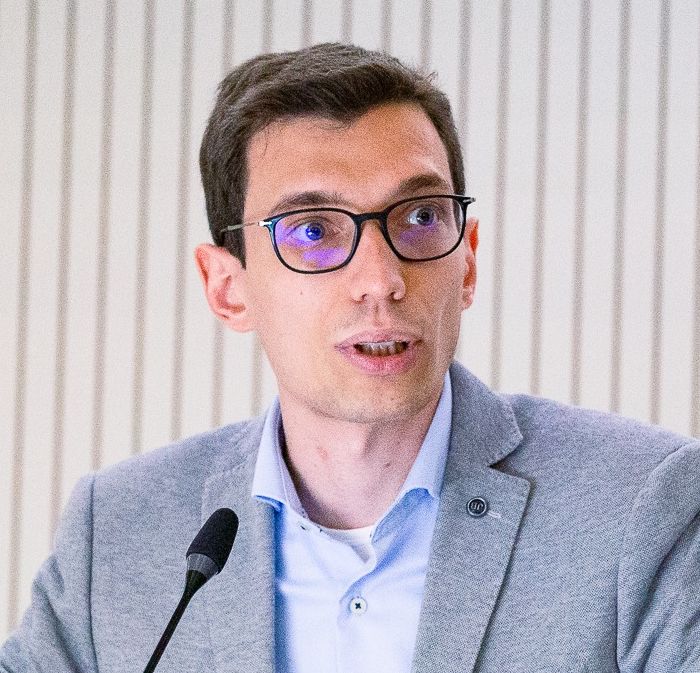 |
LORENZO BARALDI
Ricercatore t.d. art. 24 c. 3 lett. B Dipartimento di Ingegneria "Enzo Ferrari"
|
Home |
Curriculum(pdf) |
Didattica |
Pubblicazioni
2024
- AIGeN: An Adversarial Approach for Instruction Generation in VLN
[Relazione in Atti di Convegno]
Rawal, Niyati; Bigazzi, Roberto; Baraldi, Lorenzo; Cucchiara, Rita
abstract
2024
- Adapt to Scarcity: Few-Shot Deepfake Detection via Low-Rank Adaptation
[Relazione in Atti di Convegno]
Cappelletti, Silvia; Baraldi, Lorenzo; Cocchi, Federico; Cornia, Marcella; Baraldi, Lorenzo; Cucchiara, Rita
abstract
2024
- Are Learnable Prompts the Right Way of Prompting? Adapting Vision-and-Language Models with Memory Optimization
[Articolo su rivista]
Moratelli, Nicholas; Barraco, Manuele; Cornia, Marcella; Baraldi, Lorenzo; Cucchiara, Rita
abstract
2024
- BRIDGE: Bridging Gaps in Image Captioning Evaluation with Stronger Visual Cues
[Relazione in Atti di Convegno]
Sarto, Sara; Cornia, Marcella; Baraldi, Lorenzo; Cucchiara, Rita
abstract
2024
- Contrasting Deepfakes Diffusion via Contrastive Learning and Global-Local Similarities
[Relazione in Atti di Convegno]
Baraldi, Lorenzo; Cocchi, Federico; Cornia, Marcella; Baraldi, Lorenzo; Nicolosi, Alessandro; Cucchiara, Rita
abstract
2024
- FOSSIL: Free Open-Vocabulary Semantic Segmentation through Synthetic References Retrieval
[Relazione in Atti di Convegno]
Barsellotti, Luca; Amoroso, Roberto; Baraldi, Lorenzo; Cucchiara, Rita
abstract
2024
- Fluent and Accurate Image Captioning with a Self-Trained Reward Model
[Relazione in Atti di Convegno]
Moratelli, Nicholas; Cornia, Marcella; Baraldi, Lorenzo; Cucchiara, Rita
abstract
2024
- Generating More Pertinent Captions by Leveraging Semantics and Style on Multi-Source Datasets
[Articolo su rivista]
Cornia, Marcella; Baraldi, Lorenzo; Fiameni, Giuseppe; Cucchiara, Rita
abstract
2024
- Mapping High-level Semantic Regions in Indoor Environments without Object Recognition
[Relazione in Atti di Convegno]
Bigazzi, Roberto; Baraldi, Lorenzo; Kousik, Shreyas; Cucchiara, Rita; Pavone, Marco
abstract
2024
- Multi-Class Unlearning for Image Classification via Weight Filtering
[Articolo su rivista]
Poppi, Samuele; Sarto, Sara; Cornia, Marcella; Baraldi, Lorenzo; Cucchiara, Rita
abstract
Machine Unlearning is an emerging paradigm for selectively removing the impact of training datapoints from a network. Unlike existing methods that target a limited subset or a single class, our framework unlearns all classes in a single round. We achieve this by modulating the network's components using memory matrices, enabling the network to demonstrate selective unlearning behavior for any class after training. By discovering weights that are specific to each class, our approach also recovers a representation of the classes which is explainable by design. We test the proposed framework on small- and medium-scale image classification datasets, with both convolution- and Transformer-based backbones, showcasing the potential for explainable solutions through unlearning.
2024
- Parents and Children: Distinguishing Multimodal DeepFakes from Natural Images
[Articolo su rivista]
Amoroso, Roberto; Morelli, Davide; Cornia, Marcella; Baraldi, Lorenzo; Del Bimbo, Alberto; Cucchiara, Rita
abstract
2024
- Safe-CLIP: Removing NSFW Concepts from Vision-and-Language Models
[Relazione in Atti di Convegno]
Poppi, Samuele; Poppi, Tobia; Cocchi, Federico; Cornia, Marcella; Baraldi, Lorenzo; Cucchiara, Rita
abstract
2024
- The Revolution of Multimodal Large Language Models: A Survey
[Relazione in Atti di Convegno]
Caffagni, Davide; Cocchi, Federico; Barsellotti, Luca; Moratelli, Nicholas; Sarto, Sara; Baraldi, Lorenzo; Baraldi, Lorenzo; Cornia, Marcella; Cucchiara, Rita
abstract
2024
- Towards Retrieval-Augmented Architectures for Image Captioning
[Articolo su rivista]
Sarto, Sara; Cornia, Marcella; Baraldi, Lorenzo; Nicolosi, Alessandro; Cucchiara, Rita
abstract
2024
- Training-Free Open-Vocabulary Segmentation with Offline Diffusion-Augmented Prototype Generation
[Relazione in Atti di Convegno]
Barsellotti, Luca; Amoroso, Roberto; Cornia, Marcella; Baraldi, Lorenzo; Cucchiara, Rita
abstract
2024
- What’s Outside the Intersection? Fine-grained Error Analysis for Semantic Segmentation Beyond IoU
[Relazione in Atti di Convegno]
Bernhard, Maximilian; Amoroso, Roberto; Kindermann, Yannic; Baraldi, Lorenzo; Cucchiara, Rita; Tresp, Volker; Schubert, Matthias
abstract
2024
- Wiki-LLaVA: Hierarchical Retrieval-Augmented Generation for Multimodal LLMs
[Relazione in Atti di Convegno]
Caffagni, Davide; Cocchi, Federico; Moratelli, Nicholas; Sarto, Sara; Cornia, Marcella; Baraldi, Lorenzo; Cucchiara, Rita
abstract
2023
- Embodied Agents for Efficient Exploration and Smart Scene Description
[Relazione in Atti di Convegno]
Bigazzi, Roberto; Cornia, Marcella; Cascianelli, Silvia; Baraldi, Lorenzo; Cucchiara, Rita
abstract
2023
- Enhancing Open-Vocabulary Semantic Segmentation with Prototype Retrieval
[Relazione in Atti di Convegno]
Barsellotti, Luca; Amoroso, Roberto; Baraldi, Lorenzo; Cucchiara, Rita
abstract
2023
- Evaluating synthetic pre-Training for handwriting processing tasks
[Articolo su rivista]
Pippi, V.; Cascianelli, S.; Baraldi, L.; Cucchiara, R.
abstract
In this work, we explore massive pre-training on synthetic word images for enhancing the performance on four benchmark downstream handwriting analysis tasks. To this end, we build a large synthetic dataset of word images rendered in several handwriting fonts, which offers a complete supervision sig-nal. We use it to train a simple convolutional neural network (ConvNet) with a fully supervised objective. The vector representations of the images obtained from the pre-trained ConvNet can then be consid-ered as encodings of the handwriting style. We exploit such representations for Writer Retrieval, Writer Identification, Writer Verification, and Writer Classification and demonstrate that our pre-training strat-egy allows extracting rich representations of the writers' style that enable the aforementioned tasks with competitive results with respect to task-specific State-of-the-Art approaches.& COPY; 2023 Elsevier B.V. All rights reserved.
2023
- Fashion-Oriented Image Captioning with External Knowledge Retrieval and Fully Attentive Gates
[Articolo su rivista]
Moratelli, Nicholas; Barraco, Manuele; Morelli, Davide; Cornia, Marcella; Baraldi, Lorenzo; Cucchiara, Rita
abstract
Research related to fashion and e-commerce domains is gaining attention in computer vision and multimedia communities. Following this trend, this article tackles the task of generating fine-grained and accurate natural language descriptions of fashion items, a recently-proposed and under-explored challenge that is still far from being solved. To overcome the limitations of previous approaches, a transformer-based captioning model was designed with the integration of external textual memory that could be accessed through k-nearest neighbor (kNN) searches. From an architectural point of view, the proposed transformer model can read and retrieve items from the external memory through cross-attention operations, and tune the flow of information coming from the external memory thanks to a novel fully attentive gate. Experimental analyses were carried out on the fashion captioning dataset (FACAD) for fashion image captioning, which contains more than 130k fine-grained descriptions, validating the effectiveness of the proposed approach and the proposed architectural strategies in comparison with carefully designed baselines and state-of-the-art approaches. The presented method constantly outperforms all compared approaches, demonstrating its effectiveness for fashion image captioning.
