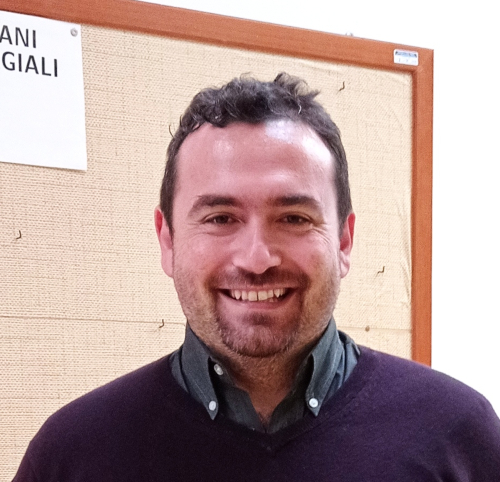 |
Marco PRATO
Professore Associato Dipartimento di Scienze Fisiche, Informatiche e Matematiche sede ex-Matematica
|
Home |
Curriculum(pdf) |
Didattica |
Pubblicazioni
2024
- A new proximal heavy ball inexact line-search algorithm
[Articolo su rivista]
Bonettini, S.; Prato, M.; Rebegoldi, S.
abstract
We study a novel inertial proximal-gradient method for composite optimization. The
proposed method alternates between a variablemetric proximal-gradient iterationwith
momentum and an Armijo-like linesearch based on the sufficient decrease of a suitable
merit function. The linesearch procedure allows for a major flexibility on the choice of
the algorithm parameters. We prove the convergence of the iterates sequence towards
a stationary point of the problem, in a Kurdyka–Łojasiewicz framework. Numerical
experiments on a variety of convex and nonconvex problems highlight the superiority
of our proposal with respect to several standard methods, especially when the inertial
parameter is selected by mimicking the Conjugate Gradient updating rule.
2023
- An abstract convergence framework with application to inertial inexact forward–backward methods
[Articolo su rivista]
Bonettini, S.; Ochs, P.; Prato, M.; Rebegoldi, S.
abstract
In this paper we introduce a novel abstract descent scheme suited for the minimization of proper and lower semicontinuous functions. The proposed abstract scheme generalizes a set of properties that are crucial for the convergence of several first-order methods designed for nonsmooth nonconvex optimization problems. Such properties guarantee the convergence of the full sequence of iterates to a stationary point, if the objective function satisfies the Kurdyka–Łojasiewicz property. The abstract framework allows for the design of new algorithms. We propose two inertial-type algorithms with implementable inexactness criteria for the main iteration update step. The first algorithm, i2Piano, exploits large steps by adjusting a local Lipschitz constant. The second algorithm, iPila, overcomes the main drawback of line-search based methods by enforcing a descent only on a merit function instead of the objective function. Both algorithms have the potential to escape local minimizers (or stationary points) by leveraging the inertial feature. Moreover, they are proved to enjoy the full convergence guarantees of the abstract descent scheme, which is the best we can expect in such a general nonsmooth nonconvex optimization setup using first-order methods. The efficiency of the proposed algorithms is demonstrated on two exemplary image deblurring problems, where we can appreciate the benefits of performing a linesearch along the descent direction inside an inertial scheme.
2023
- CTprintNet: An Accurate and Stable Deep Unfolding Approach for Few-View CT Reconstruction
[Articolo su rivista]
LOLI PICCOLOMINI, Elena; Prato, Marco; Scipione, Margherita; Sebastiani, Andrea
abstract
In this paper, we propose a new deep learning approach based on unfolded neural networks for the reconstruction of X-ray computed tomography images from few views. We start from a model-based approach in a compressed sensing framework, described by the minimization of a least squares function plus an edge-preserving prior on the solution. In particular, the proposed network automatically estimates the internal parameters of a proximal interior point method for the solution of the optimization problem. The numerical tests performed on both a synthetic and a real dataset show the effectiveness of the framework in terms of accuracy and robustness with respect to noise on the input sinogram when compared to other different data-driven approaches.
2023
- DCT-Former: Efficient Self-Attention with Discrete Cosine Transform
[Articolo su rivista]
Scribano, C.; Franchini, G.; Prato, M.; Bertogna, M.
abstract
Since their introduction the Trasformer architectures emerged as the dominating architectures for both natural language processing and, more recently, computer vision applications. An intrinsic limitation of this family of “fully-attentive” architectures arises from the computation of the dot-product attention, which grows both in memory consumption and number of operations as O(n^2) where n stands for the input sequence length, thus limiting the applications that require modeling very long
sequences. Several approaches have been proposed so far in the literature to mitigate this issue, with varying degrees of success. Our idea takes inspiration from the world of lossy data compression (such as the JPEG algorithm) to derive an approximation of the attention module by leveraging the properties of the Discrete Cosine Transform. An
extensive section of experiments shows that our method takes up less memory for the same performance, while also drastically reducing inference time. Moreover, we assume that the results of our research might serve as a starting point for a broader family of deep neural models with reduced memory footprint. The implementation will be made publicly available at https://github.com/cscribano/DCT-Former-Public.
2023
- Denoising Diffusion Models on Model-Based Latent Space
[Articolo su rivista]
Scribano, C.; Pezzi, D.; Franchini, G.; Prato, M.
abstract
With the recent advancements in the field of diffusion generative models, it has been shown that defining the generative process in the latent space of a powerful pretrained autoencoder can offer substantial advantages. This approach, by abstracting away imperceptible image details and introducing substantial spatial compression, renders the learning of the generative process more manageable while significantly reducing computational and memory demands. In this work, we propose to replace autoencoder coding with a model-based coding scheme based on traditional lossy image compression techniques; this choice not only further diminishes computational expenses but also allows us to probe the boundaries of latent-space image generation. Our objectives culminate in the proposal of a valuable approximation for training continuous diffusion models within a discrete space, accompanied by enhancements to the generative model for categorical values. Beyond the good results obtained for the problem at hand, we believe that the proposed work holds promise for enhancing the adaptability of generative diffusion models across diverse data types beyond the realm of imagery.
2023
- Explainable bilevel optimization: An application to the Helsinki deblur challenge
[Articolo su rivista]
Bonettini, Silvia; Franchini, Giorgia; Pezzi, Danilo; Prato, Marco
abstract
In this paper we present a bilevel optimization scheme for the solution of a general image deblurring problem, in which a parametric variational-like approach is encapsulated within a machine learning scheme to provide a high quality reconstructed image with automatically learned parameters. The ingredients of the variational lower level and the machine learning upper one are specifically chosen for the Helsinki Deblur Challenge 2021, in which sequences of letters are asked to be recovered from out-of-focus photographs with increasing levels of blur. Our proposed procedure for the reconstructed image consists in a fixed number of FISTA iterations applied to the minimization of an edge preserving and binarization enforcing regularized least-squares functional. The parameters defining the variational model and the optimization steps, which, unlike most deep learning approaches, all have a precise and interpretable meaning, are learned via either a similarity index or a support vector machine strategy. Numerical experiments on the test images provided by the challenge authors show significant gains with respect to a standard variational approach and performances comparable with those of some of the proposed deep learning based algorithms which require the optimization of millions of parameters.
2023
- On an iteratively reweighted linesearch based algorithm for nonconvex composite optimization
[Articolo su rivista]
Bonettini, S.; Pezzi, D.; Prato, M.; Rebegoldi, S.
abstract
In this paper we propose a new algorithm for solving a class of nonsmooth nonconvex problems, which is obtained by combining the iteratively reweighted scheme with a finite number of forward–backward iterations based on a linesearch procedure. The new method overcomes some limitations of linesearch forward–backward methods, since it can be applied also to minimize functions containing terms that are both nonsmooth and nonconvex. Moreover, the combined scheme can take advantage of acceleration techniques consisting in suitable selection rules for the algorithm parameters. We develop the convergence analysis of the new method within the framework of the Kurdyka– Lojasiewicz property. Finally, we present the results of a numerical experience on microscopy image super resolution, showing that the performances of our method are comparable or superior to those of other algorithms designed for this specific application.
2023
- Preface
[Prefazione o Postfazione]
Calatroni, L.; Donatelli, M.; Morigi, S.; Prato, M.; Rodriguez, G.; Santacesaria, M.
abstract
2022
- A fingerprint of a heterogeneous data set
[Articolo su rivista]
Spallanzani, M.; Mihaylov, G.; Prato, M.; Fontana, R.
abstract
In this paper, we describe the fingerprint method, a technique to classify bags of mixed-type measurements. The method was designed to solve a real-world industrial problem: classifying industrial plants (individuals at a higher level of organization) starting from the measurements collected from their production lines (individuals at a lower level of organization). In this specific application, the categorical information attached to the numerical measurements induced simple mixture-like structures on the global multivariate distributions associated with different classes. The fingerprint method is designed to compare the mixture components of a given test bag with the corresponding mixture components associated with the different classes, identifying the most similar generating distribution. When compared to other classification algorithms applied to several synthetic data sets and the original industrial data set, the proposed classifier showed remarkable improvements in performance.
2022
- A nested primal-dual FISTA-like scheme for composite convex optimization problems
[Articolo su rivista]
Bonettini, S.; Prato, M.; Rebegoldi, S.
abstract
We propose a nested primal–dual algorithm with extrapolation on the primal variable
suited for minimizing the sum of two convex functions, one of which is continuously
differentiable. The proposed algorithm can be interpreted as an inexact inertial
forward–backward algorithm equipped with a prefixed number of inner primal–dual
iterations for the proximal evaluation and a “warm–start” strategy for starting the
inner loop, and generalizes several nested primal–dual algorithms already available
in the literature. By appropriately choosing the inertial parameters, we prove the
convergence of the iterates to a saddle point of the problem, and provide an O(1/n)
convergence rate on the primal–dual gap evaluated at the corresponding ergodic
sequences. Numerical experiments on some image restoration problems show that
the combination of the “warm–start” strategy with an appropriate choice of the inertial
parameters is strictly required in order to guarantee the convergence to the real
minimum point of the objective function.
2022
- Biomedical Image Classification via Dynamically Early Stopped Artificial Neural Network
[Articolo su rivista]
Franchini, Giorgia; Verucchi, Micaela; Catozzi, Ambra; Porta, Federica; Prato, Marco
abstract
It is well known that biomedical imaging analysis plays a crucial role in the healthcare sector and produces a huge quantity of data. These data can be exploited to study diseases and their evolution in a deeper way or to predict their onsets. In particular, image classification represents one of the main problems in the biomedical imaging context. Due to the data complexity, biomedical image classification can be carried out by trainable mathematical models, such as artificial neural networks. When employing a neural network, one of the main challenges is to determine the optimal duration of the training phase to achieve the best performance. This paper introduces a new adaptive early stopping technique to set the optimal training time based on dynamic selection strategies to fix the learning rate and the mini-batch size of the stochastic gradient method exploited as the optimizer. The numerical experiments, carried out on different artificial neural networks for image classification, show that the developed adaptive early stopping procedure leads to the same literature performance while finalizing the training in fewer epochs. The numerical examples have been performed on the CIFAR100 dataset and on two distinct MedMNIST2D datasets which are the large-scale lightweight benchmark for biomedical image classification.
2022
- Deep Image Prior for medical image denoising, a study about parameter initialization
[Articolo su rivista]
Sapienza, Davide; Franchini, Giorgia; Govi, Elena; Bertogna, Marko; Prato, Marco
abstract
Convolutional Neural Networks are widely known and used architectures in image processing contexts, in particular for medical images. These Deep Learning techniques, known for their ability to extract high-level features, almost always require a labeled dataset, a process that can be computationally expensive. Most of the time in the biomedical context, when images are used they are noisy and the ground-truth is unknown. For this reason, and in the context of Green Artificial Intelligence, recently, an unsupervised method that employs Convolutional Neural Networks, or more precisely autoencoders, has appeared in the panorama of Deep Learning. This technique, called Deep Image Prior (DIP) by the authors, can be used in areas such as denoising, superresolution, and inpainting. Starting from these assumptions, this work analyses the robustness of these networks with respect to different types of initialization. First of all, we analyze the different types of parameters: related to the Batch Norm and the Convolutional layers. For the results, we focus on the speed of convergence and the maximum performance obtained. However, this paper aims to apply acquired information on Computer Tomography noised images. In fact, the final purpose is to test the best initializations of the first phase on a phantom image and then on a real Computer Tomography one. In fact, Computer Tomography together with Magnetic Resonance Imaging and Positron Emission Tomography are some of the diagnostic tools currently available to neuroscientists and oncologists. This work shows how initializations affect final performances and, in addition, how they should be used in the medical image reconstruction field. The section on numerical experiments shows results that on the one hand confirm the importance of a good initialization to obtain fast convergence and high performance; on the other hand, it shows how the method is robust to the processing of different image types: natural and medical. Not a single good initialization is discovered, but many of them could be chosen, according to specific necessities of the single problem.
2022
- Deep learning-assisted analysis of automobiles handling performances
[Articolo su rivista]
Sapienza, Davide; Paganelli, Davide; Prato, Marco; Bertogna, Marko; Spallanzani, Matteo
abstract
The luxury car market has demanding product development standards aimed at providing state-of-the-art features in the automotive domain. Handling performance is amongst the most important properties that must be assessed when developing a new car model. In this work, we analyse the problem of predicting subjective evaluations of automobiles handling performances from objective records of driving sessions. A record is a multi-dimensional time series describing the temporal evolution of the mechanical state of an automobile. A categorical variable quantifies the evaluations of handling properties. We describe an original deep learning system, featuring a denoising autoencoder and hierarchical attention mechanisms, that we designed to solve this task. Attention mechanisms intrinsically compute probability distributions over their inputs’
components. Combining this feature with the saliency maps technique, our system can compute heatmaps that provide a visual aid to identify the physical events conditioning its predictions.
