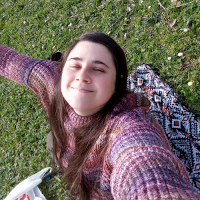 |
AMBRA DI PIANO
Dottorando Dipartimento di Ingegneria "Enzo Ferrari"
|
Home |
Curriculum(pdf) |
Pubblicazioni
2023
- The AGILE real-time analysis software system to detect short-transient events in the multi-messenger era
[Articolo su rivista]
Parmiggiani, N.; Bulgarelli, A.; Ursi, A.; Addis, A.; Baroncelli, L.; Fioretti, V.; Di Piano, A.; Panebianco, G.; Tavani, M.; Pittori, C.; Verrecchia, F.; Beneventano, D.
abstract
2022
- Agilepy: A Python Framework for AGILE Data Analysis
[Relazione in Atti di Convegno]
Bulgarelli, Andrea; Baroncelli, Leonardo; Addis, Antonio; Aboudan, Alessio; Parmiggiani, Nicol‘o.; Di Piano, Ambra; Fioretti, Valentina; Tavani, Marco; Pittori, Carlotta; Lucarelli, Fabrizio; Verrecchia, Francesco
abstract
The Italian AGILE space mission, with its Gamma-Ray Imaging Detector (GRID) instrument sensitive in the 30 Me–50 GeV γray energy band, has been operating since 2007. Agilepy is an open-source Python package to analyse AGILE/GRID data. The package is built on top of the command-line version of the AGILE Science Tools, developed by the AGILE Team, publicly available and released by ASI/SSDC. The primary purpose of the package is to provide an easy to use high-level interface to analyse AGILE/GRID data by simplifying the configuration of the tasks and ensuring straightforward access to the data. The current features are the generation and display of sky maps and light curves, the access to \gray sources catalogues, the analysis to perform spectral model and position fitting, the wavelet analysis. Agilepy also includes an interface tool providing the time evolution of the AGILE off-axis viewing angle for a chosen sky region. The Flare Advocate team also uses the tool to analyse the data during the daily monitoring of the γray sky. Agilepy (and its dependencies) can be easily installed using Anaconda....
2022
- Detection methods for the Cherenkov Telescope Array at very-short exposure times
[Articolo su rivista]
Di Piano, Ambra; Bulgarelli, Andrea; Fioretti, Valentina; Baroncelli, Leonardo; Parmiggiani, Nicolò; Longo, Francesco; Stamerra, Antonio; López-Oramas, Alicia; Stratta, Giulia; De Cesare, Giovanni
abstract
The Cherenkov Telescope Array (CTA) will be the next generation ground-based observatory for very-high-energy (VHE) gamma-ray astronomy, with the deployment of tens of highly sensitive and fast-reacting Cherenkov telescopes. It will cover a wide energy range (20 GeV - 300 TeV) with unprecedented sensitivity. To maximize the scientific return, the observatory will be provided with an online software system that will perform the first analysis of scientific data in real-time. This study investigates the precision and accuracy of available science tools and analysis techniques for the short-term detection of gamma-ray sources, in terms of sky localization, detection significance and, if significant detection is achieved, a first estimation of the integral photon flux. The scope is to evaluate the feasibility of the algorithms' implementation in the real-time analysis of CTA. In this contribution we present a general overview of the methods and some of the results for the test case of the short-term detection of a gamma-ray burst afterglow, as the VHE counterpart of a gravitational wave event....
2022
- GRB 220101A: AGILE detection
[Altro]
Ursi, A.; Menegoni, E.; Longo, F.; Pittori, C.; Verrecchia, F.; Tempesta, P.; Tavani, M.; Argan, A.; Cardillo, M.; Casentini, C.; Evangelista, Y.; Foffano, L.; Piano, G.; Lucarelli, F.; Bulgarelli, A.; di Piano, A.; Fioretti, V.; Fuschino, F.; Parmiggiani, N.; Marisaldi, M.; Pilia, M.; Trois, A.; Donnarumma, I.; Giuliani, A.; Agile, Team
abstract
The AGILE satellite detected the long GRB 220101A at T0 = 2022-01-01 05:11:22 (UTC), reported by Swift BAT (GCN #31347, #31348), Swift XRT (GCN #31349), Swift UVOT (GCN #31351), and Fermi LAT (GCN #31350). The burst is clearly visible in the AGILE scientific ratemeters of the SuperAGILE (SA; 20-60 keV), MiniCALorimeter (MCAL; 0.4-100 MeV), and AntiCoincidence (AC; 50-200 keV) detectors. The event lasted about 80 s and it released a total number of 14577 counts in the SA detector (above a background rate of 100 Hz), 95830 counts in the MCAL detector (above a background rate of 1140 Hz), and 256390 counts in the AC detector (above a background rate of 3215 Hz). The AGILE ratemeters light curves can be found at http://www.agilescienceapp.it/notices/GRB220101A_AGILE_RM.png . The event also triggered a partial high time resolution MCAL data acquisition, from T1 = 2022-01-01 05:11:21.50 +/- 0.01 s (UTC) to T2 = 2022-01-01 05:11:55.74 +/- 0.01 s (UTC), and released 10934 counts in the detector, above a background rate of 570 Hz. The time-integrated spectrum of the burst in this time interval can be fitted in the energy range 0.4-100 MeV with a power-law with ph. ind. = -1.28 (-0.11/+0.11), resulting in a reduced chi-squared of 0.96 (84 d.o.f.) and a fluence of 7.8e-05 ergs/cm^2 (90% confidence level), in the same energy range. We divided the data acquisition into four time intervals: interval a [T0-0.5 s, T0+10.0 s], interval b [T0+10.0 s, T0+17.0 s], interval c [T0+17.0 s, T0+24.0 s], and interval d [T0+24.0 s, T0+34.0 s]. The spectral analysis in the energy range 0.4-100 MeV shows an evolution of the power law photon index in the first stages of the burst, passing from -0.98 to -1.70. Details are reported below: | model | ph. ind. | red chi^2 (dof) | fluence (erg/cm^2) a | PL | 0.98 (-0.29/+0.19) | 1.00 (75) | (1.8+/-0.2)e-5 b | PL | 0.93 (-0.40/+0.22) | 0.98 (75) | (8.3+/-0.8)e-6 c | PL | 1.31 (-0.22/+0.23) | 0.99 (75) | (1.1+/-0.1)e-5 d | PL | 1.70 (-0.13/+0.29) | 1.05 (75) | (2.6+/-0.3)e-5 The MCAL light curve can be found at: http://www.agilescienceapp.it/notices/GRB220101A_AGILE_MCAL.png . Additional analysis of AGILE data is in progress. Automatic MCAL GRB alert Notices can be found at: https://gcn.gsfc.nasa.gov/agile_mcal.html....
2022
- GRB 220210A: AGILE detection
[Altro]
Ursi, A.; Foffano, L.; Verrecchia, F.; Pittori, C.; Tavani, M.; Argan, A.; Cardillo, M.; Casentini, C.; Evangelista, Y.; Menegoni, E.; Piano, G.; Lucarelli, F.; Bulgarelli, A.; di Piano, A.; Fioretti, V.; Fuschino, F.; Parmiggiani, N.; Marisaldi, M.; Pilia, M.; Trois, A.; Donnarumma, I.; Giuliani, A.; Longo, F.; Tempesta, P.; Agile, Team
abstract
The AGILE satellite detected the long GRB 220210A at T0 = 2022-02-10 23:56:38.40 +/- 0.01 s (UTC), reported by Fermi GBM (GCN #31568) and Swift BAT (GCN #31572). The burst is clearly visible in the AGILE scientific ratemeters of the MiniCALorimeter (MCAL; 0.4-100 MeV) and AntiCoincidence (AC; 50-200 keV) detectors. The event lasted about 1.9 s (in the 0.4-100 MeV energy range) and it released a total number of 2110 counts in the MCAL detector (above a background rate of 1230 Hz), and 3910 counts in the AC detector (above a background rate of 3330 Hz). The AGILE ratemeters light curves can be found at http://www.agilescienceapp.it/notices/GRB220210A_AGILE_RM.png . The event also triggered a high time resolution MCAL data acquisition, which released 1440 counts in the detector, above a background rate of 505 Hz. The time-integrated spectrum of the burst, from T0-0.19 s to T0+1.73 s, can be fitted in the energy range 0.4-5 MeV with a power-law with ph.ind. = -1.81 (-0.19,+0.21), resulting in a reduced chi-squared of 1.18 (36 d.o.f.) and a fluence of 3.40e-06 erg/cm^2 (90% confidence level), in the same energy range. The MCAL light curve can be found at http://www.agilescienceapp.it/notices/076950_GRB_571622198.404213.png . At the T0, the event was 15 deg off-axis. Additional analysis of AGILE data is in progress. Automatic MCAL GRB alert Notices can be found at: https://gcn.gsfc.nasa.gov/agile_mcal.html....
