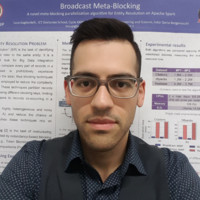 |
LUCA GAGLIARDELLI
Ricercatore t.d. art. 24 c. 3 lett. A Dipartimento di Ingegneria "Enzo Ferrari" Didattica integrativa Dipartimento di Economia "Marco Biagi"
|
Home |
Curriculum(pdf) |
Didattica |
Pubblicazioni
2024
- A Novel Methodology for Topic Identification in Hadith
[Abstract in Atti di Convegno]
Aftar, Sania; Gagliardelli, Luca; EL GANADI, Amina; Ruozzi, Federico; Bergamaschi, Sonia
abstract
2024
- GSM: A Generalized Approach to Supervised Meta-blocking for Scalable Entity Resolution
[Articolo su rivista]
Gagliardelli, Luca; Papadakis, George; Simonini, Giovanni; Bergamaschi, Sonia; Palpanas, Themis
abstract
2023
- A big data platform exploiting auditable tokenization to promote good practices inside local energy communities
[Articolo su rivista]
Gagliardelli, Luca; Zecchini, Luca; Ferretti, Luca; Beneventano, Domenico; Simonini, Giovanni; Bergamaschi, Sonia; Orsini, Mirko; Magnotta, Luca; Mescoli, Emma; Livaldi, Andrea; Gessa, Nicola; De Sabbata, Piero; D’Agosta, Gianluca; Paolucci, Fabrizio; Moretti, Fabio
abstract
The Energy Community Platform (ECP) is a modular system conceived to promote a conscious use of energy by the users inside local energy communities. It is composed of two integrated subsystems: the Energy Community Data Platform (ECDP), a middleware platform designed to support the collection and the analysis of big data about the energy consumption inside local energy communities, and the Energy Community Tokenization Platform (ECTP), which focuses on tokenizing processed source data to enable incentives through smart contracts hosted on a decentralized infrastructure possibly governed by multiple authorities. We illustrate the overall design of our system, conceived considering some real-world projects (dealing with different types of local energy community, different amounts and nature of incoming data, and different types of users), analyzing in detail the key aspects of the two subsystems. In particular, the ECDP acquires data of a different nature in a heterogeneous format from multiple sources and supports a data integration workflow and a data lake workflow, designed for different uses of the data. We motivate our technological choices and present the alternatives taken into account, both in terms of software and of architectural design. On the other hand, the ECTP operates a tokenization process via smart contracts to promote good behaviors of users within the local energy community. The peculiarity of this platform is to allow external parties to audit the correct behavior of the whole tokenization process while protecting the confidentiality of the data and the performance of the platform. The main strengths of the presented system are flexibility and scalability (guaranteed by its modular architecture), which allow its applicability to any type of local energy community.
2023
- A Big Data Platform for the Management of Local Energy Communities Data
[Relazione in Atti di Convegno]
Bergamaschi, Sonia; Gagliardelli, Luca
abstract
2023
- HKS: Efficient Data Partitioning for Stateful Streaming
[Relazione in Atti di Convegno]
Aslam, Adeel; Simonini, Giovanni; Gagliardelli, Luca; Mozzillo, Angelo; Bergamaschi, Sonia
abstract
2023
- Progetto di Basi di Dati Relazionali
[Monografia/Trattato scientifico]
Beneventano, Domenico; Bergamaschi, Sonia; Gagliardelli, Luca; Guerra, Francesco; Vincini, Maurizio
abstract
L’obiettivo del volume è fornire al lettore le nozioni fondamentali di progettazione e di realizzazione di applicazioni di basi di dati relazionali.
Relativamente alla progettazione, vengono trattate le fasi di progettazione concettuale e logica e vengono presentati i modelli dei dati Entity-Relationship e Relazionale che costituiscono gli strumenti di base, rispettivamente, per la progettazione concettuale e la progettazione logica.
Viene inoltre introdotto lo studente alla teoria della normalizzazione di basi di dati relazionali.
Relativamente alla realizzazione, vengono presentati elementi ed esempi del linguaggio standard per RDBMS (Relational Database Management Systems) SQL. Ampio spazio è dedicato ad esercizi svolti sui temi trattati.