2022
- Learning the Image Prior by Unrolling an Optimization Method
[Relazione in Atti di Convegno]
Bonettini, S.; Franchini, G.; Pezzi, D.; Prato, M.
abstract
Nowadays neural networks are omnipresent thanks to the amazing adaptability they possess, despite their poor interpretability and the difficulties they give when manipulating the parameters. On the other side, we have the classical variational approach, where the restoration is obtained as the solution of a given optimization problem. The bilevel approach is connected to both approaches and consists first in devising a parametric formulation of the variational problem, then in optimizing these parameters with respect to a given dataset of training data. In this work we analyze the classical bilevel approach in combination with unrolling techniques, where the parameters of the variational problem are trained with respect to the results obtained after a fixed number of iterations of an optimization method applied to it. This results in a large scale optimization problem which can be solved by means of stochastic methods; as we observed in our numerical experiments, the stochastic approach can produce medium accuracy results in very few epochs. Moreover, our experiments also show that the unrolling approach leads to results which are comparable with those of the original bilevel method in terms of accuracy.
2021
- A comparison of nested primal-dual forward-backward methods for Poisson image deblurring
[Relazione in Atti di Convegno]
Rebegoldi, Simone; Bonettini, Silvia; Prato, Marco
abstract
We consider an inexact version of the popular Fast Iterative Soft-Thresholding Algorithm (FISTA) suited for minimizing the sum of a differentiable convex data fidelity function plus a nondifferentiable convex regularizer whose proximal operator is not computable in closed form. The proposed method is a nested primal–dual forward–backward method inspired by the methodology developed in [10], according to which the proximal-gradient point is approximated by means of a prefixed number of inner primal-dual iterates initialized with an appropriate warmstart strategy. We report some preliminary numerical results on a weighted least squares total-variation based model for Poisson image deblurring, which show the efficiency of the proposed FISTA-like method with respect to other strategies for defining the inner loop associated to the proximal step.
2021
- Deep neural networks for inverse problems with pseudodifferential operators: an application to limited-angle tomography
[Articolo su rivista]
Bubba, T. A.; Galinier, M.; Ratti, L.; Lassas, M.; Prato, M.; Siltanen, S.
abstract
We propose a novel convolutional neural network (CNN), called PsiDONet, designed for learning pseudodifferential operators (PsiDOs) in the context of linear inverse problems. Our starting point is the Iterative Soft Thresholding Algorithm (ISTA), a well-known algorithm to solve sparsity-promoting minimization problems. We show that, under rather general assumptions on the forward operator, the unfolded iterations of ISTA can be interpreted as the successive layers of a CNN, which in turn provides fairly general network architectures that, for a specific choice of the parameters involved, allow to reproduce ISTA, or a perturbation of ISTA for which we can bound the coefficients of the filters. Our case study is the limited-angle X-ray transform and its application to limited-angle computed tomography (LA-CT). In particular, we prove that, in the case of LA-CT, the operations of upscaling, downscaling and convolution, which characterize our PsiDONet and most deep learning schemes, can be exactly determined by combining the convolutional nature of the limited angle X-ray transform and basic properties defining an orthogonal wavelet system. We test two different implementations of PsiDONet on simulated data from limited angle geometry, generated from the ellipse data set. Both implementations provide equally good and noteworthy preliminary results, showing the potential of the approach we propose and paving the way to applying the same idea to other convolutional operators which are PsiDOs or Fourier integral operators.
2020
- A hybrid interior point - Deep learning approach for poisson image deblurring
[Relazione in Atti di Convegno]
Galinier, M.; Prato, M.; Chouzenoux, E.; Pesquet, J. -C.
abstract
In this paper we address the problem of deconvolution of an image corrupted with Poisson noise by reformulating the restoration process as a constrained minimization of a suitable regularized data fidelity function. The minimization step is performed by means of an interior-point approach, in which the constraints are incorporated within the objective function through a barrier penalty and a forward-backward algorithm is exploited to build a minimizing sequence. The key point of our proposed scheme is that the choice of the regularization, barrier and step-size parameters defining the interior point approach is automatically performed by a deep learning strategy. Numerical tests on Poisson corrupted benchmark datasets show that our method can obtain very good performance when compared to a state-of-the-art variational deblurring strategy.
2020
- Convergence of inexact forward-backward algorithms using the forward-backward envelope
[Articolo su rivista]
Bonettini, S.; Prato, M.; Rebegoldi, S.
abstract
This paper deals with a general framework for inexact forward-backward algorithms aimed at minimizing the sum of an analytic function and a lower semicontinuous, subanalytic, convex term. Such framework relies on an implementable inexactness condition for the computation of the proximal operator, and a linesearch procedure which is possibly performed whenever a variable metric is allowed into the forward-backward step. The main focus of the work is the convergence of the considered scheme without additional convexity assumptions on the objective function. Toward this aim, we employ the recent concept of forward{backward envelope to define a continuously differentiable surrogate function, which coincides with the objective at its stationary points, and satisfies the so-called Kurdyka-Lojasiewicz (KL) property on its domain. We adapt the abstract convergence scheme usually exploited in the KL framework to our inexact forward-backward scheme, and prove the convergence of the iterates to a stationary point of the problem, as well as the convergence rates for the function values. Finally, we show the effectiveness and the flexibility of the proposed framework on a large-scale image restoration test problem.
2020
- Deep Unfolding of a Proximal Interior Point Method for Image Restoration
[Articolo su rivista]
Bertocchi, C.; Chouzenoux, E.; Corbineau, M. -C.; Pesquet, J. -C.; Prato, M.
abstract
Variational methods are widely applied to ill-posed inverse problems for they have the ability to embed prior knowledge about the solution. However, the level of performance of these methods significantly depends on a set of parameters, which can be estimated through computationally expensive and time-consuming methods. In contrast, deep learning offers very generic and efficient architectures, at the expense of explainability, since it is often used as a black-box, without any fine control over its output. Deep unfolding provides a convenient approach to combine variational-based and deep learning approaches. Starting from a variational formulation for image restoration, we develop iRestNet, a neural network architecture obtained by unfolding a proximal interior point algorithm. Hard constraints, encoding desirable properties for the restored image, are incorporated into the network thanks to a logarithmic barrier, while the barrier parameter, the stepsize, and the penalization weight are learned by the network. We derive explicit expressions for the gradient of the proximity operator for various choices of constraints, which allows training iRestNet with gradient descent and backpropagation. In addition, we provide theoretical results regarding the stability of the network for a common inverse problem example. Numerical experiments on image deblurring problems show that the proposed approach compares favorably with both state-of-the-art variational and machine learning methods in terms of image quality.
2020
- Efficient Block Coordinate Methods for Blind Cauchy Denoising
[Relazione in Atti di Convegno]
Rebegoldi, S.; Bonettini, S.; Prato, M.
abstract
This paper deals with the problem of image blind deconvolution in presence of Cauchy noise, a type of non-Gaussian, impulsive degradation which frequently appears in engineering and biomedical applications. We consider a regularized version of the corresponding data fidelity function, by adding the total variation regularizer on the image and a Tikhonov term on the point spread function (PSF). The resulting objective function is nonconvex with respect to both the image and PSF block, which leads to the presence of several uninteresting local minima. We propose to tackle such challenging problem by means of a block coordinate linesearch based forward backward algorithm suited for nonsmooth nonconvex optimization. The proposed method allows performing multiple forward-backward steps on each block of variables, as well as adopting variable steplengths and scaling matrices to accelerate the progress towards a stationary point. The convergence of the scheme is guaranteed by imposing a linesearch procedure at each inner step of the algorithm. We provide some practical sound rules to adaptively choose both the variable metric parameters and the number of inner iterations on each block. Numerical experiments show how the proposed approach delivers better performances in terms of efficiency and accuracy if compared to a more standard block coordinate strategy.
2020
- New convergence results for the inexact variable metric forward-backward method
[Articolo su rivista]
Bonettini, Silvia; Prato, Marco; Rebegoldi, Simone
abstract
Forward-backward methods are valid tools to solve a variety of optimization problems where the objective function is the sum of a smooth, possibly nonconvex term plus a convex, possibly nonsmooth function. The corresponding iteration is built on two main ingredients: the computation of the gradient of the smooth part and the evaluation of the proximity (or resolvent) operator associated to the convex term. One of the main difficulties, from both implementation and theoretical point of view, arises when the proximity operator is computed in an inexact way. The aim of this paper is to provide new convergence results about forward-backward methods with inexact computation of the proximity operator, under the assumption that the objective function satisfies the Kurdyka-Lojasiewicz property. In particular, we adopt an inexactness criterion which can be implemented in practice, while preserving the main theoretical properties of the proximity operator. The main result is the proof of the convergence of the iterates generated by the forward-backward algorithm in [1] to a stationary point. Convergence rate estimates are also provided. At the best of our knowledge, there exists no other inexact forward-backward algorithm with proved convergence in the nonconvex case and equipped with an explicit procedure to inexactly compute the proximity operator.
2019
- Learning image deblurring by unfolding a proximal interior point algorithm
[Relazione in Atti di Convegno]
Corbineau, M. -C.; Bertocchi, Carla; Chouzenoux, E.; Prato, M.; Pesquet, J. -C.
abstract
Image reconstruction is frequently addressed by resorting to variational methods, which account for some prior knowledge about the solution. The success of these methods, however, heavily depends on the estimation of a set of hyperparameters. Deep learning architectures are, on the contrary, very generic and efficient, but they offer very limited control over their output. In this paper we present iRestNet, a neural network architecture which combines the benefits of both approaches. iRestNet is obtained by unfolding a proximal interior point algorithm. This enables enforcing hard constraints on the pixel range of the restored image thanks to a logarithmic barrier strategy, without requiring any parameter setting. Explicit expressions for the involved proximity operator, and its differential, are derived, which allows training iRestNet with gradient descent and backpropagation. Numerical experiments on image deblurring show that the proposed approach provides good image quality results compared to state-of-the-art variational and machine learning methods.
2019
- Multiple image deblurring with high dynamic-range Poisson data
[Capitolo/Saggio]
Prato, Marco; La Camera, Andrea; Arcidiacono, Carmelo; Boccacci, Patrizia; Bertero, Mario
abstract
An interesting problem arising in astronomical imaging is the reconstruction of an image with high dynamic range, for example a set of bright point sources superimposed to smooth structures. A few methods have been proposed for dealing with this problem and their performance is not always satisfactory. In this paper we propose a solution based on the representation, already proposed elsewhere, of the image as the sum of a pointwise component and a smooth one, with different regularization for the two components. Our approach is in the framework of Poisson data and to this purpose we need efficient deconvolution methods. Therefore, first we briefly describe the application of the Scaled Gradient Projection (SGP) method to the case of different regularization schemes and subsequently we propose how to apply these methods to the case of multiple image deconvolution of high-dynamic range images, with specific reference to the Fizeau interferometer LBTI of the Large Binocular Telescope (LBT). The efficacy of the proposed methods is illustrated both on simulated images and on real images, observed with LBTI, of the Jovian moon Io. The software is available at http://www.oasis.unimore.it/site/home/software.html.
2019
- Recent advances in variable metric first-order methods
[Capitolo/Saggio]
Bonettini, S.; Porta, F.; Prato, M.; Rebegoldi, S.; Ruggiero, V.; Zanni, L.
abstract
Minimization problems often occur in modeling phenomena dealing with real-life applications that nowadays handle large-scale data and require real-time solutions. For these reasons, among all possible iterative schemes, first-order algorithms represent a powerful tool in solving such optimization problems since they admit a relatively simple implementation and avoid onerous computations during the iterations. On the other hand, a well known drawback of these methods is a possible poor convergence rate, especially showed when an high accurate solution is required. Consequently, the acceleration of first-order approaches is a very discussed field which has experienced several efforts from many researchers in the last decades. The possibility of considering a variable underlying metric changing at each iteration and aimed to catch local properties of the starting problem has been proved to be effective in speeding up first-order methods. In this work we deeply analyze a possible way to include a variable metric in first-order methods for the minimization of a functional which can be expressed as the sum of a differentiable term and a nondifferentiable one. Particularly, the strategy discussed can be realized by means of a suitable sequence of symmetric and positive definite matrices belonging to a compact set, together with an Armijo-like linesearch procedure to select the steplength along the descent direction ensuring a sufficient decrease of the objective function.
2018
- A Bregman inexact linesearch-based forward-backward algorithm for nonsmooth nonconvex optimization
[Relazione in Atti di Convegno]
Rebegoldi, S.; Bonettini, S.; Prato, M.
abstract
In this paper, we present a forward–backward linesearch–based algorithm suited for the minimization of the sum of a smooth (possibly nonconvex) function and a convex (possibly nonsmooth) term. Such algorithm first computes inexactly the proximal operator with respect to a given Bregman distance, and then ensures a sufficient decrease condition by performing a linesearch along the descent direction. The proposed approach can be seen as an instance of the more general class of descent methods presented in [1], however, unlike in [1], we do not assume the strong convexity of the Bregman distance used in the proximal evaluation. We prove that each limit point of the iterates sequence is stationary, we show how to compute an approximate proximal–gradient point with respect to a Bregman distance and, finally, we report the good numerical performance of the algorithm on a large scale image restoration problem. [1] S. Bonettini, I. Loris, F. Porta, and M. Prato 2016, Variable metric inexact line-search-based methods for nonsmooth optimization, SIAM J. Optim. 26(2), 891–921.