2022
- GRB 220308A: AGILE detection
[Altro]
Ursi, A.; di Piano, A.; Verrecchia, F.; Pittori, C.; Tavani, M.; Argan, A.; Cardillo, M.; Casentini, C.; Evangelista, Y.; Menegoni, E.; Foffano, L.; Piano, G.; Lucarelli, F.; Bulgarelli, A.; Fioretti, V.; Fuschino, F.; Parmiggiani, N.; Marisaldi, M.; Pilia, M.; Trois, A.; Donnarumma, I.; Longo, F.; Giuliani, A.; Agile, Team
abstract
The AGILE satellite detected the long GRB 220308A at T0 = 2022-03-08 05:43:08 (UTC), reported by INTEGRAL/SPI-ACS (trig#9761), Fermi/GBM (trigger no.668410535), CALET (GCN #31719), Konus-Wind (GCN #31723), Swift/BAT (GCN #31716), and triangulated by IPN (GCN #31715). The burst is clearly visible in the AGILE scientific ratemeters of the SuperAGILE (SA; 20-60 keV), MiniCALorimeter (MCAL; 0.4-100 MeV), and AntiCoincidence (AC; 50-200 keV) detectors. The event lasted about 22 s and it released a total number of 2675 counts in the SA detector (above a background rate of 140 Hz), 54840 counts in the MCAL detector (above a background rate of 1300 Hz), and 104080 counts in the AC detector (above a background rate of 3680 Hz). The AGILE ratemeter light curves can be found at https://tools.ssdc.asi.it/ImgView/Agile/GRB220308A_AGILE_RM29 . The event also triggered a partial high time resolution MCAL data acquisition, covering the main peaks of the burst, from T1 = 2022-03-08 05:43:08.21 s +/- 0.01 (UTC) to T2 = 2022-03-08 05:43:15.06 +/- 0.01 s (UTC), and released 16490 counts in the detector, above a background rate of 640 Hz. The time-integrated spectrum of the burst in this time interval can be fitted in the energy range 0.4-10 MeV with a Band function with alpha = -0.70 (frozen), beta = -2.45 (-0.11/+0.09), and Epeak = 760 (-98/+97) keV, resulting in a reduced chi-squared of 1.32 (47 d.o.f.) and a fluence of 1.4e-04 ergs/cm^2 (90% confidence level), in the same energy range. The MCAL light curve can be found at: https://tools.ssdc.asi.it/ImgView/Agile/077313_GRB_57380300810779330 . Additional analysis of AGILE data is in progress. Automatic MCAL GRB alert Notices can be found at: https://gcn.gsfc.nasa.gov/agile_mcal.html. Please note that in the MCAL notice #573803008 the T0 was affected by a temporal shift of about +19.5 sec due to episodic automated ground software problems which are then corrected offline....
2022
- GRB 220323A: AGILE/MCAL detection
[Altro]
Ursi, A.; Romani, M.; Pittori, C.; Verrecchia, F.; Tavani, M.; Argan, A.; Cardillo, M.; Casentini, C.; Evangelista, Y.; Foffano, L.; Menegoni, E.; Piano, G.; Lucarelli, F.; Bulgarelli, A.; di Piano, A.; Fioretti, V.; Fuschino, F.; Parmiggiani, N.; Marisaldi, M.; Pilia, M.; Trois, A.; Donnarumma, I.; Giuliani, A.; Longo, F.; Tempesta, P.; Agile, Team
abstract
The AGILE MiniCALorimeter (MCAL; 0.4-100 MeV) detected the short GRB 220323A at T0 = 2022-03-23 12:10:11 (UTC), reported by Fermi GBM (GCN #31782). The event lasted 0.35 s and released 295 counts in the detector, above a background rate of 630 Hz. The time-integrated spectrum of the burst can be fitted in the energy range 0.4-2 MeV with a power-law with ph.ind = 2.44 (-0.70/+1.13), resulting in a reduced chi-squared of 1.06 (21 d.o.f.) and a fluence of 5.6e-07 erg/cm^2 (90% confidence level), in the same energy range. The MCAL light curve can be found at http://www.agilescienceapp.it/notices/077531_GRB_575122215.552228.png . At the T0, the event was 134 deg off-axis. The burst is also clearly visible in the AGILE scientific ratemeters of the MCAL detector, where it released a total number of 1550 counts (above a background rate of 1230 Hz). The AGILE MCAL ratemeters light curve can be found at http://www.agilescienceapp.it/notices/GRB220323A_AGILE_RM.png . Additional analysis of AGILE data is in progress. Automatic MCAL GRB alert Notices can be found at: https://gcn.gsfc.nasa.gov/agile_mcal.html. Please note that in the MCAL notice #575122215 the T0 was affected by a temporal shift of about +4.0 sec due to episodic automated ground software problems which are then corrected offline....
2022
- GRB 220423A: AGILE detection
[Altro]
Ursi, A.; Pittori, C.; Verrecchia, F.; Tavani, M.; Argan, A.; Cardillo, M.; Casentini, C.; Evangelista, Y.; Menegoni, E.; Foffano, L.; Piano, G.; Lucarelli, F.; Bulgarelli, A.; di Piano, A.; Fioretti, V.; Fuschino, F.; Parmiggiani, N.; Marisaldi, M.; Pilia, M.; Trois, A.; Donnarumma, I.; Longo, F.; Giuliani, A.; Agile, Team
abstract
On its 15th birthday, the AGILE satellite detected the long GRB 220423A at T0 = 2022-04-23 14:14:14 (UTC), reported by INTEGRAL SPI-ACS (trigger no.9870) and CALET (trigger no.1334758343). The burst is clearly visible in the AGILE scientific ratemeters of the SuperAGILE (SA; 20-60 keV), MiniCALorimeter (MCAL; 0.4-100 MeV), and AntiCoincidence (AC; 50-200 keV) detectors. The event lasted about 20 s and it released a total number of 2150 counts in the SA detector (above a background rate of 75 Hz), 36645 counts in the MCAL detector (above a background rate of 1410 Hz), and 81820 counts in the AC detector (above a background rate of 3220 Hz). The AGILE ratemeter light curves can be found at http://www.agilescienceapp.it/notices/GRB220423A_AGILE_RM.png . Additional analysis of AGILE data is in progress. Automatic MCAL GRB alert Notices can be found at: https://gcn.gsfc.nasa.gov/agile_mcal.html....
2022
- GRB 220430A: AGILE detection
[Altro]
Ursi, A.; Longo, F.; Verrecchia, F.; Pittori, C.; Tavani, M.; Argan, A.; Cardillo, M.; Casentini, C.; Evangelista, Y.; Menegoni, E.; Foffano, L.; Piano, G.; Lucarelli, F.; Bulgarelli, A.; di Piano, A.; Fioretti, V.; Fuschino, F.; Parmiggiani, N.; Marisaldi, M.; Pilia, M.; Trois, A.; Donnarumma, I.; Giuliani, A.; Agile, Team
abstract
The AGILE satellite detected the GRB 220430A at T0 = 2022-04-30 13:53:45 (UTC), reported by Swift (GCNs #31972 and #31973). The burst is clearly visible in the AGILE scientific ratemeters of the SuperAGILE detector (SA; 20-60 keV) and in all the five panels of the AntiCoincidence system (AC Top, 50-200 keV; AC Lat, 80-200 keV). The event lasted about 25 s and it released a total number of 2685 counts in the SA detector (above a background rate of 100 Hz), 25765 counts in the MCAL detector (above a background rate of 1070 Hz), and 67565 counts in the AC detector (above a background rate of 2710 Hz). The AGILE ratemeter light curves can be found at http://www.agilescienceapp.it/notices/GRB220430A_AGILE_RM.png . As pointed out in GCN #31972, we notice that this event took place at the end of an intense X-class solar flare, clearly detected by all the AGILE scientific ratemeters. A more extended light curve can be found at http://www.agilescienceapp.it/notices/GRB220430A_AGILE_RM_all.png . Additional analysis of AGILE data is in progress. Automatic MCAL GRB alert Notices can be found at: https://gcn.gsfc.nasa.gov/agile_mcal.html....