2022
- Big Data Integration & Data-Centric AI for eHealth
[Relazione in Atti di Convegno]
Beneventano, Domenico; Bergamaschi, Sonia; Gagliardelli, Luca; Simonini, Giovanni; Zecchini, Luca
abstract
La big data integration, ovvero l’integrazione di grandi quantità di dati provenienti da molteplici sorgenti, rappresenta una delle principali sfide per l’impiego di tecniche e strumenti basati sull’intelligenza artificiale in ambito medico (eHealth). In questo contesto risulta inoltre di primaria importanza garantire la qualità dei dati su cui operano tali strumenti e tecniche (Data-Centric AI), che rivestono un ruolo ormai centrale nel settore. Le attività di ricerca del Database Group (DBGroup) del Dipartimento di Ingegneria "Enzo Ferrari" dell’Università degli Studi di Modena e Reggio Emilia si muovono in questa direzione. Presentiamo quindi i principali progetti di ricerca del DBGroup nel campo dell’eHealth, che si inseriscono nell’ambito di collaborazioni in diversi settori applicativi.
2022
- Big Data Integration for Data-Centric AI
[Abstract in Atti di Convegno]
Bergamaschi, Sonia; Beneventano, Domenico; Simonini, Giovanni; Gagliardelli, Luca; Aslam, Adeel; De Sabbata, Giulio; Zecchini, Luca
abstract
Big data integration represents one of the main challenges for the use of techniques and tools based on Artificial Intelligence (AI) in several crucial areas: eHealth, energy management, enterprise data, etc. In this context, Data-Centric AI plays a primary role in guaranteeing the quality of the data on which these tools and techniques operate. Thus, the activities of the Database Research Group (DBGroup) of the “Enzo Ferrari” Engineering Department of the University of Modena and Reggio Emilia are moving in this direction. Therefore, we present the main research projects of the DBGroup, which are part of collaborations in various application sectors.
2022
- ECDP: A Big Data Platform for the Smart Monitoring of Local Energy Communities
[Relazione in Atti di Convegno]
Gagliardelli, Luca; Zecchini, Luca; Beneventano, Domenico; Simonini, Giovanni; Bergamaschi, Sonia; Orsini, Mirko; Magnotta, Luca; Mescoli, Emma; Livaldi, Andrea; Gessa, Nicola; De Sabbata, Piero; D’Agosta, Gianluca; Paolucci, Fabrizio; Moretti3, Fabio
abstract
2022
- Generalized Supervised Meta-blocking
[Articolo su rivista]
Gagliardelli, Luca; Papadakis, George; Simonini, Giovanni; Bergamaschi, Sonia; Palpanas, Themis
abstract
Entity Resolution is a core data integration task that relies on Blocking to scale to large datasets.
Schema-agnostic blocking achieves very high recall, requires no domain knowledge and applies to data of any structuredness and schema heterogeneity. This comes at the cost of many irrelevant candidate pairs (i.e., comparisons), which can be significantly reduced by Meta-blocking techniques that leverage the entity co-occurrence patterns inside blocks: first, pairs of candidate entities are weighted in proportion to their matching likelihood, and then, pruning discards the pairs with the lowest scores.
Supervised Meta-blocking goes beyond this approach by combining multiple scores per comparison into a feature vector that is fed to a binary classifier.
By using probabilistic classifiers, Generalized Supervised Meta-blocking associates every pair of candidates with a score that can be used by any pruning algorithm. For higher effectiveness, new weighting schemes are examined as features. Through extensive experiments, we identify the best pruning algorithms, their optimal sets of features, as well as the minimum possible size of the training set.
2022
- Progressive Entity Resolution with Node Embeddings
[Relazione in Atti di Convegno]
Simonini, Giovanni; Gagliardelli, Luca; Rinaldi, Michele; Zecchini, Luca; De Sabbata, Giulio; Aslam, Adeel; Beneventano, Domenico; Bergamaschi, Sonia
abstract
Entity Resolution (ER) is the task of finding records that refer to the same real-world entity, which are called matches. ER is a fundamental pre-processing step when dealing with dirty and/or heterogeneous datasets; however, it can be very time-consuming when employing complex machine learning models to detect matches, as state-of-the-art ER methods do. Thus, when time is a critical component and having a partial ER result is better than having no result at all, progressive ER methods are employed to try to maximize the number of detected matches as a function of time.
