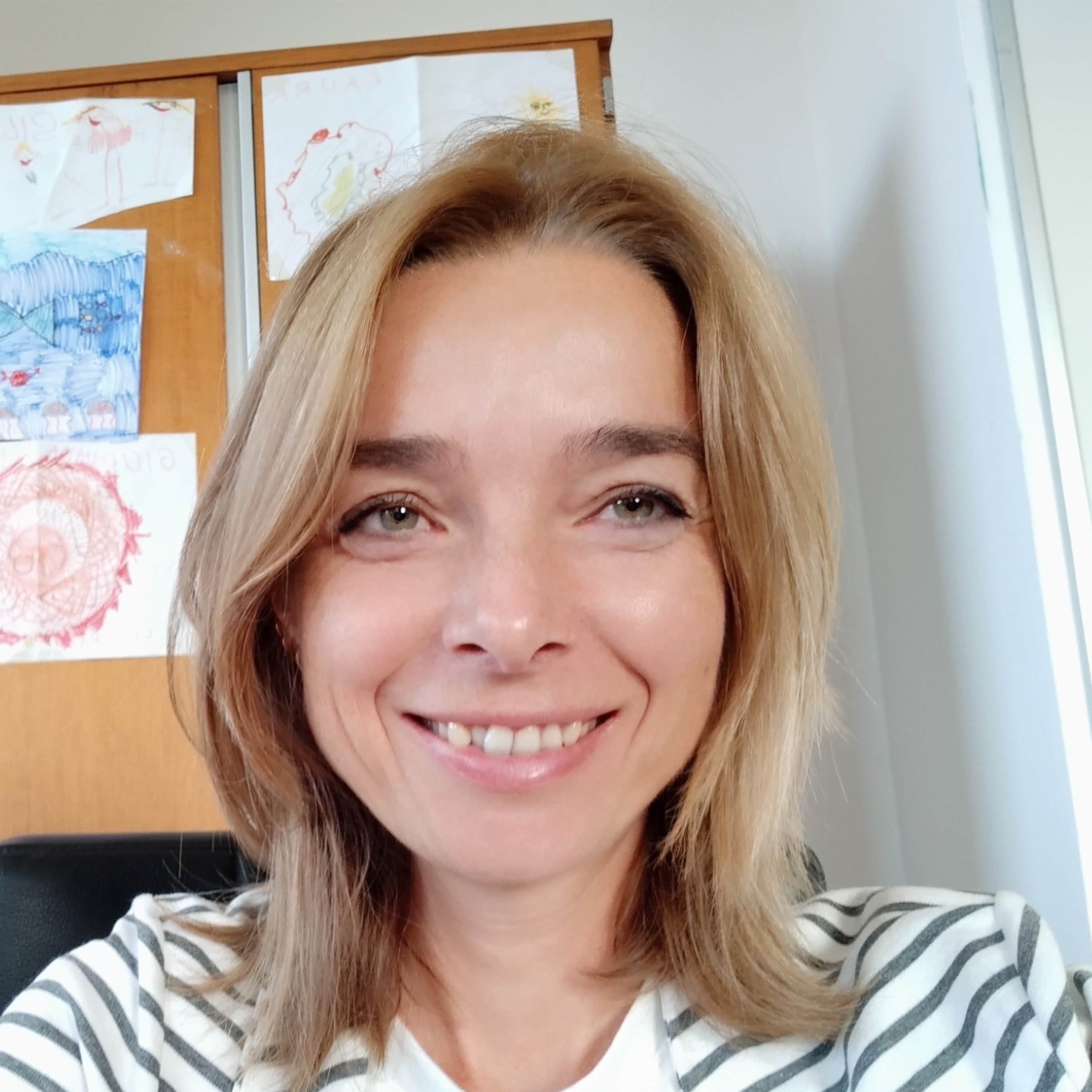 |
Silvia BONETTINI
Professore Associato Dipartimento di Scienze Fisiche, Informatiche e Matematiche sede ex-Matematica
|
Home |
Curriculum(pdf) |
Didattica |
Pubblicazioni
2024
- A new proximal heavy ball inexact line-search algorithm
[Articolo su rivista]
Bonettini, S.; Prato, M.; Rebegoldi, S.
abstract
We study a novel inertial proximal-gradient method for composite optimization. The
proposed method alternates between a variablemetric proximal-gradient iterationwith
momentum and an Armijo-like linesearch based on the sufficient decrease of a suitable
merit function. The linesearch procedure allows for a major flexibility on the choice of
the algorithm parameters. We prove the convergence of the iterates sequence towards
a stationary point of the problem, in a Kurdyka–Łojasiewicz framework. Numerical
experiments on a variety of convex and nonconvex problems highlight the superiority
of our proposal with respect to several standard methods, especially when the inertial
parameter is selected by mimicking the Conjugate Gradient updating rule.
2023
- An abstract convergence framework with application to inertial inexact forward–backward methods
[Articolo su rivista]
Bonettini, S.; Ochs, P.; Prato, M.; Rebegoldi, S.
abstract
In this paper we introduce a novel abstract descent scheme suited for the minimization of proper and lower semicontinuous functions. The proposed abstract scheme generalizes a set of properties that are crucial for the convergence of several first-order methods designed for nonsmooth nonconvex optimization problems. Such properties guarantee the convergence of the full sequence of iterates to a stationary point, if the objective function satisfies the Kurdyka–Łojasiewicz property. The abstract framework allows for the design of new algorithms. We propose two inertial-type algorithms with implementable inexactness criteria for the main iteration update step. The first algorithm, i2Piano, exploits large steps by adjusting a local Lipschitz constant. The second algorithm, iPila, overcomes the main drawback of line-search based methods by enforcing a descent only on a merit function instead of the objective function. Both algorithms have the potential to escape local minimizers (or stationary points) by leveraging the inertial feature. Moreover, they are proved to enjoy the full convergence guarantees of the abstract descent scheme, which is the best we can expect in such a general nonsmooth nonconvex optimization setup using first-order methods. The efficiency of the proposed algorithms is demonstrated on two exemplary image deblurring problems, where we can appreciate the benefits of performing a linesearch along the descent direction inside an inertial scheme.
2023
- Explainable bilevel optimization: An application to the Helsinki deblur challenge
[Articolo su rivista]
Bonettini, Silvia; Franchini, Giorgia; Pezzi, Danilo; Prato, Marco
abstract
In this paper we present a bilevel optimization scheme for the solution of a general image deblurring problem, in which a parametric variational-like approach is encapsulated within a machine learning scheme to provide a high quality reconstructed image with automatically learned parameters. The ingredients of the variational lower level and the machine learning upper one are specifically chosen for the Helsinki Deblur Challenge 2021, in which sequences of letters are asked to be recovered from out-of-focus photographs with increasing levels of blur. Our proposed procedure for the reconstructed image consists in a fixed number of FISTA iterations applied to the minimization of an edge preserving and binarization enforcing regularized least-squares functional. The parameters defining the variational model and the optimization steps, which, unlike most deep learning approaches, all have a precise and interpretable meaning, are learned via either a similarity index or a support vector machine strategy. Numerical experiments on the test images provided by the challenge authors show significant gains with respect to a standard variational approach and performances comparable with those of some of the proposed deep learning based algorithms which require the optimization of millions of parameters.
2023
- On an iteratively reweighted linesearch based algorithm for nonconvex composite optimization
[Articolo su rivista]
Bonettini, S.; Pezzi, D.; Prato, M.; Rebegoldi, S.
abstract
In this paper we propose a new algorithm for solving a class of nonsmooth nonconvex problems, which is obtained by combining the iteratively reweighted scheme with a finite number of forward–backward iterations based on a linesearch procedure. The new method overcomes some limitations of linesearch forward–backward methods, since it can be applied also to minimize functions containing terms that are both nonsmooth and nonconvex. Moreover, the combined scheme can take advantage of acceleration techniques consisting in suitable selection rules for the algorithm parameters. We develop the convergence analysis of the new method within the framework of the Kurdyka– Lojasiewicz property. Finally, we present the results of a numerical experience on microscopy image super resolution, showing that the performances of our method are comparable or superior to those of other algorithms designed for this specific application.
