sensor node. General-purpose time-frequency analysis techniques, such as the Discrete Wavelet Transform (DWT) are widely used, but are computationally demanding and may represent overkill. This work presents a feature extraction technique for biopotential time-frequency analysis, based on the modulation of finite sample differences. The technique is applied to EEG-based seizure detection (feeding a Support Vector Machine (SVM) classifier) and reaches the performance of a DWT implementation, while offering a gain of 5× in power efficiency and 41× in execution.
2016
- Scalable EEG seizure detection on an ultra low power multi-core architecture
[Relazione in Atti di Convegno]
Benatti, S.; Montagna, F.; Rossi, D.; Benini, L.
abstract
Energy efficient processing architectures represent key elements for wearable and implantable medical devices. Signal processing of neural data is a challenge in new designs of Brain Machine Interfaces (BMI). A highly efficient multi-core platform, designed for ultra low power processing allows the execution of complex algorithms complying with real time requirements. This paper describes the implementation and optimization of a seizure detection algorithm on a multi-core digital integrated circuit designed for energy efficient applications. The proposed architecture is able to implement ultra low power parallel processing seizure detection on 23 electrodes within a power budget of 1 mW, outperforming implementations on commercial MCUs by up to 100 times in terms of performance and up to 80 times in terms of energy efficiency still providing high versatility and scalability, opening the way to the development of efficient implantable and wearable smart systems.
2015
- A Versatile Embedded Platform for EMG Acquisition and Gesture Recognition
[Articolo su rivista]
Benatti, S.; Casamassima, F.; Milosevic, B.; Farella, E.; Schonle, P.; Fateh, S.; Burger, T.; Huang, Q.; Benini, L.
abstract
Wearable devices offer interesting features, such as low cost and user friendliness, but their use for medical applications is an open research topic, given the limited hardware resources they provide. In this paper, we present an embedded solution for real-time EMG-based hand gesture recognition. The work focuses on the multi-level design of the system, integrating the hardware and software components to develop a wearable device capable of acquiring and processing EMG signals for real-time gesture recognition. The system combines the accuracy of a custom analog front end with the flexibility of a low power and high performance microcontroller for on-board processing. Our system achieves the same accuracy of high-end and more expensive active EMG sensors used in applications with strict requirements on signal quality. At the same time, due to its flexible configuration, it can be compared to the few wearable platforms designed for EMG gesture recognition available on market. We demonstrate that we reach similar or better performance while embedding the gesture recognition on board, with the benefit of cost reduction. To validate this approach, we collected a dataset of 7 gestures from 4 users, which were used to evaluate the impact of the number of EMG channels, the number of recognized gestures and the data rate on the recognition accuracy and on the computational demand of the classifier. As a result, we implemented a SVM recognition algorithm capable of real-time performance on the proposed wearable platform, achieving a classification rate of 90%, which is aligned with the state-of-the-art off-line results and a 29.7 mW power consumption, guaranteeing 44 hours of continuous operation with a 400 mAh battery.
2015
- Digitally controlled feedback for DC offset cancellation in a wearable multichannel EMG platform
[Relazione in Atti di Convegno]
Tomasini, M.; Benatti, S.; Casamassima, F.; Milosevic, B.; Fateh, S.; Farella, E.; Benini, L.
abstract
Wearable systems capable to capture vital signs allow the development of advanced medical applications. One notable example is the use of surface electromyography (EMG) to gather muscle activation potentials, in principle an easy input for prosthesis control. However, the acquisition of such signals is affected by high variability and ground loop problems. Moreover, the input impedance influenced in time by motion and perspiration determines an offset, which can be orders of magnitude higher than the signal of interest. We propose a wearable device equipped with a digitally controlled Analog Front End (AFE) for biopotentials acquisition with zero-offset. The proposed AFE solution has an internal Digital to Analog Converter (DAC) used to adjust independently the reference of each channel removing any DC offset. The analog integrated circuit is coupled with a microcontroller, which periodically estimates the offset and implements a closed loop feedback on the analog part. The proposed approach was tested on EMG signals acquired from 4 subjects while performing different activities and shows that the system correctly acquires signals with no DC offset.
2015
- Experimental evaluation of a sEMG-based human-robot interface for human-like grasping tasks
[Relazione in Atti di Convegno]
Meattini, R.; Benatti, S.; Scarcia, U.; Benini, L.; Melchiorri, C.
abstract
In this paper we present a Human-Robot Interface (HRI) to control a robotic hand via myoelectric signals for grasping tasks. The system is composed by the UB Hand IV as robotic device, and by the Cerebro wearable board as acquisition hardware of the signals from surface skin electrodes. The approach implemented for the HRI relies on a pair of antagonistic flexor-extensor muscles that control both the closure and the grasp stiffness of the robotic hand. Humans accomplish a large variety of grasps thanks to precise impedance regulation: the aim of this study is to emulate this capability on a robotic hand using a user's muscles driven HRI. Experiments conducted with healty subjects showed a short training time together with high success rate of grasp-related tasks, where the users of the HRI were able to naturally modulate the hand's degrees of control by means of forearm muscle contractions. The results show that the system is suitable for further developments for telemanipulation and prosthetic applications.