In this paper, we study how to perform progressive ER by exploiting graph embeddings. The basic idea is to represent candidate matches in a graph: each node is a record and each edge is a possible comparison to check—we build that on top of a well-known, established graph-based ER framework. We experimentally show that our method performs better than existing state-of-the-art progressive ER methods on real-world benchmark datasets.
2021
- LigAdvisor: A versatile and user-friendly web-platform for drug design
[Articolo su rivista]
Pinzi, L.; Tinivella, A.; Gagliardelli, L.; Beneventano, D.; Rastelli, G.
abstract
Although several tools facilitating in silico drug design are available, their results are usually difficult to integrate with publicly available information or require further processing to be fully exploited. The rational design of multi-target ligands (polypharmacology) and the repositioning of known drugs towards unmet therapeutic needs (drug repurposing) have raised increasing attention in drug discovery, although they usually require careful planning of tailored drug design strategies. Computational tools and data-driven approaches can help to reveal novel valuable opportunities in these contexts, as they enable to efficiently mine publicly available chemical, biological, clinical, and disease-related data. Based on these premises, we developed LigAdvisor, a data-driven webserver which integrates information reported in DrugBank, Protein Data Bank, UniProt, Clinical Trials and Therapeutic Target Database into an intuitive platform, to facilitate drug discovery tasks as drug repurposing, polypharmacology, target fishing and profiling. As designed, LigAdvisor enables easy integration of similarity estimation results with clinical data, thereby allowing a more efficient exploitation of information in different drug discovery contexts. Users can also develop customizable drug design tasks on their own molecules, by means of ligand- and target-based search modes, and download their results. LigAdvisor is publicly available at https://ligadvisor.unimore.it/.
2021
- Reproducible experiments on Three-Dimensional Entity Resolution with JedAI
[Articolo su rivista]
Mandilaras, George; Papadakis, George; Gagliardelli, Luca; Simonini, Giovanni; Thanos, Emmanouil; Giannakopoulos, George; Bergamaschi, Sonia; Palpanas, Themis; Koubarakis, Manolis; Lara-Clares, Alicia; Farina, Antonio
abstract
In Papadakis et al. [1], we presented the latest release of JedAI, an open-source Entity Resolution (ER) system that allows for building a large variety of end-to-end ER pipelines. Through a thorough experimental evaluation, we compared a schema-agnostic ER pipeline based on blocks with another schema-based ER pipeline based on similarity joins. We applied them to 10 established, real-world datasets and assessed them with respect to effectiveness and time efficiency. Special care was taken to juxtapose their scalability, too, using seven established, synthetic datasets. Moreover, we experimentally compared the effectiveness of the batch schema-agnostic ER pipeline with its progressive counterpart. In this companion paper, we describe how to reproduce the entire experimental study that pertains to JedAI’s serial execution through its intuitive user interface. We also explain how to examine the robustness of the parameter configurations we have selected.
2021
- The Case for Multi-task Active Learning Entity Resolution
[Relazione in Atti di Convegno]
Simonini, Giovanni; Saccani, Henrique; Gagliardelli, Luca; Zecchini, Luca; Beneventano, Domenico; Bergamaschi, Sonia
abstract
2021
- The Italian National Registry for FSHD: an enhanced data integration and an analytics framework towards Smart Health Care and Precision Medicine for a rare disease
[Articolo su rivista]
Bettio, C.; Salsi, V.; Orsini, M.; Calanchi, E.; Magnotta, L.; Gagliardelli, L.; Kinoshita, J.; Bergamaschi, S.; Tupler, R.