2023
- From Show to Tell: A Survey on Deep Learning-based Image Captioning
[Articolo su rivista]
Stefanini, Matteo; Cornia, Marcella; Baraldi, Lorenzo; Cascianelli, Silvia; Fiameni, Giuseppe; Cucchiara, Rita
abstract
2023
- Fully-Attentive Iterative Networks for Region-based Controllable Image and Video Captioning
[Articolo su rivista]
Cornia, Marcella; Baraldi, Lorenzo; Ayellet, Tal; Cucchiara, Rita
abstract
2023
- Let's ViCE! Mimicking Human Cognitive Behavior in Image Generation Evaluation
[Relazione in Atti di Convegno]
Betti, Federico; Staiano, Jacopo; Baraldi, Lorenzo; Baraldi, Lorenzo; Cucchiara, Rita; Sebe, Nicu
abstract
2023
- Positive-Augmented Constrastive Learning for Image and Video Captioning Evaluation
[Relazione in Atti di Convegno]
Sarto, Sara; Barraco, Manuele; Cornia, Marcella; Baraldi, Lorenzo; Cucchiara, Rita
abstract
The CLIP model has been recently proven to be very effective for a variety of cross-modal tasks, including the evaluation of captions generated from vision-and-language models. In this paper, we propose a new recipe for a contrastive-based evaluation metric for image captioning, namely Positive-Augmented Contrastive learning Score (PAC-S), that in a novel way unifies the learning of a contrastive visual-semantic space with the addition of generated images and text on curated data. Experiments spanning several datasets demonstrate that our new metric achieves the highest correlation with human judgments on both images and videos, outperforming existing reference-based metrics like CIDEr and SPICE and reference-free metrics like CLIP-Score. Finally, we test the system-level correlation of the proposed metric when considering popular image captioning approaches, and assess the impact of employing different cross-modal features. We publicly release our source code and trained models.
2023
- Sharing Cultural Heritage—The Case of the Lodovico Media Library
[Articolo su rivista]
Al Kalak, Matteo; Baraldi, Lorenzo
abstract
2023
- Superpixel Positional Encoding to Improve ViT-based Semantic Segmentation Models
[Relazione in Atti di Convegno]
Amoroso, Roberto; Tomei, Matteo; Baraldi, Lorenzo; Cucchiara, Rita
abstract
2023
- SynthCap: Augmenting Transformers with Synthetic Data for Image Captioning
[Relazione in Atti di Convegno]
Caffagni, Davide; Barraco, Manuele; Cornia, Marcella; Baraldi, Lorenzo; Cucchiara, Rita
abstract
Image captioning is a challenging task that combines Computer Vision and Natural Language Processing to generate descriptive and accurate textual descriptions for input images. Research efforts in this field mainly focus on developing novel architectural components to extend image captioning models and using large-scale image-text datasets crawled from the web to boost final performance. In this work, we explore an alternative to web-crawled data and augment the training dataset with synthetic images generated by a latent diffusion model. In particular, we propose a simple yet effective synthetic data augmentation framework that is capable of significantly improving the quality of captions generated by a standard Transformer-based model, leading to competitive results on the COCO dataset.
2023
- Towards Explainable Navigation and Recounting
[Relazione in Atti di Convegno]
Poppi, Samuele; Rawal, Niyati; Bigazzi, Roberto; Cornia, Marcella; Cascianelli, Silvia; Baraldi, Lorenzo; Cucchiara, Rita
abstract
Explainability and interpretability of deep neural networks have become of crucial importance over the years in Computer Vision, concurrently with the need to understand increasingly complex models. This necessity has fostered research on approaches that facilitate human comprehension of neural methods. In this work, we propose an explainable setting for visual navigation, in which an autonomous agent needs to explore an unseen indoor environment while portraying and explaining interesting scenes with natural language descriptions. We combine recent advances in ongoing research fields, employing an explainability method on images generated through agent-environment interaction. Our approach uses explainable maps to visualize model predictions and highlight the correlation between the observed entities and the generated words, to focus on prominent objects encountered during the environment exploration. The experimental section demonstrates that our approach can identify the regions of the images that the agent concentrates on to describe its point of view, improving explainability.
2023
- Unveiling the Impact of Image Transformations on Deepfake Detection: An Experimental Analysis
[Relazione in Atti di Convegno]
Cocchi, Federico; Baraldi, Lorenzo; Poppi, Samuele; Cornia, Marcella; Baraldi, Lorenzo; Cucchiara, Rita
abstract
With the recent explosion of interest in visual Generative AI, the field of deepfake detection has gained a lot of attention. In fact, deepfake detection might be the only measure to counter the potential proliferation of generated media in support of fake news and its consequences. While many of the available works limit the detection to a pure and direct classification of fake versus real, this does not translate well to a real-world scenario. Indeed, malevolent users can easily apply post-processing techniques to generated content, changing the underlying distribution of fake data. In this work, we provide an in-depth analysis of the robustness of a deepfake detection pipeline, considering different image augmentations, transformations, and other pre-processing steps. These transformations are only applied in the evaluation phase, thus simulating a practical situation in which the detector is not trained on all the possible augmentations that can be used by the attacker. In particular, we analyze the performance of a k-NN and a linear probe detector on the COCOFake dataset, using image features extracted from pre-trained models, like CLIP and DINO. Our results demonstrate that while the CLIP visual backbone outperforms DINO in deepfake detection with no augmentation, its performance varies significantly in presence of any transformation, favoring the robustness of DINO.
2023
- Video Surveillance and Privacy: A Solvable Paradox?
[Articolo su rivista]
Cucchiara, Rita; Baraldi, Lorenzo; Cornia, Marcella; Sarto, Sara
abstract
2023
- With a Little Help from your own Past: Prototypical Memory Networks for Image Captioning
[Relazione in Atti di Convegno]
Barraco, Manuele; Sarto, Sara; Cornia, Marcella; Baraldi, Lorenzo; Cucchiara, Rita
abstract
2022
- A Computational Approach for Progressive Architecture Shrinkage in Action Recognition
[Articolo su rivista]
Tomei, Matteo; Baraldi, Lorenzo; Fiameni, Giuseppe; Bronzin, Simone; Cucchiara, Rita
abstract
2022
- ALADIN: Distilling Fine-grained Alignment Scores for Efficient Image-Text Matching and Retrieval
[Relazione in Atti di Convegno]
Messina, Nicola; Stefanini, Matteo; Cornia, Marcella; Baraldi, Lorenzo; Falchi, Fabrizio; Amato, Giuseppe; Cucchiara, Rita
abstract
2022
- Boosting Modern and Historical Handwritten Text Recognition with Deformable Convolutions
[Articolo su rivista]
Cascianelli, Silvia; Cornia, Marcella; Baraldi, Lorenzo; Cucchiara, Rita
abstract
Handwritten Text Recognition (HTR) in free-layout pages is a challenging image understanding task that can provide a relevant boost to the digitization of handwritten documents and reuse of their content. The task becomes even more challenging when dealing with historical documents due to the variability of the writing style and degradation of the page quality. State-of-the-art HTR approaches typically couple recurrent structures for sequence modeling with Convolutional Neural Networks for visual feature extraction. Since convolutional kernels are defined on fixed grids and focus on all input pixels independently while moving over the input image, this strategy disregards the fact that handwritten characters can vary in shape, scale, and orientation even within the same document and that the ink pixels are more relevant than the background ones. To cope with these specific HTR difficulties, we propose to adopt deformable convolutions, which can deform depending on the input at hand and better adapt to the geometric variations of the text. We design two deformable architectures and conduct extensive experiments on both modern and historical datasets. Experimental results confirm the suitability of deformable convolutions for the HTR task.
2022
- CaMEL: Mean Teacher Learning for Image Captioning
[Relazione in Atti di Convegno]
Barraco, Manuele; Stefanini, Matteo; Cornia, Marcella; Cascianelli, Silvia; Baraldi, Lorenzo; Cucchiara, Rita
abstract
2022
- Dual-Branch Collaborative Transformer for Virtual Try-On
[Relazione in Atti di Convegno]
Fenocchi, Emanuele; Morelli, Davide; Cornia, Marcella; Baraldi, Lorenzo; Cesari, Fabio; Cucchiara, Rita
abstract
Image-based virtual try-on has recently gained a lot of attention in both the scientific and fashion industry communities due to its challenging setting and practical real-world applications. While pure convolutional approaches have been explored to solve the task, Transformer-based architectures have not received significant attention yet. Following the intuition that self- and cross-attention operators can deal with long-range dependencies and hence improve the generation, in this paper we extend a Transformer-based virtual try-on model by adding a dual-branch collaborative module that can exploit cross-modal information at generation time. We perform experiments on the VITON dataset, which is the standard benchmark for the task, and on a recently collected virtual try-on dataset with multi-category clothing, Dress Code. Experimental results demonstrate the effectiveness of our solution over previous methods and show that Transformer-based architectures can be a viable alternative for virtual try-on.
2022
- Embodied Navigation at the Art Gallery
[Relazione in Atti di Convegno]
Bigazzi, Roberto; Landi, Federico; Cascianelli, Silvia; Cornia, Marcella; Baraldi, Lorenzo; Cucchiara, Rita
abstract
Embodied agents, trained to explore and navigate indoor photorealistic environments, have achieved impressive results on standard datasets and benchmarks. So far, experiments and evaluations have involved domestic and working scenes like offices, flats, and houses. In this paper, we build and release a new 3D space with unique characteristics: the one of a complete art museum. We name this environment ArtGallery3D (AG3D). Compared with existing 3D scenes, the collected space is ampler, richer in visual features, and provides very sparse occupancy information. This feature is challenging for occupancy-based agents which are usually trained in crowded domestic environments with plenty of occupancy information. Additionally, we annotate the coordinates of the main points of interest inside the museum, such as paintings, statues, and other items. Thanks to this manual process, we deliver a new benchmark for PointGoal navigation inside this new space. Trajectories in this dataset are far more complex and lengthy than existing ground-truth paths for navigation in Gibson and Matterport3D. We carry on extensive experimental evaluation using our new space for evaluation and prove that existing methods hardly adapt to this scenario. As such, we believe that the availability of this 3D model will foster future research and help improve existing solutions.