2018
- A block coordinate variable metric linesearch based proximal gradient method
[Articolo su rivista]
Bonettini, Silvia; Prato, Marco; Rebegoldi, Simone
abstract
In this paper we propose an alternating block version of a variable metric linesearch proximal gradient method. This algorithm addresses problems where the objective function is the sum of a smooth term, whose variables may be coupled, plus a separable part given by the sum of two or more convex, possibly nonsmooth functions, each depending on a single block of variables. Our approach is characterized by the possibility of performing several proximal gradient steps for updating every block of variables and by the Armijo backtracking linesearch for adaptively computing the steplength parameter. Under the assumption that the objective function satisfies the Kurdyka- Lojasiewicz property at each point of its domain and the gradient of the smooth part is locally Lipschitz continuous, we prove the convergence of the iterates sequence generated by the method. Numerical experience on an image blind deconvolution problem show the improvements obtained by adopting a variable number of inner block
iterations combined with a variable metric in the computation of the proximal operator.
2017
- A comparison of edge-preserving approaches for differential interference contrast microscopy
[Articolo su rivista]
Rebegoldi, Simone; Bautista, Lola; Blanc Féraud, Laure; Prato, Marco; Zanni, Luca; Plata, Arturo
abstract
In this paper we address the problem of estimating the phase from color images acquired with differential-interference-contrast microscopy. In particular, we consider the nonlinear and nonconvex optimization problem obtained by regularizing a least-squares-like discrepancy term with an edge-preserving functional, given by either the hypersurface potential or the total variation one. We investigate the analytical properties of the resulting objective functions, proving the existence of minimum points, and we propose effective optimization tools able to obtain in both the smooth and the nonsmooth case accurate reconstructions with a reduced computational demand.
2017
- On the convergence of a linesearch based proximal-gradient method for nonconvex optimization
[Articolo su rivista]
Bonettini, Silvia; Loris, Ignace; Porta, Federica; Prato, Marco; Rebegoldi, Simone
abstract
We consider a variable metric linesearch based proximal gradient method for the minimization of the sum of a smooth, possibly nonconvex function plus a convex, possibly nonsmooth term. We prove convergence of this iterative algorithm to a critical point if the objective function satisfies the Kurdyka- Lojasiewicz property at each point of its domain, under the assumption that a limit point exists. The proposed method is applied to a wide collection of image processing problems and our numerical tests show that our algorithm results to be flexible, robust and competitive when compared to recently proposed approaches able to address the optimization problems arising in the considered applications.
2016
- A cyclic block coordinate descent method with generalized gradient projections
[Articolo su rivista]
Bonettini, Silvia; Prato, Marco; Rebegoldi, Simone
abstract
The aim of this paper is to present the convergence analysis of a very general class of gradient projection methods for smooth, constrained, possibly nonconvex, optimization. The key features of these methods are the Armijo linesearch along a suitable descent direction and the non Euclidean metric employed to compute the gradient projection. We develop a very general framework from the point of view of block–coordinate descent methods, which are useful when the constraints are separable. In our numerical experiments we consider a large scale image restoration problem to illustrate the impact of the metric choice on the practical performances of the corresponding algorithm.
2016
- On the constrained minimization of smooth Kurdyka– Lojasiewicz functions with the scaled gradient projection method
[Relazione in Atti di Convegno]
Prato, Marco; Bonettini, Silvia; Loris, Ignace; Porta, Federica; Rebegoldi, Simone
abstract
The scaled gradient projection (SGP) method is a first-order optimization method applicable to the constrained minimization of smooth functions and exploiting a scaling matrix
multiplying the gradient and a variable steplength parameter to improve the convergence of the scheme. For a general nonconvex function, the limit points of the sequence generated by SGP have been proved to be stationary, while in the convex case and with some restrictions on the choice of the scaling matrix the sequence itself converges to a constrained minimum point. In this paper we extend these convergence results by showing that the SGP sequence converges to a limit point provided that the objective function satisfies the Kurdyka– Lojasiewicz property at each point of its domain and its gradient is Lipschitz continuous.
2016
- Phase estimation in Differential-Interference-Contrast (DIC) microscopy
[Relazione in Atti di Convegno]
Bautista, Lola; Rebegoldi, Simone; Blanc Féraud, Laure; Prato, Marco; Zanni, Luca; Plata, Arturo
abstract
We present a gradient-based optimization method for the estimation of a specimen’s phase function from polychromatic DIC images. The method minimizes the sum of a nonlinear least-squares discrepancy measure and a smooth approximation of the total variation. A new formulation of the gradient and a recent updating rule for the choice of the step size are both exploited to reduce computational time. Numerical simulations on two computer-generated objects show significant improvements, both in efficiency and accuracy, with respect to a more standard choice of the step size.
2016
- Poster Previews for Conference 9909: Adaptive Optics Systems V - Part 2 of 2
[Poster]
Camera, A. L.; Carbillet, M.; Prato, M.; Boccacci, P.; Bertero, M.
abstract
The Software Package AIRY (Astronomical Image Restoration in interferometrY) is a complete tool for simulation and deconvolution of astronomical data, which can be either a post-adaptive-optics image from a single dish telescope or a set of multiple images from a Fizeau interferometer. IDL-based and freely downloadable, the Software Package AIRY is a scientific package of the CAOS Problem-Solving Environment. It is made of different modules, each one performing a specific task: Simulation, pre-processing, deconvolution, and analysis of the data. We here present its last version, containing a new optimized method for the deconvolution problem based on the scaled-gradient projection (SGP) algorithm extended with different regularization functions, and a new module based on our multi-component method. Finally, we provide a few example projects describing our multi-step approach recently developed for deblurring of high dynamic range images. By using AIRY v. 7.0, users have a powerful tool for simulating the observations and for reconstructing their real data.
2016
- TV-regularized phase reconstruction in differential-interference-contrast (DIC) microscopy
[Relazione in Atti di Convegno]
Rebegoldi, Simone; Bautista, Lola; Blanc Féraud, Laure; Prato, Marco; Zanni, Luca; Plata, Arturo
abstract
In this paper we address the problem of reconstructing the phase from color images acquired with differential-interference-contrast (DIC) microscopy. In particular, we reformulate the problem as the minimization of a least-squares fidelity function regularized with of a total variation term, and we address the solution by exploiting a recently proposed inexact forward-backward approach. The effectiveness of this method is assessed on a realistic synthetic test.
2016
- The software package AIRY 7.0: new efficient deconvolution methods for post-adaptive optics data
[Relazione in Atti di Convegno]
La Camera, Andrea; Carbillet, Marcel; Prato, Marco; Boccacci, Patrizia; Bertero, Mario
abstract
The Software Package AIRY (acronym of Astronomical Image Restoration in interferometrY) is a complete tool for the simulation and the deconvolution of astronomical images. The data can be a post-adaptive-optics image of a single dish telescope or a set of multiple images of a Fizeau interferometer. Written in IDL and freely downloadable, AIRY is a package of the CAOS Problem-Solving Environment. It is made of different modules, each one performing a specific task, e.g. simulation, deconvolution, and analysis of the data. In this paper we present the last version of AIRY containing a new optimized method for the deconvolution problem based on the scaled-gradient projection (SGP) algorithm extended with different regularization functions. Moreover a new module based on our multi-component method is added to AIRY. Finally we provide a few example projects describing our multi-step method recently developed for deblurring of high dynamic range images. By using AIRY v.7.0, users have a powerful tool for simulating the observations and for reconstructing their real data. © (2016) COPYRIGHT Society of Photo-Optical Instrumentation Engineers (SPIE). Downloading of the abstract is permitted for personal use only.
2016
- Variable metric inexact line-search based methods for nonsmooth optimization
[Articolo su rivista]
Bonettini, Silvia; Loris, Ignace; Porta, Federica; Prato, Marco
abstract
We develop a new proximal--gradient method for minimizing the sum of a differentiable, possibly nonconvex, function plus a convex, possibly non differentiable, function. The key features of the proposed method are the definition of a suitable descent direction, based on the proximal operator associated to the convex part of the objective function, and an Armijo--like rule to determine the step size along this direction ensuring the sufficient decrease of the objective function. In this frame, we especially address the possibility of adopting a metric which may change at each iteration and an inexact computation of the proximal point defining the descent direction. For the more general nonconvex case, we prove that all limit points of the iterates sequence are stationary, while for convex objective functions we prove the convergence of the whole sequence to a minimizer, under the assumption that a minimizer exists. In the latter case, assuming also that the gradient of the smooth part of the objective function is Lipschitz, we also give a convergence rate estimate, showing the O(1/k) complexity with respect to the function values. We also discuss verifiable sufficient conditions for the inexact proximal point and we present the results of two numerical tests on total variation based image restoration problems, showing that the proposed approach is competitive with other state-of-the-art methods.
2015
- A blind deconvolution method for ground based telescopes and Fizeau interferometers
[Articolo su rivista]
Prato, Marco; La Camera, A.; Bonettini, Silvia; Rebegoldi, Simone; Bertero, M.; Boccacci, P.
abstract
In the case of ground-based telescopes equipped with adaptive optics systems, the point spread function (PSF) is only poorly known or completely unknown. Moreover, an accurate modeling of the PSF is in general not available. Therefore in several imaging situations the so-called blind deconvolution methods, aiming at estimating both the scientific target and the PSF from the detected image, can be useful. A blind deconvolution problem is severely ill-posed and, in order to reduce the extremely large number of possible solutions, it is necessary to introduce sensible constraints on both the scientific target and the PSF. In a previous paper we proposed a sound mathematical approach based on a suitable inexact alternating minimization strategy for minimizing the generalizedKullback-Leibler divergence, assuring global convergence. In the framework of this method we showed that an important constraint on the PSF is the upper bound which can be derived from the knowledge of its Strehl ratio. The efficacy of the approach was demonstrated by means of numerical simulations. In this paper, besides improving the previous approach by the use of a further constraint on the unknown scientific target, we extend it to the case of multiple images of the same target obtained with different PSFs. The main application we have in mind is to Fizeau interferometry. As it is known this is a special feature of the Large Binocular Telescope (LBT). Of the two expected interferometers for LBT, one, LINC-NIRVANA, is forthcoming while the other, LBTI, is already operating and has provided the first Fizeau images, demonstrating the possibility of reaching the resolution of a 22.8 m telescope. Therefore the extension of our blind method to this imaging modality seems to be timely. The method is applied to realistic simulations of imaging both by single mirrors and Fizeau interferometers. Successes and failures of the method in the imaging of stellar fields are demonstrated in simple cases. These preliminary results look promising at least in specific situations. The IDL code of the proposed method is available on request and will be included in the forthcoming version of the Software Package AIRY (v.6.1).
2015
- A convergent least-squares regularized blind deconvolution approach
[Articolo su rivista]
Cornelio, Anastasia; Porta, Federica; Prato, Marco
abstract
The aim of this work is to present a new and efficient optimization method for the solution of blind deconvolution problems with data corrupted by Gaussian noise, which can be reformulated as a constrained minimization problem whose unknowns are the point spread function (PSF) of the acquisition system and the true image. The objective function we consider is the weighted sum of the least-squares fit-to-data discrepancy and possible regularization terms accounting for specific features to be preserved in both the image and the PSF. The solution of the corresponding minimization problem is addressed by means of a proximal alternating linearized minimization (PALM) algorithm, in which the updating procedure is made up of one step of a gradient projection method along the arc and the choice of the parameter identifying the steplength in the descent direction is performed
automatically by exploiting the optimality conditions of the problem. The resulting approach is a particular case of a general scheme whose convergence to stationary points of the constrained minimization problem has been recently proved. The effectiveness of the iterative method is validated in several numerical simulations in image reconstruction problems.
2015
- A new steplength selection for scaled gradient methods with application to image deblurring
[Articolo su rivista]
Porta, Federica; Prato, Marco; Zanni, Luca
abstract
Gradient methods are frequently used in large scale image deblurring problems since they avoid the onerous computation of the Hessian matrix of the objective function. Second order information is typically sought by a clever choice of the steplength parameter defining the descent direction, as in the case of the well-known Barzilai and Borwein rules. In a recent paper, a strategy for the steplength selection approximating the inverse of some eigenvalues of the Hessian matrix has been proposed for gradient methods applied to unconstrained minimization problems. In the quadratic case, this approach is based on a Lanczos process applied every m iterations to the matrix of the gradients computed in the previous m iterations, but the idea can be extended to a general objective function. In this paper we extend this rule to the case of scaled gradient projection methods applied
to constrained minimization problems, and we test the effectiveness of the proposed strategy in image deblurring problems in both the presence and the absence of an
explicit edge-preserving regularization term.
2015
- A scaled gradient projection method for Bayesian learning in dynamical systems
[Articolo su rivista]
Bonettini, Silvia; Chiuso, A.; Prato, Marco
abstract
A crucial task in system identification problems is the selection of the most appropriate model class, and is classically addressed resorting to cross-validation or using order selection criteria based on asymptotic arguments. As recently suggested in the literature, this can be addressed in a Bayesian framework, where model complexity is regulated by few hyperparameters, which can be estimated via marginal likelihood maximization. It is thus of primary importance to design effective optimization methods to solve the corresponding optimization problem. If the unknown impulse response is modeled as a Gaussian process with a suitable kernel, the maximization of the marginal likelihood leads to a challenging nonconvex optimization problem, which requires a stable and effective solution strategy.
In this paper we address this problem by means of a scaled gradient projection algorithm, in which the scaling matrix and the steplength parameter play a crucial role to provide a meaningful solution in a computational time comparable with second order methods. In particular, we propose both a generalization of the split gradient approach to design the scaling matrix in the presence of box constraints, and an effective implementation of the gradient and objective function.
The extensive numerical experiments carried out on several test problems show that our method is very effective in providing in few tenths of a second solutions of the problems with accuracy comparable with state-of-the-art approaches. Moreover, the flexibility of the proposed strategy makes it easily adaptable to a wider range of problems arising in different areas of machine learning, signal processing and system identification.