abstract
Background: The Italian Clinical network for FSHD (ICNF) has established the Italian National Registry for FSHD (INRF), collecting data from patients affected by Facioscapulohumeral dystrophy (FSHD) and their relatives. The INRF has gathered data from molecular analysis, clinical evaluation, anamnestic information, and family history from more than 3500 participants. Methods: A data management framework, called Mediator Environment for Multiple Information Sources (MOMIS) FSHD Web Platform, has been developed to provide charts, maps and search tools customized for specific needs. Patients’ samples and their clinical information derives from the Italian Clinical network for FSHD (ICNF), a consortium consisting of fourteen neuromuscular clinics distributed across Italy. The tools used to collect, integrate, and visualize clinical, molecular and natural history information about patients affected by FSHD and their relatives are described. Results: The INRF collected the molecular data regarding FSHD diagnosis conducted on 7197 subjects and identified 3362 individuals carrying a D4Z4 Reduced Allele (DRA): 1634 were unrelated index cases. In 1032 cases the molecular testing has been extended to 3747 relatives, 1728 carrying a DRA. Since 2009 molecular analysis has been accompanied by clinical evaluation based standardized evaluation protocols. In the period 2009–2020, 3577 clinical forms have been collected, 2059 follow the Comprehensive Clinical Evaluation form (CCEF). The integration of standardized clinical information and molecular data has made possible to demonstrate the wide phenotypic variability of FSHD. The MOMIS (Mediator Environment for Multiple Information Sources) data integration framework allowed performing genotype–phenotype correlation studies, and generated information of medical importance either for clinical practice or genetic counseling. Conclusion: The platform implemented for the FSHD Registry data collection based on OpenClinica meets the requirement to integrate patient/disease information, as well as the need to adapt dynamically to security and privacy concerns. Our results indicate that the quality of data collection in a multi-integrated approach is fundamental for clinical and epidemiological research in a rare disease and may have great value in allowing us to redefine diagnostic criteria and disease markers for FSHD. By extending the use of the MOMIS data integration framework to other countries and the longitudinal systematic collection of standardized clinical data will facilitate the understanding of disease natural history and offer valuable inputs towards trial readiness. This approach is of high significance to FSHD medical community and also to rare disease research in general.
2020
- BLAST2: An Efficient Technique for Loose Schema Information Extraction from Heterogeneous Big Data Sources
[Articolo su rivista]
BENEVENTANO, Domenico; BERGAMASCHI, Sonia; GAGLIARDELLI, LUCA; SIMONINI, GIOVANNI
abstract
We present BLAST2 a novel technique to efficiently extract loose schema information, i.e., metadata that can serve as a surrogate of the schema alignment task within the Entity Resolution (ER) process — to identify records that refer to the same real-world entity — when integrating multiple, heterogeneous and voluminous data sources. The loose schema information is exploited for reducing the overall complexity of ER, whose naïve solution would imply O(n^2) comparisons, where is the number of entity representations involved in the process and can be extracted by both structured and unstructured data sources. BLAST2 is completely unsupervised yet able to achieve almost the same precision and recall of supervised state-of-the-art schema alignment techniques when employed for Entity Resolution tasks, as shown in our experimental evaluation performed on two real-world data sets (composed of 7 and 10 data sources, respectively).
2020
- RulER: Scaling Up Record-level Matching Rules
[Relazione in Atti di Convegno]
Gagliardelli, Luca; Simonini, Giovanni; Bergamaschi, Sonia
abstract
2020
- Scaling up Record-level Matching Rules
[Relazione in Atti di Convegno]
Gagliardelli, L.; Simonini, G.; Bergamaschi, S.
abstract
Record-level matching rules are chains of similarity join pred-icates on multiple attributes employed to join records that refer to the same real-world object when an explicit foreign key is not available on the data sets at hand. They are widely employed by data scientists and practitioners that work with data lakes, open data, and data in the wild. In this work we present a novel technique that allows to efficiently exe-cute record-level matching rules on parallel and distributed systems and demonstrate its efficiency on a real-wold data set.
2020
- Three-dimensional Entity Resolution with JedAI
[Articolo su rivista]
Papadakis, G.; Mandilaras, G.; Gagliardelli, L.; Simonini, G.; Thanos, E.; Giannakopoulos, G.; Bergamaschi, S.; Palpanas, T.; Koubarakis, M.