2022
- GRB 220511A: AGILE detection
[Altro]
Ursi, A.; Pittori, C.; Verrecchia, F.; Tavani, M.; Argan, A.; Cardillo, M.; Casentini, C.; Evangelista, Y.; Menegoni, E.; Foffano, L.; Piano, G.; Lucarelli, F.; Bulgarelli, A.; di Piano, A.; Fioretti, V.; Fuschino, F.; Panebianco, G.; Parmiggiani, N.; Marisaldi, M.; Pilia, M.; Trois, A.; Romani, M.; Donnarumma, I.; Longo, F.; Giuliani, A.; Agile, Team
abstract
The AGILE satellite detected the GRB 220511A at T0 = 2022-05-11 13:41:57 (UTC), reported by Fermi GBM (GCN #32018). The burst is clearly visible in the AGILE scientific ratemeters of the SuperAGILE (SA; 20-60 keV), MiniCALorimeter (MCAL; 0.4-100 MeV), and AntiCoincidence (AC; 50-200 keV) detectors. The event lasted about 3 s and it released a total number of 410 counts in the SA detector (above a background rate of 70 Hz), 5150 counts in the MCAL detector (above a background rate of 1230 Hz), and 15270 counts in the AC detector (above a background rate of 3500 Hz). The AGILE ratemeter light curves can be found at http://www.agilescienceapp.it/notices/GRB220511A_AGILE_RM.png . Additional analysis of AGILE data is in progress. Automatic MCAL GRB alert Notices can be found at: https://gcn.gsfc.nasa.gov/agile_mcal.html....
2022
- GRB 220527A: AGILE detection
[Altro]
Ursi, A.; Piano, G.; Pittori, C.; Verrecchia, F.; Tavani, M.; Argan, A.; Cardillo, M.; Casentini, C.; Evangelista, Y.; Foffano, L.; Menegoni, E.; Lucarelli, F.; Bulgarelli, A.; di Piano, A.; Fioretti, V.; Fuschino, F.; Parmiggiani, N.; Romani, M.; Marisaldi, M.; Pilia, M.; Trois, A.; Donnarumma, I.; Giuliani, A.; Longo, F.; Tempesta, P.; Agile, Team
abstract
The AGILE satellite detected a burst at T0 = 2022-05-27 09:17:14.55 (UTC). The event is clearly visible in the AGILE scientific ratemeters of the SuperAGILE (SA; 20-60 keV), MiniCALorimeter (MCAL; 0.4-100 MeV), and AntiCoincidence (AC; 50-200 keV) detectors. The event lasted about 15 s and it released a total number of 1860 counts in the SA detector (above a background rate of 50 Hz), 27875 counts in the MCAL detector (above a background rate of 1380 Hz), and 67515 counts in the AC detector (above a background rate of 3470 Hz). The AGILE ratemeter light curves can be found at http://www.agilescienceapp.it/notices/GRB220527A_AGILE_RM.png . The event also triggered a high time resolution MCAL data acquisition, where it released 12500 counts in the detector, above a background rate of 630 Hz. We notice that most of the signal is revealed in the E < 1.4 MeV energy channels. The MCAL light curve can be found at http://www.agilescienceapp.it/notices/GRB220527A_078464_580727834.552109.png . Additional analysis of AGILE data is in progress. Automatic MCAL GRB alert Notices can be found at: https://gcn.gsfc.nasa.gov/agile_mcal.html....
2022
- GRB 220623A: AGILE detection
[Altro]
Ursi, A.; Lucarelli, F.; Pittori, C.; Verrecchia, F.; Tavani, M.; Argan, A.; Cardillo, M.; Casentini, C.; Evangelista, Y.; Foffano, L.; Menegoni, E.; Piano, G.; Bulgarelli, A.; di Piano, A.; Fioretti, V.; Parmiggiani, N.; Romani, M.; Marisaldi, M.; Pilia, M.; Trois, A.; Donnarumma, I.; Giuliani, A.; Longo, F.; Tempesta, P.; Agile, Team
abstract
The AGILE satellite detected the GRB 220623A at T0 = 2022-06-23 07:04:14 s (UTC), reported by Swift BAT (GCN #32243), Swift XRT (GCN #32244), and AstroSat CZTI (GCN #32246). The burst is clearly visible in the AGILE scientific ratemeters of the SuperAGILE (SA; 20-60 keV), MiniCALorimeter (MCAL; 0.4-100 MeV), and AntiCoincidence (AC; 50-200 keV) detectors. The event lasted about 25 s and it released a total number of 1950 counts in the SA detector (above a background rate of 85 Hz), 29800 counts in the MCAL detector (above a background rate of 1050 Hz), and 72370 counts in the AC detector (above a background rate of 2770 Hz). The AGILE ratemeters light curves can be found at http://www.agilescienceapp.it/notices/GRB220623A_AGILE_RM.png . The event also triggered a partial high time resolution MCAL data acquisition, from T1 = 2022-06-23 07:04:09.65 s (UTC) to T2 = 2022-06-23 07:04:16.15 s (UTC), and released 4200 counts in the detector, above a background rate of 530 Hz. The MCAL light curve can be found at http://www.agilescienceapp.it/notices/GRB220623A_078852_583052654.000000.png . At the T0, the event was 35 deg off-axis. Additional analysis of AGILE data is in progress. Automatic MCAL GRB alert Notices can be found at: https://gcn.gsfc.nasa.gov/agile_mcal.html....
2022
- GRB 220624A: AGILE detection
[Altro]
Ursi, A.; Foffano, L.; Tavani, M.; Argan, A.; Cardillo, M.; Casentini, C.; Evangelista, Y.; Menegoni, E.; Piano, G.; Bulgarelli, A.; di Piano, A.; Fioretti, V.; Parmiggiani, N.; Lucarelli, F.; Pittori, C.; Verrecchia, F.; Romani, M.; Marisaldi, M.; Pilia, M.; Trois, A.; Donnarumma, I.; Giuliani, A.; Longo, F.; Tempesta, P.; Agile, Team
abstract
The AGILE satellite detected the long GRB 220624A at T0 = 2022-06-24 02:58:35 s (UTC), reported by Fermi GBM (GCN #32256). The burst is clearly visible in the AGILE scientific ratemeters of the SuperAGILE (SA; 20-60 keV), MiniCALorimeter (MCAL; 0.4-100 MeV), and AntiCoincidence (AC; 50-200 keV) detectors. The event lasted about 57 s and it released a total number of 5930 counts in the SA detector (above a background rate of 70 Hz), 88920 counts in the MCAL detector (above a background rate of 1135 Hz), and 212145 counts in the AC detector (above a background rate of 3195 Hz). The AGILE ratemeters light curves can be found at http://www.agilescienceapp.it/notices/GRB220624A_AGILE_RM.png . The event also triggered a partial high time resolution MCAL data acquisition, from T1 = 2022-06-24 02:58:34.36 s (UTC) to T2 = 2022-06-24 02:58:46.85 s (UTC), and released 14630 counts in the detector, above a background rate of 620 Hz. The MCAL light curve can be found at http://www.agilescienceapp.it/notices/GRB220624A_078864_583124315.000000.png . At the T0, the event was 20 deg off-axis. Additional analysis of AGILE data is in progress. Automatic MCAL GRB alert Notices can be found at: https://gcn.gsfc.nasa.gov/agile_mcal.html....
2022
- GRB 220624A: AGILE/GRID analysis
[Altro]
Verrecchia, F.; Ursi, A.; Giuliani, A.; Foffano, L.; Tavani, M.; Longo, F.; Lucarelli, F.; Pittori, C.; Piano, G.; Argan, A.; Cardillo, M.; Evangelista, Y.; Menegoni, E.; Bulgarelli, A.; di Piano, A.; Fioretti, V.; Parmiggiani, N.; Marisaldi, M.; Pilia, M.; Trois, A.; Donnarumma, I.; Agile, Team
abstract
The Gamma-Ray Imaging Detector (GRID) of AGILE detected a gamma-ray transient temporally coincident with the long GRB 220624A reported by Fermi/GBM in GCN #32256 and also detected by AGILE/MCAL (Ursi et al., GCN #32259). A preliminary GRID analysis shows a detection with a statistical significance above 8 sigma over a time integration of 20 s starting from the T0 of GRB 220624A, at a sky position compatible with that of the GRB. We obtained 25 events 2 of which are notably above 1 GeV. The GRB position was about 30 deg off-axis from the GRID boresight. These measurements were obtained with AGILE observing a large portion of the sky in spinning mode. Additional analysis of AGILE data is in progress....