2015
- Application of cyclic block generalized gradient projection methods to Poisson blind deconvolution
[Relazione in Atti di Convegno]
Rebegoldi, Simone; Bonettini, Silvia; Prato, Marco
abstract
The aim of this paper is to consider a modification of a block coordinate gradient projection method with Armijo linesearch along the descent direction in which the projection on the feasible set is performed according to a variable non Euclidean metric. The stationarity of the limit points of the resulting scheme has recently been proved under some general assumptions on the generalized gradient projections employed. Here we tested some examples of methods belonging to this class on a blind deconvolution problem from data affected by Poisson noise, and we illustrate the impact of the projection operator choice on the practical performances of the corresponding algorithm.
2015
- New convergence results for the scaled gradient projection method
[Articolo su rivista]
Bonettini, Silvia; Prato, Marco
abstract
The aim of this paper is to deepen the convergence analysis of the scaled gradient projection (SGP) method, proposed by Bonettini et al. in a recent paper for constrained smooth optimization. The main feature of SGP is the presence of a variable scaling matrix multiplying the gradient, which may change at each iteration. In the last few years, an extensive numerical experimentation showed that SGP equipped with a suitable choice of the scaling matrix is a very effective tool for solving large scale variational problems arising in image and signal processing. In spite of the very reliable numerical results observed, only a weak convergence theorem is provided establishing that any limit point of the sequence generated by SGP is stationary. Here, under the only assumption that the objective function is convex and that a solution exists, we prove that the sequence generated by SGP converges to a minimum point, if the scaling matrices sequence satisfies a simple and implementable condition. Moreover, assuming that the gradient of the objective function is Lipschitz continuous, we are also able to prove the O(1/k) convergence rate with respect to the objective function values. Finally, we present the results of a numerical experience on some relevant image restoration problems, showing that the proposed scaling matrix selection rule performs well also from the computational point of view.
2015
- The scaled gradient projection method: an application to nonconvex optimization
[Relazione in Atti di Convegno]
Prato, Marco; La Camera, Andrea; Bonettini, Silvia; Bertero, Mario
abstract
The scaled gradient projection (SGP) method is a variable metric forward-backward algorithm designed for constrained differentiable optimization problems, as those obtained by
reformulating several signal and image processing problems according to standard statistical approaches. The main SGP features are a variable scaling matrix multiplying the gradient direction at each iteration and an adaptive steplength parameter chosen by generalizing the well-known Barzilai-Borwein rules.
An interesting result is that SGP can be exploited within an alternating minimization approach in order to address optimization problems in which the unknown can be splitted in several blocks, each with a given convex and closed feasible set. Classical examples of applications belonging to this class are the non-negative matrix factorization and the blind deconvolution problems.
In this work we applied this method to the blind deconvolution of multiple images of the same target obtained with different PSFs. In particular, for our experiments we considered the NASA funded Fizeau interferometer LBTI of the Large Binocular Telescope, which is already operating on Mount Graham and has provided the first Fizeau images, demonstrating the possibility of reaching the resolution of a 22.8m telescope. Due to the Poisson nature of the noise a®ecting the measured images, the resulting optimization problem consists in the minimization of the sum of several Kullback-Leibler divergences, constrained in suitable feasible sets accounting for the different features to be preserved in the object and the PSFs.
2014
- Accelerated gradient methods for the X-ray imaging of solar flares
[Articolo su rivista]
Bonettini, Silvia; Prato, Marco
abstract
In this paper we present new optimization strategies for the reconstruction of X-ray images of solar flares by means of the data collected by the Reuven Ramaty High Energy Solar Spectroscopic Imager (RHESSI). The imaging concept of the satellite is based of rotating modulation collimator instruments, which allow the use of both Fourier imaging approaches and reconstruction techniques based on the straightforward inversion of the modulated count profiles. Although in the last decade a greater attention has been devoted to the former strategies due to their very limited computational cost, here we consider the latter model and investigate the effectiveness of different accelerated gradient methods for the solution of the corresponding constrained minimization problem. Moreover, regularization is introduced through either an early stopping of the iterative procedure, or a Tikhonov term added to the discrepancy function, by means of a discrepancy principle accounting for the Poisson nature of the noise affecting the data. The research that led to the present paper was partially supported by a grant of group GNCS of INdAM.
2014
- Alternating minimization for Poisson blind deconvolution in astronomy
[Relazione in Atti di Convegno]
Prato, Marco; Bonettini, Silvia; A., La Camera; Rebegoldi, Simone
abstract
Although the continuous progresses in the design of devices which reduce the distorting effects of an optical system, a correct model of the point spread function (PSF) is often unavailable and in general it has to be estimated manually from a measured image. As an alternative to this approach, one can address the so-called blind deconvolution problem, in which the reconstruction of both the target distribution and the model is performed simultaneously by considering the minimization of a fit-to-data function in which both the object and the PSF are unknown. Due to the strong ill-posedness of the resulting inverse problem, suitable a priori information are needed to recover a meaningful solution, which can be included in the minimization problem under the form of constraints on the unknowns. In this work we consider a recent optimization algorithm for the solution of the blind deconvolution problem from data affected by Poisson noise, and we propose a strategy to automatically select its parameters based on a measure of the optimality condition violation. Some numerical simulations on astronomical images show that the proposed approach allows to provide reconstructions very close to those obtained by manually optimizing the algorithm parameters.
2014
- An alternating minimization method for blind deconvolution from Poisson data
[Relazione in Atti di Convegno]
Prato, Marco; A., La Camera; Bonettini, Silvia
abstract
Blind deconvolution is a particularly challenging inverse problem since information on both the desired target and the acquisition system have to be inferred from the measured data. When the collected data are affected by Poisson noise, this problem is typically addressed by the minimization of the Kullback-Leibler divergence, in which the unknowns are sought in particular feasible sets depending on the a priori information provided by the specific application. If these sets are separated, then the resulting constrained minimization problem can be addressed with an inexact alternating strategy. In this paper we apply this optimization tool to the problem of reconstructing astronomical images from adaptive optics systems, and we show that the proposed approach succeeds in providing very good results in the blind deconvolution of nondense stellar clusters.
2014
- An alternating minimization method for blind deconvolution in astronomy
[Poster]
Rebegoldi, Simone; Bonettini, Silvia; A., La Camera; Prato, Marco
abstract
Blind deconvolution is the problem of image deblurring when both the original object and the blur are unknown. In this work, we show a particular astronomical imaging problem, in which p images of the same astronomical object are acquired and convolved with p different Point Spread Functions (PSFs). According to the maximum likelihood approach, this becomes a constrained minimization problem with p+1 blocks of variables, whose objective function is globally non convex. Thanks to the separable structure of the constraints, the problem can be treated by means of an inexact alternating minimization method whose limit points are stationary for the function. This method has been tested on some realistic datasets and the numerical results are hereby reported to show its effectiveness on both sparse and diffuse astronomical objects.
2014
- Strehl-constrained blind deconvolution of post-adaptive optics data & the Software Package AIRY, v. 6.1
[Poster]
M., Carbillet; A., La Camera; J., Deguignet; Prato, Marco; M., Bertero; E., Aristidi; P., Boccacci
abstract
We first briefly present the last version of the Software Package AIRY, version 6.1, a CAOS-based tool which includes various deconvolution methods, accelerations, regularizations, super-resolution, boundary effects reduction, PSF extraction/extrapolation, stopping rules, and constraints in the case of iterative blind deconvolution (IBD). Then, we focus on a new formulation of our Strehl-constrained (SC) IBD, here quantitatively compared to the original formulation for simulated data of FLAO/LBT in the near-infrared domain, showing their equivalence. Next, we extend the application of the original method to the visible domain with simulated data of ODISSEE/M´eO, testing also the robustness of the method with respect to the Strehl ratio (SR) estimation.
2014
- Strehl-constrained reconstruction of post-adaptive optics data & the Software Package AIRY, v. 6.1
[Relazione in Atti di Convegno]
Marcel, Carbillet; Andrea La, Camera; Jeremy, Deguigneta; Prato, Marco; Mario, Bertero; Eric, Aristidia; Patrizia, Boccacci
abstract
We first briefly present the last version of the Software Package AIRY, version 6.1, a CAOS-based tool which includes various deconvolution methods, accelerations, regularizations, super-resolution, boundary effects reduction, point-spread function extraction/extrapolation, stopping rules, and constraints in the case of iterative blind deconvolution (IBD). Then, we focus on a new formulation of our Strehl-constrained IBD, here quantitatively compared to the original formulation for simulated near-infrared data of an 8-m class telescope equipped with adaptive optics (AO), showing their equivalence. Next, we extend the application of the original method to the visible domain with simulated data of an AO-equipped 1.5-m telescope, testing also the robustness of the method
with respect to the Strehl ratio estimation.
2013
- A convergent blind deconvolution method for post-adaptive-optics astronomical imaging
[Articolo su rivista]
Prato, Marco; A., La Camera; Bonettini, Silvia; M., Bertero
abstract
In this paper we propose a blind deconvolution method which applies to data perturbed by Poisson noise. The objective function is a generalized Kullback-Leibler (KL) divergence, depending on both the unknown object and unknown point spread function (PSF), without the addition of regularization terms; constrained minimization, with suitable convex constraints on both unknowns, is considered. The problem is nonconvex and we propose to solve it by means of an inexact alternating minimization method, whose global convergence to stationary points of the objective function has been recently proved in a general setting. The method is iterative and each iteration, also called outer iteration, consists of alternating an update of the object and the PSF by means of fixed numbers of iterations, also called inner iterations, of the scaled gradient projection (SGP) method. Therefore the method is similar to other proposed methods based on the Richardson-Lucy (RL) algorithm, with SGP replacing RL. The use of SGP has two advantages: first, it allows to prove global convergence of the blind method; secondly, it allows the introduction of different constraints on the object and the PSF. The specific constraint on the PSF, besides non-negativity and normalization, is an upper bound derived from the so-called Strehl ratio (SR), which is the ratio between the peak value of an aberrated versus a perfect wavefront. Therefore a typical application, but not the unique one, is to the imaging of modern telescopes equipped with adaptive optics systems for partial correction of the aberrations due to atmospheric turbulence.
In the paper we describe in detail the algorithm and we recall the results leading to its convergence. Moreover we illustrate its effectiveness by means of numerical experiments whose results indicate that the method, pushed to convergence, is very promising in the reconstruction of non-dense stellar clusters. The case of more complex astronomical targets is also considered, but in this case regularization by early stopping of the outer iterations is required. However the proposed method, based on SGP, allows the generalization to the case of differentiable regularization terms added to the KL divergence, even if this generalization is outside the scope of this paper.
2013
- A deconvolution algorithm for imaging problems from Fourier data
[Articolo su rivista]
Prato, Marco
abstract
In this paper we address the problem of reconstructing a two-dimensional
image starting from the knowledge on nonuniform samples of its Fourier Transform. Such inverse problem has a natural semidiscrete formulation, that is analyzed together with its fully discrete counterpart. In particular, the image restoration problem in this case can be reformulated as the minimization of the data discrepancy under nonnegativity constraints, possibly with the addition of a further equality constraint on the total flux of the image. Moreover, we show that such problem is equivalent to a deconvolution in the image space, that represents a key property allowing the desing of a computationally efficient algorithm based on Fast Fourier Transforms to address its solution. Our proposal to compute a regularized solution in the discrete case involves a gradient projection method, with an adaptive choice for the steplength parameter that improves the convergence rate. A numerical experimentation on simulated data from the NASA RHESSI mission is also performed.
2013
- A new semi-blind deconvolution approach for Fourier-based image restoration: an application in astronomy
[Articolo su rivista]
Bonettini, Silvia; Cornelio, Anastasia; Prato, Marco
abstract
The aim of this paper is to develop a new optimization algorithm for the restoration of an image starting from samples of its Fourier Transform, when only partial information about the data frequencies is provided. The corresponding constrained optimization problem is approached with a cyclic block alternating scheme, in which projected gradient methods are used to find a regularized solution. Our algorithm is then applied to the imaging of high-energy radiation emitted during a solar flare through the analysis of the photon counts collected by the NASA RHESSI satellite. Numerical experiments on simulated data show that, both in presence and in absence of statistical noise, the proposed approach provides some improvements in the reconstructions.
2013
- A practical use of regularization for supervised learning with
kernel methods
[Articolo su rivista]
Prato, Marco; Zanni, Luca
abstract
In several supervised learning applications, it happens that reconstruction methods have to be applied repeatedly before being able to achieve the final solution. In these situations, the availability of learning algorithms able to provide effective predictors in a very short time may lead to remarkable improvements in the overall computational requirement. In this paper we consider the kernel ridge regression problem and we look for solutions given by a linear combination of kernel functions plus a constant term. In particular, we show that the unknown coefficents of the linear combination and the constant term can be obtained very fastly by applying specific regularization algorithms directly to the linear system arising from the Empirical Risk Minimization problem. From the numerical experiments carried out on benchmark datasets, we observed that in some cases the same results achieved after hours of calculations can be obtained in few seconds, thus showing that these strategies are very well-suited for time-consuming applications.
2013
- An image reconstruction method from Fourier data with uncertainties on the spatial frequencies
[Poster]
Cornelio, Anastasia; Bonettini, Silvia; Prato, Marco
abstract
In this work we develop a new optimization algorithm for image reconstruction problems from Fourier data with uncertainties on the spatial frequencies corresponding to the measured data. By considering such dependency on the frequencies as a further unknown, we obtain a so-called semi-blind deconvolution. Both the image and the spatial frequencies are obtained as solutions of a reformulated constrained optimization problem, approached by an alternating scheme. Numerical tests on simulated data, based on the imaging hardware of the NASA RHESSI satellite, show that the proposed approach provides some improvements in the reconstruction.