abstract
Entity Resolution (ER) is the task of detecting different entity profiles that describe the same real-world objects. To facilitate its execution, we have developed JedAI, an open-source system that puts together a series of state-of-the-art ER techniques that have been proposed and examined independently, targeting parts of the ER end-to-end pipeline. This is a unique approach, as no other ER tool brings together so many established techniques. Instead, most ER tools merely convey a few techniques, those primarily developed by their creators. In addition to democratizing ER techniques, JedAI goes beyond the other ER tools by offering a series of unique characteristics: (i) It allows for building and benchmarking millions of ER pipelines. (ii) It is the only ER system that applies seamlessly to any combination of structured and/or semi-structured data. (iii) It constitutes the only ER system that runs seamlessly both on stand-alone computers and clusters of computers — through the parallel implementation of all algorithms in Apache Spark. (iv) It supports two different end-to-end workflows for carrying out batch ER (i.e., budget-agnostic), a schema-agnostic one based on blocks, and a schema-based one relying on similarity joins. (v) It adapts both end-to-end workflows to budget-aware (i.e., progressive) ER. We present in detail all features of JedAI, stressing the core characteristics that enhance its usability, and boost its versatility and effectiveness. We also compare it to the state-of-the-art in the field, qualitatively and quantitatively, demonstrating its state-of-the-art performance over a variety of large-scale datasets from different domains. The central repository of the JedAI's code base is here: https://github.com/scify/JedAIToolkit. A video demonstrating the JedAI's Web application is available here: https://www.youtube.com/watch?v=OJY1DUrUAe8.
2019
- Entity Resolution and Data Fusion: An Integrated Approach
[Relazione in Atti di Convegno]
Beneventano, Domenico; Bergamaschi, Sonia; Gagliardelli, Luca; Simonini, Giovanni
abstract
2019
- Scaling entity resolution: A loosely schema-aware approach
[Articolo su rivista]
Simonini, Giovanni; Gagliardelli, Luca; Bergamaschi, Sonia; Jagadish, H. V.
abstract
In big data sources, real-world entities are typically represented with a variety of schemata and formats (e.g., relational records, JSON objects, etc.). Different profiles (i.e., representations) of an entity often contain redundant and/or inconsistent information. Thus identifying which profiles refer to the same entity is a fundamental task (called Entity Resolution) to unleash the value of big data. The naïve all-pairs comparison solution is impractical on large data, hence blocking methods are employed to partition a profile collection into (possibly overlapping) blocks and limit the comparisons to profiles that appear in the same block together. Meta-blocking is the task of restructuring a block collection, removing superfluous comparisons. Existing meta-blocking approaches rely exclusively on schema-agnostic features, under the assumption that handling the schema variety of big data does not pay-off for such a task. In this paper, we demonstrate how “loose” schema information (i.e., statistics collected directly from the data) can be exploited to enhance the quality of the blocks in a holistic loosely schema-aware (meta-)blocking approach that can be used to speed up your favorite Entity Resolution algorithm. We call it Blast (Blocking with Loosely-Aware Schema Techniques). We show how Blast can automatically extract the loose schema information by adopting an LSH-based step for efficiently handling volume and schema heterogeneity of the data. Furthermore, we introduce a novel meta-blocking algorithm that can be employed to efficiently execute Blast on MapReduce-like systems (such as Apache Spark). Finally, we experimentally demonstrate, on real-world datasets, how Blast outperforms the state-of-the-art (meta-)blocking approaches.
2019
- SparkER: Scaling Entity Resolution in Spark
[Relazione in Atti di Convegno]
Gagliardelli, Luca; Simonini, Giovanni; Beneventano, Domenico; Bergamaschi, Sonia
abstract
We present SparkER, an ER tool that can scale practitioners’ favorite ER algorithms. SparkER has been devised to take full ad- vantage of parallel and distributed computation as well (running on top of Apache Spark). The first SparkER version was focused on the blocking step and implements both schema-agnostic and Blast meta-blocking approaches (i.e. the state-of-the-art ones); a GUI for SparkER, to let non-expert users to use it in an unsupervised mode, was developed. The new version of SparkER to be shown in this demo, extends significantly the tool. Entity matching and Entity Clustering modules have been added. Moreover, in addition to the completely unsupervised mode of the first version, a supervised mode has been added. The user can be assisted in supervising the entire process and in injecting his knowledge in order to achieve the best result. During the demonstration, attendees will be shown how SparkER can significantly help in devising and debugging ER algorithms.