2022
- A nested primal-dual FISTA-like scheme for composite convex optimization problems
[Articolo su rivista]
Bonettini, S.; Prato, M.; Rebegoldi, S.
abstract
We propose a nested primal–dual algorithm with extrapolation on the primal variable
suited for minimizing the sum of two convex functions, one of which is continuously
differentiable. The proposed algorithm can be interpreted as an inexact inertial
forward–backward algorithm equipped with a prefixed number of inner primal–dual
iterations for the proximal evaluation and a “warm–start” strategy for starting the
inner loop, and generalizes several nested primal–dual algorithms already available
in the literature. By appropriately choosing the inertial parameters, we prove the
convergence of the iterates to a saddle point of the problem, and provide an O(1/n)
convergence rate on the primal–dual gap evaluated at the corresponding ergodic
sequences. Numerical experiments on some image restoration problems show that
the combination of the “warm–start” strategy with an appropriate choice of the inertial
parameters is strictly required in order to guarantee the convergence to the real
minimum point of the objective function.
2022
- Learning the Image Prior by Unrolling an Optimization Method
[Relazione in Atti di Convegno]
Bonettini, S.; Franchini, G.; Pezzi, D.; Prato, M.
abstract
Nowadays neural networks are omnipresent thanks to the amazing adaptability they possess, despite their poor interpretability and the difficulties they give when manipulating the parameters. On the other side, we have the classical variational approach, where the restoration is obtained as the solution of a given optimization problem. The bilevel approach is connected to both approaches and consists first in devising a parametric formulation of the variational problem, then in optimizing these parameters with respect to a given dataset of training data. In this work we analyze the classical bilevel approach in combination with unrolling techniques, where the parameters of the variational problem are trained with respect to the results obtained after a fixed number of iterations of an optimization method applied to it. This results in a large scale optimization problem which can be solved by means of stochastic methods; as we observed in our numerical experiments, the stochastic approach can produce medium accuracy results in very few epochs. Moreover, our experiments also show that the unrolling approach leads to results which are comparable with those of the original bilevel method in terms of accuracy.
2021
- A comparison of nested primal-dual forward-backward methods for Poisson image deblurring
[Relazione in Atti di Convegno]
Rebegoldi, Simone; Bonettini, Silvia; Prato, Marco
abstract
We consider an inexact version of the popular Fast Iterative Soft-Thresholding Algorithm (FISTA) suited for minimizing the sum of a differentiable convex data fidelity function plus a nondifferentiable convex regularizer whose proximal operator is not computable in closed form. The proposed method is a nested primal–dual forward–backward method inspired by the methodology developed in [10], according to which the proximal-gradient point is approximated by means of a prefixed number of inner primal-dual iterates initialized with an appropriate warmstart strategy. We report some preliminary numerical results on a weighted least squares total-variation based model for Poisson image deblurring, which show the efficiency of the proposed FISTA-like method with respect to other strategies for defining the inner loop associated to the proximal step.
2021
- Variable metric techniques for forward–backward methods in imaging
[Articolo su rivista]
Bonettini, S.; Porta, F.; Ruggiero, V.; Zanni, L.
abstract
Variable metric techniques are a crucial ingredient in many first order optimization algorithms. In practice, they consist in a rule for computing, at each iteration, a suitable symmetric, positive definite scaling matrix to be multiplied to the gradient vector. Besides quasi-Newton BFGS techniques, which represented the state-of-the-art since the 70's, new approaches have been proposed in the last decade in the framework of imaging problems expressed in variational form. Such recent approaches are appealing since they can be applied to large scale problems without adding significant computational costs and they produce an impressive improvement in the practical performances of first order methods. These scaling strategies are strictly connected to the shape of the specific objective function and constraints of the optimization problem they are applied to; therefore, they are able to effectively capture the problem features. On the other side, this strict problem dependence makes difficult extending the existing techniques to more general problems. Moreover, in spite of the experimental evidence of their practical effectiveness, their theoretical properties are not well understood. The aim of this paper is to investigate these issues; in particular, we develop a unified framework for scaling techniques, multiplicative algorithms and the Majorization–Minimization approach. With this inspiration, we propose a scaling matrix rule for variable metric first order methods applied to nonnegatively constrained problems exploiting a suitable structure of the objective function. Finally, we evaluate the effectiveness of the proposed approach on some image restoration problems.
2020
- Convergence of inexact forward-backward algorithms using the forward-backward envelope
[Articolo su rivista]
Bonettini, S.; Prato, M.; Rebegoldi, S.
abstract
This paper deals with a general framework for inexact forward-backward algorithms aimed at minimizing the sum of an analytic function and a lower semicontinuous, subanalytic, convex term. Such framework relies on an implementable inexactness condition for the computation of the proximal operator, and a linesearch procedure which is possibly performed whenever a variable metric is allowed into the forward-backward step. The main focus of the work is the convergence of the considered scheme without additional convexity assumptions on the objective function. Toward this aim, we employ the recent concept of forward{backward envelope to define a continuously differentiable surrogate function, which coincides with the objective at its stationary points, and satisfies the so-called Kurdyka-Lojasiewicz (KL) property on its domain. We adapt the abstract convergence scheme usually exploited in the KL framework to our inexact forward-backward scheme, and prove the convergence of the iterates to a stationary point of the problem, as well as the convergence rates for the function values. Finally, we show the effectiveness and the flexibility of the proposed framework on a large-scale image restoration test problem.
2020
- Efficient Block Coordinate Methods for Blind Cauchy Denoising
[Relazione in Atti di Convegno]
Rebegoldi, S.; Bonettini, S.; Prato, M.
abstract
This paper deals with the problem of image blind deconvolution in presence of Cauchy noise, a type of non-Gaussian, impulsive degradation which frequently appears in engineering and biomedical applications. We consider a regularized version of the corresponding data fidelity function, by adding the total variation regularizer on the image and a Tikhonov term on the point spread function (PSF). The resulting objective function is nonconvex with respect to both the image and PSF block, which leads to the presence of several uninteresting local minima. We propose to tackle such challenging problem by means of a block coordinate linesearch based forward backward algorithm suited for nonsmooth nonconvex optimization. The proposed method allows performing multiple forward-backward steps on each block of variables, as well as adopting variable steplengths and scaling matrices to accelerate the progress towards a stationary point. The convergence of the scheme is guaranteed by imposing a linesearch procedure at each inner step of the algorithm. We provide some practical sound rules to adaptively choose both the variable metric parameters and the number of inner iterations on each block. Numerical experiments show how the proposed approach delivers better performances in terms of efficiency and accuracy if compared to a more standard block coordinate strategy.
2020
- New convergence results for the inexact variable metric forward-backward method
[Articolo su rivista]
Bonettini, Silvia; Prato, Marco; Rebegoldi, Simone
abstract
Forward-backward methods are valid tools to solve a variety of optimization problems where the objective function is the sum of a smooth, possibly nonconvex term plus a convex, possibly nonsmooth function. The corresponding iteration is built on two main ingredients: the computation of the gradient of the smooth part and the evaluation of the proximity (or resolvent) operator associated to the convex term. One of the main difficulties, from both implementation and theoretical point of view, arises when the proximity operator is computed in an inexact way. The aim of this paper is to provide new convergence results about forward-backward methods with inexact computation of the proximity operator, under the assumption that the objective function satisfies the Kurdyka-Lojasiewicz property. In particular, we adopt an inexactness criterion which can be implemented in practice, while preserving the main theoretical properties of the proximity operator. The main result is the proof of the convergence of the iterates generated by the forward-backward algorithm in [1] to a stationary point. Convergence rate estimates are also provided. At the best of our knowledge, there exists no other inexact forward-backward algorithm with proved convergence in the nonconvex case and equipped with an explicit procedure to inexactly compute the proximity operator.
2019
- Recent advances in variable metric first-order methods
[Capitolo/Saggio]
Bonettini, S.; Porta, F.; Prato, M.; Rebegoldi, S.; Ruggiero, V.; Zanni, L.
abstract
Minimization problems often occur in modeling phenomena dealing with real-life applications that nowadays handle large-scale data and require real-time solutions. For these reasons, among all possible iterative schemes, first-order algorithms represent a powerful tool in solving such optimization problems since they admit a relatively simple implementation and avoid onerous computations during the iterations. On the other hand, a well known drawback of these methods is a possible poor convergence rate, especially showed when an high accurate solution is required. Consequently, the acceleration of first-order approaches is a very discussed field which has experienced several efforts from many researchers in the last decades. The possibility of considering a variable underlying metric changing at each iteration and aimed to catch local properties of the starting problem has been proved to be effective in speeding up first-order methods. In this work we deeply analyze a possible way to include a variable metric in first-order methods for the minimization of a functional which can be expressed as the sum of a differentiable term and a nondifferentiable one. Particularly, the strategy discussed can be realized by means of a suitable sequence of symmetric and positive definite matrices belonging to a compact set, together with an Armijo-like linesearch procedure to select the steplength along the descent direction ensuring a sufficient decrease of the objective function.