2013
- An image reconstruction method from Fourier data with uncertainties on the spatial frequencies
[Relazione in Atti di Convegno]
Cornelio, Anastasia; Bonettini, Silvia; Prato, Marco
abstract
In this paper the reconstruction of a two-dimensional image from a nonuniform sampling of its Fourier transform is considered, in the presence of uncertainties on the frequencies corresponding to the measured data. The problem therefore becomes a blind deconvolution, in which the unknowns are both the image to be reconstructed and the exact frequencies. The availability of information on the image and the frequencies allows to reformulate the problem as a constrained minimization of the least squares functional. A regularized solution of this optimization problem is achieved by early stopping an alternating minimization scheme. In particular, a gradient projection method is employed at each step to compute an inexact solution of the minimization subproblems. The resulting algorithm is applied on some numerical examples arising in a real-world astronomical application.
2013
- Deconvolution-based super-resolution for post-adaptive-optics data
[Relazione in Atti di Convegno]
M., Carbillet; A., La Camera; O., Chesneau; F., Millour; J. H. V., Girard; Prato, Marco
abstract
This article presents preliminary results on NACO/VLT images of close binary stars obtained by means of a Richardson-Lucy-based algorithm of super-resolution, where down to roughly a half-resolution element is attained, and with confirmation from VLTI observations in one of the cases treated. A new gradient method, the scaled gradient projection (SGP), permitting the acceleration of the used method, is also tested with the same scope.
2013
- Deconvolution-based super-resolution for post-adaptive-optics data
[Poster]
M., Carbillet; A., La Camera; O., Chesneau; F., Millour; J. H. V., Girard; Prato, Marco
abstract
This poster presents preliminary results on NACO/VLT images of close binary stars obtained by means of a Richardson-Lucy-based algorithm of super-resolution, where down to less than a half-resolution element is
attained, and with confirmation from VLTI observations in one of the cases treated. A new gradient method, the scaled gradient projection (SGP), permitting the acceleration of the used method, is also tested with the same scope.
2013
- Filter factor analysis of scaled gradient methods for linear least squares
[Relazione in Atti di Convegno]
Porta, F.; Cornelio, A.; Zanni, L.; Prato, M.
abstract
A typical way to compute a meaningful solution of a linear least squares problem involves the introduction of a filter factors array, whose aim is to avoid noise amplification due to the presence of small singular values. Beyond the classical direct regularization approaches, iterative gradient methods can be thought as filtering methods, due to their typical capability to recover the desired components of the true solution at the first iterations. For an iterative method, regularization is achieved by stopping the procedure before the noise introduces artifacts, making the iteration number playing the role of the regularization parameter. In this paper we want to investigate the filtering and regularizing effects of some first-order algorithms, showing in particular which benefits can be gained in recovering the filters of the true solution by means of a suitable scaling matrix.
2013
- On the filtering effect of iterative regularization algorithms for discrete inverse problems
[Articolo su rivista]
Cornelio, Anastasia; Porta, Federica; Prato, Marco; Zanni, Luca
abstract
Many real-world applications are addressed through a linear least-squares
problem formulation, whose solution is calculated by means of an iterative approach. A huge amount of studies has been carried out in the optimization field to provide the fastest methods for the reconstruction of the solution, involving choices of adaptive parameters and scaling matrices. However, in presence of an ill-conditioned model and real data, the need of a regularized solution instead of the least-squares one changed the point of view in favour of iterative algorithms able to combine a fast execution with a stable behaviour with respect to the restoration error. In this paper we analyze some classical and recent gradient approaches for the linear least-squares problem by looking at their way of filtering the singular values, showing in particular the effects of scaling matrices and non-negative constraints in recovering the correct filters of the solution. An original analysis of the filtering effect for the image deblurring problem with Gaussian noise on the data is also provided.
2013
- Point Spread Function extraction in crowdedelds using blind deconvolution
[Poster]
L., Schreiber; A., La Camera; Prato, Marco; E., Diolaiti
abstract
The extraction of the Point Spread Function (PSF) from astronomical data is an important issue for data reduction packages for stellar photometry that use PSF tting. High resolution Adaptive Optics images are characterized by a highly structured PSF that cannot be represented by any simple analytical model. Even a numerical PSF extracted from the frame can be aected by the eld crowding eects. In this paper we use blind deconvolution in order to nd an approximation of both the unknown object and the unknown PSF. In particular we adopt an iterative inexact alternating minimization method where each iteration (that we called outer iteration) consists in alternating an
update of the object and of the PSF by means of xed numbers of (inner) iterations of the Scaled Gradient Projection (SGP) method. The use of SGP allows the introduction of dierent constraints on the object and on the PSF. In particular, we introduce a constraint on the PSF which is an upper bound derived from the Strehl ratio (SR), to be provided together with the input data. In this contribution we show the photometric error dependence on the crowding, having simulated images generated with synthetic PSFs available from the Phase-A study of the E-ELT MCAO system (MAORY) and dierent crowding conditions.
2013
- Point spread function extraction in crowded fields using blind deconvolution
[Relazione in Atti di Convegno]
L., Schreiber; A., La Camera; Prato, Marco; E., Diolaiti
abstract
The extraction of the Point Spread Function (PSF) from astronomical data is an important issue for data reduction packages for stellar photometry that use PSF fitting. High resolution Adaptive Optics images are characterized by a highly structured PSF that cannot be represented by any simple analytical model. Even a numerical PSF extracted from the frame can be affected by the field crowding effects. In this paper we use blind deconvolution in order to find an approximation of both the unknown object and the unknown PSF. In particular we adopt an iterative inexact alternating minimization method where each iteration (that we call outer iteration) consists in alternating an update of the object and of the PSF by means of fixed numbers of (inner) iterations of the Scaled Gradient Projection (SGP) method. The use of SGP allows the introduction of different constraints on the object and on the PSF. In particular, we introduce a constraint on the PSF which is an upper bound derived from the Strehl ratio (SR), to be provided together with the input data. In this contribution we show the photometric error dependence on the crowding, having simulated images generated with synthetic PSFs available from the Phase-A study of the E-ELT MCAO system (MAORY) and different crowding conditions.
2013
- Scaled gradient projection methods for astronomical imaging
[Relazione in Atti di Convegno]
M., Bertero; P., Boccacci; Prato, Marco; Zanni, Luca
abstract
We describe recently proposed algorithms, denoted scaled gradient projection (SGP) methods, which provide efficient and accurate reconstructions of astronomical images. We restrict the presentation to the case of data affected by Poisson noise and of nonnegative solutions; both maximum likelihood and Bayesian approaches are considered. Numerical results are presented for discussing the practical behaviour of the SGP methods.
2012
- A practical use of regularization for supervised learning with kernel methods
[Poster]
Prato, Marco; Zanni, Luca
abstract
In several supervised learning applications, it happens that reconstruction methods have to be applied repeatedly before being able to achieve the final solution. In these situations, the availability of learning algorithms able to provide effective predictors in a very short time may lead to remarkable improvements in the overall computational requirement. Here we consider the kernel ridge regression problem and we look for predictors given by a linear combination of kernel functions plus a constant term, showing that an effective solution can be obtained very fastly by applying specific regularization algorithms directly to the linear system arising from the Empirical Risk Minimization problem.
2012
- Accuracy of funduscopy to identify true edema versus pseudoedema of the optic disc
[Articolo su rivista]
A., Carta; Favilla, Stefania; Prato, Marco; S., Bianchi Marzoli; A. A., Sadun; P., Mora
abstract
Purpose: Differential diagnosis between true optic disc edema(ODE) and optic disc pseudo-edema (PODE) remains a clinicalchallenge even for experienced neuro-ophthalmologists. The aim of the study was to assess the accuracy, sensitivity, and specificity of the funduscopic diagnosis of ODE.Methods: Observational, cross-sectional, two-center study ofsubjects referred for presumed acute ODE. Fundoscopy wasconducted in each center by two blinded neuro-ophthalmologistswho completed a forced-choice form concerning thepresence/absence of the ten clinical signs of ODE. Data from 122patients were used for modeling analysis. There were 74 patients in the ODE group and 48 patients in the PODE group. Main outcome measures were accuracy, sensitivity, and specificity in identifying true ODE from all possible combinations of ophthalmoscopic signs, provided by Support Vector Machine (SVM) analysis.Results: To identify ODE the sign SWELLING (i.e., swelling of theperipapillary retinal nerve fiber layer) had the highest accuracy(0.92; 95% CI: 0.82–0.97). All three outcomes showed littlevariation when the combination consisted of more than four signs.The best four-sign combination was: SWELLING, HEMORRHAGES,papilla ELEVATION, and CONGESTION of peripapillary vessels(accuracy = 0.93, 95% CI: 0.83–0.98; sensitivity = 0.95; andspecificity = 0.89).Conclusions: In our series of presumed ODE, 48/122 (39%) caseswere finally diagnosed as PODE. The sign SWELLING had thehighest accuracy as a single sign, and was a component of the most accurate combinations of signs. Accuracy reached a high plateau level if at least 4 (out of 10) ophthalmoscopic signs were present.
2012
- Confronto fra differenti tecniche per la determinazione dei carbonati
[Poster]
A., Piazzalunga; V., Bernardoni; E., Cuccia; P., Fermo; E., Yubero Funes; D., Massabò; U., Molteni; M. R., Perrone; P., Prati; Prato, Marco; G., Valli; I., Vassura; R., Vecchi
abstract
Il particolato carbonioso solitamente viene classificato nei suoi due costituenti principali: carbonio organico (OC) e carbonio elementare (EC). La componente carbonatica (CC) o inorganica viene infatti spesso trascurata a causa del suo basso contributo alle concentrazioni di particolato fine. In presenza di particolari sorgenti (i.e. attività cavatorie, cementifici, polveri sahariane) il carbonato può però contribuire in modo significativo alle concentrazioni di particolato. La quantificazione accurata del CC è resa difficile dal suo comportamento basico: le particelle di carbonato, una volta campionate, possono reagire con le particelle di solfato e nitrato d’ammonio, con una conseguente perdita dello ione carbonato e dello ione ammonio [1].La presenza di CC, se non opportunamente valutata, può inoltre rappresentare un importante interferente nella corretta determinazione di OC ed EC tramite la tecnica termo-ottica (TOT). In questo lavoro sono stati utilizzati i campioni provenienti da 4 diversi siti interessati da elevate concentrazioni di CC: Massa Carrara (estrazione del marmo), Elche – Spagna (cementificio), Lecce e Rimini (polveri Sahariane) e sono state confrontate diverse tecniche di misura per la quantificazione del CC. Sui campioni di Massa Carrara campionati su filtri in teflon è stata effettuata un’estrazione a diversi pH per la completa solubilizzazione del carbonato e la quantificazione del CC tramite il bilancio ionico è stata messa a confronto con quella ottenuta tramite FT-IR [2]. Sui campioni di Lecce e Rimini (filtri in fibra di quarzo) il bilancio ionico è stato confrontato con la quantificazione del CC ottenuta dalla deconvoluzione delle curve di evoluzione della CO2 dell’analisi TOT [1]. La disponibilità di particolato di diversa granulometria (PTS, PM10, PM2.5) campionato simultaneamente a Rimini ha permesso, oltre alla determinazione della distribuzione dimensionale del CC, anche la valutazione dell’influenza nell’analisi TOT dell’effetto catalitico dovuto alla presenza di carbonati. Sui campioni di Elche invece sono stati confrontati i risultati dell’analisi TOT ottenuti con due diversi protocolli NIOSH e EUSAAR_2.
2012
- Efficient deconvolution methods for astronomical imaging: algorithms and IDL-GPU codes
[Articolo su rivista]
Prato, Marco; Cavicchioli, Roberto; Zanni, Luca; P., Boccacci; M., Bertero
abstract
Context. The Richardson-Lucy (RL) method is the most popular deconvolution method in Astronomy because it preserves the number of counts and the nonnegativity of the original object. Regularization is, in general, obtained by an early stopping of RL iterations; in the case of point-wise objects such as binaries or open star clusters, iterations can be pushed to convergence. However, it is well known that RL is not an efficient method: in most cases and, in particular, for low noise levels, acceptable solutions are obtained at the cost of hundreds or thousands of iterations. Therefore, several approaches for accelerating RL have been proposed. They are mainly based on the remark that RL is a scaled gradient method for the minimization of the Kullback-Leibler (KL) divergence, or Csiszar I-divergence, which represents the data-fidelity function in the case of Poisson noise. In this framework, a line search along the descent direction is considered for reducing the number of iterations.Aims. In a recent paper, a general optimization method, denoted as scaled gradient projection (SGP) method , has been proposed for the constrained minimization of continuously differentiable convex functions. It is applicable to the nonnegative minimization of the KL divergence. If the scaling suggested by RL is used in this method, then it provides a considerable speedup of RL. Therefore the aim of this paper is to apply SGP to a number of imaging problems in Astronomy such as single image deconvolution, multiple image deconvolution and boundary effect correction.Methods. Deconvolution methods are proposed by applying SGP to the minimization of the KL divergence for the imaging problems mentioned above and the corresponding algorithms are derived and implemented in IDL. For all the algorithms several stopping rules are introduced, including one based on a recently proposed discrepancy principle for Poisson data. For a further increase of efficiency, implementation on GPU (Graphic Processing Unit) is also considered.Results. The proposed algorithms are tested on simulated images. The speedup of SGP methods with respect to the corresponding RL methods strongly depends on the problem and on the specific object to be reconstructed, and in our simulationsit ranges from about 4 to more than 30. Moreover, significant speedups up to two orders of magnitude have been observed between the serial and parallel implementations of the algorithms. The codes are available upon request.
2012
- Efficient multi-image deconvolution in astronomy
[Poster]
Cavicchioli, Roberto; Prato, Marco; Zanni, Luca; Boccacci, P.; Bertero, M.
abstract
The deconvolution of astronomical images by the Richardson-Lucy method (RLM) is extended here to the problem of multiple image deconvolution
and the reduction of boundary effects. We show the multiple image RLM in its accelerated gradient-version SGP (Scaled Gradient Projection).