2018
- BigDedup: a Big Data Integration toolkit for Duplicate Detection in Industrial Scenarios
[Relazione in Atti di Convegno]
Gagliardelli, Luca; Zhu, Song; Simonini, Giovanni; Bergamaschi, Sonia
abstract
Duplicate detection aims to identify different records in data sources that refers to the same real-world entity. It is a fundamental task for: item catalogs fusion, customer databases integration, fraud detection, and more. In this work we present BigDedup, a toolkit able to detect duplicate records on Big Data sources in an efficient manner. BigDedup makes available the state-of-the-art duplicate detection techniques on Apache Spark, a modern framework for distributed computing in Big Data scenarios. It can be used in two different ways: (i) through a simple graphic interface that permit the user to process structured and unstructured data in a fast and effective way; (ii) as a library that provides different components that can be easily extended and customized. In the paper we show how to use BigDedup and its usefulness through some industrial examples.
2018
- Enhancing Loosely Schema-aware Entity Resolution with User Interaction
[Relazione in Atti di Convegno]
Simonini, Giovanni; Gagliardelli, Luca; Zhu, Song; Bergamaschi, Sonia
abstract
Entity Resolution (ER) is a fundamental task of data integration: it identifies different representations (i.e., profiles) of the same real-world entity in databases. To compare all possible profile pairs through an ER algorithm has a quadratic complexity. Blocking is commonly employed to avoid that: profiles are grouped into blocks according to some features, and ER is performed only for entities of the same block. Yet, devising blocking criteria and ER algorithms for data with highly schema heterogeneity is a difficult and error-prone task calling for automatic methods and debugging tools.
In our previous work, we presented Blast, an ER system that can scale practitioners’ favorite Entity Resolution algorithms. In current version, Blast has been devised to take full advantage of parallel and distributed computation as well (running on top of Apache Spark). It implements the state-of-the-art unsuper- vised blocking method based on automatically extracted loose schema information. It comes with a GUI, which allows: (i) to visualize, understand, and (optionally) manually modify the loose schema information automatically extracted (i.e., injecting user’s knowledge in the system); (ii) to retrieve resolved entities through a free-text search box, and to visualize the process that lead to that result (i.e., the provenance). Experimental results on real-world datasets show that these two functionalities can significantly enhance Entity Resolution results.
2018
- How improve Set Similarity Join based on prefix approach in distributed environment
[Relazione in Atti di Convegno]
Zhu, Song; Gagliardelli, Luca; Simonini, Giovanni; Beneventano, Domenico
abstract
Set similarity join is an essential operation in data integration and big data analytics, that finds similar pairs of records where the records contain string or set-based data. To cope with the increasing scale of the data, several techniques have been proposed to perform set similarity joins using distributed frameworks, such as the MapReduce framework. In particular, Vernica et al. [3] proposed a MapReduce implementation of the so-called PPJoin algorithm [2], which in a recent study, was experimentally demonstrated as one of the best set similarity join algorithm [4]. These techniques, however, usually produce huge amounts of duplicates in order to perform parallel processing successfully. The large number of duplicates incurs on both large shuffle cost and unnecessary computation cost, which significantly decrease the performance. Moreover, these approaches do not provide a load balancing guarantee, which results in a skewness problem and negatively affects the scalability properties of these techniques.
To address these problems, in this paper, we propose a duplicate-free framework, called TTJoin, to perform set simi- larity joins efficiently by utilizing an innovative filter based on prefix tokens and we implement it with one of most popular distributed framework, i.e., Apache Spark. Experiments on real world datasets demonstrate the effectiveness of proposed solution with respect to either traditional PPJoin and the MapReduce implementation proposed in [3].
2018
- MOMIS Dashboard: a powerful data analytics tool for Industry 4.0
[Relazione in Atti di Convegno]
Magnotta, Luca; Gagliardelli, Luca; Simonini, Giovanni; Orsini, Mirko; Bergamaschi, Sonia
abstract
In this work we present the MOMIS Dashboard, an interactive data analytics tool to explore and visualize data sources content through several kind of dynamic views (e.g. maps, bar, line, pie, etc.). The software tool is very versatile, and supports the connection to the main relational DBMS and Big Data sources. Moreover, it can be connected to MOMIS, a powerful Open Source Data Integration system, able to integrate heterogeneous data sources as enterprise information systems as well as sensors data. MOMIS Dashboard provides a secure permission management to limit data access on the basis of a user role, and a Designer to create and share personalized insights on the company KPIs, facilitating the enterprise collaboration. We illustrate the MOMIS Dashboard efficacy in a real enterprise scenario: a production monitoring platform to analyze real-time and historical data collected through sensors located on production machines that optimize production, energy consumption, and enable preventive maintenance.