2022
- GRB 220624A: AGILE/GRID refined analysis
[Altro]
Verrecchia, F.; Ursi, A.; Giuliani, A.; Tavani, M.; Longo, F.; Foffano, L.; Lucarelli, F.; Pittori, C.; Romani, M.; Piano, G.; Argan, A.; Cardillo, M.; Casentini, C.; Evangelista, Y.; Menegoni, E.; Bulgarelli, A.; di Piano, A.; Fioretti, V.; Parmiggiani, N.; Marisaldi, M.; Pilia, M.; Trois, A.; Donnarumma, I.; Tempesta, P.; Agile, Team
abstract
he Gamma-Ray Imaging Detector (GRID) of AGILE detected gamma-ray transient emission consistent with the long GRB 220624A reported by Fermi/GBM (GCN #32256) at T0 = 2022-06-24 02:58:35 s (UTC), as already reported in Verrecchia et al. GCN #32261 and also detected by AGILE/MCAL (Ursi et al., GCN #32259). An improved localization has been reported by the IPN (Kozyrev et al. GCN #32265). A refined AGILE/GRID data analysis in the energy range 30 MeV - 10 GeV shows a detection with a statistical significance of about 10 sigma over a time integration of 20 s starting at the T0 of GRB 220624A, at a sky position with Galactic coordinates l=260 deg, b=75 deg, with and an error radius of 4 deg (90% statistical + systematic error; R.A.= 182 deg, Decl.= 16 deg (J2000)). The GRID error circle includes the IPN position. During the 20s integration, we detected a total of 41 events (3 background events estimated through a Li&Ma analysis), 17 events at energies above 100 MeV, and 3 events above 1 GeV. These measurements were obtained with AGILE observing a large portion of the sky in spinning mode. Additional analysis of AGILE data is in progress....
2022
- GRB 220910A: AGILE detection
[Altro]
Ursi, A.; Menegoni, E.; Tavani, M.; Argan, A.; Cardillo, M.; Casentini, C.; Evangelista, Y.; Foffano, L.; Piano, G.; Bulgarelli, A.; di Piano, A.; Fioretti, V.; Panebianco, G.; Parmiggiani, N.; Lucarelli, F.; Pittori, C.; Verrecchia, F.; Romani, M.; Marisaldi, M.; Pilia, M.; Trois, A.; Donnarumma, I.; Giuliani, A.; Longo, F.; Tempesta, P.
abstract
...
2022
- Gamma-ray rebrightening of the blazar PKS 1424-418 detected by AGILE
[Altro]
Verrecchia, F.; Piano, G.; Pittori, C.; Tavani, M.; Panebianco, G.; Bulgarelli, A.; Fioretti, V.; Parmiggiani, N.; Addis, A.; Baroncelli, L.; Di Piano, A.; Lucarelli, F.; Vercellone, S.; Cardillo, M.; Ursi, A.; Casentini, C.; Donnarumma, I.; Gianotti, F.; Trifoglio, M.; Giuliani, A.; Mereghetti, S.; Caraveo, P.; Perotti, F.; Chen, A.; Argan, A.; Costa, E.; Del Monte, E.; Evangelista, Y.; Feroci, M.; Foffano, L.; Lapshov, I.; Menegoni, E.; Pacciani, L.; Soffitta, P.; Vittorini, V.; Lazzarotto, F.; Di Cocco, G.; Fuschino, F.; Galli, M.; Labanti, C.; Marisaldi, M.; Pellizzoni, A.; Pilia, M.; Trois, A.; Barbiellini, G.; Longo, F.; Vallazza, E.; Morselli, A.; Picozza, P.; Prest, M.; Lipari, P.; Zanello, D.; Cattaneo, P. W.; Rappoldi, A.; Ferrari, A.; Paoletti, F.; Antonelli, L. A.; Giommi, P.; Salotti, L.; Valentini, G.; D'Amico, F.
abstract
The GRID gamma-ray detector onboard the AGILE satellite is detecting a gamma-ray rebrightening from the blazar PKS 1424-418 (z = 1.522, White et al., ApJ 327 561, 1988) already reported to be in an optical and GeV flaring activity period, either by Fermi/LAT (ATel #15525) and by AGILE/GRID (#15527)....
2022
- Multi-wavelength study of the galactic PeVatron candidate LHAASO J2108+5157
[Articolo su rivista]
Abe, S.; Aguasca-Cabot, A.; Agudo, I.; Alvarez Crespo, N.; Antonelli, L. A.; Aramo, C.; Arbet-Engels, A.; Artero, M.; Asano, K.; Aubert, P.; Baktash, A.; Bamba, A.; Baquero Larriva, A.; Baroncelli, L.; Barres de Almeida, U.; Barrio, J. A.; Batkovic, I.; Baxter, J.; Becerra González, J.; Bernardini, E.; Bernardos, M. I.; Bernete Medrano, J.; Berti, A.; Bhattacharjee, P.; Biederbeck, N.; Bigongiari, C.; Bissaldi, E.; Blanch, O.; Bordas, P.; Buisson, C.; Bulgarelli, A.; Burelli, I.; Buscemi, M.; Cardillo, M.; Caroff, S.; Carosi, A.; Cassol, F.; Cauz, D.; Ceribella, G.; Chai, Y.; Cheng, K.; Chiavassa, A.; Chikawa, M.; Chytka, L.; Cifuentes, A.; Contreras, J. L.; Cortina, J.; Costantini, H.; D'Amico, G.; Dalchenko, M.; De Angelis, A.; de Bony de Lavergne, M.; De Lotto, B.; de Menezes, R.; Deleglise, G.; Delgado, C.; Delgado Mengual, J.; della Volpe, D.; Dellaiera, M.; Di Piano, A.; Di Pierro, F.; Di Tria, R.; Di Venere, L.; Díaz, C.; Dominik, R. M.; Dominis Prester, D.; Donini, A.; Dorner, D.; Doro, M.; Elsässer, D.; Emery, G.; Escudero, J.; Fallah Ramazani, V.; Ferrara, G.; Fiasson, A.; Freixas Coromina, L.; Fröse, S.; Fukami, S.; Fukazawa, Y.; Garcia, E.; Garcia López, R.; Gasparrini, D.; Geyer, D.; Giesbrecht Paiva, J.; Giglietto, N.; Giordano, F.; Giro, E.; Gliwny, P.; Godinovic, N.; Grau, R.; Green, D.; Green, J.; Gunji, S.; Hackfeld, J.; Hadasch, D.; Hahn, A.; Hashiyama, K.; Hassan, T.; Hayashi, K.; Heckmann, L.; Heller, M.; Herrera Llorente, J.; Hirotani, K.; Hoffmann, D.; Horns, D.; Houles, J.; Hrabovsky, M.; Hrupec, D.; Hui, D.; Hütten, M.; Imazawa, R.; Inada, T.; Inome, Y.; Ioka, K.; Iori, M.; Ishio, K.; Iwamura, Y.; Jacquemont, M.; Jimenez Martinez, I.; Jurysek, J.; Kagaya, M.; Karas, V.; Katagiri, H.; Kataoka, J.; Kerszberg, D.; Kobayashi, Y.; Kong, A.; Kubo, H.; Kushida, J.; Lainez, M.; Lamanna, G.; Lamastra, A.; Le Flour, T.; Linhoff, M.; Longo, F.; López-Coto, R.; López-Moya, M.; López-Oramas, A.; Loporchio, S.; Lorini, A.; Luque-Escamilla, P. L.; Majumdar, P.; Makariev, M.; Mandat, D.; Manganaro, M.; Manicò, G.; Mannheim, K.; Mariotti, M.; Marquez, P.; Marsella, G.; Martí, J.; Martinez, O.; Martínez, G.; Martínez, M.; Marusevec, P.; Mas-Aguilar, A.; Maurin, G.; Mazin, D.; Mestre Guillen, E.; Micanovic, S.; Miceli, D.; Miener, T.; Miranda, J. M.; Mirzoyan, R.; Mizuno, T.; Molero Gonzalez, M.; Molina, E.; Montaruli, T.; Monteiro, I.; Moralejo, A.; Morcuende, D.; Morselli, A.; Mrakovcic, K.; Murase, K.; Nagai, A.; Nakamori, T.; Nickel, L.; Nievas, M.; Nishijima, K.; Noda, K.; Nosek, D.; Nozaki, S.; Ohishi, M.; Ohtani, Y.; Okazaki, N.; Okumura, A.; Orito, R.; Otero-Santos, J.; Palatiello, M.; Paneque, D.; Pantaleo, F. R.; Paoletti, R.; Paredes, J. M.; Pavletić, L.; Pech, M.; Pecimotika, M.; Pietropaolo, E.; Pirola, G.; Podobnik, F.; Poireau, V.; Polo, M.; Pons, E.; Prandini, E.; Prast, J.; Priyadarshi, C.; Prouza, M.; Rando, R.; Rhode, W.; Ribó, M.; Rizi, V.; Rodriguez Fernandez, G.; Saito, T.; Sakurai, S.; Sanchez, D. A.; Šarić, T.; Saturni, F. G.; Scherpenberg, J.; Schleicher, B.; Schmuckermaier, F.; Schubert, J. L.; Schussler, F.; Schweizer, T.; Seglar Arroyo, M.; Sitarek, J.; Sliusar, V.; Spolon, A.; Strišković, J.; Strzys, M.; Suda, Y.; Sunada, Y.; Tajima, H.; Takahashi, M.; Takahashi, H.; Takata, J.; Takeishi, R.; Tam, P. H. T.; Tanaka, S. J.; Tateishi, D.; Temnikov, P.; Terada, Y.; Terauchi, K.; Terzic, T.; Teshima, M.; Tluczykont, M.; Tokanai, F.; Torres, D. F.; Travnicek, P.; Truzzi, S.; Tutone, A.; Uhlrich, G.; Vacula, M.; Vázquez Acosta, M.; Verguilov, V.; Viale, I.; Vigliano, A.; Vigorito, C. F.; Vitale, V.; Voutsinas, G.; Vovk, I.; Vuillaume, T.; Walter, R.; Will, M.; Yamamoto, T.; Yamazaki, R.; Yoshida, T.; Yoshikoshi, T.; Zywucka, N.; Balbo, M.; Eckert, D.; Tramacere, A.