Numerical simulations indicate that the approach can provide excellent results with a considerable reduction of the boundary effects. Also exploiting
GPUlib applied to the IDL code, we obtained a remarkable acceleration of up to two orders of magnitude.
2012
- Semi-blind deconvolution for Fourier-based image restoration
[Poster]
Cornelio, Anastasia; Bonettini, Silvia; Prato, Marco
abstract
In this work we develop a new optimization algorithm for image reconstruction problems from Fourier data with uncertanties on the spatial
frequencies corresponding to the measured data. By considering such dependency on the frequencies as a further unknown, we obtain a so-called
semi-blind deconvolution. Both the image and the spatial frequencies are obtained as solutions of a reformulated constrained optimization problem,
approached by an alternating scheme. Numerical tests on simulated data, based on the imaging hardware of the NASA RHESSI satellite, show
that the proposed approach provides some improvements in the reconstruction.
2011
- A regularization algorithm for decoding perceptual temporal profiles from fMRI data
[Articolo su rivista]
Prato, Marco; Favilla, Stefania; Zanni, Luca; Porro, Carlo Adolfo; Baraldi, Patrizia
abstract
In several biomedical fields, researchers are faced with regression problems that can be stated as Statistical Learning problems. One example is given by decoding brain states from functional magnetic resonance imaging (fMRI) data. Recently, it has been shown that the general Statistical Learning problem can be restated as a linear inverse problem. Hence, new algorithms were proposed to solve this inverse problem in the context of Reproducing Kernel Hilbert Spaces. In this paper, we detail one iterative learning algorithm belonging to this class, called ν-method, and test its effectiveness in a between-subjects regression framework. Specifically, our goal was to predict the perceived pain intensity based on fMRI signals, during an experimental model of acute prolonged noxious stimulation. We found that, using a linear kernel, the psychophysical time profile was well reconstructed, while pain intensity was in some cases significantly over/underestimated. No substantial differences in terms of accuracy were found between the proposed approach and one of the state-of-the-art learning methods, the Support Vector Machines. Nonetheless, adopting the ν-method yielded a significant reduction in computational time, an advantage that became more evident when a relevant feature selection procedure was implemented. The ν-method can be easily extended and included in typical approaches for binary or multiple classification problems, and therefore it seems well-suited to build effective brain activity estimators.
2011
- Composition of Fine and Coarse Particles in a coastal site of the Central Mediterranean: carbonaceous specie contributions
[Articolo su rivista]
M. R., Perrone; A., Piazzalunga; Prato, Marco; I., Carofalo
abstract
Total Suspended Particulate (TSP) and PM2.5 samples simultaneously collected at a coastal site (40.4°N; 18.1°E) in the central Mediterranean are analyzed to investigate the relative role of ions (Cl-, NO3-, SO42-, Na+, NH4+, K+, Mg2+, Ca2+) and carbonaceous species in the fine (PM2.5) and coarse (TSP-PM2.5) sampled mass and contribute to the characterization of the Central Mediterranean particulate. A methodology is described to determine carbonate carbon (CC), organic carbon (OC), and elemental carbon (EC) levels from Thermal Optical Transmittance (TOT) measurements since carbonate particles may significantly contribute to the Mediterranean particulate. We have found that CC levels vary up to 1.7 $\mu$g/m3 and 0.8 $\mu$g/m3 in the coarse and fine fraction, respectively. OC and EC levels vary up to 3.0 $\mu$g/m3 and 1.5 $\mu$g/m3, respectively in the coarse fraction, and vary within the 2.2-10 $\mu$g/m3 and 0.5-5 $\mu$g/m3 range, respectively in the fine fraction. Hence, it is shown that OC levels may be quite overestimated mainly in the coarse fraction, if the CC contribution is not accounted for. CO32- levels (calculated from CC concentrations) account on average for 6% and 10% of the fine (PM2.5) and coarse (TSP-PM2.5) sampled mass, respectively and allow balancing the anion deficit resulting from the ionic balance of ions detected by ion-chromatography (IC). Total carbon TC = (OC+EC) accounts on average for 29% and 6% of the fine and coarse mass, respectively. IC ions account for 38% and 17% of the fine and coarse mass, respectively. OC, EC, SO42-, NH4+, and K+ are the major components in the fine fraction, accounting on average for 84% of the analyzed PM2.5mass. Marine- and crust-originated ions (Cl-, Mg2+, Na+, Ca2+, CO32-) and NO3- are mainly in the coarse fraction and represent on average 83% of the analyzed coarse mass. A discussion on the main reactions leading to the loss of ammonium particulate in the coarse fraction is provided. It is also shown that the Cl-/Na+ ratio varies within the 0.1-0.8 and 0.0-1.0 range in the fine and coarse particle fraction, respectively for the occurrence of Cl depletion processes.
2011
- Deducing electron properties from hard X-ray observations
[Articolo su rivista]
E. P., Kontar; J. C., Brown; A. G., Emslie; W., Hajdas; G. D., Holman; G. J., Hurford; J., Kasparova; P. C. V., Mallik; A. M., Massone; M. L., Mcconnell; M., Piana; Prato, Marco; E. J., Schmahl; E., Suarez Garcia
abstract
Because hard X-rays represent prompt, optically-thin, radiation from energetic electrons, they are a relatively straightforward, and hence valuable, tool in the diagnostic study of flare-accelerated electrons. The observed X-ray flux (and polarization state) is fundamentally a convolution of the cross-section for the hard X-ray emission process(es) in question with the electron distribution function, which is in turn a function of energy,direction, spatial location and time. To address the problems of particle propagation and acceleration one needs to infer as much information as possible on this electron distribution function, through a deconvolution of this fundamental relationship.This review presents recent progress toward this goal using spectroscopic, imaging and polarization measurements, primarily from the Ramaty High Energy Solar Spectroscopic Imager (RHESSI). Previous conclusions regarding the energy, angular (pitch angle) and spatial distributions of energetic electrons in solar flares are critically reviewed. We discuss the role of several radiation processes: free-free electron-ion, free-free electron-electron, free-bound electron-ion, and Compton back-scatter, using both spectroscopic and imaging techniques.The unprecedented quality of the RHESSI data allows for the first time model-independent inference of not only electron energy spectra, but also the angular distributions of the X-ray-emitting electrons. In addition, RHESSI's imaging spectroscopy capability has revealed hitherto unknown details of solar flare morphology, and hard X-ray polarization measurements have imposed significant new constraints on the degree of anisotropy of theaccelerated electron distribution.
2011
- Diagnostica differenziale oftalmoscopica tra edema e pseudoedema della papilla ottica
[Relazione in Atti di Convegno]
A., Carta; P., Mora; Favilla, Stefania; Prato, Marco; S., Ghirardini; S., Bianchi Marzoli
abstract
L'edema del disco ottico (ODE: Optic Disc Edema) è un segno clinico allarmante data la sua possibile associazione con patologie che possono portare a cecità o addirittura minacciare la vita del paziente; per esempio, l'edema bilaterale della papilla ottica (papilledema) è segno di Ipertensione Intracranica, spesso dovuta alla presenza di una neoplasia intracranica in espansione. Esistono tuttavia delle condizioni oculari benigne (varianti anatomiche) che mimano un edema della papilla; tali condizioni sono responsabili di uno pseudo edema della papilla ottica (PODE). La diagnosi differenziale tra un vero edema e uno pseudoedema della testa del nervo ottico può essere difficile e problematica con misdiagnosi allarmanti che capitano frequentemente nella pratica clinica, dovute alla sovrapposizione di aspetti morfologici similari tra l'ODE e la PODE. Sottostimare un vero edema papillare in fase acuta con ritardo nella diagnosi può essere molto pericoloso per il paziente, così come una misdiagnosi a fronte di uno pseudoedema può portare ad un percorso diagnostico-terapeutico errato con costi sociali elevati, ospedalizzazione ed esami invasivi potenzialmente pericolosi e ingiustificati. Anche se l'OCT viene oggi ampliamente utilizzato in ambito specialistico, l'esame di prima esecuzione per valutare la presenza di un edema resta l'oftalmoscopia indiretta del nervo ottico.L'alterazione del flusso assonico produce delle alterazioni morfologiche sia di tipo meccanico che di tipo vascolare. Tali alterazioni rappresentano i 10 segni oftalmoscopici dell'edema della testa dell'ottico. Tuttavia, alcuni di questi segni possono essere presenti nel caso di un PODE, rendendo difficile una diagnostica differenziale, soprattutto se la valutazione oftalmoscopica viene effettuata da personale medico che non sia un neuroftalmologo, ovvero poco familiare con l'osservazione della papilla ottica. Scopo di questa relazione è quello di illustrare le laterazioni tipiche dell'ODE oltre che verificare la minima combinazione di segni oftalmoscopici necessari a garantire una accuratezza elevata per una diagnosi corretta di ODE.
2011
- Image Reconstruction from Nonuniform Fourier Data
[Poster]
Prato, Marco; Bonettini, Silvia
abstract
In many scientific frameworks (e.g., radio and high energy astronomy, medical imaging) the data at one's disposal are encoded in the form of sparse and nonuniform samples of the desired unknown object's Fourier Transform. From the numerical point of view, reconstructing an image from sparse Fourier data is an ill-posed inverse problem in the sense of Hadamard, since there are infinite possible images which match the available Fourier samples. Moreover, the irregular distribution of such samples in the frequency space makes the use of any FFT-based reconstruction algorithm impossible, unless an interpolation and resampling (also known as gridding) procedure is previously applied to the original data. However, if the distribution of the Fourier samples in the frequency space is particularly irregular and/or the signal-to-noise ratio is poor, then the gridding step might either distort the information enclosed in the data or amplify the noise level on the re-sampled data with the result of artefacts formation and undesirable effects in the corresponding reconstructed image.This talk will deal with a different approach to the reconstruction of an image from a nonuniform sampling of its Fourier transform which acts straightly on the data without interpolation and re-sampling operations, exploiting in this way the real nature of the data themselves. In particular, we show that the minimization of the data discrepancy is equivalent to a deconvolution problem with a suitable kernel and we address its solution by means of a gradient projection method with an adaptive steplength parameter, chosen via an alternation of the two Barzilai–Borwein rules. Since the objective function involves a convolution operator, the algorithm can be effectively implemented exploiting the Fast Fourier Transform. The proposed algorithm is tested in a real-world problem, namely the restoration of X-ray images of the Sun during the solar flares by means of the datasets provided by the NASA RHESSI satellite.
2011
- Image reconstruction in astronomy, medicine and microscopy
[Poster]
Bonettini, Silvia; Prato, Marco; Ruggiero, V.; Zanella, R.; Zanghirati, G.; Zanni, Luca
abstract
The aim of the project is to develop image reconstruction methods for applications in microscopy, astronomy and medicine, and to release software especially tailored for the specific application. The image acquisition process produces a degradation of the information with both deterministic and statistical features. For this reason, an image enhancement is needed in order to remove the noise and the blurring effects. The underlying mathematical model belongs to the class of inverse problems, which are very difficult to solve, especially when the specific features of real applications are included in the model. Reliable reconstruction methods have to take into account of the statistics in the image formation process, of several physical constraints and of important features to be preserved in the restored image, as edges and details. The proposed reconstruction methods are based on constrained optimization methods which are well suited for the processing of large size images and also for the 3D case. The research group has a long-term experience in optimization methods for such kind of applications and in the realization of algorithms on parallel and distributed systems, as the GPUs.
2011
- Learning from examples: methodologies and software
[Poster]
Bonettini, Silvia; Prato, Marco; Ruggiero, V.; Zanella, R.; Zanghirati, G.; Zanni, Luca
abstract
The project’s goal is to apply advanced Machine Learning methodologies, mainly based on SVMs, and the related effective numerical methods for the solution of the underlying optimization problem. The analysis aims to identify the most suitable solution strategies for a given application context, or for a specific problem instance, by exploiting the characteristics of its mathematical model. In addition to the binary classification, also multiclass and (nonlinear) regression problems can be considered. The algorithmic study is followed by a code prototyping, with the possible development of a scalar or high-performance software, tailored to apply the studied strategies to the particular needs for the user. The research group makes its skills on Numerical Analysis, Numerical Optimization, scalar and concurrent programming available to the project.