2018
- A Bregman inexact linesearch-based forward-backward algorithm for nonsmooth nonconvex optimization
[Relazione in Atti di Convegno]
Rebegoldi, S.; Bonettini, S.; Prato, M.
abstract
In this paper, we present a forward–backward linesearch–based algorithm suited for the minimization of the sum of a smooth (possibly nonconvex) function and a convex (possibly nonsmooth) term. Such algorithm first computes inexactly the proximal operator with respect to a given Bregman distance, and then ensures a sufficient decrease condition by performing a linesearch along the descent direction. The proposed approach can be seen as an instance of the more general class of descent methods presented in [1], however, unlike in [1], we do not assume the strong convexity of the Bregman distance used in the proximal evaluation. We prove that each limit point of the iterates sequence is stationary, we show how to compute an approximate proximal–gradient point with respect to a Bregman distance and, finally, we report the good numerical performance of the algorithm on a large scale image restoration problem. [1] S. Bonettini, I. Loris, F. Porta, and M. Prato 2016, Variable metric inexact line-search-based methods for nonsmooth optimization, SIAM J. Optim. 26(2), 891–921.
2018
- A block coordinate variable metric linesearch based proximal gradient method
[Articolo su rivista]
Bonettini, Silvia; Prato, Marco; Rebegoldi, Simone
abstract
In this paper we propose an alternating block version of a variable metric linesearch proximal gradient method. This algorithm addresses problems where the objective function is the sum of a smooth term, whose variables may be coupled, plus a separable part given by the sum of two or more convex, possibly nonsmooth functions, each depending on a single block of variables. Our approach is characterized by the possibility of performing several proximal gradient steps for updating every block of variables and by the Armijo backtracking linesearch for adaptively computing the steplength parameter. Under the assumption that the objective function satisfies the Kurdyka- Lojasiewicz property at each point of its domain and the gradient of the smooth part is locally Lipschitz continuous, we prove the convergence of the iterates sequence generated by the method. Numerical experience on an image blind deconvolution problem show the improvements obtained by adopting a variable number of inner block
iterations combined with a variable metric in the computation of the proximal operator.
2018
- A nonsmooth regularization approach based on shearlets for Poisson noise removal in ROI tomography
[Articolo su rivista]
Bubba, Tatiana Alessandra; Porta, Federica; Zanghirati, Gaetano; Bonettini, Silvia
abstract
Due to its potential to lower exposure to X-ray radiation and reduce the scanning time, region-of-interest (ROI) computed tomography (CT) is particularly appealing for a wide range of biomedical applications. To overcome the severe ill-posedness caused by the truncation of projection measurements, ad hoc strategies are required, since traditional CT reconstruction algorithms result in instability to noise, and may give inaccurate results for small ROI. To handle this difficulty, we propose a nonsmooth convex optimization model based on ℓ1 shearlet regularization, whose solution is addressed by means of the variable metric inexact line search algorithm (VMILA), a proximal-gradient method that enables the inexact computation of the proximal point defining the descent direction. We compare the reconstruction performance of our strategy against a smooth total variation (sTV) approach, by using both Poisson noisy simulated data and real data from fan-beam CT geometry. The results show that, while for synthetic data both shearets and sTV perform well, for real data, the proposed nonsmooth shearlet-based approach outperforms sTV, since the localization and directional properties of shearlets allow to detect finer structures of a textured image. Finally, our approach appears to be insensitive to the ROI size and location.
2018
- Inertial variable metric techniques for the inexact forward-backward algorithm
[Articolo su rivista]
Bonettini, S.; Rebegoldi, S.; Ruggiero, V.
abstract
One of the most popular approaches for the minimization of a convex functional given by the sum of a differentiable term and a nondifferentiable one is the forward-backward method with extrapolation. The main reason making this method very appealing for a wide range of applications is that it achieves a O(1/k2) convergence rate in the objective function values, which is optimal for a first order method. Recent contributions on this topic are related to the convergence of the iterates to a minimizer and the possibility of adopting a variable metric in the proximal step. Moreover, it has been also proved that the objective function convergence rate is actually o(1/k2). However, these results are obtained under the assumption that the minimization subproblem involved in the backward step is computed exactly, which is clearly not realistic in a variety of relevant applications. In this paper, we analyze the convergence properties when both variable metric and inexact computation of the backward step are allowed. To do this, we adopt a suitable inexactness criterion and we devise implementable conditions on both the accuracy of the inexact backward step computation and the variable metric selection, so that the o(1/k2) rate and the convergence of the iterates are preserved. The effectiveness of the proposed approach is also validated with a numerical experience showing the effects of the combination of inexactness with variable metric techniques.
2018
- Shearlet-based regularized reconstruction in region-of-interest computed tomography
[Articolo su rivista]
Bubba, Tatiana Alessandra; Labate, Demetrio; Zanghirati, Gaetano; Bonettini, Silvia
abstract
Region of interest (ROI) tomography has gained increasing attention in recent years due to its potential to reducing radiation exposure and shortening the scanning time. However, tomographic reconstruction from ROI-focused illumination involves truncated projection data and typically results in higher numerical instability even when the reconstruction problem has unique solution. To address this problem, both ad hoc analytic formulas and iterative numerical schemes have been proposed in the literature. In this paper, we introduce a novel approach for ROI tomographic reconstruction, formulated as a convex optimization problem with a regularized term based on shearlets. Our numerical implementation consists of an iterative scheme based on the scaled gradient projection method and it is tested in the context of fan-beam CT. Our results show that our approach is essentially insensitive to the location of the ROI and remains very stable also when the ROI size is rather small.
2017
- On the convergence of a linesearch based proximal-gradient method for nonconvex optimization
[Articolo su rivista]
Bonettini, Silvia; Loris, Ignace; Porta, Federica; Prato, Marco; Rebegoldi, Simone
abstract
We consider a variable metric linesearch based proximal gradient method for the minimization of the sum of a smooth, possibly nonconvex function plus a convex, possibly nonsmooth term. We prove convergence of this iterative algorithm to a critical point if the objective function satisfies the Kurdyka- Lojasiewicz property at each point of its domain, under the assumption that a limit point exists. The proposed method is applied to a wide collection of image processing problems and our numerical tests show that our algorithm results to be flexible, robust and competitive when compared to recently proposed approaches able to address the optimization problems arising in the considered applications.
2016
- A Variable Metric Forward-Backward Method with Extrapolation
[Articolo su rivista]
Bonettini, Silvia; Porta, Federica; Ruggiero, V.
abstract
Forward-backward methods are a very useful tool for the minimization of a functional given by the sum of a differentiable term and a nondifferentiable one, and their investigation has comprised several efforts from many researchers in the last decade. In this paper we focus on the convex case and, inspired by recent approaches for accelerating first-order iterative schemes, we develop a scaled inertial forward-backward algorithm which is based on a metric changing at each iteration and on a suitable extrapolation step. Unlike standard forward-backward methods with extrapolation, our scheme is able to handle functions whose domain is not the entire space. Both an O(1/k^2) convergence rate estimate on the objective function values and the convergence of the sequence of the iterates are proved. Numerical experiments on several test problems arising from image processing, compressed sensing, and statistical inference show the effectiveness of the proposed method in comparison to well-performing state-of-the-art algorithms.
2016
- A cyclic block coordinate descent method with generalized gradient projections
[Articolo su rivista]
Bonettini, Silvia; Prato, Marco; Rebegoldi, Simone
abstract
The aim of this paper is to present the convergence analysis of a very general class of gradient projection methods for smooth, constrained, possibly nonconvex, optimization. The key features of these methods are the Armijo linesearch along a suitable descent direction and the non Euclidean metric employed to compute the gradient projection. We develop a very general framework from the point of view of block–coordinate descent methods, which are useful when the constraints are separable. In our numerical experiments we consider a large scale image restoration problem to illustrate the impact of the metric choice on the practical performances of the corresponding algorithm.