2017
- BigBench workload executed by using Apache Flink
[Relazione in Atti di Convegno]
Bergamaschi, Sonia; Gagliardelli, Luca; Simonini, Giovanni; Zhu, Song
abstract
Many of the challenges that have to be faced in Industry 4.0 involve the management and analysis of huge amount of data (e.g. sensor data management and machine-fault prediction in industrial manufacturing, web-logs analysis in e-commerce). To handle the so-called Big Data management and analysis, a plethora of frameworks has been proposed in the last decade. Many of them are focusing on the parallel processing paradigm, such as MapReduce, Apache Hive, Apache Flink. However, in this jungle of frameworks, the performance evaluation of these technologies is not a trivial task, and strictly depends on the application requirements. The scope of this paper is to compare two of the most employed and promising frameworks to manage big data: Apache Flink and Apache Hive, which are general purpose distributed platforms under the umbrella of the Apache Software Foundation. To evaluate these two frameworks we use the benchmark BigBench, developed for Apache Hive. We re-implemented the most significant queries of Apache Hive BigBench to make them work on Apache Flink, in order to be able to compare the results of the same queries executed on both frameworks. Our results show that Apache Flink, if it is configured well, is able to outperform Apache Hive.
2017
- From Data Integration to Big Data Integration
[Capitolo/Saggio]
Bergamaschi, Sonia; Beneventano, Domenico; Mandreoli, Federica; Martoglia, Riccardo; Guerra, Francesco; Orsini, Mirko; Po, Laura; Vincini, Maurizio; Simonini, Giovanni; Zhu, Song; Gagliardelli, Luca; Magnotta, Luca
abstract
Abstract. The Database Group (DBGroup, www.dbgroup.unimore.it) and Information System Group (ISGroup, www.isgroup.unimore.it) re- search activities have been mainly devoted to the Data Integration Research Area. The DBGroup designed and developed the MOMIS data integration system, giving raise to a successful innovative enterprise DataRiver (www.datariver.it), distributing MOMIS as open source. MOMIS provides an integrated access to structured and semistructured data sources and allows a user to pose a single query and to receive a single unified answer. Description Logics, Automatic Annotation of schemata plus clustering techniques constitute the theoretical framework. In the context of data integration, the ISGroup addressed problems related to the management and querying of heterogeneous data sources in large-scale and dynamic scenarios. The reference architectures are the Peer Data Management Systems and its evolutions toward dataspaces. In these contexts, the ISGroup proposed and evaluated effective and efficient mechanisms for network creation with limited information loss and solutions for mapping management query reformulation and processing and query routing. The main issues of data integration have been faced: automatic annotation, mapping discovery, global query processing, provenance, multi- dimensional Information integration, keyword search, within European and national projects. With the incoming new requirements of integrating open linked data, textual and multimedia data in a big data scenario, the research has been devoted to the Big Data Integration Research Area. In particular, the most relevant achieved research results are: a scalable entity resolution method, a scalable join operator and a tool, LODEX, for automatically extracting metadata from Linked Open Data (LOD) resources and for visual querying formulation on LOD resources. Moreover, in collaboration with DATARIVER, Data Integration was successfully applied to smart e-health.
2017
- SparkER: an Entity Resolution framework for Apache Spark
[Software]
Gagliardelli, Luca; Simonini, Giovanni; Zhu, Song; Bergamaschi, Sonia
abstract
Entity Resolution is a crucial task for many applications, but its nave solution has a low efficiency due to its quadratic complexity. Usually, to reduce this complexity, blocking is employed to cluster similar entities in order to reduce the global number of comparisons. Meta-Blocking (MB) approach aims to restructure the block collection in order to reduce the number of comparisons, obtaining better results in term of execution time. However, these techniques alone are not sufficient to work in the context of Big Data, where typically the records to be compared are in the order of hundreds of million. Parallel implementations of MB have been proposed in the literature, but all of them are built on Hadoop MapReduce, which is known to have a low efficiency on modern cluster architecture. We implement a Meta-Blocking technique for Apache Spark. Unlike Hadoop, Apache Spark uses a different paradigm to manage the tasks: it does not need to save the partial results on disk, keeping them in memory, which guarantees a shorter execution time. We reimplemented the state-of-the-art MB techniques, creating a new algorithm in order to exploit the Spark architecture. We tested our algorithm over several established datasets, showing that ours Spark implementation outperforms other existing ones based on Hadoop.