2022
- Explaining Transformer-based Image Captioning Models: An Empirical Analysis
[Articolo su rivista]
Cornia, Marcella; Baraldi, Lorenzo; Cucchiara, Rita
abstract
2022
- Focus on Impact: Indoor Exploration with Intrinsic Motivation
[Articolo su rivista]
Bigazzi, Roberto; Landi, Federico; Cascianelli, Silvia; Baraldi, Lorenzo; Cornia, Marcella; Cucchiara, Rita
abstract
2022
- Investigating Bidimensional Downsampling in Vision Transformer Models
[Relazione in Atti di Convegno]
Bruno, Paolo; Amoroso, Roberto; Cornia, Marcella; Cascianelli, Silvia; Baraldi, Lorenzo; Cucchiara, Rita
abstract
Vision Transformers (ViT) and other Transformer-based architectures for image classification have achieved promising performances in the last two years. However, ViT-based models require large datasets, memory, and computational power to obtain state-of-the-art results compared to more traditional architectures. The generic ViT model, indeed, maintains a full-length patch sequence during inference, which is redundant and lacks hierarchical representation. With the goal of increasing the efficiency of Transformer-based models, we explore the application of a 2D max-pooling operator on the outputs of Transformer encoders. We conduct extensive experiments on the CIFAR-100 dataset and the large ImageNet dataset and consider both accuracy and efficiency metrics, with the final goal of reducing the token sequence length without affecting the classification performance. Experimental results show that bidimensional downsampling can outperform previous classification approaches while requiring relatively limited computation resources.
2022
- Matching Faces and Attributes Between the Artistic and the Real Domain: the PersonArt Approach
[Articolo su rivista]
Cornia, Marcella; Tomei, Matteo; Baraldi, Lorenzo; Cucchiara, Rita
abstract
2022
- Retrieval-Augmented Transformer for Image Captioning
[Relazione in Atti di Convegno]
Sarto, Sara; Cornia, Marcella; Baraldi, Lorenzo; Cucchiara, Rita
abstract
2022
- Spot the Difference: A Novel Task for Embodied Agents in Changing Environments
[Relazione in Atti di Convegno]
Landi, Federico; Bigazzi, Roberto; Cornia, Marcella; Cascianelli, Silvia; Baraldi, Lorenzo; Cucchiara, Rita
abstract
2022
- The LAM Dataset: A Novel Benchmark for Line-Level Handwritten Text Recognition
[Relazione in Atti di Convegno]
Cascianelli, Silvia; Pippi, Vittorio; Maarand, Martin; Cornia, Marcella; Baraldi, Lorenzo; Kermorvant, Christopher; Cucchiara, Rita
abstract
2022
- The Unreasonable Effectiveness of CLIP features for Image Captioning: an Experimental Analysis
[Relazione in Atti di Convegno]
Barraco, Manuele; Cornia, Marcella; Cascianelli, Silvia; Baraldi, Lorenzo; Cucchiara, Rita
abstract
2021
- A Novel Attention-based Aggregation Function to Combine Vision and Language
[Relazione in Atti di Convegno]
Stefanini, Matteo; Cornia, Marcella; Baraldi, Lorenzo; Cucchiara, Rita
abstract
The joint understanding of vision and language has been recently gaining a lot of attention in both the Computer Vision and Natural Language Processing communities, with the emergence of tasks such as image captioning, image-text matching, and visual question answering. As both images and text can be encoded as sets or sequences of elements - like regions and words - proper reduction functions are needed to transform a set of encoded elements into a single response, like a classification or similarity score. In this paper, we propose a novel fully-attentive reduction method for vision and language. Specifically, our approach computes a set of scores for each element of each modality employing a novel variant of cross-attention, and performs a learnable and cross-modal reduction, which can be used for both classification and ranking. We test our approach on image-text matching and visual question answering, building fair comparisons with other reduction choices, on both COCO and VQA 2.0 datasets. Experimentally, we demonstrate that our approach leads to a performance increase on both tasks. Further, we conduct ablation studies to validate the role of each component of the approach.
2021
- Assessing the Role of Boundary-level Objectives in Indoor Semantic Segmentation
[Relazione in Atti di Convegno]
Amoroso, Roberto; Baraldi, Lorenzo; Cucchiara, Rita
abstract
Providing fine-grained and accurate segmentation maps of indoor scenes is a challenging task with relevant applications in the fields of augmented reality, image retrieval, and personalized robotics. While most of the recent literature on semantic segmentation has focused on outdoor scenarios, the generation of accurate indoor segmentation maps has been partially under-investigated. With the goal of increasing the accuracy of semantic segmentation in indoor scenarios, we focus on the analysis of boundary-level objectives, which foster the generation of fine-grained boundaries between different semantic classes and which have never been explored in the case of indoor segmentation. In particular, we test and devise variants of both the Boundary and Active Boundary losses, two recent proposals which deal with the prediction of semantic boundaries. Through experiments on the NYUDv2 dataset, we quantify the role of such losses in terms of accuracy and quality of boundary prediction and demonstrate the accuracy gain of the proposed variants.
2021
- Estimating (and fixing) the Effect of Face Obfuscation in Video Recognition
[Relazione in Atti di Convegno]
Tomei, Matteo; Baraldi, Lorenzo; Bronzin, Simone; Cucchiara, Rita
abstract
2021
- Explore and Explain: Self-supervised Navigation and Recounting
[Relazione in Atti di Convegno]
Bigazzi, Roberto; Landi, Federico; Cornia, Marcella; Cascianelli, Silvia; Baraldi, Lorenzo; Cucchiara, Rita
abstract
Embodied AI has been recently gaining attention as it aims to foster the development of autonomous and intelligent agents. In this paper, we devise a novel embodied setting in which an agent needs to explore a previously unknown environment while recounting what it sees during the path. In this context, the agent needs to navigate the environment driven by an exploration goal, select proper moments for description, and output natural language descriptions of relevant objects and scenes. Our model integrates a novel self-supervised exploration module with penalty, and a fully-attentive captioning model for explanation. Also, we investigate different policies for selecting proper moments for explanation, driven by information coming from both the environment and the navigation. Experiments are conducted on photorealistic environments from the Matterport3D dataset and investigate the navigation and explanation capabilities of the agent as well as the role of their interactions.
2021
- Improving Indoor Semantic Segmentation with Boundary-level Objectives
[Relazione in Atti di Convegno]
Amoroso, Roberto; Baraldi, Lorenzo; Cucchiara, Rita
abstract
While most of the recent literature on semantic segmentation has focused on outdoor scenarios, the generation of accurate indoor segmentation maps has been partially under-investigated, although being a relevant task with applications in augmented reality, image retrieval, and personalized robotics.
With the goal of increasing the accuracy of semantic segmentation in indoor scenarios, we develop and propose two novel boundary-level training objectives, which foster the generation of accurate boundaries between different semantic classes.
In particular, we take inspiration from the Boundary and Active Boundary losses, two recent proposals which deal with the prediction of semantic boundaries, and propose modified geometric distance functions that improve predictions at the boundary level.
Through experiments on the NYUDv2 dataset, we assess the appropriateness of our proposal in terms of accuracy and quality of boundary prediction and demonstrate its accuracy gain.
2021
- Learning to Read L'Infinito: Handwritten Text Recognition with Synthetic Training Data
[Relazione in Atti di Convegno]
Cascianelli, Silvia; Cornia, Marcella; Baraldi, Lorenzo; Piazzi, Maria Ludovica; Schiuma, Rosiana; Cucchiara, Rita
abstract
Deep learning-based approaches to Handwritten Text Recognition (HTR) have shown remarkable results on publicly available large datasets, both modern and historical. However, it is often the case that historical manuscripts are preserved in small collections, most of the time with unique characteristics in terms of paper support, author handwriting style, and language. State-of-the-art HTR approaches struggle to obtain good performance on such small manuscript collections, for which few training samples are available. In this paper, we focus on HTR on small historical datasets and propose a new historical dataset, which we call Leopardi, with the typical characteristics of small manuscript collections, consisting of letters by the poet Giacomo Leopardi, and devise strategies to deal with the training data scarcity scenario. In particular, we explore the use of carefully designed but cost-effective synthetic data for pre-training HTR models to be applied to small single-author manuscripts. Extensive experiments validate the suitability of the proposed approach, and both the Leopardi dataset and synthetic data will be available to favor further research in this direction.
2021
- Learning to Select: A Fully Attentive Approach for Novel Object Captioning
[Relazione in Atti di Convegno]
Cagrandi, Marco; Cornia, Marcella; Stefanini, Matteo; Baraldi, Lorenzo; Cucchiara, Rita
abstract
2021
- Multimodal Attention Networks for Low-Level Vision-and-Language Navigation
[Articolo su rivista]
Landi, Federico; Baraldi, Lorenzo; Cornia, Marcella; Corsini, Massimiliano; Cucchiara, Rita
abstract
Vision-and-Language Navigation (VLN) is a challenging task in which an agent needs to follow a language-specified path to reach a target destination. The goal gets even harder as the actions available to the agent get simpler and move towards low-level, atomic interactions with the environment. This setting takes the name of low-level VLN. In this paper, we strive for the creation of an agent able to tackle three key issues: multi-modality, long-term dependencies, and adaptability towards different locomotive settings. To that end, we devise "Perceive, Transform, and Act" (PTA): a fully-attentive VLN architecture that leaves the recurrent approach behind and the first Transformer-like architecture incorporating three different modalities -- natural language, images, and low-level actions for the agent control. In particular, we adopt an early fusion strategy to merge lingual and visual information efficiently in our encoder. We then propose to refine the decoding phase with a late fusion extension between the agent's history of actions and the perceptual modalities. We experimentally validate our model on two datasets: PTA achieves promising results in low-level VLN on R2R and achieves good performance in the recently proposed R4R benchmark. Our code is publicly available at https://github.com/aimagelab/perceive-transform-and-act.