2016
- On the constrained minimization of smooth Kurdyka– Lojasiewicz functions with the scaled gradient projection method
[Relazione in Atti di Convegno]
Prato, Marco; Bonettini, Silvia; Loris, Ignace; Porta, Federica; Rebegoldi, Simone
abstract
The scaled gradient projection (SGP) method is a first-order optimization method applicable to the constrained minimization of smooth functions and exploiting a scaling matrix
multiplying the gradient and a variable steplength parameter to improve the convergence of the scheme. For a general nonconvex function, the limit points of the sequence generated by SGP have been proved to be stationary, while in the convex case and with some restrictions on the choice of the scaling matrix the sequence itself converges to a constrained minimum point. In this paper we extend these convergence results by showing that the SGP sequence converges to a limit point provided that the objective function satisfies the Kurdyka– Lojasiewicz property at each point of its domain and its gradient is Lipschitz continuous.
2016
- Scaling techniques for $epsilon$-subgradient methods
[Articolo su rivista]
Bonettini, Silvia; Benfenati, Alessandro; Ruggiero, Valeria
abstract
The recent literature on first order methods for smooth optimization shows that significant improvements on the practical convergence behavior can be achieved with variable step size and scaling for the gradient, making this class of algorithms attractive for a variety of relevant applications. In this paper we introduce a variable metric in the context of the -subgradient methods for nonsmooth, convex problems, in combination with two different step size selection strategies. We develop the theoretical convergence analysis of the proposed approach in the general framework of forward-backward -subgradient splitting methods and we also discuss practical implementation issues. In order to illustrate the effectiveness of the method, we consider a specific problem in the image restoration framework and we numerically evaluate the effects of a variable scaling and of the step length selection strategy on the convergence behavior.
2016
- The ROI CT problem: a shearlet-based regularization approach
[Relazione in Atti di Convegno]
Bubba, TATIANA ALESSANDRA; Porta, Federica; Zanghirati, Gaetano; Bonettini, Silvia
abstract
The possibility to significantly reduce the X-ray radiation dose and shorten the scanning time is particularly appealing, especially for the medical imaging community. Region-of-interest Computed Tomography (ROI CT) has this potential and, for this reason, is currently receiving increasing attention. Due to the truncation of projection images, ROI CT is a rather challenging problem. Indeed, the ROI reconstruction problem is severely ill-posed in general and naive local reconstruction algorithms tend to be very unstable. To obtain a stable and reliable reconstruction, under suitable noise circumstances, we formulate the ROI CT problem as a convex optimization problem with a regularization term based on shearlets, and possibly nonsmooth. For the solution, we propose and analyze an iterative approach based on the variable metric inexact line-search algorithm (VMILA). The reconstruction performance of VMILA is compared against different regularization conditions, in the case of fan-beam CT simulated data. The numerical tests show that our approach is insensitive to the location of the ROI and
remains very stable also when the ROI size is rather small.
2016
- Variable metric inexact line-search based methods for nonsmooth optimization
[Articolo su rivista]
Bonettini, Silvia; Loris, Ignace; Porta, Federica; Prato, Marco
abstract
We develop a new proximal--gradient method for minimizing the sum of a differentiable, possibly nonconvex, function plus a convex, possibly non differentiable, function. The key features of the proposed method are the definition of a suitable descent direction, based on the proximal operator associated to the convex part of the objective function, and an Armijo--like rule to determine the step size along this direction ensuring the sufficient decrease of the objective function. In this frame, we especially address the possibility of adopting a metric which may change at each iteration and an inexact computation of the proximal point defining the descent direction. For the more general nonconvex case, we prove that all limit points of the iterates sequence are stationary, while for convex objective functions we prove the convergence of the whole sequence to a minimizer, under the assumption that a minimizer exists. In the latter case, assuming also that the gradient of the smooth part of the objective function is Lipschitz, we also give a convergence rate estimate, showing the O(1/k) complexity with respect to the function values. We also discuss verifiable sufficient conditions for the inexact proximal point and we present the results of two numerical tests on total variation based image restoration problems, showing that the proposed approach is competitive with other state-of-the-art methods.
2015
- A blind deconvolution method for ground based telescopes and Fizeau interferometers
[Articolo su rivista]
Prato, Marco; La Camera, A.; Bonettini, Silvia; Rebegoldi, Simone; Bertero, M.; Boccacci, P.
abstract
In the case of ground-based telescopes equipped with adaptive optics systems, the point spread function (PSF) is only poorly known or completely unknown. Moreover, an accurate modeling of the PSF is in general not available. Therefore in several imaging situations the so-called blind deconvolution methods, aiming at estimating both the scientific target and the PSF from the detected image, can be useful. A blind deconvolution problem is severely ill-posed and, in order to reduce the extremely large number of possible solutions, it is necessary to introduce sensible constraints on both the scientific target and the PSF. In a previous paper we proposed a sound mathematical approach based on a suitable inexact alternating minimization strategy for minimizing the generalizedKullback-Leibler divergence, assuring global convergence. In the framework of this method we showed that an important constraint on the PSF is the upper bound which can be derived from the knowledge of its Strehl ratio. The efficacy of the approach was demonstrated by means of numerical simulations. In this paper, besides improving the previous approach by the use of a further constraint on the unknown scientific target, we extend it to the case of multiple images of the same target obtained with different PSFs. The main application we have in mind is to Fizeau interferometry. As it is known this is a special feature of the Large Binocular Telescope (LBT). Of the two expected interferometers for LBT, one, LINC-NIRVANA, is forthcoming while the other, LBTI, is already operating and has provided the first Fizeau images, demonstrating the possibility of reaching the resolution of a 22.8 m telescope. Therefore the extension of our blind method to this imaging modality seems to be timely. The method is applied to realistic simulations of imaging both by single mirrors and Fizeau interferometers. Successes and failures of the method in the imaging of stellar fields are demonstrated in simple cases. These preliminary results look promising at least in specific situations. The IDL code of the proposed method is available on request and will be included in the forthcoming version of the Software Package AIRY (v.6.1).
2015
- A scaled gradient projection method for Bayesian learning in dynamical systems
[Articolo su rivista]
Bonettini, Silvia; Chiuso, A.; Prato, Marco
abstract
A crucial task in system identification problems is the selection of the most appropriate model class, and is classically addressed resorting to cross-validation or using order selection criteria based on asymptotic arguments. As recently suggested in the literature, this can be addressed in a Bayesian framework, where model complexity is regulated by few hyperparameters, which can be estimated via marginal likelihood maximization. It is thus of primary importance to design effective optimization methods to solve the corresponding optimization problem. If the unknown impulse response is modeled as a Gaussian process with a suitable kernel, the maximization of the marginal likelihood leads to a challenging nonconvex optimization problem, which requires a stable and effective solution strategy.
In this paper we address this problem by means of a scaled gradient projection algorithm, in which the scaling matrix and the steplength parameter play a crucial role to provide a meaningful solution in a computational time comparable with second order methods. In particular, we propose both a generalization of the split gradient approach to design the scaling matrix in the presence of box constraints, and an effective implementation of the gradient and objective function.
The extensive numerical experiments carried out on several test problems show that our method is very effective in providing in few tenths of a second solutions of the problems with accuracy comparable with state-of-the-art approaches. Moreover, the flexibility of the proposed strategy makes it easily adaptable to a wider range of problems arising in different areas of machine learning, signal processing and system identification.
2015
- Application of cyclic block generalized gradient projection methods to Poisson blind deconvolution
[Relazione in Atti di Convegno]
Rebegoldi, Simone; Bonettini, Silvia; Prato, Marco
abstract
The aim of this paper is to consider a modification of a block coordinate gradient projection method with Armijo linesearch along the descent direction in which the projection on the feasible set is performed according to a variable non Euclidean metric. The stationarity of the limit points of the resulting scheme has recently been proved under some general assumptions on the generalized gradient projections employed. Here we tested some examples of methods belonging to this class on a blind deconvolution problem from data affected by Poisson noise, and we illustrate the impact of the projection operator choice on the practical performances of the corresponding algorithm.