2011
- Methodology to determine carbonate carbon from Thermal Optical Transmittance measurements
[Poster]
M. R., Perrone; A., Piazzalunga; Prato, Marco
abstract
Carbonate carbon (CC) is often not considered in atmospheric aerosol chemistry studies which comprise the measurement of elemental carbon (EC) and organic carbon (OC). The reason for this may be its low contribution to fine particle mass in most area along with the difficulties in its analytical determination in atmospheric aerosol collected on filter matrices. Carbonate particles are expected to significantly contribute to the Mediterranean PM mainly during the intrusion of air masses from North Africa. However, the CC fraction in particulate matter may not be negligible if high concentrations of mineral dust, either natural (natural erosion, sand storms) or originating from street abrasion or construction sites are present. Some thermal–optical methods have recently been used to determine carbonate carbon, along with different organic fractions and EC and it has been shown that the interference of CC with the signal of EC or OC may lead to overestimations of either of these two carbon fractions during thermal-optical analysis (Karanasiou et al., 2010). The use of a sample pretreatment with HCl fumes to eliminate CC prior to the thermal analysis is suggested to avoid the interference by carbonate particles. We have used the sample pretreatment to identify the CC contribution to the flame ionization detector (FID) signal from TOT measurements and implement a numerical procedure to determine CC, EC, and OC levels. The time evolution analysis of the FID signal before and after the treatment with HCl fumes of TSP and PM2.5 samples, has revealed that the CC peak may occurs within the 220-250 s time interval and that it is characterized by a full-with-at-half-maximum (FWHM) deltat* = 25±3 s, in accordance with previous studies. We have assumed that the CC volatilization contributes to the FID signal with a pulse which can be fitted by a Gaussian function with the peak at the time ti (within the 220-250 s time interval) and the FWHM width deltati = 25±3 s, to determine CC levels. In particular, we have calculated the Gaussian function area ascribed to the CC volatilization and the area of the calibration signal, to quantify CC levels in the analyzed PM samples. This calculation has been carried out through a fitting procedure, in which the FID signal is represented as a weighted sum of Gaussian functions, S(t), through an algorithm implemented by ourselves in Matlab®. In fact, we have assumed that S(t) can be represented by a linear combination of Gaussia functions, where ai, ti and sigmai represent amplitude, peak-time and standard deviation of the Gaussian function i and N represents the total number of Gaussian functions. The FID signal has at first been interpolated with cubic splines to obtain a straightforward calculation of its first and second derivatives. Then, through the analysis of the second derivative minima we have automatically identified the number N of Gaussian functions composing S(t). A FWHM deltati = 25±3 s has been imposed to the Gaussian function ascribed to CC, in accordance with experimental results. We have assumed that CC level uncertainties are mainly due to the uncertainties of the parameters defining the Gaussian function fitting the CC volatilization signal. The implemented technique has been tested by determining CC, OC, and EC levels in 26 TSP and PM2.5 samples which have simultaneously collected over south-eastern Italy, in the Central Mediterranean. We have found that uncertainties on CC levels vary from 0.1% up to 9% and from 0.2% up to 20% in TSP and PM2.5 samples, respectively. It has also been shown that OC levels may be quite overestimated mainly in the coarse fraction, if the CC contribution is not accounted for. Figure 1 shows as an example Ca2+ and CO32- levels (calculated from CC concentrations) in the 26 analyzed TSP samples. The good correlation between Ca2+ and CO32- levels support the reliability of the implemented technique: carbonate-
2011
- SGP-IDL: a Scaled Gradient Projection method for image deconvolution in an Interactive Data Language environment
[Software]
Prato, Marco; Cavicchioli, Roberto; Zanni, Luca; Boccacci, P.; Bertero, M.
abstract
An Interactive Data Language (IDL) package for the single and multiple deconvolution of 2D images corrupted by Poisson noise, with the optional inclusion of a boundary effect correction. Following a maximum likelihood approach, SGP-IDL computes a deconvolved image by early stopping of the scaled gradient projection (SGP) algorithm for the solution of the optimization problem coming from the minimization of the generalized Kullback-Leibler divergence between the computed image and the observed image. The algorithms have been implemented also for Graphic Processing Units (GPUs).
2010
- A novel gradient projection approach for Fourier-based image restoration
[Relazione in Atti di Convegno]
Bonettini, Silvia; Prato, Marco
abstract
This work deals with the ill-posed inverse problem of reconstructing a two-dimensional image of an unknownobject starting from sparse and nonuniform measurements of its Fourier Transform. In particular, if we consider a prioriinformation about the target image (e.g., the nonnegativity of the pixels), this inverse problem can be reformulated as aconstrained optimization problem, in which the stationary points of the objective function can be viewed as the solutionsof a deconvolution problem with a suitable kernel. We propose a fast and effective gradient-projection iterative algorithmto provide regularized solutions of such a deconvolution problem by early stopping the iterations. Preliminary results on areal-world application in astronomy are presented.
2010
- A regularization algorithm for decoding perceptual profiles
[Poster]
Favilla, Stefania; Prato, Marco; Zanni, Luca; Porro, Carlo Adolfo; Baraldi, Patrizia
abstract
In this study we wished to test the feasibility of predicting the perceived pain intensity in healthy volunteers, based on fMRI signals collected during an experimental pain paradigm lasting several minutes. This model of acute prolonged (tonic) pain bears some similarities with clinically relevant conditions, such as prolonged ongoing activity in nociceptors and spontaneous fluctuations of perceived pain intensity over time.To predict individual pain profile, we tested and optimized one methodological approach based on new regularization learning algorithms on this regression problem.
2010
- Nonnegative image reconstruction from sparse Fourier data: a new deconvolution algorithm
[Articolo su rivista]
Bonettini, Silvia; Prato, Marco
abstract
This paper deals with image restoration problems where the data are nonuniform samples of the Fourier transform of the unknown object. We study the inverse problem in both semidiscrete and fully discrete formulations, and our analysis leads to an optimization problem involving the minimization of the data discrepancy under nonnegativity constraints. In particular we show that such problem is equivalent to a deconvolution problem in the image space. We propose a practical algorithm, based on the gradient projection method, to compute a regularized solution in the discrete case. The key point in our deconvolution-based approach is that the Fast Fourier Transform can be employed in the algorithm implementation without the need of preprocessing the data. A numerical experimentation on simulated and real datafrom the NASA RHESSI mission is also performed.
2010
- Space-D: a software for nonnegative image deconvolution from sparse Fourier data
[Software]
Bonettini, Silvia; Prato, Marco
abstract
This code deals with image restoration problems where the data are nonuniform samples of the Fourier transform of the unknown object. We propose a practical algorithm, based on the gradient projection method, to compute a regularized solution in the discrete case. The key point in our deconvolution-based approach is that the fast Fourier transform can be employed in the algorithm implementation without the need of preprocessing the data.
2009
- A Regularized Visibility-Based Approach to Astronomical Imaging Spectroscopy
[Articolo su rivista]
Prato, Marco; M., Piana; A. G., Emslie; G. J., Hurford; E. P., Kontar; A. M., Massone
abstract
We develop a formal procedure for the analysis of imaging spectroscopy data, i.e., remote sensing observations of the structure of a radiation source as a function of an observed parameter (e.g., radiation wavelength, frequency, or energy) and two-dimensional location in the observation plane of the instrument used. In general, imaging spectroscopy involves inversions of both spatial and spectral information. “Traditional” approaches typically proceed by performing the spatial inversion first, and then applying spectral deconvolution algorithms on a “pixel-by-pixel” basis across the source to deduce the (line-of-sight-weighted) form of the “source function” (a function involving only physical properties of the source itself) at each location in the observation plane. However, in the special case where spatial information is encoded in the form of visibilities (two-dimensional spatial Fourier transforms of the source structure), it is advantageous, both conceptually and computationally, to reverse the order of the steps in this procedure. In such an alternative approach, the spectral inversion is performed first, yielding visibilities of the unknown source function, and then these source function visibilities are spatially transformed to yield in situ information on the source, as a function of both energy and position. We illustrate the power and fidelity of this method using simulated data and apply it to hard X-ray observations of a solar flare on April 15, 2002. We also discuss briefly its broader applicability.
2009
- From BOLD-FMRI signals to the prediction of subjective pain perception through a regularization algorithm
[Relazione in Atti di Convegno]
Prato, Marco; Favilla, Stefania; Baraldi, Patrizia; Porro, Carlo Adolfo; Zanni, Luca
abstract
Functional magnetic resonance imaging, in particular theBOLD-fMRI technique, plays a dominant role in humanbrain mapping studies, mostly because of its noninvasivenessand relatively high spatio-temporal resolution.The main goal of fMRI data analysis has been to revealthe distributed patterns of brain areas involved in specificfunctions, by applying a variety of statistical methods withmodel-based or data-driven approaches. In the last years,several studies have taken a different approach, where thedirection of analysis is reversed in order to probe whetherfMRI signals can be used to predict perceptual or cognitivestates. In this study we test the feasibility of predicting theperceived pain intensity in healthy volunteers, based on fMRIsignals collected during an experimental pain paradigm lastingseveral minutes. In particular, we introduce a methodologicalapproach based on new regularization learning algorithmsfor regression problems.
2009
- Hard X-ray imaging of solar flares using interpolated visibilities
[Articolo su rivista]
A. M., Massone; A. G., Emslie; G. J., Hurford; Prato, Marco; E. P., Kontar; M., Piana
abstract
The Reuven Ramaty High Energy Solar Spectroscopic Imager (RHESSI) produces solar flare images with the finest angular and spectral resolutions ever achieved at hard X-ray energies. Because this instrument uses indirect, collimator-based imaging techniques, the native output of which is in the form of visibilities (two dimensional spatial Fourier components of the image), thedevelopment and application of robust, accurate, visibility-based image reconstruction techniques is required. Recognizing that the density of spatial-frequency (u;v) coverage by RHESSI is much sparser than that normally encountered in radio astronomy, we therefore introduce a method for image reconstruction from a relatively sparse distribution of sampled visibilities. The method involves spline interpolation at spatial frequencies less than the largest sampled frequency, and the imposition of a positivity constraint on the image to reduce the ringing effects resulting from an unconstrained Fourier transform inversion procedure.Using simulated images consisting both of assumed mathematical forms, and of the type of structure typically associated with solar flares, we validate the fidelity, accuracy and robustness with which the new procedure recovers input images. The method faithfully recovers both single and multiple sources, both compact and extended, over a dynamic range of $\sim$ 10:1.The performance of the method, which we term uv_smooth, is compared with other RHESSI image reconstruction algorithms currently in use and its advantages summarized. We also illustrate the application of the method using RHESSI observations of four solar flares.
2009
- Reconstruction of solar flare images using interpolated visibilities
[Poster]
Prato, Marco; Massone, A. M.; Piana, M.; Emslie, A. G.; Hurford, G. J.
abstract
One possibility to create images of high energy X-rays (or other radiations that cast shadows) is the use of a set of Rotational Modulation Collimators (or RMCs). The combined effect of the collimators' grids and the hardware rotation is a set of spatial Fourier components, called visibilities, sampled on spatial frequencies distributed over a set of concentric circles. I will introduce a fast and reliable method for X-ray imaging by applying an inverse FFT code to interpolated visibilities. I will also show that super-resolution effects can be obtained by utilizing a projected iterative algorithm.
2009
- Regularization Methods for the Solution of Inverse Problems in Solar X-ray and Imaging Spectroscopy
[Articolo su rivista]
Prato, Marco
abstract
Astronomical practice often requires addressing remote sensing problems, whereby the radiation emitted by a source far in the sky and measured through ‘ad hoc’ observational techniques, contains very indirect information on the physical processat the basis of the emission. The main difficulties in this investigations rely on the poor quality of the measurements and on the ill-posedness of the mathematical model describing the relation between the measured data and the target functions. In the present paper we consider a set of problems in solar physics in the framework of the NASA Ramaty High Energy Solar Spectroscopic Imager (RHESSI) mission. The data analysis activity is essentially based on the regularization theory for ill-posed inverse problems and a review of the main regularization methods applied in this analysis is given. Furthermore, we describe the main results of these applications, in the case ofboth synthetic data and real observations recorded by RHESSI.
2009
- The location of centroids in photon and electron maps of solar flares
[Articolo su rivista]
Prato, Marco; A. G., Emslie; E. P., Kontar; A. M., Massone; M., Piana
abstract
We explore the use of centroid coordinates as a means to identify the “locations” of electron–proton bremsstrahlung hard X-ray sources in solar flares. Differences between the coordinates of the electron and photon centroids are derived and explained. For electron propagation in a collision-dominated target, with either a uniform or an exponential density profile, the position of the electron centroid can be calculated analytically. We compare these analytic forms to data from a flare event on 2002 February 20. We first spectrally invert the native photon visibility data to obtain “electron visibilities,” which are in turn used to constructelectron flux images at various electron energies E. Centroids of these maps are then obtained by straightforward numerical integration over the electron maps. This comparison allows us to infer the density structure in the two compact sources visible, and we discuss the (somewhat unexpected) results thus obtained.
2008
- A visibility-based approach using regularization for imaging-spectroscopy in solar X-ray astronomy
[Articolo su rivista]
Prato, Marco; A. M., Massone; M., Piana; A. G., Emslie; G. J., Hurford; E. P., Kontar; R. A., Schwartz
abstract
The Reuven Ramaty High-Energy Solar Spectroscopic Imager (RHESSI) is a nine-collimators satellite detecting X-rays and $\gamma$-rays emitted by the Sun during flares. As thespacecraft rotates, imaging information is encoded as rapid time-variations of the detected flux. We recently proposed a method for the construction of electron flux maps at different electron energies from sets of count visibilities (i.e., direct, calibrated measurements of specific Fourier components of the source spatial structure) measured by RHESSI. The method requires the application of regularized inversion for the synthesis of electron visibility spectra and of imaging techniques for the reconstruction of two-dimensional electron flux maps. The method, already tested on real events registered by RHESSI, is validated in this paper by means of simulated realistic data.
2008
- Construction of electron flux images in solar flares using interpolated visibilities
[Poster]
Emslie, A. G.; Massone, A. M.; Piana, M.; Prato, Marco; Hurford, G. J.
abstract
We report on a novel method for recovering both hard X-ray images, and also their corresponding "electron flux images" from RHESSI solar flare data. The method uses the discrete set of visibilities (spatial Fourier components) observed by RHESSI and an interpolation algorithm to generate smoothed visibilities over a wide range of spatial frequencies. These smoothed visibilities are then converted to images through standard imaging algorithms. Preliminary results show that this method is capable of recovering features in simulated images over a wider dynamic range than in possible with the discrete visibility set alone. Following the procedures in Massone et al. (2007, Ap. J., 670, 857), the resulting visibilities can be used to determine variations in the electron flux spectrum throughout the flare volume.