2021
- Out of the Box: Embodied Navigation in the Real World
[Relazione in Atti di Convegno]
Bigazzi, Roberto; Landi, Federico; Cornia, Marcella; Cascianelli, Silvia; Baraldi, Lorenzo; Cucchiara, Rita
abstract
The research field of Embodied AI has witnessed substantial progress in visual navigation and exploration thanks to powerful simulating platforms and the availability of 3D data of indoor and photorealistic environments. These two factors have opened the doors to a new generation of intelligent agents capable of achieving nearly perfect PointGoal Navigation. However, such architectures are commonly trained with millions, if not billions, of frames and tested in simulation. Together with great enthusiasm, these results yield a question: how many researchers will effectively benefit from these advances?
In this work, we detail how to transfer the knowledge acquired in simulation into the real world. To that end, we describe the architectural discrepancies that damage the Sim2Real adaptation ability of models trained on the Habitat simulator and propose a novel solution tailored towards the deployment in real-world scenarios. We then deploy our models on a LoCoBot, a Low-Cost Robot equipped with a single Intel RealSense camera. Different from previous work, our testing scene is unavailable to the agent in simulation. The environment is also inaccessible to the agent beforehand, so it cannot count on scene-specific semantic priors. In this way, we reproduce a setting in which a research group (potentially from other fields) needs to employ the agent visual navigation capabilities as-a-Service. Our experiments indicate that it is possible to achieve satisfying results when deploying the obtained model in the real world.
2021
- RMS-Net: Regression and Masking for Soccer Event Spotting
[Relazione in Atti di Convegno]
Tomei, Matteo; Baraldi, Lorenzo; Calderara, Simone; Bronzin, Simone; Cucchiara, Rita
abstract
2021
- Revisiting The Evaluation of Class Activation Mapping for Explainability: A Novel Metric and Experimental Analysis
[Relazione in Atti di Convegno]
Poppi, Samuele; Cornia, Marcella; Baraldi, Lorenzo; Cucchiara, Rita
abstract
2021
- Video action detection by learning graph-based spatio-temporal interactions
[Articolo su rivista]
Tomei, Matteo; Baraldi, Lorenzo; Calderara, Simone; Bronzin, Simone; Cucchiara, Rita
abstract
Action Detection is a complex task that aims to detect and classify human actions in video clips. Typically, it has been addressed by processing fine-grained features extracted from a video classification backbone. Recently, thanks to the robustness of object and people detectors, a deeper focus has been added on relationship modelling. Following this line, we propose a graph-based framework to learn high-level interactions between people and objects, in both space and time. In our formulation, spatio-temporal relationships are learned through self-attention on a multi-layer graph structure which can connect entities from consecutive clips, thus considering long-range spatial and temporal dependencies. The proposed module is backbone independent by design and does not require end-to-end training. Extensive experiments are conducted on the AVA dataset, where our model demonstrates state-of-the-art results and consistent improvements over baselines built with different backbones. Code is publicly available at https://github.com/aimagelab/STAGE_action_detection.
2021
- Watch Your Strokes: Improving Handwritten Text Recognition with Deformable Convolutions
[Relazione in Atti di Convegno]
Cojocaru, Iulian; Cascianelli, Silvia; Baraldi, Lorenzo; Corsini, Massimiliano; Cucchiara, Rita
abstract
Handwritten Text Recognition (HTR) in free-layout pages is a valuable yet challenging task which aims to automatically understand handwritten texts. State-of-the-art approaches in this field usually encode input images with Convolutional Neural Networks, whose kernels are typically defined on a fixed grid and focus on all input pixels independently. However, this is in contrast with the sparse nature of handwritten pages, in which only pixels representing the ink of the writing are useful for the recognition task. Furthermore, the standard convolution operator is not explicitly designed to take into account the great variability in shape, scale, and orientation of handwritten characters. To overcome these limitations, we investigate the use of deformable convolutions for handwriting recognition. This type of convolution deform the convolution kernel according to the content of the neighborhood, and can therefore be more adaptable to geometric variations and other deformations of the text. Experiments conducted on the IAM and RIMES datasets demonstrate that the use of deformable convolutions is a promising direction for the design of novel architectures for handwritten text recognition.
2021
- Working Memory Connections for LSTM
[Articolo su rivista]
Landi, Federico; Baraldi, Lorenzo; Cornia, Marcella; Cucchiara, Rita
abstract
2020
- A Unified Cycle-Consistent Neural Model for Text and Image Retrieval
[Articolo su rivista]
Cornia, Marcella; Baraldi, Lorenzo; Tavakoli, Hamed R.; Cucchiara, Rita
abstract
2020
- Ai4ar: An ai-based mobile application for the automatic generation of ar contents
[Relazione in Atti di Convegno]
Pierdicca, R.; Paolanti, M.; Frontoni, E.; Baraldi, L.
abstract
Augmented reality (AR) is the process of using technology to superimpose images, text or sounds on top of what a person can already see. Art galleries and museums started to develop AR applications to increase engagement and provide an entirely new kind of exploration experience. However, the creation of contents results a very time consuming process, thus requiring an ad-hoc development for each painting to be increased. In fact, for the creation of an AR experience on any painting, it is necessary to choose the points of interest, to create digital content and then to develop the application. If this is affordable for the great masterpieces of an art gallery, it would be impracticable for an entire collection. In this context, the idea of this paper is to develop AR applications based on Artificial Intelligence. In particular, automatic captioning techniques are the key core for the implementation of AR application for improving the user experience in front of a painting or an artwork in general. The study has demonstrated the feasibility through a proof of concept application, implemented for hand held devices, and adds to the body of knowledge in mobile AR application as this approach has not been applied in this field before.
2020
- Explaining Digital Humanities by Aligning Images and Textual Descriptions
[Articolo su rivista]
Cornia, Marcella; Stefanini, Matteo; Baraldi, Lorenzo; Corsini, Massimiliano; Cucchiara, Rita
abstract
Replicating the human ability to connect Vision and Language has recently been gaining a lot of attention in the Computer Vision and the Natural Language Processing communities. This research effort has resulted in algorithms that can retrieve images from textual descriptions and vice versa, when realistic images and sentences with simple semantics are employed and when paired training data is provided. In this paper, we go beyond these limitations and tackle the design of visual-semantic algorithms in the domain of the Digital Humanities. This setting not only advertises more complex visual and semantic structures but also features a significant lack of training data which makes the use of fully-supervised approaches infeasible. With this aim, we propose a joint visual-semantic embedding that can automatically align illustrations and textual elements without paired supervision. This is achieved by transferring the knowledge learned on ordinary visual-semantic datasets to the artistic domain. Experiments, performed on two datasets specifically designed for this domain, validate the proposed strategies and quantify the domain shift between natural images and artworks.
2020
- Meshed-Memory Transformer for Image Captioning
[Relazione in Atti di Convegno]
Cornia, Marcella; Stefanini, Matteo; Baraldi, Lorenzo; Cucchiara, Rita
abstract
Transformer-based architectures represent the state of the art in sequence modeling tasks like machine translation and language understanding. Their applicability to multi-modal contexts like image captioning, however, is still largely under-explored. With the aim of filling this gap, we present M² - a Meshed Transformer with Memory for Image Captioning. The architecture improves both the image encoding and the language generation steps: it learns a multi-level representation of the relationships between image regions integrating learned a priori knowledge, and uses a mesh-like connectivity at decoding stage to exploit low- and high-level features. Experimentally, we investigate the performance of the M² Transformer and different fully-attentive models in comparison with recurrent ones. When tested on COCO, our proposal achieves a new state of the art in single-model and ensemble configurations on the "Karpathy" test split and on the online test server. We also assess its performances when describing objects unseen in the training set.
Trained models and code for reproducing the experiments are publicly available at :https://github.com/aimagelab/meshed-memory-transformer.
2020
- SMArT: Training Shallow Memory-aware Transformers for Robotic Explainability
[Relazione in Atti di Convegno]
Cornia, Marcella; Baraldi, Lorenzo; Cucchiara, Rita
abstract
2020
- Spaghetti Labeling: Directed Acyclic Graphs for Block-Based Connected Components Labeling
[Articolo su rivista]
Bolelli, Federico; Allegretti, Stefano; Baraldi, Lorenzo; Grana, Costantino
abstract
Connected Components Labeling is an essential step of many Image Processing and Computer Vision tasks. Since the first proposal of a labeling algorithm, which dates back to the sixties, many approaches have optimized the computational load needed to label an image. In particular, the use of decision forests and state prediction have recently appeared as valuable strategies to improve performance. However, due to the overhead of the manual construction of prediction states and the size of the resulting machine code, the application of these strategies has been restricted to small masks, thus ignoring the benefit of using a block-based approach. In this paper, we combine a block-based mask with state prediction and code compression: the resulting algorithm is modeled as a Directed Rooted Acyclic Graph with multiple entry points, which is automatically generated without manual intervention. When tested on synthetic and real datasets, in comparison with optimized implementations of state-of-the-art algorithms, the proposed approach shows superior performance, surpassing the results obtained by all compared approaches in all settings.
2020
- Towards Reliable Experiments on the Performance of Connected Components Labeling Algorithms
[Articolo su rivista]
Bolelli, Federico; Cancilla, Michele; Baraldi, Lorenzo; Grana, Costantino
abstract
The problem of labeling the connected components of a binary image is well-defined and several proposals have been presented in the past. Since an exact solution to the problem exists, algorithms mainly differ on their execution speed. In this paper, we propose and describe YACCLAB, Yet Another Connected Components Labeling Benchmark. Together with a rich and varied dataset, YACCLAB contains an open source platform to test new proposals and to compare them with publicly available competitors. Textual and graphical outputs are automatically generated for many kinds of tests, which analyze the methods from different perspectives. An extensive set of experiments among state-of-the-art techniques is reported and discussed.