2015
- New convergence results for the scaled gradient projection method
[Articolo su rivista]
Bonettini, Silvia; Prato, Marco
abstract
The aim of this paper is to deepen the convergence analysis of the scaled gradient projection (SGP) method, proposed by Bonettini et al. in a recent paper for constrained smooth optimization. The main feature of SGP is the presence of a variable scaling matrix multiplying the gradient, which may change at each iteration. In the last few years, an extensive numerical experimentation showed that SGP equipped with a suitable choice of the scaling matrix is a very effective tool for solving large scale variational problems arising in image and signal processing. In spite of the very reliable numerical results observed, only a weak convergence theorem is provided establishing that any limit point of the sequence generated by SGP is stationary. Here, under the only assumption that the objective function is convex and that a solution exists, we prove that the sequence generated by SGP converges to a minimum point, if the scaling matrices sequence satisfies a simple and implementable condition. Moreover, assuming that the gradient of the objective function is Lipschitz continuous, we are also able to prove the O(1/k) convergence rate with respect to the objective function values. Finally, we present the results of a numerical experience on some relevant image restoration problems, showing that the proposed scaling matrix selection rule performs well also from the computational point of view.
2015
- Shearlet-based regularized ROI reconstruction in fan beam computed tomography
[Relazione in Atti di Convegno]
Bubba T., A.; Labate, D.; Zanghirati, G.; Bonettini, Silvia; Goossens, B.
abstract
Region-of-interest (ROI) reconstruction in computed tomography (CT) is a problem receiving increasing attention in the medical imaging community, due to its potential to lower exposure to X-ray radiation and to reduce the scanning time. Since the ROI reconstruction problem requires to deal with truncated projection images, classical CT reconstruction algorithms tend to become very unstable and the solution of this problem requires either ad hoc analytic formulas or more sophisticated numerical schemes. In this paper, we introduce a novel approach for ROI CT reconstruction, formulated as a convex optimization problem with a regularized functional based on shearlets or wavelets. Our numerical implementation consists of an iterative algorithm based on the scaled gradient projection method. As illustrated by numerical tests in the context of fan beam CT, our algorithm is insensitive to the location of the ROI and remains very stable also when the ROI size is rather small.
2015
- The scaled gradient projection method: an application to nonconvex optimization
[Relazione in Atti di Convegno]
Prato, Marco; La Camera, Andrea; Bonettini, Silvia; Bertero, Mario
abstract
The scaled gradient projection (SGP) method is a variable metric forward-backward algorithm designed for constrained differentiable optimization problems, as those obtained by
reformulating several signal and image processing problems according to standard statistical approaches. The main SGP features are a variable scaling matrix multiplying the gradient direction at each iteration and an adaptive steplength parameter chosen by generalizing the well-known Barzilai-Borwein rules.
An interesting result is that SGP can be exploited within an alternating minimization approach in order to address optimization problems in which the unknown can be splitted in several blocks, each with a given convex and closed feasible set. Classical examples of applications belonging to this class are the non-negative matrix factorization and the blind deconvolution problems.
In this work we applied this method to the blind deconvolution of multiple images of the same target obtained with different PSFs. In particular, for our experiments we considered the NASA funded Fizeau interferometer LBTI of the Large Binocular Telescope, which is already operating on Mount Graham and has provided the first Fizeau images, demonstrating the possibility of reaching the resolution of a 22.8m telescope. Due to the Poisson nature of the noise a®ecting the measured images, the resulting optimization problem consists in the minimization of the sum of several Kullback-Leibler divergences, constrained in suitable feasible sets accounting for the different features to be preserved in the object and the PSFs.
2014
- Accelerated gradient methods for the X-ray imaging of solar flares
[Articolo su rivista]
Bonettini, Silvia; Prato, Marco
abstract
In this paper we present new optimization strategies for the reconstruction of X-ray images of solar flares by means of the data collected by the Reuven Ramaty High Energy Solar Spectroscopic Imager (RHESSI). The imaging concept of the satellite is based of rotating modulation collimator instruments, which allow the use of both Fourier imaging approaches and reconstruction techniques based on the straightforward inversion of the modulated count profiles. Although in the last decade a greater attention has been devoted to the former strategies due to their very limited computational cost, here we consider the latter model and investigate the effectiveness of different accelerated gradient methods for the solution of the corresponding constrained minimization problem. Moreover, regularization is introduced through either an early stopping of the iterative procedure, or a Tikhonov term added to the discrepancy function, by means of a discrepancy principle accounting for the Poisson nature of the noise affecting the data. The research that led to the present paper was partially supported by a grant of group GNCS of INdAM.
2014
- Alternating minimization for Poisson blind deconvolution in astronomy
[Relazione in Atti di Convegno]
Prato, Marco; Bonettini, Silvia; A., La Camera; Rebegoldi, Simone
abstract
Although the continuous progresses in the design of devices which reduce the distorting effects of an optical system, a correct model of the point spread function (PSF) is often unavailable and in general it has to be estimated manually from a measured image. As an alternative to this approach, one can address the so-called blind deconvolution problem, in which the reconstruction of both the target distribution and the model is performed simultaneously by considering the minimization of a fit-to-data function in which both the object and the PSF are unknown. Due to the strong ill-posedness of the resulting inverse problem, suitable a priori information are needed to recover a meaningful solution, which can be included in the minimization problem under the form of constraints on the unknowns. In this work we consider a recent optimization algorithm for the solution of the blind deconvolution problem from data affected by Poisson noise, and we propose a strategy to automatically select its parameters based on a measure of the optimality condition violation. Some numerical simulations on astronomical images show that the proposed approach allows to provide reconstructions very close to those obtained by manually optimizing the algorithm parameters.
2014
- An Alternating Extragradient Method with Non Euclidean Projections for Saddle Point Problems
[Articolo su rivista]
Bonettini, S.; Ruggiero, V.
abstract
In this work we analyze a first order method especially tailored for smooth saddle point problems, based on an alternating extragradient scheme. The proposed method is based on three successive projection steps, which can be computed also with respect to non Euclidean metrics.
2014
- An alternating minimization method for blind deconvolution from Poisson data
[Relazione in Atti di Convegno]
Prato, Marco; A., La Camera; Bonettini, Silvia
abstract
Blind deconvolution is a particularly challenging inverse problem since information on both the desired target and the acquisition system have to be inferred from the measured data. When the collected data are affected by Poisson noise, this problem is typically addressed by the minimization of the Kullback-Leibler divergence, in which the unknowns are sought in particular feasible sets depending on the a priori information provided by the specific application. If these sets are separated, then the resulting constrained minimization problem can be addressed with an inexact alternating strategy. In this paper we apply this optimization tool to the problem of reconstructing astronomical images from adaptive optics systems, and we show that the proposed approach succeeds in providing very good results in the blind deconvolution of nondense stellar clusters.
2014
- An alternating minimization method for blind deconvolution in astronomy
[Poster]
Rebegoldi, Simone; Bonettini, Silvia; A., La Camera; Prato, Marco
abstract
Blind deconvolution is the problem of image deblurring when both the original object and the blur are unknown. In this work, we show a particular astronomical imaging problem, in which p images of the same astronomical object are acquired and convolved with p different Point Spread Functions (PSFs). According to the maximum likelihood approach, this becomes a constrained minimization problem with p+1 blocks of variables, whose objective function is globally non convex. Thanks to the separable structure of the constraints, the problem can be treated by means of an inexact alternating minimization method whose limit points are stationary for the function. This method has been tested on some realistic datasets and the numerical results are hereby reported to show its effectiveness on both sparse and diffuse astronomical objects.
2014
- Primal-dual first order methods for total variation image restoration in presence of Poisson noise
[Relazione in Atti di Convegno]
Bonettini, Silvia; Benfenati, A.; Ruggiero, V.
abstract
Image restoration often requires the minimization of a convex, possibly nonsmooth functional, given by the sum of a data fidelity measure plus a regularization term. In order to face the lack of smoothness, alternative formulations of the minimization problem could be exploited via the duality principle. Indeed, the primal-dual and the dual formulation have been well explored in the literature when the data suffer from Gaussian noise and, thus, the data fidelity term is quadratic. Unfortunately, the most part of the approaches proposed for the Gaussian are difficult to apply to general data discrepancy terms, such as the Kullback-Leibler divergence. In this work we propose primal-dual methods which apply to the minimization of the sum of general convex functions and whose iteration is easy to compute, regardless of the form of the objective function, since it essentially consists in a subgradient projection step. We provide the convergence analysis and we suggest some strategies to improve the convergence speed by means of a careful selection of the steplength parameters. A numerical experience on Total Variation based denoising and deblurring problems from Poisson data shows the behavior of the proposed method with respect to other state-of-the-art algorithms.