abstract
LHAASO J2108+5157 is one of the few known unidentified Ultra-High-Energy (UHE) gamma-ray sources with no Very-High-Energy (VHE) counterpart, recently discovered by the LHAASO collaboration. We observed LHAASO J2108+5157 in the X-ray band with XMM-Newton in 2021 for a total of 3.8 hours and at TeV energies with the Large-Sized Telescope prototype (LST-1), yielding 49 hours of good quality data. In addition, we analyzed 12 years of Fermi-LAT data, to better constrain emission of its High-Energy (HE) counterpart 4FGL J2108.0+5155. We found an excess (3.7 sigma) in the LST-1 data at energies E > 3 TeV. Further analysis in the whole LST-1 energy range assuming a point-like source, resulted in a hint (2.2 sigma) of hard emission which can be described with a single power law with photon index Gamma = 1.6 +- 0.2 between 0.3 - 100 TeV. We did not find any significant extended emission which could be related to a Supernova Remnant (SNR) or Pulsar Wind Nebula (PWN) in the XMM-Newton data, which puts strong constraints on possible synchrotron emission of relativistic electrons. The LST-1 and LHAASO observations can be explained as inverse Compton dominated leptonic emission of relativistic electrons with cutoff energy of 100+70-30 TeV. The low magnetic field in the source imposed by the X-ray upper limits on synchrotron emission is compatible with a hypothesis of a TeV halo. Furthermore, the spectral properties of the HE counterpart are consistent with a hypothesis of Geminga-like pulsar, which would be able to power the VHE-UHE emission. LST-1 and Fermi-LAT upper limits impose strong constraints on hadronic scenario of pi-0 decay dominated emission from accelerated protons interacting with nearby molecular clouds, requiring hard spectral index, which is incompatible with the standard diffusive acceleration scenario....
2022
- On the AGILE detection of GRB 220423A
[Altro]
Tavani, M.; Argan, A.; Cardillo, M.; Casentini, C.; Evangelista, Y.; Menegoni, E.; Foffano, L.; Piano, G.; Ursi, A.; Lucarelli, F.; Pittori, C.; Verrecchia, F.; Bulgarelli, A.; di Piano, A.; Fioretti, V.; Fuschino, F.; Parmiggiani, N.; Marisaldi, M.; Pilia, M.; Trois, A.; Donnarumma, I.; Longo, F.; Giuliani, A.; Agile, Team
abstract
We notice that today, April 23rd 2022, is the 15th anniversary of the AGILE launch. The AGILE instrument has been flawlessly operating in orbit since then. We take this opportunity to thank ASI, the AGILE scientific Team and the ground segment team for their committment to the excellence of the mission....
2022
- Ongoing gamma-ray flare from the blazar PKS 1424-418 detected by AGILE
[Altro]
Piano, G.; Panebianco, G.; Pittori, C.; Verrecchia, F.; Tavani, M.; Bulgarelli, A.; Fioretti, V.; Parmiggiani, N.; Addis, A.; Baroncelli, L.; Di Piano, A.; Lucarelli, F.; Vercellone, S.; Cardillo, M.; Ursi, A.; Casentini, C.; Donnarumma, I.; Gianotti, F.; Trifoglio, M.; Giuliani, A.; Mereghetti, S.; Caraveo, P.; Perotti, F.; Chen, A.; Argan, A.; Costa, E.; Del Monte, E.; Evangelista, Y.; Feroci, M.; Foffano, L.; Lapshov, I.; Menegoni, E.; Pacciani, L.; Soffitta, P.; Vittorini, V.; Lazzarotto, F.; Di Cocco, G.; Fuschino, F.; Galli, M.; Labanti, C.; Marisaldi, M.; Pellizzoni, A.; Pilia, M.; Trois, A.; Barbiellini, G.; Longo, F.; Vallazza, E.; Morselli, A.; Picozza, P.; Prest, M.; Lipari, P.; Zanello, D.; Cattaneo, P. W.; Rappoldi, A.; Ferrari, A.; Paoletti, F.; Antonelli, L. A.; Giommi, P.; Salotti, L.; Valentini, G.; D'Amico, F.
abstract
The GRID detector onboard the AGILE satellite is detecting a bright gamma-ray flare from the blazar PKS 1424-418 (z = 1.522, White et al., ApJ 327 561, 1988)....
2022
- Prospects for Galactic transient sources detection with the Cherenkov Telescope Array
[Relazione in Atti di Convegno]
López-Oramas, A.; Bulgarelli, A.; Chaty, S.; Chernyakova, M.; Gnatyk, R.; Hnatyk, B.; Kantzas, D.; Markoff, S.; Mckeague, S.; Mereghetti, S.; Mestre, E.; di Piano, A.; Romano, P.; Sadeh, I.; Sergijenko, O.; Sidoli, L.; Spolon, A.; de Oña Wilhelmi, E.; Piano, G.; L., Zampieri
abstract
Several types of Galactic sources, like magnetars, microquasars, novae or pulsar wind nebulae flares, display transient emission in the X-ray band. Some of these sources have also shown emission at MeV--GeV energies. However, none of these Galactic transients have ever been detected in the very-high-energy (VHE; E$>$100 GeV) regime by any Imaging Air Cherenkov Telescope (IACT). The Galactic Transient task force is a part of the Transient Working group of the Cherenkov Telescope Array (CTA) Consortium. The task force investigates the prospects of detecting the VHE counterpart of such sources, as well as their study following Target of Opportunity (ToO) observations. In this contribution, we will show some of the results of exploring the capabilities of CTA to detect and observe Galactic transients; we assume different array configurations and observing strategies....
2022
- Searching for very-high-energy electromagnetic counterparts to gravitational-wave events with the Cherenkov Telescope Array
[Relazione in Atti di Convegno]
Patricelli, Barbara; Carosi, Alessandro; Nava, Lara; Seglar-Arroyo, Monica; Schüssler, Fabian; Stamerra, Antonio; Adelfio, Andrea; Ashkar, Halim; Bulgarelli, Andrea; Di Girolamo, Tristano; Di Piano, Ambra; Gasparetto, Thomas; Green, Jarred; Longo, Francesco; Agudo, Ivan; Berti, Alessio; Bissaldi, Elisabetta; Cella, Giancarlo; Circiello, Antonio; Covino, Stefano; Ghirlanda, Giancarlo; Humensky, Brian; Inoue, Susumu; Lefaucheur, Julien; Filipovic, Miroslav; Razzano, Massimiliano; Ribeiro, Deivid; Sergijenko, Olga; Stratta, Giulia; Vergani, Susanna
abstract
The detection of electromagnetic (EM) emission following the gravitational wave (GW) event GW170817 opened the era of multi-messenger astronomy with GWs and provided the first direct evidence that at least a fraction of binary neutron star (BNS) mergers are progenitors of short Gamma-Ray Bursts (GRBs). GRBs are also expected to emit very-high energy (VHE, > 100 GeV) photons, as proven by the recent MAGIC and H.E.S.S. observations. One of the challenges for future multi-messenger observations will be the detection of such VHE emission from GRBs in association with GWs. In the next years, the Cherenkov Telescope Array (CTA) will be a key instrument for the EM follow-up of GW events in the VHE range, owing to its unprecedented sensitivity, rapid response, and capability to monitor a large sky area via scan-mode operation. We present the CTA GW follow-up program, with a focus on the searches for short GRBs possibly associated with BNS mergers. We investigate the possible observational strategies and we outline the prospects for the detection of VHE EM counterparts to transient GW events....