2019
- A Deep-learning-based approach to VM behavior Identification in Cloud Systems
[Relazione in Atti di Convegno]
Stefanini, M.; Lancellotti, R.; Baraldi, L.; Calderara, S.
abstract
2019
- Art2Real: Unfolding the Reality of Artworks via Semantically-Aware Image-to-Image Translation
[Relazione in Atti di Convegno]
Tomei, Matteo; Cornia, Marcella; Baraldi, Lorenzo; Cucchiara, Rita
abstract
The applicability of computer vision to real paintings and artworks has been rarely investigated, even though a vast heritage would greatly benefit from techniques which can understand and process data from the artistic domain. This is partially due to the small amount of annotated artistic data, which is not even comparable to that of natural images captured by cameras. In this paper, we propose a semantic-aware architecture which can translate artworks to photo-realistic visualizations, thus reducing the gap between visual features of artistic and realistic data. Our architecture can generate natural images by retrieving and learning details from real photos through a similarity matching strategy which leverages a weakly-supervised semantic understanding of the scene. Experimental results show that the proposed technique leads to increased realism and to a reduction in domain shift, which improves the performance of pre-trained architectures for classification, detection, and segmentation. Code is publicly available at: https://github.com/aimagelab/art2real.
2019
- Artpedia: A New Visual-Semantic Dataset with Visual and Contextual Sentences in the Artistic Domain
[Relazione in Atti di Convegno]
Stefanini, Matteo; Cornia, Marcella; Baraldi, Lorenzo; Corsini, Massimiliano; Cucchiara, Rita
abstract
As vision and language techniques are widely applied to realistic images, there is a growing interest in designing visual-semantic models suitable for more complex and challenging scenarios. In this paper, we address the problem of cross-modal retrieval of images and sentences coming from the artistic domain. To this aim, we collect and manually annotate the Artpedia dataset that contains paintings and textual sentences describing both the visual content of the paintings and other contextual information. Thus, the problem is not only to match images and sentences, but also to identify which sentences actually describe the visual content of a given image. To this end, we devise a visual-semantic model that jointly addresses these two challenges by exploiting the latent alignment between visual and textual chunks. Experimental evaluations, obtained by comparing our model to different baselines, demonstrate the effectiveness of our solution and highlight the challenges of the proposed dataset. The Artpedia dataset is publicly available at: http://aimagelab.ing.unimore.it/artpedia.
2019
- Connected Components Labeling on DRAGs: Implementation and Reproducibility Notes
[Relazione in Atti di Convegno]
Bolelli, Federico; Cancilla, Michele; Baraldi, Lorenzo; Grana, Costantino
abstract
In this paper we describe the algorithmic implementation details of "Connected Components Labeling on DRAGs'' (Directed Rooted Acyclic Graphs), studying the influence of parameters on the results. Moreover, a detailed description of how to install, setup and use YACCLAB (Yet Another Connected Components LAbeling Benchmark) to test DRAG is provided.
2019
- Embodied Vision-and-Language Navigation with Dynamic Convolutional Filters
[Relazione in Atti di Convegno]
Landi, Federico; Baraldi, Lorenzo; Corsini, Massimiliano; Cucchiara, Rita
abstract
In Vision-and-Language Navigation (VLN), an embodied agent needs to reach a target destination with the only guidance of a natural language instruction. To explore the environment and progress towards the target location, the agent must perform a series of low-level actions, such as rotate, before stepping ahead. In this paper, we propose to exploit dynamic convolutional filters to encode the visual information and the lingual description in an efficient way. Differently from some previous works that abstract from the agent perspective and use high-level navigation spaces, we design a policy which decodes the information provided by dynamic convolution into a series of low-level, agent friendly actions. Results show that our model exploiting dynamic filters performs better than other architectures with traditional convolution, being the new state of the art for embodied VLN in the low-level action space. Additionally, we attempt to categorize recent work on VLN depending on their architectural choices and distinguish two main groups: we call them low-level actions and high-level actions models. To the best of our knowledge, we are the first to propose this analysis and categorization for VLN.
2019
- Image-to-Image Translation to Unfold the Reality of Artworks: an Empirical Analysis
[Relazione in Atti di Convegno]
Tomei, Matteo; Cornia, Marcella; Baraldi, Lorenzo; Cucchiara, Rita
abstract
State-of-the-art Computer Vision pipelines show poor performances on artworks and data coming from the artistic domain, thus limiting the applicability of current architectures to the automatic understanding of the cultural heritage. This is mainly due to the difference in texture and low-level feature distribution between artistic and real images, on which state-of-the-art approaches are usually trained. To enhance the applicability of pre-trained architectures on artistic data, we have recently proposed an unpaired domain translation approach which can translate artworks to photo-realistic visualizations. Our approach leverages semantically-aware memory banks of real patches, which are used to drive the generation of the translated image while improving its realism. In this paper, we provide additional analyses and experimental results which demonstrate the effectiveness of our approach. In particular, we evaluate the quality of generated results in the case of the translation of landscapes, portraits and of paintings coming from four different styles using automatic distance metrics. Also, we analyze the response of pre-trained architecture for classification, detection and segmentation both in terms of feature distribution and entropy of prediction, and show that our approach effectively reduces the domain shift of paintings. As an additional contribution, we also provide a qualitative analysis of the reduction of the domain shift for detection, segmentation and image captioning.
2019
- M-VAD Names: a Dataset for Video Captioning with Naming
[Articolo su rivista]
Pini, Stefano; Cornia, Marcella; Bolelli, Federico; Baraldi, Lorenzo; Cucchiara, Rita
abstract
Current movie captioning architectures are not capable of mentioning characters with their proper name, replacing them with a generic "someone" tag. The lack of movie description datasets with characters' visual annotations surely plays a relevant role in this shortage. Recently, we proposed to extend the M-VAD dataset by introducing such information. In this paper, we present an improved version of the dataset, namely M-VAD Names, and its semi-automatic annotation procedure. The resulting dataset contains 63k visual tracks and 34k textual mentions, all associated with character identities. To showcase the features of the dataset and quantify the complexity of the naming task, we investigate multimodal architectures to replace the "someone" tags with proper character names in existing video captions. The evaluation is further extended by testing this application on videos outside of the M-VAD Names dataset.
2019
- Recognizing social relationships from an egocentric vision perspective
[Capitolo/Saggio]
Alletto, Stefano; Cornia, Marcella; Baraldi, Lorenzo; Serra, Giuseppe; Cucchiara, Rita
abstract
In this chapter we address the problem of partitioning social gatherings into interacting groups in egocentric scenarios. People in the scene are tracked, their head pose and 3D location are estimated. Following the formalism of the f-formation, we define with the orientation and distance an inherently social pairwise feature capable of describing how two people stand in relation to one another. We present a Structural SVM based approach to learn how to weight each component of the feature vector depending on the social situation is applied to. To better understand the social dynamics, we also estimate what we call social relevance of each subject in a group using a saliency attentive model. Extensive tests on two publicly available datasets show that our solution achieves encouraging results when detecting social groups and their relevant subjects in the challenging egocentric scenarios.
2019
- Show, Control and Tell: A Framework for Generating Controllable and Grounded Captions
[Relazione in Atti di Convegno]
Cornia, Marcella; Baraldi, Lorenzo; Cucchiara, Rita
abstract
Current captioning approaches can describe images using black-box architectures whose behavior is hardly controllable and explainable from the exterior. As an image can be described in infinite ways depending on the goal and the context at hand, a higher degree of controllability is needed to apply captioning algorithms in complex scenarios. In this paper, we introduce a novel framework for image captioning which can generate diverse descriptions by allowing both grounding and controllability. Given a control signal in the form of a sequence or set of image regions, we generate the corresponding caption through a recurrent architecture which predicts textual chunks explicitly grounded on regions, following the constraints of the given control. Experiments are conducted on Flickr30k Entities and on COCO Entities, an extended version of COCO in which we add grounding annotations collected in a semi-automatic manner. Results demonstrate that our method achieves state of the art performances on controllable image captioning, in terms of caption quality and diversity. Code and annotations are publicly available at: https://github.com/aimagelab/show-control-and-tell.
2019
- Towards Cycle-Consistent Models for Text and Image Retrieval
[Relazione in Atti di Convegno]
Cornia, Marcella; Baraldi, Lorenzo; Rezazadegan Tavakoli, Hamed; Cucchiara, Rita
abstract
Cross-modal retrieval has been recently becoming an hot-spot research, thanks to the development of deeply-learnable architectures. Such architectures generally learn a joint multi-modal embedding space in which text and images could be projected and compared. Here we investigate a different approach, and reformulate the problem of cross-modal retrieval as that of learning a translation between the textual and visual domain. In particular, we propose an end-to-end trainable model which can translate text into image features and vice versa, and regularizes this mapping with a cycle-consistency criterion. Preliminary experimental evaluations show promising results with respect to ordinary visual-semantic models.
2019
- Visual-Semantic Alignment Across Domains Using a Semi-Supervised Approach
[Relazione in Atti di Convegno]
Carraggi, Angelo; Cornia, Marcella; Baraldi, Lorenzo; Cucchiara, Rita
abstract
Visual-semantic embeddings have been extensively used as a powerful model for cross-modal retrieval of images and sentences. In this setting, data coming from different modalities can be projected in a common embedding space, in which distances can be used to infer the similarity between pairs of images and sentences. While this approach has shown impressive performances on fully supervised settings, its application to semi-supervised scenarios has been rarely investigated. In this paper we propose a domain adaptation model for cross-modal retrieval, in which the knowledge learned from a supervised dataset can be transferred on a target dataset in which the pairing between images and sentences is not known, or not useful for training due to the limited size of the set. Experiments are performed on two target unsupervised scenarios, respectively related to the fashion and cultural heritage domain. Results show that our model is able to effectively transfer the knowledge learned on ordinary visual-semantic datasets, achieving promising results. As an additional contribution, we collect and release the dataset used for the cultural heritage domain.
2019
- What was Monet seeing while painting? Translating artworks to photo-realistic images
[Relazione in Atti di Convegno]
Tomei, Matteo; Baraldi, Lorenzo; Cornia, Marcella; Cucchiara, Rita
abstract
State of the art Computer Vision techniques exploit the availability of large-scale datasets, most of which consist of images captured from the world as it is. This brings to an incompatibility between such methods and digital data from the artistic domain, on which current techniques under-perform. A possible solution is to reduce the domain shift at the pixel level, thus translating artistic images to realistic copies. In this paper, we present a model capable of translating paintings to photo-realistic images, trained without paired examples. The idea is to enforce a patch level similarity between real and generated images, aiming to reproduce photo-realistic details from a memory bank of real images. This is subsequently adopted in the context of an unpaired image-to-image translation framework, mapping each image from one distribution to a new one belonging to the other distribution. Qualitative and quantitative results are presented on Monet, Cezanne and Van Gogh paintings translation tasks, showing that our approach increases the realism of generated images with respect to the CycleGAN approach.