2013
- A convergent blind deconvolution method for post-adaptive-optics astronomical imaging
[Articolo su rivista]
Prato, Marco; A., La Camera; Bonettini, Silvia; M., Bertero
abstract
In this paper we propose a blind deconvolution method which applies to data perturbed by Poisson noise. The objective function is a generalized Kullback-Leibler (KL) divergence, depending on both the unknown object and unknown point spread function (PSF), without the addition of regularization terms; constrained minimization, with suitable convex constraints on both unknowns, is considered. The problem is nonconvex and we propose to solve it by means of an inexact alternating minimization method, whose global convergence to stationary points of the objective function has been recently proved in a general setting. The method is iterative and each iteration, also called outer iteration, consists of alternating an update of the object and the PSF by means of fixed numbers of iterations, also called inner iterations, of the scaled gradient projection (SGP) method. Therefore the method is similar to other proposed methods based on the Richardson-Lucy (RL) algorithm, with SGP replacing RL. The use of SGP has two advantages: first, it allows to prove global convergence of the blind method; secondly, it allows the introduction of different constraints on the object and the PSF. The specific constraint on the PSF, besides non-negativity and normalization, is an upper bound derived from the so-called Strehl ratio (SR), which is the ratio between the peak value of an aberrated versus a perfect wavefront. Therefore a typical application, but not the unique one, is to the imaging of modern telescopes equipped with adaptive optics systems for partial correction of the aberrations due to atmospheric turbulence.
In the paper we describe in detail the algorithm and we recall the results leading to its convergence. Moreover we illustrate its effectiveness by means of numerical experiments whose results indicate that the method, pushed to convergence, is very promising in the reconstruction of non-dense stellar clusters. The case of more complex astronomical targets is also considered, but in this case regularization by early stopping of the outer iterations is required. However the proposed method, based on SGP, allows the generalization to the case of differentiable regularization terms added to the KL divergence, even if this generalization is outside the scope of this paper.
2013
- A new semi-blind deconvolution approach for Fourier-based image restoration: an application in astronomy
[Articolo su rivista]
Bonettini, Silvia; Cornelio, Anastasia; Prato, Marco
abstract
The aim of this paper is to develop a new optimization algorithm for the restoration of an image starting from samples of its Fourier Transform, when only partial information about the data frequencies is provided. The corresponding constrained optimization problem is approached with a cyclic block alternating scheme, in which projected gradient methods are used to find a regularized solution. Our algorithm is then applied to the imaging of high-energy radiation emitted during a solar flare through the analysis of the photon counts collected by the NASA RHESSI satellite. Numerical experiments on simulated data show that, both in presence and in absence of statistical noise, the proposed approach provides some improvements in the reconstructions.
2013
- An image reconstruction method from Fourier data with uncertainties on the spatial frequencies
[Relazione in Atti di Convegno]
Cornelio, Anastasia; Bonettini, Silvia; Prato, Marco
abstract
In this paper the reconstruction of a two-dimensional image from a nonuniform sampling of its Fourier transform is considered, in the presence of uncertainties on the frequencies corresponding to the measured data. The problem therefore becomes a blind deconvolution, in which the unknowns are both the image to be reconstructed and the exact frequencies. The availability of information on the image and the frequencies allows to reformulate the problem as a constrained minimization of the least squares functional. A regularized solution of this optimization problem is achieved by early stopping an alternating minimization scheme. In particular, a gradient projection method is employed at each step to compute an inexact solution of the minimization subproblems. The resulting algorithm is applied on some numerical examples arising in a real-world astronomical application.
2013
- An image reconstruction method from Fourier data with uncertainties on the spatial frequencies
[Poster]
Cornelio, Anastasia; Bonettini, Silvia; Prato, Marco
abstract
In this work we develop a new optimization algorithm for image reconstruction problems from Fourier data with uncertainties on the spatial frequencies corresponding to the measured data. By considering such dependency on the frequencies as a further unknown, we obtain a so-called semi-blind deconvolution. Both the image and the spatial frequencies are obtained as solutions of a reformulated constrained optimization problem, approached by an alternating scheme. Numerical tests on simulated data, based on the imaging hardware of the NASA RHESSI satellite, show that the proposed approach provides some improvements in the reconstruction.
2013
- Scaling techniques for gradient projection-type methods in astronomical image deblurring
[Articolo su rivista]
Bonettini, Silvia; G., Landi; E., Loli Piccolomini; Zanni, Luca
abstract
The aim of this paper is to present a computational study on scaling techniques in gradient projection-type (GP-type) methods for deblurring of astronomical images corrupted by Poisson noise. In this case, the imaging problem is formulated as a non-negatively constrained minimization problem in which the objective function is the sum of a fit-to-data term, the Kullback–Leibler divergence, and a Tikhonov regularization term. The considered GP-type methods are formulated by a common iteration formula, where the scaling matrix and the step-length parameter characterize the different algorithms. Within this formulation, both first-order and Newton-like methods are analysed, with particular attention to those implementation features and behaviours relevant for the image restoration problem. The numerical experiments show that suited scaling strategies can enable the GP methods to quickly approximate accurate reconstructions and then are useful for designing effective image deblurring algorithms.
2012
- Analysis of interior point methods for edge preserving removal of Poisson noise
[Articolo su rivista]
Bonettini, S.; Ruggiero, V.
abstract
The aim of this paper is to analyze the behavior of the interior point (IP) approach for an image processing application that requires to solve a large scale nonlinear programming problem, such as the denoising of an image corrupted by Poisson noise.
2012
- On the convergence of primal-dual hybrid gradient algorithms for total variation image restoration
[Articolo su rivista]
Bonettini, S.; Ruggiero, V.
abstract
In this paper we establish the convergence of a general primal-dual method for nonsmooth convex optimization problems whose structure is typical in the imaging framework, as, for example, in the Total Variation image restoration problems.
2012
- Semi-blind deconvolution for Fourier-based image restoration
[Poster]
Cornelio, Anastasia; Bonettini, Silvia; Prato, Marco
abstract
In this work we develop a new optimization algorithm for image reconstruction problems from Fourier data with uncertanties on the spatial
frequencies corresponding to the measured data. By considering such dependency on the frequencies as a further unknown, we obtain a so-called
semi-blind deconvolution. Both the image and the spatial frequencies are obtained as solutions of a reformulated constrained optimization problem,
approached by an alternating scheme. Numerical tests on simulated data, based on the imaging hardware of the NASA RHESSI satellite, show
that the proposed approach provides some improvements in the reconstruction.
2011
- An alternating extragradient method for total variation based image restoration from Poisson data
[Articolo su rivista]
Bonettini, S.; Ruggiero, V.
abstract
Variational models are a valid tool for edge-preserving image restoration from data affected by Poisson noise. This paper deals with total variation and hypersurface regularization in combination with the Kullbach Leibler divergence as a data fidelity function. We propose an iterative method, based on an alternating extragradient scheme, which is able to solve, in a numerically stable way, the primal–dual formulation of both total variation and hypersurface regularization problems. In this method, tailored for general smooth saddle-point problems, the stepsize parameter can be adaptively computed so that the convergence of the scheme is proved under mild assumptions. In the numerical experience, we focus the attention on the artificial smoothing parameter that makes different the total variation and hypersurface regularization. A set of experiments on image denoising and deblurring problems is performed in order to evaluate the influence of this smoothing parameter on the stability of the proposed method and on the features of the restored images.