2022
- Southern African Large Telescope Spectroscopy of BL Lacs for the CTA project
[Relazione in Atti di Convegno]
Abdalla, H.; Abe, H.; Abe, S.; Abusleme, A.; Acero, F.; Acharyya, A.; Acin Portella, V.; Ackley, K.; Adam, R.; Adams, C.; Adhikari, S. S.; Aguado-Ruesga, I.; Agudo, I.; Aguilera, R.; Aguirre-Santaella, A.; Aharonian, F.; Alberdi, A.; Alfaro, R.; Alfaro, J.; Alispach, C.; Aloisio, R.; Alves Batista, R.; Amans, J. -P.; Amati, L.; Amato, E.; Ambrogi, L.; Ambrosi, G.; Ambrosio, M.; Ammendola, R.; Anderson, J.; Anduze, M.; Anguner, E. O.; Antonelli, L. A.; Antonuccio, V.; Antoranz, P.; Anutarawiramkul, R.; Aragunde Gutierrez, J.; Aramo, C.; Araudo, A.; Araya, M.; Arbet-Engels, A.; Arcaro, C.; Arendt, V.; Armand, C.; Armstrong, T.; Arqueros, F.; Arrabito, L.; Arsioli, B.; Artero, M.; Asano, K.; Ascasibar, Y.; Aschersleben, J.; Ashley, M.; Attina, P.; Aubert, P.; Singh, C. B.; Baack, D.; Babic, A.; Backes, M.; Baena, V.; Bajtlik, S.; Baktash, A.; Balazs, C.; Balbo, M.; Ballester, O.; Ballet, J.; Balmaverde, B.; Bamba, A.; Bandiera, R.; Baquero Larriva, A.; Barai, P.; Barbier, C.; Barbosa Martins, V.; Barcelo, M.; Barkov, M.; Barnard, M.; Baroncelli, L.; Barres De Almeida, U.; Barrio, J. A.; Bastieri, D.; Batista, P. I.; Batkovic, I.; Bauer, C.; Bautista-Gonzalez, R.; Baxter, J.; Becciani, U.; Becerra Gonzalez, J.; Becherini, Y.; Beck, G.; Becker Tjus, J.; Bednarek, W.; Belfiore, A.; Bellizzi, L.; Belmont, R.; Benbow, W.; Berge, D.; Bernardini, E.; Bernardos, M. I.; Bernlohr, K.; Berti, A.; Berton, M.; Bertucci, B.; Beshley, V.; Bhatt, N.; Bhattacharyya, S.; Bhattacharyya, W.; Bhattacharyya, S.; Bi, B.; Bicknell, G.; Biederbeck, N.; Bigongiari, C.; Biland, A.; Bird, R.; Bissaldi, E.; Biteau, J.; Bitossi, M.; Blanch, O.; Blank, M.; Blazek, J.; Bobin, J.; Boccato, C.; Bocchino, F.; Boehm, C.; Bohacova, M.; Boisson, C.; Boix, J.; Bolle, J. -P.; Bolmont, J.; Bonanno, G.; Bonavolonta, C.; Bonneau Arbeletche, L.; Bonnoli, G.; Bordas, P.; Borkowski, J.; Borquez, S.; Bose, R.; Bose, D.; Bosnjak, Z.; Bottacini, E.; Bottcher, M.; Botticella, M. T.; Boutonnet, C.; Bouyjou, F.; Bozhilov, V.; Bozzo, E.; Brahimi, L.; Braiding, C.; Brau-Nogue, S.; Breen, S.; Bregeon, J.; Breuhaus, M.; Brill, A.; Brisken, W.; Brocato, E.; Brown, A. M.; Brugge, K.; Brun, P.; Brun, P.; Brun, F.; Brunetti, L.; Brunetti, G.; Bruno, P.; Bruno, A.; Bruzzese, A.; Bucciantini, N.; Buckley, J.; Buhler, R.; Bulgarelli, A.; Bulik, T.; Bunning, M.; Bunse, M.; Burton, M.; Burtovoi, A.; Buscemi, M.; Buschjager, S.; Busetto, G.; Buss, J.; Byrum, K.; Caccianiga, A.; Cadoux, F.; Calanducci, A.; Calderon, C.; Calvo Tovar, J.; Cameron, R.; Campana, P.; Canestrari, R.; Cangemi, F.; Cantlay, B.; Capalbi, M.; Capasso, M.; Cappi, M.; Caproni, A.; Capuzzo-Dolcetta, R.; Caraveo, V.; Cardenas, P.; Cardiel, L.; Cardillo, M.; Carlile, C.; Caroff, S.; Carosi, R.; Carosi, A.; Carquin, E.; Carrere, M.; Casandjian, J. -M.; Casanova, S.; Cascone, E.; Cassol, F.; Castro-Tirado, A. J.; Catalani, F.; Catalano, O.; Cauz, D.; Ceccanti, A.; Celestino Silva, C.; Celli, S.; Cerny, K.; Cerruti, M.; Chabanne, E.; Chadwick, P.; Chai, Y.; Chambery, P.; Champion, C.; Chandra, S.; Chaty, S.; Chen, A.; Cheng, K.; Chernyakova, M.; Chiaro, G.; Chiavassa, A.; Chikawa, M.; Chitnis, V. R.; Chudoba, J.; Chytka, L.; Cikota, S.; Circiello, A.; Clark, P.; Colak, M.; Colombo, E.; Colome, J.; Colonges, S.; Comastri, A.; Compagnino, A.; Conforti, V.; Congiu, E.; Coniglione, R.; Conrad, J.; Conte, F.; Contreras, J. L.; Coppi, P.; Cornat, R.; Coronado-Blazquez, J.; Cortina, J.; Costa, A.; Costantini, H.; Cotter, G.; Courty, B.; Covino, S.; Crestan, S.; Cristofari, P.; Crocker, R.; Croston, J.; Cubuk, K.; Cuevas, O.; Cui, X.; Cusumano, G.; Cutini, S.; D(')Ai, A.; D(')Amico, G.; D(')Ammando, F.; D(')Avanzo, P.; Da Vela, P.; Dadina, M.; Dai, S.; Dalchenko, M.; Dall(')Ora, M.; Daniel, M. K.; Dauguet, J.; Davids, I.; Davies, J.; Dawson, B.; De Angelis, A.; De Araujo Carvalho, A. E.; De Bony De Lavergne, M.; De Caprio, V.; De Cesare, G.; De Frondat, F.; De Gouveia Dal Pino, E. M.; De La Calle, I.; De Lotto, B.; De Luca, A.; De Martin
abstract
In the last two decades, very-high-energy gamma-ray astronomy has reached maturity: over 200 sources have been detected, both Galactic and extragalactic, by ground-based experiments. At present, Active Galactic Nuclei (AGN) make up about 40% of the more than 200 sources detected at very high energies with ground-based telescopes, the majority of which are blazars, i.e. their jets are closely aligned with the line of sight to Earth and three quarters of which are classified as high-frequency peaked BL Lac objects. One challenge to studies of the cosmological evolution of BL Lacs is the difficulty of obtaining redshifts from their nearly featureless, continuum-dominated spectra. It is expected that a significant fraction of the AGN to be detected with the future Cherenkov Telescope Array (CTA) observatory will have no spectroscopic redshifts, compromising the reliability of BL Lac population studies, particularly of their cosmic evolution. We started an effort in 2019 to measure the redshifts of a large fraction of the AGN that are likely to be detected with CTA, using the Southern African Large Telescope (SALT). In this contribution, we present two results from an on-going SALT program focused on the determination of BL Lac object redshifts that will be relevant for the CTA observatory.