2018
- A Hierarchical Quasi-Recurrent approach to Video Captioning
[Relazione in Atti di Convegno]
Bolelli, Federico; Baraldi, Lorenzo; Grana, Costantino
abstract
Video captioning has picked up a considerable measure of attention thanks to the use of Recurrent Neural Networks, since they can be utilized to both encode the input video and to create the corresponding description. In this paper, we present a recurrent video encoding scheme which can find and exploit the layered structure of the video. Differently from the established encoder-decoder approach, in which a video is encoded continuously by a recurrent layer, we propose to employ Quasi-Recurrent Neural Networks, further extending their basic cell with a boundary detector which can recognize discontinuity points between frames or segments and likewise modify the temporal connections of the encoding layer. We assess our approach on a large scale dataset, the Montreal Video Annotation dataset. Experiments demonstrate that our approach can find suitable levels of representation of the input information, while reducing the computational requirements.
2018
- Aligning Text and Document Illustrations: towards Visually Explainable Digital Humanities
[Relazione in Atti di Convegno]
Baraldi, Lorenzo; Cornia, Marcella; Grana, Costantino; Cucchiara, Rita
abstract
While several approaches to bring vision and language together are emerging, none of them has yet addressed the digital humanities domain, which, nevertheless, is a rich source of visual and textual data. To foster research in this direction, we investigate the learning of visual-semantic embeddings for historical document illustrations, devising both supervised and semi-supervised approaches. We exploit the joint visual-semantic embeddings to automatically align illustrations and textual elements, thus providing an automatic annotation of the visual content of a manuscript. Experiments are performed on the Borso d'Este Holy Bible, one of the most sophisticated illuminated manuscript from the Renaissance, which we manually annotate aligning every illustration with textual commentaries written by experts. Experimental results quantify the domain shift between ordinary visual-semantic datasets and the proposed one, validate the proposed strategies, and devise future works on the same line.
2018
- Attentive Models in Vision: Computing Saliency Maps in the Deep Learning Era
[Articolo su rivista]
Cornia, Marcella; Abati, Davide; Baraldi, Lorenzo; Palazzi, Andrea; Calderara, Simone; Cucchiara, Rita
abstract
Estimating the focus of attention of a person looking at an image or a video is a crucial step which can enhance many vision-based inference mechanisms: image segmentation and annotation, video captioning, autonomous driving are some examples. The early stages of the attentive behavior are typically bottom-up; reproducing the same mechanism means to find the saliency embodied in the images, i.e. which parts of an image pop out of a visual scene. This process has been studied for decades both in neuroscience and in terms of computational models for reproducing the human cortical process. In the last few years, early models have been replaced by deep learning architectures, that outperform any early approach compared against public datasets. In this paper, we discuss the effectiveness of convolutional neural networks (CNNs) models in saliency prediction. We present a set of Deep Learning architectures developed by us, which can combine both bottom-up cues and higher-level semantics, and extract spatio-temporal features by means of 3D convolutions to model task-driven attentive behaviors. We will show how these deep networks closely recall the early saliency models, although improved with the semantics learned from the human ground-truth. Eventually, we will present a use-case in which saliency prediction is used to improve the automatic description of images.
2018
- Automatic Image Cropping and Selection using Saliency: an Application to Historical Manuscripts
[Relazione in Atti di Convegno]
Cornia, Marcella; Pini, Stefano; Baraldi, Lorenzo; Cucchiara, Rita
abstract
Automatic image cropping techniques are particularly important to improve the visual quality of cropped images and can be applied to a wide range of applications such as photo-editing, image compression, and thumbnail selection. In this paper, we propose a saliency-based image cropping method which produces significant cropped images by only relying on the corresponding saliency maps. Experiments on standard image cropping datasets demonstrate the benefit of the proposed solution with respect to other cropping methods. Moreover, we present an image selection method that can be effectively applied to automatically select the most representative pages of historical manuscripts thus improving the navigation of historical digital libraries.
2018
- Connected Components Labeling on DRAGs
[Relazione in Atti di Convegno]
Bolelli, Federico; Baraldi, Lorenzo; Cancilla, Michele; Grana, Costantino
abstract
In this paper we introduce a new Connected Components Labeling (CCL) algorithm which exploits a novel approach to model decision problems as Directed Acyclic Graphs with a root, which will be called Directed Rooted Acyclic Graphs (DRAGs). This structure supports the use of sets of equivalent actions, as required by CCL, and optimally leverages these equivalences to reduce the number of nodes (decision points). The advantage of this representation is that a DRAG, differently from decision trees usually exploited by the state-of-the-art algorithms, will contain only the minimum number of nodes required to reach the leaf corresponding to a set of condition values. This combines the benefits of using binary decision trees with a reduction of the machine code size. Experiments show a consistent improvement of the execution time when the model is applied to CCL.
2018
- LAMV: Learning to align and match videos with kernelized temporal layers
[Relazione in Atti di Convegno]
Baraldi, Lorenzo; Douze, Matthijs; Cucchiara, Rita; Jégou, Hervé
abstract
This paper considers a learnable approach for comparing and aligning videos. Our architecture builds upon and revisits temporal match kernels within neural networks: we propose a new temporal layer that finds temporal alignments by maximizing the scores between two sequences of vectors, according to a time-sensitive similarity metric parametrized in the Fourier domain. We learn this layer with a temporal proposal strategy, in which we minimize a triplet loss that takes into account both the localization accuracy and the recognition rate. We evaluate our approach on video alignment, copy detection and event retrieval. Our approach outperforms the state on the art on temporal video alignment and video copy detection datasets in comparable setups. It also attains the best reported results for particular event search, while precisely aligning videos.
2018
- Paying More Attention to Saliency: Image Captioning with Saliency and Context Attention
[Articolo su rivista]
Cornia, Marcella; Baraldi, Lorenzo; Serra, Giuseppe; Cucchiara, Rita
abstract
Image captioning has been recently gaining a lot of attention thanks to the impressive achievements shown by deep captioning architectures, which combine Convolutional Neural Networks to extract image representations, and Recurrent Neural Networks to generate the corresponding captions. At the same time, a significant research effort has been dedicated to the development of saliency prediction models, which can predict human eye fixations. Despite saliency information could be useful to condition an image captioning architecture, by providing an indication of what is salient and what is not, no model has yet succeeded in effectively incorporating these two techniques. In this work, we propose an image captioning approach in which a generative recurrent neural network can focus on different parts of the input image during the generation of the caption, by exploiting the conditioning given by a saliency prediction model on which parts of the image are salient and which are contextual. We demonstrate, through extensive quantitative and qualitative experiments on large scale datasets, that our model achieves superior performances with respect to different image captioning baselines with and without saliency. Finally, we also show that the trained model can focus on salient and contextual regions during the generation of the caption in an appropriate way.
2018
- Predicting Human Eye Fixations via an LSTM-based Saliency Attentive Model
[Articolo su rivista]
Cornia, Marcella; Baraldi, Lorenzo; Serra, Giuseppe; Cucchiara, Rita
abstract
Data-driven saliency has recently gained a lot of attention thanks to the use of Convolutional Neural Networks for predicting gaze fixations. In this paper we go beyond standard approaches to saliency prediction, in which gaze maps are computed with a feed-forward network, and present a novel model which can predict accurate saliency maps by incorporating neural attentive mechanisms. The core of our solution is a Convolutional LSTM that focuses on the most salient regions of the input image to iteratively refine the predicted saliency map. Additionally, to tackle the center bias typical of human eye fixations, our model can learn a set of prior maps generated with Gaussian functions. We show, through an extensive evaluation, that the proposed architecture outperforms the current state of the art on public saliency prediction datasets. We further study the contribution of each key component to demonstrate their robustness on different scenarios.
2018
- SAM: Pushing the Limits of Saliency Prediction Models
[Relazione in Atti di Convegno]
Cornia, Marcella; Baraldi, Lorenzo; Serra, Giuseppe; Cucchiara, Rita
abstract
The prediction of human eye fixations has been recently gaining a lot of attention thanks to the improvements shown by deep architectures. In our work, we go beyond classical feed-forward networks to predict saliency maps and propose a Saliency Attentive Model which incorporates neural attention mechanisms to iteratively refine predictions. Experiments demonstrate that the proposed strategy overcomes by a considerable margin the state of the art on the largest dataset available for saliency prediction. Here, we provide experimental results on other popular saliency datasets to confirm the effectiveness and the generalization capabilities of our model, which enable us to reach the state of the art on all considered datasets.
2017
- A Video Library System Using Scene Detection and Automatic Tagging
[Relazione in Atti di Convegno]
Baraldi, Lorenzo; Grana, Costantino; Cucchiara, Rita
abstract
We present a novel video browsing and retrieval system for edited videos, in which videos are automatically decomposed into meaningful and storytelling parts (i.e. scenes) and tagged according to their transcript. The system relies on a Triplet Deep Neural Network which exploits multimodal features, and has been implemented as a set of extensions to the eXo Platform Enterprise Content Management System (ECMS). This set of extensions enable the interactive visualization of a video, its automatic and semi-automatic annotation, as well as a keyword-based search inside the video collection. The platform also allows a natural integration with third-party add-ons, so that automatic annotations can be exploited outside the proposed platform.
2017
- Attentive Models in Vision: Computing Saliency Maps in the Deep Learning Era
[Relazione in Atti di Convegno]
Cornia, Marcella; Abati, Davide; Baraldi, Lorenzo; Palazzi, Andrea; Calderara, Simone; Cucchiara, Rita
abstract
Estimating the focus of attention of a person looking at an image or a video is a crucial step which can enhance many vision-based inference mechanisms: image segmentation and annotation, video captioning, autonomous driving are some examples. The early stages of the attentive behavior are typically bottom-up; reproducing the same mechanism means to find the saliency embodied in the images, i.e. which parts of an image pop out of a visual scene. This process has been studied for decades in neuroscience and in terms of computational models for reproducing the human cortical process.