2011
- Image Reconstruction from Nonuniform Fourier Data
[Poster]
Prato, Marco; Bonettini, Silvia
abstract
In many scientific frameworks (e.g., radio and high energy astronomy, medical imaging) the data at one's disposal are encoded in the form of sparse and nonuniform samples of the desired unknown object's Fourier Transform. From the numerical point of view, reconstructing an image from sparse Fourier data is an ill-posed inverse problem in the sense of Hadamard, since there are infinite possible images which match the available Fourier samples. Moreover, the irregular distribution of such samples in the frequency space makes the use of any FFT-based reconstruction algorithm impossible, unless an interpolation and resampling (also known as gridding) procedure is previously applied to the original data. However, if the distribution of the Fourier samples in the frequency space is particularly irregular and/or the signal-to-noise ratio is poor, then the gridding step might either distort the information enclosed in the data or amplify the noise level on the re-sampled data with the result of artefacts formation and undesirable effects in the corresponding reconstructed image.This talk will deal with a different approach to the reconstruction of an image from a nonuniform sampling of its Fourier transform which acts straightly on the data without interpolation and re-sampling operations, exploiting in this way the real nature of the data themselves. In particular, we show that the minimization of the data discrepancy is equivalent to a deconvolution problem with a suitable kernel and we address its solution by means of a gradient projection method with an adaptive steplength parameter, chosen via an alternation of the two Barzilai–Borwein rules. Since the objective function involves a convolution operator, the algorithm can be effectively implemented exploiting the Fast Fourier Transform. The proposed algorithm is tested in a real-world problem, namely the restoration of X-ray images of the Sun during the solar flares by means of the datasets provided by the NASA RHESSI satellite.
2011
- Image reconstruction in astronomy, medicine and microscopy
[Poster]
Bonettini, Silvia; Prato, Marco; Ruggiero, V.; Zanella, R.; Zanghirati, G.; Zanni, Luca
abstract
The aim of the project is to develop image reconstruction methods for applications in microscopy, astronomy and medicine, and to release software especially tailored for the specific application. The image acquisition process produces a degradation of the information with both deterministic and statistical features. For this reason, an image enhancement is needed in order to remove the noise and the blurring effects. The underlying mathematical model belongs to the class of inverse problems, which are very difficult to solve, especially when the specific features of real applications are included in the model. Reliable reconstruction methods have to take into account of the statistics in the image formation process, of several physical constraints and of important features to be preserved in the restored image, as edges and details. The proposed reconstruction methods are based on constrained optimization methods which are well suited for the processing of large size images and also for the 3D case. The research group has a long-term experience in optimization methods for such kind of applications and in the realization of algorithms on parallel and distributed systems, as the GPUs.
2011
- Inexact block coordinate descent methods with application to the nonnegative matrix factorization
[Articolo su rivista]
Bonettini, S.
abstract
This work is concerned with the cyclic block coordinate descent method, or nonlinear Gauss-Seidel method, where the solution of an optimization problem is achieved by partitioning the variables in blocks and successively minimizing with respect to each block. The properties of the objective function that guarantee the convergence of such alternating scheme have been widely investigated in the literature and it is well known that, without suitable convexity hypotheses, the method may fail to locate the stationary points when more than two blocks of variables are employed.
2011
- Learning from examples: methodologies and software
[Poster]
Bonettini, Silvia; Prato, Marco; Ruggiero, V.; Zanella, R.; Zanghirati, G.; Zanni, Luca
abstract
The project’s goal is to apply advanced Machine Learning methodologies, mainly based on SVMs, and the related effective numerical methods for the solution of the underlying optimization problem. The analysis aims to identify the most suitable solution strategies for a given application context, or for a specific problem instance, by exploiting the characteristics of its mathematical model. In addition to the binary classification, also multiclass and (nonlinear) regression problems can be considered. The algorithmic study is followed by a code prototyping, with the possible development of a scalar or high-performance software, tailored to apply the studied strategies to the particular needs for the user. The research group makes its skills on Numerical Analysis, Numerical Optimization, scalar and concurrent programming available to the project.
2010
- A novel gradient projection approach for Fourier-based image restoration
[Relazione in Atti di Convegno]
Bonettini, Silvia; Prato, Marco
abstract
This work deals with the ill-posed inverse problem of reconstructing a two-dimensional image of an unknownobject starting from sparse and nonuniform measurements of its Fourier Transform. In particular, if we consider a prioriinformation about the target image (e.g., the nonnegativity of the pixels), this inverse problem can be reformulated as aconstrained optimization problem, in which the stationary points of the objective function can be viewed as the solutionsof a deconvolution problem with a suitable kernel. We propose a fast and effective gradient-projection iterative algorithmto provide regularized solutions of such a deconvolution problem by early stopping the iterations. Preliminary results on areal-world application in astronomy are presented.
2010
- Nonnegative image reconstruction from sparse Fourier data: a new deconvolution algorithm
[Articolo su rivista]
Bonettini, Silvia; Prato, Marco
abstract
This paper deals with image restoration problems where the data are nonuniform samples of the Fourier transform of the unknown object. We study the inverse problem in both semidiscrete and fully discrete formulations, and our analysis leads to an optimization problem involving the minimization of the data discrepancy under nonnegativity constraints. In particular we show that such problem is equivalent to a deconvolution problem in the image space. We propose a practical algorithm, based on the gradient projection method, to compute a regularized solution in the discrete case. The key point in our deconvolution-based approach is that the Fast Fourier Transform can be employed in the algorithm implementation without the need of preprocessing the data. A numerical experimentation on simulated and real datafrom the NASA RHESSI mission is also performed.
2010
- On the Uniqueness of the Solution of Image Reconstruction Problems with Poisson Data
[Relazione in Atti di Convegno]
Bonettini, S.; Ruggiero, V.
abstract
The paper is concerned with the uniqueness of the Maximum a Posteriori estimate for restoration problems of data corrupted by Poisson noise, when we have to minimize a combination of the generalized Kullback-Leibler divergence and a regularization penalty function. The aim of this paper is to prove the uniqueness result for 2D and 3D problems for several penalty functions, such as an edge preserving functional, a simple case of the class of Markov Random Field (MRF) regularization functionals and the classical Tikhonov regularization.
2010
- Space-D: a software for nonnegative image deconvolution from sparse Fourier data
[Software]
Bonettini, Silvia; Prato, Marco
abstract
This code deals with image restoration problems where the data are nonuniform samples of the Fourier transform of the unknown object. We propose a practical algorithm, based on the gradient projection method, to compute a regularized solution in the discrete case. The key point in our deconvolution-based approach is that the fast Fourier transform can be employed in the algorithm implementation without the need of preprocessing the data.
2009
- A scaled gradient projection method for constrained image deblurring
[Articolo su rivista]
Bonettini, Silvia; Zanella, Riccardo; Zanni, Luca
abstract
A class of scaled gradient projection methods for optimization problems with simple constraints is considered. These iterative algorithms can be useful in variational approaches to image deblurring that lead to minimized convex nonlinear functions subject to non-negativity constraints and, in some cases, toan additional flux conservation constraint. Aspecial gradient projection method is introduced that exploits effective scaling strategies and steplength updating rules, appropriately designed for improving the convergence rate. We give convergence results for this scheme and we evaluate its effectiveness by means of an extensive computational study on the minimization problems arising from the maximum likelihood approach to image deblurring. Comparisons with the standard expectation maximization algorithm and with other iterative regularization schemes are also reported to show the computational gainprovided by the proposed method.
2009
- Gradient projection approaches for optimization problems in image deblurring and denoising
[Poster]
Bonettini, Silvia; F., Benvenuto; Zanella, Riccardo; Zanni, Luca; M., Bertero
abstract
Gradient type methods are widely used approaches for nonlinearprogramming in image processing, due to their simplicity, low memory requirement and ability to provide medium-accurate solutions without excessive computational costs. In this work we discuss some improved gradient projection methods for constrained optimization problems in image deblurring and denoising. Crucial feature of these approaches is the combination of special steplength rules and scaled gradient directions, appropriately designed to achieve a better convergence rate. Convergence results are given by exploiting monotone or nonmonotone line-search strategies along the feasible direction. The effectiveness of the algorithms is evaluated on the problems arising from the maximum likelihood approach to the deconvolution of images and from the edge-preserving removal of Poisson noise. Numerical results obtained by facing large scale problems involving images of several mega-pixels on graphics processors are also reported.