2022
- The AGILE real-time analysis pipelines in the multi-messenger era
[Relazione in Atti di Convegno]
Parmiggiani, Nicolò; Bulgarelli, Andrea; Ursi, Alessandro; Fioretti, Valentina; Baroncelli, Leonardo; Addis, Antonio; Di Piano, Ambra; Pittori, Carlotta; Verrecchia, Francesco; Lucarelli, Fabrizio; Tavani, Marco; Beneventano, Domenico
abstract
In the multi-messenger era, space and ground-based observatories usually develop real-time analysis (RTA) pipelines to rapidly detect transient events and promptly share information with the scientific community to enable follow-up observations. These pipelines can also react to science alerts shared by other observatories through networks such as the Gamma-Ray Coordinates Network (GCN) and the Astronomer's Telegram (ATels). AGILE is a space mission launched in 2007 to study X-ray and gamma-ray phenomena. This contribution presents the technologies used to develop two types of AGILE pipelines using the RTApipe framework and an overview of the main scientific results. The first type performs automated analyses on new AGILE data to detect transient events and automatically sends AGILE notices to the GCN network. Since May 2019, this pipeline has sent more than 50 automated notices with a few minutes delay since data arrival. The second type of pipeline reacts to multi-messenger external alerts (neutrinos, gravitational waves, GRBs, and other transients) received through the GCN network and performs hundreds of analyses searching for counterparts in all AGILE instruments' data. The AGILE Team uses these pipelines to perform fast follow-up of science alerts reported by other facilities, which resulted in the publishing of several ATels and GCN circulars....
2022
- The Gamma-Flash real-time data pipeline for ground observation of terrestrial gamma-ray flashes
[Relazione in Atti di Convegno]
Addis, A.; Aboudan, A.; Bulgarelli, A.; Baroncelli, L.; Campana, R.; De Rosa, A.; Di Piano, A.; Fuschino, F.; Parmiggiani, N.; Ursi, A.; Virgilli, E.
abstract
Gamma-Flash is an Italian project funded by the Italian Space Agency (ASI) and led by the National Institute for Astrophysics (INAF), devoted to the observation and study of high-energy phenomena, such as terrestrial gamma-ray flashes and gamma-ray glows produced in Earth's atmosphere during thunderstorms. The project represents the ground-based supplement to the work of the ASI AGILE satellite in this particular field. This contribution presents the architecture of the Gamma-Flash data pipeline placed at the Osservatorio Climatico "O. Vittori" on the top of Mt. Cimone (2165 m a.s.l., Northern-Central Italy). It consists of RedPitaya ARM-FPGA boards designed for acquiring events at different energies from scintillator crystals coupled to photomultiplier tubes, and a main computer that executes a real-time software pipeline. The software performs several data processing steps, data acquisition, data reduction level, algorithms for waveform selection, and finally it produces the cumulative energy spectrum of the gamma radiation collected by the photomultipliers. Data is stored in different layers, each with a different purpose, and it is available to the scientific community as HDF5 files. The pipeline has a modular architecture to provide good maintenance and flexibility, allowing for easy extensions in the future. A specific subset of data is stored in a database connected to a real-time graphical dashboard for quick-look analysis, showing the acquisition products and the environmental telemetry data....
2022
- The RTApipe framework for the gamma-ray real-time analysis software development
[Articolo su rivista]
Parmiggiani, N.; Bulgarelli, A.; Beneventano, D.; Fioretti, V.; Di Piano, A.; Baroncelli, L.; Addis, A.; Tavani, M.; Pittori, C.; Oya, I.
abstract
In the multi-messenger era, coordinating observations between astronomical facilities is mandatory to study transient phenomena (e.g. Gamma-ray bursts) and is achieved by sharing information with the scientific community through networks such as the Gamma-ray Coordinates Network. The facilities usually develop real-time scientific analysis pipelines to detect transient events, alert the astrophysical community, and speed up the reaction time of science alerts received from other observatories. We present in this work the RTApipe framework, designed to facilitate the development of real-time scientific analysis pipelines for present and future gamma-ray observatories. This framework provides pipeline architecture and automatisms, allowing the researchers to focus on the scientific aspects and integrate existing science tools developed with different technologies. The pipelines automatically execute all the configured analyses during the data acquisition. This framework can be interfaced with science alerts networks to perform follow-up analysis of transient events shared by other facilities. The analyses are performed in parallel and can be prioritised. The workload is highly scalable on a cluster of machines. The framework provides the required services using containerisation technology for easy deployment. We present the RTA pipelines developed for the AGILE space mission and the prototype of the SAG system for the ground-based future Cherenkov Telescope Array observatory confirming that the RTApipe framework can be used to successfully develop pipelines for the gamma-ray observatories, both space and ground-based.
2022
- The Science Alert Generation system of the Cherenkov Telescope Array Observatory
[Articolo su rivista]
Bulgarelli, Andrea; Caroff, Sami; Addis, Antonio; Aubert, Pierre; Baroncelli, Leonardo; De Cesare, Giovanni; Di Piano, Ambra; Fioretti, Valentina; Garcia, Enrique; Maurin, Gilles; Parmiggiani, Nicolò; Vuillaume, Thomas; Oya, Igor; Hoischen, Clemens
abstract
The Cherenkov Telescope Array (CTA) Observatory, with dozens of telescopes located in both the Northern and Southern Hemispheres, will be the largest ground-based gamma-ray observatory and will provide broad energy coverage from 20 GeV to 300 TeV. The large effective area and field-of-view, coupled with the fast slewing capability and unprecedented sensitivity, make CTA a crucial instrument for the future of ground-based gamma-ray astronomy. To maximise the scientific return, the array will send alerts on transients and variable phenomena (e.g. gamma-ray burst, active galactic nuclei, gamma-ray binaries, serendipitous sources). Rapid and effective communication to the community requires a reliable and automated system to detect and issue candidate science alerts. This automation will be accomplished by the Science Alert Generation (SAG) pipeline, a key system of the CTA Observatory. SAG is part of the Array Control and Data Acquisition (ACADA) working group. The SAG working group develops the pipelines to perform data reconstruction, data quality monitoring, science monitoring and real-time alert issuing during observations to the Transients Handler functionality of ACADA. SAG is the system that performs the first real-time scientific analysis after the data acquisition. The system performs analysis on multiple time scales (from seconds to hours). abrb{SAG must issue candidate science alerts within} 20 seconds from the data taking and with sensitivity at least half of the CTA nominal sensitivity. These challenging requirements must be fulfilled by managing trigger rates of tens of kHz from the arrays. Dedicated and highly optimised software and hardware architecture must thus be designed and tested. In this work, we present the general architecture of the ACADA-SAG system....
2022
- The Second AGILE MCAL Gamma-Ray Burst Catalog: 13 yr of Observations
[Articolo su rivista]
Ursi, A.; Romani, M.; Verrecchia, F.; Pittori, C.; Tavani, M.; Marisaldi, M.; Galli, M.; Labanti, C.; Parmiggiani, N.; Bulgarelli, A.; Addis, A.; Baroncelli, L.; Cardillo, M.; Casentini, C.; Cattaneo, P. W.; Chen, A.; Di Piano, A.; Fuschino, F.; Longo, F.; Lucarelli, F.; Morselli, A.; Piano, G.; Vercellone, S.
abstract
We present the results of a systematic search and analysis of GRBs detected by the Astrorivelatore Gamma ad Immagini LEggero (AGILE) MiniCALorimeter (MCAL; 0.4-100 MeV) over a time frame of 13 yr, from 2007 to 2020 November. The MCAL GRB sample consists of 503 bursts triggered by MCAL, 394 of which were fully detected onboard with high time resolution. The sample consists of about 44% short GRBs and 56% long GRBs. In addition, 109 bursts triggered partial MCAL onboard data acquisitions, providing further detections that can be used for joint analyses or triangulations. More than 90% of these GRBs were also detected by the AGILE Scientific RateMeters (RMs), providing simultaneous observations between 20 keV and 100 MeV. We performed spectral analysis of these events in the 0.4-50 MeV energy range. We could fit the time-integrated spectrum of 258 GRBs with a single power-law model, resulting in a mean photon index beta of-2.3. Among them, 43 bursts could also be fitted with a Band model, with peak energy above 400 keV, resulting in a mean low-energy photon index alpha = -0.6, a mean high-energy photon index beta = -2.5, and a mean peak energy E ( p ) = 640 keV. The AGILE MCAL GRB sample mostly consists of hard-spectrum GRBs, with a large fraction of short-duration events. We discuss properties and features of the MCAL bursts, whose detections can be used to perform joint broad-band analysis with other missions, and to provide insights on the high-energy component of the prompt emission in the tens of mega electron volt energy range.