In the last few years, early models have been replaced by deep learning architectures, that outperform any early approach compared against public datasets.
In this paper, we propose a discussion on why convolutional neural networks (CNNs) are so accurate in saliency prediction. We present our DL architectures which combine both bottom-up cues and higher-level semantics, and incorporate the concept of time in the attentional process through LSTM recurrent architectures. Eventually, we present a video-specific architecture based on the C3D network, which can extracts spatio-temporal features by means of 3D convolutions to model task-driven attentive behaviors. The merit of this work is to show how these deep networks are not mere brute-force methods tuned on massive amount of data, but represent well-defined architectures which recall very closely the early saliency models, although improved with the semantics learned by human ground-thuth.
2017
- Hierarchical Boundary-Aware Neural Encoder for Video Captioning
[Relazione in Atti di Convegno]
Baraldi, Lorenzo; Grana, Costantino; Cucchiara, Rita
abstract
The use of Recurrent Neural Networks for video captioning has recently gained a lot of attention, since they can be used both to encode the input video and to generate the corresponding description. In this paper, we present a recurrent video encoding scheme which can discover and leverage the hierarchical structure of the video. Unlike the classical encoder-decoder approach, in which a video is encoded continuously by a recurrent layer, we propose a novel LSTM cell, which can identify discontinuity points between frames or segments and modify the temporal connections of the encoding layer accordingly. We evaluate our approach on three large-scale datasets: the Montreal Video Annotation dataset, the MPII Movie Description dataset and the Microsoft Video Description Corpus. Experiments show that our approach can discover appropriate hierarchical representations of input videos and improve the state of the art results on movie description datasets.
2017
- Layout analysis and content classification in digitized books
[Relazione in Atti di Convegno]
Corbelli, Andrea; Baraldi, Lorenzo; Balducci, Fabrizio; Grana, Costantino; Cucchiara, Rita
abstract
Automatic layout analysis has proven to be extremely important in the process of digitization of large amounts of documents. In this paper we present a mixed approach to layout analysis, introducing a SVM-aided layout segmentation process and a classification process based on local and geometrical features. The final output of the automatic analysis algorithm is a complete and structured annotation in JSON format, containing the digitalized text as well as all the references to the illustrations of the input page, and which can be used by visualization interfaces as well as annotation interfaces. We evaluate our algorithm on a large dataset built upon the first volume of the “Enciclopedia Treccani”.
2017
- Modeling Multimodal Cues in a Deep Learning-based Framework for Emotion Recognition in the Wild
[Relazione in Atti di Convegno]
Pini, Stefano; Ben Ahmed, Olfa; Cornia, Marcella; Baraldi, Lorenzo; Cucchiara, Rita; Huet, Benoit
abstract
In this paper, we propose a multimodal deep learning architecture for emotion recognition in video regarding our participation to the audio-video based sub-challenge of the Emotion Recognition in the Wild 2017 challenge. Our model combines cues from multiple video modalities, including static facial features, motion patterns related to the evolution of the human expression over time, and audio information. Specifically, it is composed of three sub-networks trained separately: the first and second ones extract static visual features and dynamic patterns through 2D and 3D Convolutional Neural Networks (CNN), while the third one consists in a pretrained audio network which is used to extract useful deep acoustic signals from video. In the audio branch, we also apply Long Short Term Memory (LSTM) networks in order to capture the temporal evolution of the audio features. To identify and exploit possible relationships among different modalities, we propose a fusion network that merges cues from the different modalities in one representation. The proposed architecture outperforms the challenge baselines (38.81% and 40.47%): we achieve an accuracy of 50.39% and 49.92% respectively on the validation and the testing data.
2017
- NeuralStory: an Interactive Multimedia System for Video Indexing and Re-use
[Relazione in Atti di Convegno]
Baraldi, Lorenzo; Grana, Costantino; Cucchiara, Rita
abstract
In the last years video has been swamping the Internet: websites, social networks, and business multimedia systems are adopting video as the most important form of communication and information. Video are normally accessed as a whole and are not indexed in the visual content. Thus, they are often uploaded as short, manually cut clips with user-provided annotations, keywords and tags for retrieval.
In this paper, we propose a prototype multimedia system which addresses these two limitations: it overcomes the need of human intervention in the video setting, thanks to fully deep learning-based solutions, and decomposes the storytelling structure of the video into coherent parts. These parts can be shots, key-frames, scenes and semantically related stories, and are exploited to provide an automatic annotation of the visual content, so that parts of video can be easily retrieved. This also allows a principled re-use of the video itself: users of the platform can indeed produce new storytelling by means of multi-modal presentations, add text and other media, and propose a different visual organization of the content. We present the overall solution, and some experiments on the re-use capability of our platform in edutainment by conducting an extensive user valuation %with students from primary schools.
2017
- Preface
[Relazione in Atti di Convegno]
Grana, C.; Baraldi, L.
abstract
2017
- Recognizing and Presenting the Storytelling Video Structure with Deep Multimodal Networks
[Articolo su rivista]
Baraldi, Lorenzo; Grana, Costantino; Cucchiara, Rita
abstract
In this paper, we propose a novel scene detection algorithm which employs semantic, visual, textual and audio cues. We also show how the hierarchical decomposition of the storytelling video structure can improve retrieval results presentation with semantically and aesthetically effective thumbnails. Our method is built upon two advancements of the state of the art: 1) semantic feature extraction which builds video specific concept detectors; 2) multimodal feature embedding learning, that maps the feature vector of a shot to a space in which the Euclidean distance has task specific semantic properties. The proposed method is able to decompose the video in annotated temporal segments which allow for a query specific thumbnail extraction. Extensive experiments are performed on different data sets to demonstrate the effectiveness of our algorithm. An in-depth discussion on how to deal with the subjectivity of the task is conducted and a strategy to overcome the problem is suggested.
2017
- Towards Video Captioning with Naming: a Novel Dataset and a Multi-Modal Approach
[Relazione in Atti di Convegno]
Pini, Stefano; Cornia, Marcella; Baraldi, Lorenzo; Cucchiara, Rita
abstract
Current approaches for movie description lack the ability to name characters with their proper names, and can only indicate people with a generic "someone" tag. In this paper we present two contributions towards the development of video description architectures with naming capabilities: firstly, we collect and release an extension of the popular Montreal Video Annotation Dataset in which the visual appearance of each character is linked both through time and to textual mentions in captions. We annotate, in a semi-automatic manner, a total of 53k face tracks and 29k textual mentions on 92 movies. Moreover, to underline and quantify the challenges of the task of generating captions with names, we present different multi-modal approaches to solve the problem on already generated captions.
2017
- Visual Saliency for Image Captioning in New Multimedia Services
[Relazione in Atti di Convegno]
Cornia, Marcella; Baraldi, Lorenzo; Serra, Giuseppe; Cucchiara, Rita
abstract
Image and video captioning are important tasks in visual data analytics, as they concern the capability of describing visual content in natural language. They are the pillars of query answering systems, improve indexing and search and allow a natural form of human-machine interaction. Even though promising deep learning strategies are becoming popular, the heterogeneity of large image archives makes this task still far from being solved. In this paper we explore how visual saliency prediction can support image captioning. Recently, some forms of unsupervised machine attention mechanisms have been spreading, but the role of human attention prediction has never been examined extensively for captioning. We propose a machine attention model driven by saliency prediction to provide captions in images, which can be exploited for many services on cloud and on multimedia data. Experimental evaluations are conducted on the SALICON dataset, which provides groundtruths for both saliency and captioning, and on the large Microsoft COCO dataset, the most widely used for image captioning.
2016
- A Browsing and Retrieval System for Broadcast Videos using Scene Detection and Automatic Annotation
[Relazione in Atti di Convegno]
Baraldi, Lorenzo; Grana, Costantino; Messina, Alberto; Cucchiara, Rita
abstract
This paper presents a novel video access and retrieval system for edited videos. The key element of the proposal is that videos are automatically decomposed into semantically coherent parts (called scenes) to provide a more manageable unit for browsing, tagging and searching. The system features an automatic annotation pipeline, with which videos are tagged by exploiting both the transcript and the video itself. Scenes can also be retrieved with textual queries; the best thumbnail for a query is selected according to both semantics and aesthetics criteria.
2016
- A Deep Multi-Level Network for Saliency Prediction
[Relazione in Atti di Convegno]
Cornia, Marcella; Baraldi, Lorenzo; Serra, Giuseppe; Cucchiara, Rita
abstract
This paper presents a novel deep architecture for saliency prediction. Current state of the art models for saliency prediction employ Fully Convolutional networks that perform a non-linear combination of features extracted from the last convolutional layer to predict saliency maps. We propose an architecture which, instead, combines features extracted at different levels of a Convolutional Neural Network (CNN). Our model is composed of three main blocks: a feature extraction CNN, a feature encoding network, that weights low and high level feature maps, and a prior learning network.
We compare our solution with state of the art saliency models on two public benchmarks datasets. Results show that our model outperforms under all evaluation metrics on the SALICON dataset, which is currently the largest public dataset for saliency prediction, and achieves competitive results on the MIT300 benchmark.
2016
- Analysis and Re-use of Videos in Educational Digital Libraries with Automatic Scene Detection
[Relazione in Atti di Convegno]
Baraldi, Lorenzo; Grana, Costantino; Cucchiara, Rita
abstract
The advent of modern approaches to education, like Massive Open Online Courses (MOOC), made video the basic media for educating and transmitting knowledge. However, IT tools are still not adequate to allow video content re-use, tagging, annotation and personalization. In this paper we analyze the problem of identifying coherent sequences, called scenes, in order to provide the users with a more manageable editing unit. A simple spectral clustering technique is proposed and compared with state-of-the-art results. We also discuss correct ways to evaluate the performance of automatic scene detection algorithms.