2009
- Improving the angular resolution of coded aperture instruments using a modified Lucy-Richardson algorithm for deconvolution
[Relazione in Atti di Convegno]
Sambo, L.; Stephen, J. B.; Bonettini, S.; Zanghirati, G.; Frontera, F.
abstract
A problem with coded-mask telescopes is the achievable angular resolution. For example, with the standard cross-correlation (CC) analysis, the INTEGRAL IBIS/ISGRI angular resolution is about 13'. We are currently investigating an iterative Lucy-Richardson (LR) algorithm. The LR algorithm can be used effectively when the PSF is known, but little or no information is available for the noise. This algorithm maximizes the probability of the restored image, under the assumption that the noise is Poisson distributed, which is appropriate for photon noise in the data, and converges to the maximum likelihood solution. We have modified the classical LR algorithm, adding non-negative constraints. It doesn't take into account of the features leading to a difference in PSF depending on position in the field of view (dead pixels, gaps between modules etc), which are easily corrected for in the classical CC analysis, so we must correct for these either after the restoration of the image or by modifing the data before the sky reconstruction. We present some results using real IBIS data indicating the power of the proposed reconstruction algorithm.
2009
- Nonnegatively constrained image deblurring with an inexact interior point method
[Articolo su rivista]
Bonettini, S.; Serafini, T.
abstract
Nonlinear image deblurring procedures based on probabilistic considerations have been widely investigated in the literature. This approach leads to model the deblurring problem as a large scale optimization problem, with a nonlinear, convex objective function and non-negativity constraints on the sign of the variables. The interior point methods have shown in the last years to be very reliable in nonlinear programs. In this paper we propose an inexact Newton interior point (IP) algorithm designed for the solution of the deblurring problem. The numerical experience compares the IP method with another state-of-the-art method, the Lucy Richardson algorithm, and shows a significant improvement of the processing time.
2008
- Accelerated gradient methods for constrained image deblurring
[Articolo su rivista]
Bonettini, Silvia; Zanella, Riccardo; Zanni, Luca; Bertero, M.
abstract
In this paper we propose a special gradient projection method for the image deblurring problem, in the framework of the maximum likelihood approach. We present the method in a very general form and we give convergence results under standard assumptions. Then we consider the deblurring problem and the generality of the proposed algorithm allows us to add a energy conservation constraint to the maximum likelihood problem. In order to improve the convergence rate, we devise appropriate scaling strategies and steplength updating rules, especially designed for this application. The eectiveness of the method is evaluated by means of a computational study on astronomical images corrupted by Poisson noise. Comparisons with standard methods for image restoration, such as the expectation maximization algorithm, are also reported.
2007
- A nonmonotone semismooth inexact Newton method
[Articolo su rivista]
Bonettini, S.; Tinti, F.
abstract
In this work we propose a variant of the inexact Newton method for the solution of semismooth nonlinear systems of equations. We introduce a nonmonotone scheme, which couples the inexact features with the nonmonotone strategies. For the nonmonotone scheme, we present the convergence theorems. Finally, we show how we can apply these strategies in the variational inequalities context and we present some numerical examples.
2007
- Inner solvers for interior point methods for large scale nonlinear programming
[Articolo su rivista]
Bonettini, Silvia; Galligani, Emanuele; V., Ruggiero
abstract
This paper deals with the solution of nonlinear programming problems arising from elliptic control problems by an interior point scheme. At each step of the scheme, we have to solve a large scale symmetric and indefinite system; inner iterative solvers, with an adaptive stopping rule, can be used in order to avoid unnecessary inner iterations, especially when the current outer iterate is far from the solution.In this work, we analyse the method of multipliers and the preconditioned conjugate gradient method as inner solvers for interior point schemes. We discuss the convergence of the whole approach, the implementation details and report the results of numerical experimentation on a set of large scale test problems arising from the discretization of elliptic control problems. A comparison with other interior point codes is also reported.
2007
- On the solution of indefinite systems arising in nonlinear programming problems
[Articolo su rivista]
Bonettini, S.; Ruggiero, V.; Tinti, F.
abstract
This work is concerned with the convergence properties and the numerical analysis of the preconditioned conjugate gradient (PCG) method applied to the solution of indefinite linear systems arising in nonlinear optimization. Our approach is based on the choice of quasidefinite preconditioners and of a suitable factorization routine. Some theoretical and numerical results about these preconditioners are obtained. Furthermore, we show the behaviour of the PCG method for different formulations of the indefinite system and we compare the effectiveness of the proposed variants.
2007
- Some iterative methods for the solution of a symmetric indefinite KKT system
[Articolo su rivista]
Bonettini, S.; Ruggiero, V.
abstract
This paper is concerned with the numerical solution of a Karush–Kuhn–Tucker system. Such symmetric indefinite system arises when we solve a nonlinear programming problem by an Interior-Point (IP) approach. In this framework, we discuss the effectiveness of two inner iterative solvers: the method of multipliers and the preconditioned conjugate gradient method. We discuss the implementation details of these algorithms in an IP scheme and we report the results of a numerical comparison on a set of large scale test-problems arising from the discretization of elliptic control problems.
2006
- Metodi di tipo Newton interior point in ottimizzazione vincolata nonlineare di grandi dimensioni
[Articolo su rivista]
Bonettini, S.
abstract
La presente nota riguarda l'analisi e lo sviluppo di metodi interior point per la soluzione numerica di problemi di programmazione nonlineare. In particolare tale analisi viene affrontata nel contesto del metodo di Newton inesatto. Un contributo originale consiste nell'introduzione di una variante non monotona del metodo di Newton inesatto e di una classe di metodi di Newton interior point non monotoni.
2005
- A nonmonotone inexact Newton method
[Articolo su rivista]
Bonettini, Silvia
abstract
In this paper, we describe a variant of the inexact Newton method for solving nonlinear systems of equations.We define a nonmonotone inexact Newton step and a nonmonotone backtracking strategy. For this nonmonotone inexact Newton scheme, we present the convergence theorems. Finally, we show how we can apply these strategies to the inexact Newton interior-point method and we present some numerical examples.
2005
- An inexact Newton method combined with Hestenes multipliers' scheme for the solution of Karush-Kuhn-Tucker systems
[Articolo su rivista]
Bonettini, Silvia; Galligani, Emanuele; V., Ruggiero
abstract
In this work a Newton interior-point method for the solution of Karush-Kuhn-Tucker systems is presented.A crucial feature of this iterative method is the solution, at each iteration, of the inner subproblem. This subproblem is a linear-quadratic programming problem, that can solved approximately by an inner iterative method such as the Hestenes multipliers' method.A deep analysis on the choices of the parameters of the method (perturbation and damping parameters) has been done.The global convergence of the Newton interior-point method is proved when it is viewed as an inexact Newton method for the solution of nonlinear systems with restriction on the sign of some variables.The Newton interior-point method is numerically evaluated on large scale test problems arising from elliptic optimal control problems which show the effectiveness of the approach.
2005
- Inner solvers for interior point methods for large scale nonlinear programming
[Working paper]
Bonettini, Silvia; Galligani, Emanuele; Ruggiero, V.
abstract
This paper deals with the solution of nonlinear programming problems arising from elliptic control problems by an interior point scheme. At each step of the scheme, we have to solve a large scale symmetric and indefinite system; inner iterative solvers, with adaptive stopping rule, can be used in order to avoid unnecessary inner iterations, especially when the current outer iterate is far from the solution. In this work, we analyze the method of multipliers and the preconditioned conjugate gradient method as inner solvers for interior point schemes. We discuss on the convergence of the whole approach, on the implementation details and we report results of a numerical experimentation on a set of large scale test problems arising from the discretization of elliptic control problems. A comparison with other interior point codes is also reported.
2004
- Hestenes method for symmetric indefinite systems in interior-point method
[Articolo su rivista]
Bonettini, Silvia; Galligani, Emanuele; V., Ruggiero
abstract
This paper deals with the analysis and the solution of the Karush-Kuhn-Tucker (KKT) system that arises at each iteration of an Interior-Point (IP) method for minimizing a nonlinear function subject to equality and inequality constraints.This system is generally large and sparse and it can be reduced so that the coefficient matrix is still sparse, symmetric and indefinite, with size equal to the number of the primal variables and of the equality constraints. Instead of transforming this reduced system to a quasidefinite form by regularization techniques used in available codes on IP methods, under standard assumptions on the nonlinear problem, the system can be viewed as the optimality Lagrange conditions for a linear equality constrained quadratic programming problem, so that Hestenes multipliers' method can be applied. Numerical experiments on elliptic control problems with boundary and distributed control show the effectiveness of Hestenes scheme as inner solver for IP methods.