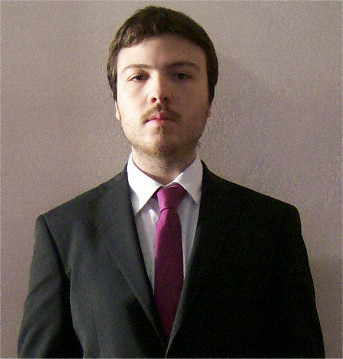 |
MARCELLO PIETRI
Ricercatore t.d. art. 24 c. 3 lett. A Dipartimento di Scienze e Metodi dell'Ingegneria Docente a contratto Dipartimento di Scienze e Metodi dell'Ingegneria
|
Home |
Curriculum(pdf) |
Didattica |
Pubblicazioni
2023
- 5G MEC Architecture for Vulnerable Road Users Management Through Smart City Data Fusion
[Relazione in Atti di Convegno]
Rossini, Enrico; Pietri, Marcello; Cavicchioli, Roberto; Picone, Marco; Mamei, Marco; Querio, Roberto; Colazzo, Laura; Procopio, Roberto
abstract
2015
- A scalable monitor for large systems
[Relazione in Atti di Convegno]
Andreolini, M.; Pietri, M.; Tosi, S.; Lancellotti, R.
abstract
Current monitoring solutions are not well suited to monitoring large data centers in different ways: lack of scalability, scarce representativity of global state conditions, inability in guaranteeing persistence in service delivery, and the impossibility of monitoring multitenant applications. In this paper, we present a novel monitoring architecture that strives to address these problems. It integrates a hierarchical scheme to monitor the resources in a cluster with a distributed hash table (DHT) to broadcast system state information among different monitors. This architecture strives to obtain high scalability, effectiveness and resilience, as well as the possibility of monitoring services spanning across different clusters or even different data centers of the cloud provider. We evaluate the scalability of the proposed architecture through an experimental analysis and we measure the overhead of the DHT-based communication scheme.
2015
- Adaptive, scalable and reliable monitoring of big data on clouds
[Articolo su rivista]
Andreolini, Mauro; Colajanni, Michele; Pietri, Marcello; Tosi, Stefania
abstract
Real-time monitoring of cloud resources is crucial for a variety of tasks such as performance analysis, workload management, capacity planning and fault detection. Applications producing big data make the monitoring task very difficult at high sampling frequencies because of high computational and communication overheads in collecting, storing, and managing information. We present an adaptive algorithm for monitoring big data applications that adapts the intervals of sampling and frequency of updates to data characteristics and administrator needs. Adaptivity allows us to limit computational and communication costs and to guarantee high reliability in capturing relevant load changes. Experimental evaluations performed on a large testbed show the ability of the proposed adaptive algorithm to reduce resource utilization and communication overhead of big data monitoring without penalizing the quality of data, and demonstrate our improvements to the state of the art.
2014
- Monitoring large cloud-based systems
[Relazione in Atti di Convegno]
Andreolini, Mauro; Pietri, Marcello; Tosi, Stefania; Balboni, Andrea
abstract
Large scale cloud-based services are built upon a multitude of hardware and software resources, disseminated
in one or multiple data centers. Controlling and managing these resources requires the integration of several
pieces of software that may yield a representative view of the data center status. Today’s both closed and
open-source monitoring solutions fail in different ways, including the lack of scalability, scarce representativity
of global state conditions, inability in guaranteeing persistence in service delivery, and the impossibility of
monitoring multi-tenant applications. In this paper, we present a novel monitoring architecture that addresses
the aforementioned issues. It integrates a hierarchical scheme to monitor the resources in a cluster with a
distributed hash table (DHT) to broadcast system state information among different monitors. This architecture
strives to obtain high scalability, effectiveness and resilience, as well as the possibility of monitoring
services spanning across different clusters or even different data centers of the cloud provider. We evaluate the
scalability of the proposed architecture through a bottleneck analysis achieved by experimental results.
2014
- Resilient and adaptive networked systems
[Capitolo/Saggio]
Andreolini, M.; Casolari, S.; Pietri, M.; Tosi, S.
abstract
Nowadays, networks form the backbone of most of the computing systems, and modern system infrastructures must accommodate continuously changing demands for different types of workloads and time constraints. In a similar context, adaptive management of virtualized application environments among networked systems is becoming one of the most important strategies to guarantee resilience and performance of available computing resources. In this chapter, Mauro Andreolini et al. analyze the management algorithms that decide, in an adaptive manner, the transparent reallocation of live sessions of virtual machines in large numbers of networked hosts. They discuss the main challenges and solutions related to the adaptive activation of the migration process.
2013
- Real-time adaptive algorithm for resource monitoring
[Relazione in Atti di Convegno]
Andreolini, Mauro; Colajanni, Michele; Pietri, Marcello; Stefania, Tosi
abstract
In large scale systems, real-time monitoring of hardware and software resources is a crucial means for any management purpose. In architectures consisting of thousands of servers and hundreds of thousands of component resources, the amount of data monitored at high sampling frequencies represents an overhead on system performance and communication, while reducing sampling may cause quality degradation. We present a real-time adaptive algorithm for scalable data monitoring that is able to adapt the frequency of sampling and data updating for a twofold goal: to minimize computational and communication costs, to guarantee that reduced samples do not affect the accuracy of information about resources. Experiments carried out on heterogeneous data traces referring to synthetic and real environments confirm that the proposed adaptive approach reduces utilization and communication overhead without penalizing the quality of data with respect to existing monitoring algorithms.
2012
- A scalable architecture for real-time monitoring of large information systems
[Relazione in Atti di Convegno]
Andreolini, Mauro; Colajanni, Michele; Pietri, Marcello
abstract
Data centers supporting cloud-based services are characterized by a huge number of hardware and software resources often cooperating in complex and unpredictable ways. Understanding the state of these systems for reasons of management and service level agreement requires scalable monitoring architectures that should gather and evaluate continuosly large flows in almost real-time periods. We propose a novel monitoring architecture that, by combining a hierarchical approach with decentralized monitors, addresses these challenges. In this context, fully centralized systems do not scale to the required number of flows, while pure peer-to-peer architectures cannot provide a global view of the system state. We evaluate the monitoring architecture for computational units of gathering and evaluation in real contexts that demonstrate the scalability potential of the proposed system.