2017
- The Italian FSHD registry: An enhanced data integration and analytics framework for smart health care
[Relazione in Atti di Convegno]
Orsini, Mirko; Calanchi, Enrico; Magnotta, Luca; Gagliardelli, Luca; Govi, Monica; Mele, Fabiano; Tupler, Rossella
abstract
Facioscapulohumeral dystrophy (FSHD) is a rare genetic disease that has been described more than a hundred years ago. The Miogen Lab has been able to collect a large amount of data on patients affected by FSHD and their relatives over the years, also extending the research to their ancestors. Collected data include molecular analysis, clinical information on health status, family pedigree and geographic origin. The challenge of FSHD Registry is to investigate these large amount of information, discover additional elements related to disease onset and better understand the clinical progression and genetic inheritance of the disease, exploiting data integration capabilities and Big Data techniques. In this paper we describe the tools we used to collect, integrate and display these data in a framework that allows users to search among clinical records to elaborate brief reports and discover new relations on collected data. The solution provides charts, maps and search tools customized on the specific needs that came to light during the collaboration between DataRiver and Miogen Lab, joining the clinical knowledge of the latter with the information technology expertise of the former. The framework offers a single entry point for all genomic and therapeutic studies.
2016
- Driving Innovation in Youth Policies With Open Data
[Relazione in Atti di Convegno]
Beneventano, Domenico; Bergamaschi, Sonia; Gagliardelli, Luca; Po, Laura
abstract
In December 2007, thirty activists held a meeting in California to define the concept of open public data. For the first time eight Open Government Data (OPG) principles were settled; OPG should be Complete, Primary (reporting data at an high level of granularity), Timely, Accessible, Machine processable, Non-discriminatory, Non-proprietary, License-free. Since the inception of the Open Data philosophy there has been a constant increase in information released improving the communication channel between public administrations and their citizens.
Open data offers government, companies and citizens information to make better decisions. We claim Public Administrations, that are the main producers and one of the consumers of Open Data, might effectively extract important information by integrating its own data with open data sources.
This paper reports the activities carried on during a research project on Open Data for Youth Policies. The project was devoted to explore the youth situation in the municipalities and provinces of the Emilia Romagna region (Italy), in particular, to examine data on population, education and work. We identified interesting data sources both from the open data community and from the private repositories of local governments related to the Youth Policies. The selected sources have been integrated and, the result of the integration by means of a useful navigator tool have been shown up. In the end, we published new information on the web as Linked Open Data. Since the process applied and the tools used are generic, we trust this paper to be an example and a guide for new projects that aims to create new knowledge through Open Data.
2015
- Open Data for Improving Youth Policies
[Relazione in Atti di Convegno]
Beneventano, Domenico; Bergamaschi, Sonia; Gagliardelli, Luca; Po, Laura
abstract
The Open Data \textit{philosophy} is based on the idea that certain data should be made available to all citizens, in an open form, without any copyright restrictions, patents or other mechanisms of control.
Various government have started to publish open data, first of all USA and UK in 2009, and in 2015, the Open Data Barometer project (www.opendatabarometer.org) states that on 77 diverse states across the world, over 55 percent have developed some form of Open Government Data initiative.
We claim Public Administrations, that are the main producers and one of the consumers of Open Data, might effectively extract important information by integrating its own data with open data sources.This paper reports the activities carried on during a one-year research project on Open Data for Youth Policies.
The project was mainly devoted to explore the youth situation in the municipalities and provinces of the Emilia Romagna region (Italy), in particular, to examine data on population, education and work.The project goals were: to identify interesting data sources both from the open data community and from the private repositories of local governments of Emilia Romagna region related to the Youth Policies; to integrate them and, to show up the result of the integration by means of a useful navigator tool; in the end, to publish new information on the web as Linked Open Data.
This paper also reports the main issues encountered that may seriously affect the entire process of consumption, integration till the publication of open data.