2016
- Context Change Detection for an Ultra-Low Power Low-Resolution Ego-Vision Imager
[Relazione in Atti di Convegno]
Paci, Francesco; Baraldi, Lorenzo; Serra, Giuseppe; Cucchiara, Rita; Benini, Luca
abstract
With the increasing popularity of wearable cameras, such as GoPro or Narrative Clip, research on continuous activity monitoring from egocentric cameras has received a lot of attention. Research in hardware and software is devoted to find new efficient, stable and long-time running solutions;
however, devices are too power-hungry for truly always-on operation, and are aggressively duty-cycled to achieve acceptable lifetimes. In this paper we present a wearable system for context change detection based on an egocentric camera with ultra-low power consumption that can collect data 24/7. Although the resolution of the captured images is low, experimental results in real scenarios demonstrate how our approach, based on Siamese Neural Networks, can achieve visual context awareness. In particular, we compare our solution with hand-crafted features and with state of art technique and propose a novel and challenging dataset composed of roughly 30000 low-resolution images.
2016
- Historical Document Digitization through Layout Analysis and Deep Content Classification
[Relazione in Atti di Convegno]
Corbelli, Andrea; Baraldi, Lorenzo; Grana, Costantino; Cucchiara, Rita
abstract
Document layout segmentation and recognition is an important task in the creation of digitized documents collections, especially when dealing with historical documents.
This paper presents an hybrid approach to layout segmentation as well as a strategy to classify document regions, which is applied to the process of digitization of an historical encyclopedia. Our layout analysis method merges a classic top-down approach and a bottom-up classification process based on local geometrical features, while regions are classified by means of features extracted from a Convolutional Neural Network merged in a Random Forest classifier. Experiments are conducted on the first volume of the ``Enciclopedia Treccani'', a large dataset containing 999 manually annotated pages from the historical Italian encyclopedia.
2016
- Multi-Level Net: a Visual Saliency Prediction Model
[Relazione in Atti di Convegno]
Cornia, Marcella; Baraldi, Lorenzo; Serra, Giuseppe; Cucchiara, Rita
abstract
State of the art approaches for saliency prediction are based on Full Convolutional Networks, in which saliency maps are built using the last layer. In contrast, we here present a novel model that predicts saliency maps exploiting a non-linear combination of features coming from different layers of the network. We also present a new loss function to deal with the imbalance issue on saliency masks. Extensive results on three public datasets demonstrate the robustness of our solution. Our model outperforms the state of the art on SALICON, which is the largest and unconstrained dataset available, and obtains competitive results on MIT300 and CAT2000 benchmarks.
2016
- Optimized Connected Components Labeling with Pixel Prediction
[Relazione in Atti di Convegno]
Grana, Costantino; Baraldi, Lorenzo; Bolelli, Federico
abstract
In this paper we propose a new paradigm for connected components labeling, which employs a general approach to minimize the number of memory accesses, by exploiting the information provided by already seen pixels, removing the need to check them again. The scan phase of our proposed algorithm is ruled by a forest of decision trees connected into a single graph. Every tree derives from a reduction of the complete optimal decision tree. Experimental results demonstrated that on low density images our method is slightly faster than the fastest conventional labeling algorithms.
2016
- Scene-driven Retrieval in Edited Videos using Aesthetic and Semantic Deep Features
[Relazione in Atti di Convegno]
Baraldi, Lorenzo; Grana, Costantino; Cucchiara, Rita
abstract
This paper presents a novel retrieval pipeline for video collections, which aims to retrieve the most significant parts of an edited video for a given query, and represent them with thumbnails which are at the same time semantically meaningful and aesthetically remarkable. Videos are first segmented into coherent and story-telling scenes, then a retrieval algorithm based on deep learning is proposed to retrieve the most significant scenes for a textual query. A ranking strategy based on deep features is finally used to tackle the problem of visualizing the best thumbnail. Qualitative and quantitative experiments are conducted on a collection of edited videos to demonstrate the effectiveness of our approach.
2016
- Shot, scene and keyframe ordering for interactive video re-use
[Relazione in Atti di Convegno]
Baraldi, L.; Grana, C.; Borghi, G.; Vezzani, R.; Cucchiara, R.
abstract
This paper presents a complete system for shot and scene detection in broadcast videos, as well as a method to select the best representative key-frames, which could be used in new interactive interfaces for accessing large collections of edited videos. The final goal is to enable an improved access to video footage and the re-use of video content with the direct management of user-selected video-clips.
2016
- YACCLAB - Yet Another Connected Components Labeling Benchmark
[Relazione in Atti di Convegno]
Grana, Costantino; Bolelli, Federico; Baraldi, Lorenzo; Vezzani, Roberto
abstract
The problem of labeling the connected components (CCL) of a binary image is well-defined and several proposals have been presented in the past. Since an exact solution to the problem exists and should be mandatory provided as output, algorithms mainly differ on their execution speed. In this paper, we propose and describe YACCLAB, Yet Another Connected Components Labeling Benchmark. Together with a rich and varied dataset, YACCLAB contains an open source platform to test new proposals and to compare them with publicly available competitors. Textual and graphical outputs are automatically generated for three kinds of test, which analyze the methods from different perspectives. The fairness of the comparisons is guaranteed by running on the same system and over the same datasets. Examples of usage and the corresponding comparisons among state-of-the-art techniques are reported to confirm the potentiality of the benchmark.
2015
- A Deep Siamese Network for Scene Detection in Broadcast Videos
[Relazione in Atti di Convegno]
Baraldi, Lorenzo; Grana, Costantino; Cucchiara, Rita
abstract
We present a model that automatically divides broadcast videos into coherent scenes by learning a distance measure between shots. Experiments are performed to demonstrate the effectiveness of our approach by comparing our algorithm against recent proposals for automatic scene segmentation. We also propose an improved performance measure that aims to reduce the gap between numerical evaluation and expected results, and propose and release a new benchmark dataset.
2015
- Gesture Recognition using Wearable Vision Sensors to Enhance Visitors' Museum Experiences
[Articolo su rivista]
Baraldi, Lorenzo; Paci, Francesco; Serra, Giuseppe; Cucchiara, Rita
abstract
We introduce a novel approach to cultural heritage experience: by means of ego-vision embedded devices we develop a system, which offers a more natural and entertaining way of accessing museum knowledge. Our method is based on distributed self-gesture and artwork recognition, and does not need fixed cameras nor radio-frequency identifications sensors. We propose the use of dense trajectories sampled around the hand region to perform self-gesture recognition, understanding the way a user naturally interacts with an artwork, and demonstrate that our approach can benefit from distributed training. We test our algorithms on publicly available data sets and we extend our experiments to both virtual and real museum scenarios, where our method shows robustness when challenged with real-world data. Furthermore, we run an extensive performance analysis on our ARM-based wearable device.
2015
- Measuring scene detection performance
[Relazione in Atti di Convegno]
Baraldi, Lorenzo; Grana, Costantino; Cucchiara, Rita
abstract
In this paper we evaluate the performance of scene detection techniques, starting from the classic precision/recall approach, moving to the better designed coverage/overflow measures, and finally proposing an improved metric, in order to solve frequently observed cases in which the numeric interpretation is different from the expected results. Numerical evaluation is performed on two recent proposals for automatic scene detection, and comparing them with a simple but effective novel approach. Experimental results are conducted to show how different measures may lead to different interpretations.
2015
- Scene segmentation using temporal clustering for accessing and re-using broadcast video
[Relazione in Atti di Convegno]
Baraldi, Lorenzo; Grana, Costantino; Cucchiara, Rita
abstract
Scene detection is a fundamental tool for allowing effective video browsing and re-using. In this paper we present a model that automatically divides videos into coherent scenes, which is based on a novel combination of local image descriptors and temporal clustering techniques. Experiments are performed to demonstrate the effectiveness of our approach, by comparing our algorithm against two recent proposals for automatic scene segmentation. We also propose improved performance measures that aim to reduce the gap between numerical evaluation and expected results.
2015
- Shot and Scene Detection via Hierarchical Clustering for Re-using Broadcast Video
[Relazione in Atti di Convegno]
Baraldi, Lorenzo; Grana, Costantino; Cucchiara, Rita
abstract
Video decomposition techniques are fundamental tools for allowing effective video browsing and re-using. In this work, we consider the problem of segmenting broadcast videos into coherent scenes, and propose a scene detection algorithm based on hierarchical clustering, along with a very fast state-of-the-art shot segmentation approach. Experiments are performed to demonstrate the effectiveness of our algorithms, by comparing against recent proposals for automatic shot and scene segmentation.
2014
- Gesture Recognition in Ego-Centric Videos using Dense Trajectories and Hand Segmentation
[Relazione in Atti di Convegno]
Baraldi, Lorenzo; Paci, Francesco; Serra, Giuseppe; Benini, Luca; Cucchiara, Rita
abstract
We present a novel method for monocular hand gesture recognition in ego-vision scenarios that deals with static and dynamic gestures and can achieve high accuracy results using a few positive samples. Specifically, we use and extend the dense trajectories approach that has been successfully introduced for action recognition. Dense features are extracted around regions selected by a new hand segmentation technique that integrates superpixel classification, temporal and spatial coherence. We extensively testour gesture recognition and segmentation algorithms on public datasets and propose a new dataset shot with a wearable camera. In addition, we demonstrate that our solution can work in near real-time on a wearable device.
2013
- Hand Segmentation for Gesture Recognition in EGO-Vision
[Relazione in Atti di Convegno]
Serra, Giuseppe; Camurri, Marco; Baraldi, Lorenzo; Michela, Benedetti; Cucchiara, Rita
abstract
Portable devices for first-person camera views will play a central role in future interactive systems. One necessary step for feasible human-computer guided activities is gesture recognition, preceded by a reliable hand segmentation from egocentric vision. In this work we provide a novel hand segmentation algorithm based on Random Forest superpixel classification that integrates light, time and space consistency. We also propose a gesture recognition method based Exemplar SVMs since it requires a only small set of positive samples, hence it is well suitable for the egocentric video applications. Furthermore, this method is enhanced by using segmented images instead of full frames during test phase. Experimental results show that our hand segmentation algorithm outperforms the state-of-the-art approaches and improves the gesture recognition accuracy on both the publicly available EDSH dataset and our dataset designed for cultural heritage applications.