2008
- Determining the spatial variation of accelerated electron spectra in solar flares
[Relazione in Atti di Convegno]
A. G., Emslie; G. J., Hurford; E. P., Kontar; A. M., Massone; M., Piana; Prato, Marco; Y., Xu
abstract
The RHESSI spacecraft images hard X-ray emission from solar flares with an angular resolution down to $sim$ 2" and an energy resolution of 1 keV. In principle, images in different count energy bands may be combined to derive the hard X-ray spectrum for different features in the flare and hence to determine the variation of the (line-of-sight-integrated) electron spectrum with position in the image. However, images in different count energy bands are each subject to an independent level of statistical noise. Because of the inherent ill-posedness of the count $
ightarrow$ electron spectral inversion problem, this noise is considerably amplified upon spectral inversion to obtain the parent electron spectrum for the feature, to the point where unphysical features can emerge. Imaging information is gathered by RHESSI’s Rotating Modulation Collimator (RMC) imagingsystem. For such an instrument, spatial information is gathered not as a set of spatial images, but rather as a set of (energy-dependent) spatial Fourier components (termed visibilities). We report here on a novel technique which uses these spatial Fourier components in count space to derive, via a regularized spectral inversion process, the corresponding spatial Fourier components for the electron distribution, in such a way that the resulting electron visibilities, and so the images that are constructed from them, vary smoothly with electron energy E. "Stacking" such images then results in smooth, physically plausible, electron spectra for prominent features in the flare. Application of visibility-based analysis techniques has also permitted an assessment of the density and volume of the electron acceleration region, and so the number of particles it contains. This, plus information on the rate of particle acceleration to hard-X-ray-producing energies [obtained directly from the hard X-ray spectrum $I(epsilon)$] allows us to deduce the specific acceleration rate (particles $s^{-1}$ per particle). The values of this key quantity are compared with the predictions of variouselectron acceleration scenarios.
2008
- Imaging spectroscopy of hard X-ray sources in solar flares using regularized analysis of source visibilities
[Relazione in Atti di Convegno]
A. M., Massone; M., Piana; Prato, Marco
abstract
The Reuven Ramaty High-Energy Solar Spectroscopic Imager (RHESSI) uses rotational modulation synthesis for imaging hard X-ray flares with unprecedented spatial and spectral resolution. As the spacecraft rotates, imaging information is encoded as rapid time-variations of the detected flux. We introduce a novel method for imaging spectroscopy analysis of hard X-ray emission which reconstructs electron flux maps at different energies involving regularized inversion of X-ray count visibility spectra (i.e., direct, calibrated measurements of specific Fourier components of the source spatial structure). Starting from the reconstructed electron images it is possible to extract and compare electron flux spectra from different regions, which is a crucial step for the comprehension of the acceleration mechanisms during the solar flare.
2008
- Inverse problems in machine learning: an application to brain activity interpretation
[Articolo su rivista]
Prato, Marco; Zanni, Luca
abstract
In a typical machine learning problem one has to build a model from a finite training set which is able to generalize the properties characterizing the examples of the training set to new examples. The model has to reflect as much as possible the set of trainingexamples but, especially in real-world problems in which the data are often corrupted by different sources of noise, it has to avoid a too strict dependence on the training examples themselves. Recent studies on the relationship between this kind of learning problem and the regularization theory for ill-posed inverse problems have given rise to new regularized learning algorithms. In this paper we recall some of these learning methods and we propose an accelerated version of the classical Landweber iterative scheme which results particularly efficient from thecomputational viewpoint. Finally, we compare the performances of these methods with the classical Support Vector Machines learning algorithm on a real-world experiment concerning brain activity interpretation through the analysis of functional magnetic resonance imaging data.
2008
- Parametrical imaging in solar astronomy using visibilities
[Abstract in Atti di Convegno]
M., Piana; A. M., Massone; A. G., Emslie; G. J., Hurford; E. P., Kontar; Prato, Marco; R. A., Schwartz
abstract
Visibilities are calibrated measurements of sampled Fourier components of a source distribution. In astronomical imaging they are provided, for instance, by rotation modulationcollimators in X-ray imaging or by pairs of antennas in a radio interferometer. Here we present a novel technique which allows the imaging of important physical parameters in the source fromthe analysis of measured visibilities. This method is based on the application of a regularization approach in the spatial frequency domain. We show its effectiveness in an application to X-raysolar imaging spectroscopy with real visibilities measured by the Reuven Ramaty High Energy Solar Spectroscopy Imager (RHESSI).
2008
- Predicting subjective pain perception based on BOLD-fMRI signals: a new machine learning approach
[Relazione in Atti di Convegno]
Favilla, Stefania; Prato, Marco; Zanni, Luca; Porro, Carlo Adolfo; Baraldi, Patrizia
abstract
Functional magnetic resonance imaging, in particular the BOLD-fMRI technique, plays a dominant role in human brain mapping studies, mostly because of its non-invasiveness, good spatial and acceptable temporal resolution in comparison with other techniques. The main goal of fMRI data analysis has been to reveal the distributed patterns of brain areas involved in specific functions and their interactions, by applying a variety of univariate or multivariate statistical methods with model-basedor data-driven approaches. In the last few years, a growing number of studies have taken a different approach, where the direction of analysis is reversed in order to probe whether fMRI signals can be used to predict perceptual or cognitive states. In this study we wished to test the feasibility of predicting the perceived pain intensity in healthy volunteers, based on fMRI signals collected during an experimental pain paradigm lasting several minutes. To this end, we tested and optimized one methodological approach based on new regularization learning algorithms on this regression problem.
2008
- Regularized solution of the solar bremsstrahlung inverse problem: model dependence and implementation issues
[Articolo su rivista]
A. M., Massone; M., Piana; Prato, Marco
abstract
We address an important inverse problem in solar plasma physics by means of an 'ad hoc' implementation of the Tikhonov regularization method. Our approach is validated in the case of different modifications of the mathematical model of the problem based on physical motivations, and for data sets obtained with synthetic experiments. Finally, an application to a real observation recorded by the NASA mission 'Reuven Ramaty High Energy SolarSpectroscopic Imager (RHESSI)' is considered.
2007
- Determination of electron flux spectrum images in solar flares using regularized analysis of hard X-ray source visibilities
[Abstract in Rivista]
A. G., Emslie; M., Piana; A. M., Massone; G. J., Hurford; Prato, Marco; E. P., Kontar; R. A., Schwartz
abstract
We introduce a new method for imaging spectroscopy analysis of hard X-ray emission during solar flares. The new method allows the construction of images of both count and electron flux spectra that are smoothed with respect to energy, and so more suitable for further analysis. The procedure involves regularized inversion of the count visibility spectra (i.e., the two-dimensional spatial Fourier transforms of the spectral image) to obtain smoothed forms of the corresponding electron visibility spectra. We apply the method to a solar flare observed on February 20, 2002 by the RHESSI instrument. The event is characterized by two bright footpoints with a "strand" of more diffuse emission between them. We find that the electron flux spectra at the footpoints are systematically harder than those in the region between the footpoints, and that the observed degree of hardening is consistent with that produced by Coulomb collisions between an acceleration site high in the corona and the dense chromospheric footpoint regions.
2007
- Electron flux maps of solar flares: a regularization approach to RHESSI imaging spectroscopy
[Relazione in Atti di Convegno]
A. M., Massone; M., Piana; Prato, Marco; A. G., Emslie; G. J., Hurford; E. P., Kontar; R. A., Schwartz
abstract
Reuven Ramaty High Energy Solar Spectroscopic Imager (RHESSI) is a nine-collimators satellite detecting X–rays and $\gamma$–rays emitted by the Sun during flares. We describe a novel method for the construction of electron flux maps atdifferent electron energies from sets of count visibilities measured by RHESSI. The method requires the application of regularized inversion for the synthesis of electron visibility spectra and of imaging techniques for the reconstruction of two-dimensional electron flux maps. From a physical viewpoint this approach allows the determination of spatially resolved electron spectra whose information content is fundamental for the comprehension of the acceleration mechanisms during the flaring events.
2007
- Electron flux spectral imaging of solar flares through regularized analysis of hard X-ray source visibilities
[Articolo su rivista]
M., Piana; A. M., Massone; G. J., Hurford; Prato, Marco; A. G., Emslie; E. P., Kontar; R. A., Schwartz
abstract
We introduce a new method for imaging spectroscopy analysis of hard X-ray emission during solar flares. The method avoids the "traditional" noise-sensitive step of stacking independent images made in different count-based energy intervals. Rather, it involves regularized inversion of the count visibility spectra (i.e., the two-dimensional spatial Fourier transforms of the spectral image) to obtain smoothed (regularized) forms of the corresponding electron visibility spectra. Application of conventional visibility-based imaging algorithms then yields images of the electron flux that vary smoothly with energy. We apply the method to a solar flare observed on 2002 February 20 by the RHESSI instrument. The event is characterized by two bright footpoints with a more diffuse emission between them.Analysis of the regularized electron flux images reveals that the electron flux spectra at the footpoints are systematically harder than those in the region between the footpoints and that the observed degree of hardening is consistent with that produced by Coulomb collisions between an acceleration site high in the corona and the dense chromospheric footpoint regions.
2007
- Electron-electron bremsstrahlung emission and the inference of electron flux spectra in solar flares
[Articolo su rivista]
E. P., Kontar; A. G., Emslie; A. M., Massone; M., Piana; J. C., Brown; Prato, Marco
abstract
Although both electron-ion and electron-electron bremsstrahlung contribute to the hard X-ray emission from solar flares, the latter is normally ignored. Such an omission is not justified at electron (and photon) energies above 300 keV, and inclusion of the additional electron-electron bremsstrahlung in general makes the electron spectrum required to produce a given hard X-ray spectrum steeper at high energies. Unlike electron-ion bremsstrahlung, electron-electron bremsstrahlung cannot produce photons of all energies up to the electron energy involved. The maximum possible photon energy depends on the angle between the direction of the emitting electron and the emitted photon, and this suggests a diagnostic for an upper cutoff energy and/or for the degree of beaming of the accelerated electrons. We analyze the large event of 2005 January 17 and show that the upward break around 400 keV in the observed hard X-ray spectrum is naturally accounted for by the inclusion of electron-electron bremsstrahlung. Indeed, the mean source electron spectrum recovered through a regularized inversion of the hard X-ray spectrum, using a cross section that includes both electron-ion and electron-electron terms, has a relatively constant spectral index $\delta$ over the range from electron kinetic energy E $\sim$ 200 keV to E $\sim$ 1 MeV. Such a spectrum is indicative of an acceleration mechanism without a characteristic energy or corresponding scale.
2007
- On recent machine learning algorithms for brain activity interpretation
[Poster]
Prato, Marco; Zanni, Luca; G., Zanghirati
abstract
In several biomedical and bioinformatics applications, one is faced with regression problems that can be stated as Statistical Learning problems. One example is given by the brain activity interpretation through the analysis of functional Magnetic Resonance Imaging (fMRI) data. In recent years it has been shown that the general Statistical Learning problem can be restated as a linear inverse problem. Hence, new algorithms have been proposed to solve this inverse problem in the context of reproducing kernel Hilbert spaces. These new proposals involve a numerical approach which differs from the ones concerning the classical machine learning techniques and which seems mainly suitable for the just described classes of problems; thus, it is worth to explore how effectively these new algorithms perform when compared with well-known, standard machine learning approaches. The paper will then deal with an experimentation of the new methods on a real-world experiment, as well as with a performance comparison with the support vector machines (SVMs) technique.
2007
- Raggi X dal sole: un approccio matematico alla spettroscopia per immagini
[Articolo su rivista]
M., Piana; A. M., Massone; Prato, Marco
abstract
2006
- Regularized reconstruction of the differential emission measure from solar flare hard X-ray spectra
[Articolo su rivista]
Prato, Marco; M., Piana; J. C., Brown; A. G., Emslie; E. P., Kontar; A. M., Massone
abstract
We address the problem of how to test whether an observed solar hard X-ray bremsstrahlung spectrum ($I(\epsilon)$) is consistent with a purely thermal (locally Maxwellian) distribution of source electrons, and, if so, how to reconstruct the corresponding differential emission measure ($\xi(T)$). Unlike previous analysis based on the Kramers and Bethe-Heitler approximations to the bremsstrahlung cross-section, here we use an exact (solid-angle-averaged) cross-section. We show that the problem of determining $\xi(T)$ from measurements of $I(\epsilon)$ involves two successive inverse problems: the first, to recover the mean source-electron flux spectrum ($F(E)$) from $I(\epsilon)$ and the second, to recover $\xi(T)$ from $F(E)$. We discuss the highly pathological numerical properties of this second problem within the framework of the regularization theory for linear inverse problems. In particular, we show that an iterative scheme with a positivity constraint is effective in recovering $\delta$-like forms of $\xi(T)$ while first-order Tikhonov regularization with boundary conditions works well in the case of power-law-like forms. Therefore, we introduce a restoration approach whereby the low-energy part of $F(E)$,dominated by the thermal component, is inverted by using the iterative algorithm with positivity, while the high-energy part, dominated by the power-law component, is inverted by using first-order regularization. This approach is first tested by using simulated $F(E)$ derived from a priori known forms of $\xi(T)$ and then applied to hard X-ray spectral data from the Reuven Ramaty High Energy Solar Spectroscopic Imager (RHESSI).
2004
- Anisotropic bremsstrahlung emission and the form of regularized electron flux spectra in solar flares
[Articolo su rivista]
A. M., Massone; A. G., Emslie; E. P., Kontar; M., Piana; Prato, Marco; J. C., Brown
abstract
The cross section for bremsstrahlung photon emission in solar flares is, in general, a function of the angle $\theta$ between the incoming electron and the outgoing photon directions. Thus the electron spectrum required to produce a given photon spectrum is a function of this angle, which is related to the position of the flare on the solar disk and the direction(s) of the precollision electrons relative to the local solar vertical. We compare meanelectron flux spectra for the flare of 2002 August 21 using cross sections for parameterized ranges of the angle $\theta$.Implications for the shape of the mean source electron spectrum and for the injected power in nonthermal electrons are discussed.