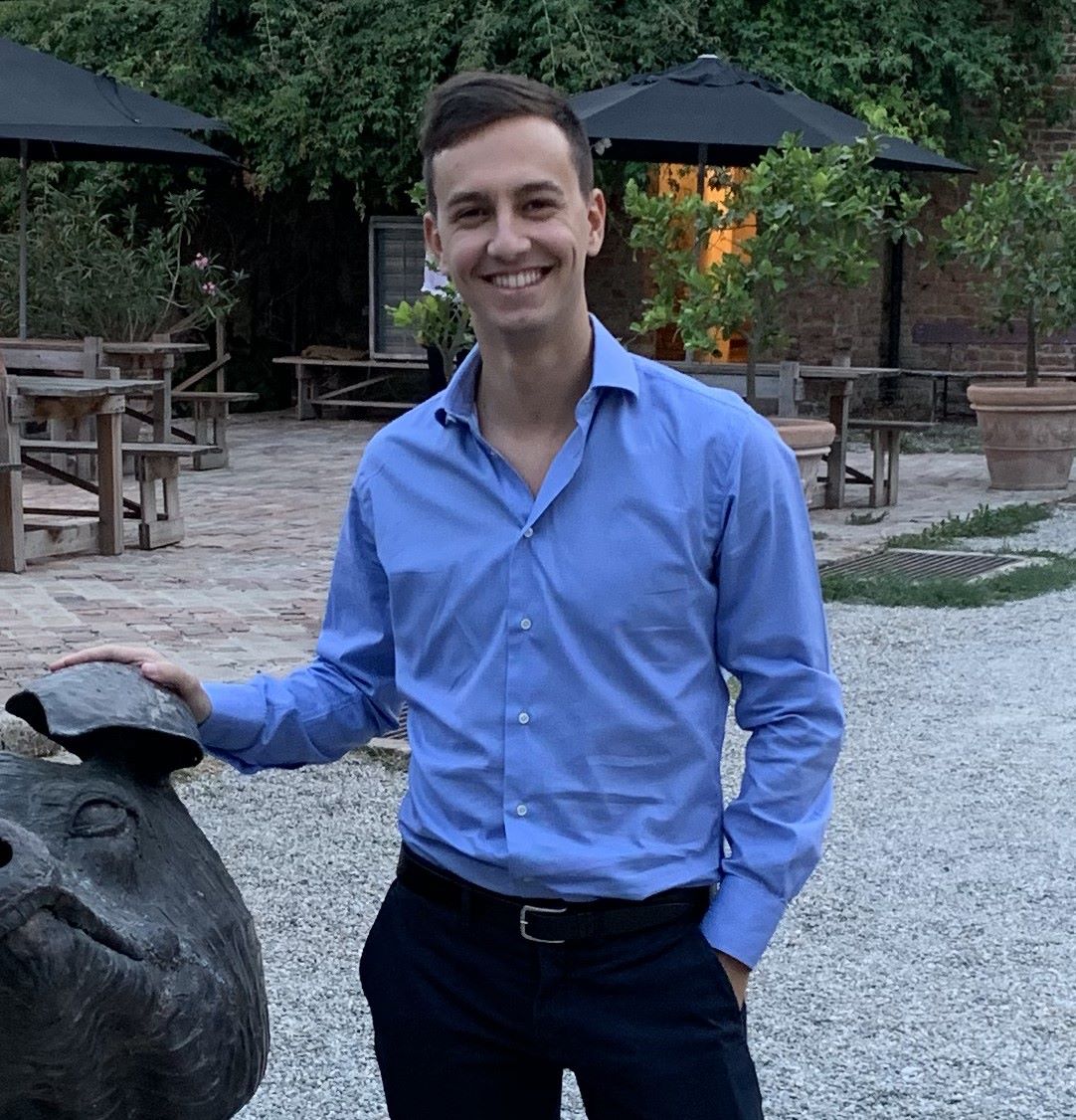 |
LUCA MARIOTTI
Docente a contratto Dipartimento di Giurisprudenza
|
Home |
Curriculum(pdf) |
Didattica |
Pubblicazioni
2023
- Automated Knowledge Graph Completion for Natural Language Understanding: Known Paths and Future Directions
[Relazione in Atti di Convegno]
Buzzega, G.; Guidetti, V.; Mandreoli, F.; Mariotti, L.; Belli, A.; Lombardi, P.
abstract
Knowledge Graphs (KGs) are large collections of structured data that can model real world knowledge and are important assets for the companies that employ them. KGs are usually constructed iteratively and often show a sparse structure. Also, as knowledge evolves, KGs must be updated and completed. Many automatic methods for KG Completion (KGC) have been proposed in the literature to reduce the costs associated with manual maintenance. Motivated by an industrial case study aiming to enrich a KG specifically designed for Natural Language Understanding tasks, this paper presents an overview of classical and modern deep learning completion methods. In particular, we delve into Large Language Models (LLMs), which are the most promising deep learning architectures. We show that their applications to KGC are affected by several shortcomings, namely they neglect the structure of KG and treat KGC as a classification problem. Such limitations, together with the brittleness of the LLMs themselves, stress the need to create KGC solutions at the interface between symbolic and neural approaches and lead to the way ahead for future research in intelligible corpus-based KGC.
2022
- On Designing a Time Sensitive Interaction Graph to Identify Twitter Opinion Leaders
[Relazione in Atti di Convegno]
Furini, M.; Mariotti, L.; Martoglia, R.; Montangero, M.
abstract
What happened on social media during the recent pandemic? Who was the opinion leader of the conversations? Who influenced whom? Were they medical doctors, ordinary people, scientific experts? Did health institutions play an important role in informing and updating citizens? Identifying opinion leaders within social platforms is of particular importance and, in this paper, we introduce the idea of a time sensitive interaction graph to identify opinion leaders within Twitter conversations. To evaluate our proposal, we focused on all the tweets posted on Twitter in the period 2020-21 and we considered just the ones that were Italian-written and were related to COVID-19. After mapping these tweets into the graph, we applied the PageRank algorithm to extract the opinion leaders of these conversations. Results show that our approach is effective in identifying opinion leaders and therefore it might be used to monitor the role that specific accounts (i.e., health authorities, politicians, city administrators) have within specific conversations.