2015
- Hybrid EMG classifier based on HMM and SVM for hand gesture recognition in prosthetics
[Relazione in Atti di Convegno]
Rossi, M.; Benatti, S.; Farella, E.; Benini, L.
abstract
Pattern recognition and classification algorithms are widely studied in natural gesture interfaces for upper limb prostheses. Robustness and accuracy of control systems are key challenge in such applications. To improve the classification performance, the conventional approach builds on classifier parameters tuning and/or feature extraction techniques. In this paper, we propose a complementary approach based on the combination of two heterogeneous classifiers: the Support Vector Machines and the Hidden Markov Models. This technique takes advantage of the robust time-independent classification of the SVM taking into account the information about history of the signal with the HMM. We demonstrate that, independently from the performance of the SVM, which can be further optimized with typical methods, the combined approach gains 12% recognition accuracy. We further comment on the applicability of this approach in resource constrained embedded implementations considering real-time requirements in the field of prosthesis control.
2015
- Multiple biopotentials acquisition system for wearable applications
[Relazione in Atti di Convegno]
Benatti, S.; Milosevic, B.; Tomasini, M.; Farella, E.; Schonle, P.; Bunjaku, P.; Rovere, G.; Fateh, S.; Huang, Q.; Benini, L.
abstract
Wearable devices for monitoring vital signs such as heart-rate, respiratory rate and blood pressure are demonstrating to have an increasing role in improving quality of life and in allowing prevention for chronic cardiac diseases. However, the design of a wearable system without reference to ground potential requires multi-level strategies to remove noise caused from power lines. This paper describes a bio-potential acquisition embedded system designed with an innovative analog front-end, showing the performance in EEG and ECG applications and the comparison between different noise reduction algorithms. We demonstrate that the proposed system is able to acquire bio-potentials with a signal quality equivalent to state-of-the-art bench-top biomedical devices and can be therefore used for monitoring purpose, with the advantages of a low-cost low-power wearable devices.
2014
- Analysis of robust implementation of an EMG pattern recognition based control
[Relazione in Atti di Convegno]
Benatti, S.; Farella, E.; Gruppioni, E.; Benini, L.
abstract
Control of active hand prostheses is an open challenge. In fact, the advances in mechatronics made available prosthetic hands with multiple active degrees of freedom; however the predominant control strategies are still not natural for the user, enabling only few gestures, thus not exploiting the prosthesis potential. Pattern recognition and machine learning techniques can be of great help when applied to surface electromyography signals to offer a natural control based on the contraction of muscles corresponding to the real movements. The implementation of such approach for an active prosthetic system offers many challenges related to the reliability of data collected to train the classification algorithm. This paper focuses on these problems and propose an implementation suitable for an embedded system. Copyright © 2014 SCITEPRESS - Science and Technology Publications. All rights reserved.
2014
- EMG-based hand gesture recognition with flexible analog front end
[Relazione in Atti di Convegno]
Benatti, S.; Milosevic, B.; Casamassima, F.; Schonle, P.; Bunjaku, P.; Fateh, S.; Huang, Q.; Benini, L.
abstract
Conditioning and processing of biological signals represent interesting challenges for wearable electronics in health applications. Information gathering from these signals requires complex hardware circuitry and dedicated computation resources. The design of innovative analog front-end integrated circuits, combined with efficient signal processing algorithms, allows the development of platforms for monitoring, activity and gesture recognition based on embedded real-time systems. This paper describes an Electromyography pattern recognition system based on the combination of low cost passive sensors, an innovative analog front-end and a low power microcontroller. The performance of the proposed system matches state-of-the-art high-end active sensors, opening the way to the development of affordable and accurate wearable devices.
2014
- Towards EMG control interface for smart garments
[Relazione in Atti di Convegno]
Benatti, S.; Benini, L.; Farella, E.
abstract
Wearable computing devices can greatly enhance the quality of life, helping interaction with smart environment, activity recognition and healthcare applications. Smart garments ofer the opportunity to integrate sensors and electronics in unobtrusive wearable systems. The paper presents a case study of an embedded hand gesture recognition system, which uses EMG electrodes embeddable in smart clothes. We analyze the main challenges of a real-time system for pattern recognition and the results of the proposed experiment demonstrate the feasibility of a real-time system for pattern recognition, which can be integrated in smart clothes.