2022
- The online observation quality system software architecture for the ASTRI Mini-Array project
[Relazione in Atti di Convegno]
Parmiggiani, N.; Bulgarelli, A.; Baroncelli, L.; Addis, A.; Fioretti, V.; Di Piano, A.; Capalbi, M.; Catalano, O.; Conforti, V.; Fiori, M.; Gianotti, F.; Iovenitti, S.; Lucarelli, F.; Maccarone, M. C.; Mineo, T.; Lombardi, S.; Pastore, V.; Russo, F.; Sangiorgi, P.; Scuderi, S.; Tosti, G.; Trifoglio, M.; Zampieri, L.
abstract
The ASTRI Mini-Array is an international collaboration led by the Italian National Institute for Astrophysics. This project aims to construct and operate an array of nine Imaging Atmospheric Cherenkov Telescopes to study gamma-ray sources at very high energy (TeV) and perform stellar intensity interferometry observations. We describe the software architecture and the technologies used to implement the Online Observation Quality System (OOQS) for the ASTRI Mini-Array project. The OOQS aims to execute data quality checks on the data acquired in real-time by the Cherenkov cameras and intensity interferometry instruments, and provides feedback to both the Central Control System and the Operator about abnormal conditions detected. The OOQS can notify other sub-systems, triggering their reaction to promptly correct anomalies. The results from the data quality analyses (e.g. camera plots, histograms, tables, and more) are stored in the Quality Archive for further investigation and they are summarised in reports available to the Operator. Once the OOQS results are stored, the operator can visualize them using the Human Machine Interface. The OOQS is designed to manage the high data rate generated by the instruments (up to 4.5 GB/s) and received from the Array Data Acquisition System through the Kafka service. The data are serialized and deserialized during the transmission using the Avro framework. The Slurm workload scheduler executes the analyses exploiting key features such as parallel analyses and scalability....
2021
- A Deep Learning Method for AGILE-GRID Gamma-Ray Burst Detection
[Articolo su rivista]
Parmiggiani, N.; Bulgarelli, A.; Fioretti, V.; Di Piano, A.; Giuliani, A.; Longo, F.; Verrecchia, F.; Tavani, M.; Beneventano, D.; Macaluso, A.
abstract
The follow-up of external science alerts received from gamma-ray burst (GRB) and gravitational wave detectors is one of the AGILE Team's current major activities. The AGILE team developed an automated real-time analysis pipeline to analyze AGILE Gamma-Ray Imaging Detector (GRID) data to detect possible counterparts in the energy range 0.1-10 GeV. This work presents a new approach for detecting GRBs using a convolutional neural network (CNN) to classify the AGILE-GRID intensity maps by improving the GRB detection capability over the Li & Ma method, currently used by the AGILE team. The CNN is trained with large simulated data sets of intensity maps. The AGILE complex observing pattern due to the so-called "spinning mode"is studied to prepare data sets to test and evaluate the CNN. A GRB emission model is defined from the second Fermi-LAT GRB catalog and convoluted with the AGILE observing pattern. Different p-value distributions are calculated, evaluating, using the CNN, millions of background-only maps simulated by varying the background level. The CNN is then used on real data to analyze the AGILE-GRID data archive, searching for GRB detections using the trigger time and position taken from the Swift-BAT, Fermi-GBM, and Fermi-LAT GRB catalogs. From these catalogs, the CNN detects 21 GRBs with a significance of ≥3σ, while the Li & Ma method detects only two GRBs. The results shown in this work demonstrate that the CNN is more effective in detecting GRBs than the Li & Ma method in this context and can be implemented into the AGILE-GRID real-time analysis pipeline.
2021
- A deep learning method for AGILE-GRID GRB detection
[Altro]
Parmiggiani, N.; Bulgarelli, A.; Fioretti, V.; Di Piano, A.; Giuliani, A.; Longo, F.; Verrecchia, F.; Tavani, M.; Beneventano, D.; Macaluso, A.
abstract
2021
- AGILE Observations of Fast Radio Bursts
[Articolo su rivista]
Verrecchia, F.; Casentini, C.; Tavani, M.; Ursi, A.; Mereghetti, S.; Pilia, M.; Cardillo, M.; Addis, A.; Barbiellini, G.; Baroncelli, L.; Bulgarelli, A.; Cattaneo, P. W.; Chen, A.; Costa, E.; Del Monte, E.; Di Piano, A.; Ferrari, A.; Fioretti, V.; Longo, F.; Lucarelli, F.; Parmiggiani, N.; Piano, G.; Pittori, C.; Rappoldi, A.; Vercellone, S.
abstract
We report on a systematic search for hard X-ray and γ-ray emission in coincidence with fast radio bursts (FRBs) observed by the AGILE satellite. We used 13 yr of AGILE archival data searching for time coincidences between exposed FRBs and events detectable by the MCAL (0.4-100 MeV) and GRID (50 MeV-30 GeV) detectors at timescales ranging from milliseconds to days/weeks. The current AGILE sky coverage allowed us to extend the search for high-energy emission preceding and following the FRB occurrence. We considered all FRB sources currently included in catalogs and identified a subsample (15 events) for which a good AGILE exposure with either MCAL or GRID was obtained. In this paper we focus on nonrepeating FRBs, compared to a few nearby repeating sources. We did not detect significant MeV or GeV emission from any event. Our hard X-ray upper limits (ULs) in the MeV energy range were obtained for timescales from submillisecond to seconds, and in the GeV range from minutes to weeks around event times. We focus on a subset of five nonrepeating and two repeating FRB sources whose distances are most likely smaller than that of 180916.J0158+65 (150 Mpc). For these sources, our MeV ULs translate into ULs on the isotropically emitted energy of about 3 1046 erg, comparable to that observed in the 2004 giant flare from the Galactic magnetar SGR 1806-20. On average, these nearby FRBs emit radio pulses of energies significantly larger than the recently detected SGR 1935+2154 and are not yet associated with intense MeV flaring.
2021
- AGILE confirmation of the gamma-ray flaring activity from the blazar BL Lac
[Altro]
Piano, G.; Pittori, C.; Verrecchia, F.; Vercellone, S.; Donnarumma, I.; Tavani, M.; Bulgarelli, A.; Fioretti, V.; Parmiggiani, N.; Addis, A.; Baroncelli, L.; Di Piano, A.; Lucarelli, F.; Cardillo, M.; Ursi, A.; Casentini, C.; Gianotti, F.; Trifoglio, M.; Giuliani, A.; Mereghetti, S.; Caraveo, P.; Perotti, F.; Chen, A.; Argan, A.; Costa, E.; Del Monte, E.; Evangelista, Y.; Feroci, M.; Foffano, L.; Lapshov, I.; Menegoni, E.; Pacciani, L.; Soffitta, P.; Vittorini, V.; Lazzarotto, F.; Di Cocco, G.; Fuschino, F.; Galli, M.; Labanti, C.; Marisaldi, M.; Pellizzoni, A.; Pilia, M.; Trois, A.; Barbiellini, G.; Longo, F.; Vallazza, E.; Morselli, A.; Picozza, P.; Prest, M.; Lipari, P.; Zanello, D.; Cattaneo, P. W.; Rappoldi, A.; Ferrari, A.; Paoletti, F.; Antonelli, L. A.; Giommi, P.; Salotti, L.; Valentini, G.; D'Amico, F.
abstract
AGILE is detecting enhanced gamma-ray activity above 100 MeV from the blazar BL Lacertae. Integrating from 2021-07-11 UT 00:00:00 to 2021-07-13 UT 12:00:00 (MJD: 59406.0 - 59408.5), a preliminary multi-source maximum likelihood analysis yields a detection of 6 sigma and a flux F( > 100 MeV) = (3.2 +/- 0.9) x 10^-6 photons/cm^2/s....
2021
- AGILE confirmation of the gamma-ray flaring activity from the two blazars PKS 0514-459 and TXS 1700+685
[Altro]
Bulgarelli, A.; Pittori, C.; Tavani, M.; Fioretti, V.; Parmiggiani, N.; Addis, A.; Baroncelli, L.; Di Piano, A.; Lucarelli, F.; Verrecchia, F.; Cardillo, M.; Casentini, C.; Piano, G.; Ursi, A.; Donnarumma, I.; Vercellone, S.; Gianotti, F.; Trifoglio, M.; Giuliani, A.; Mereghetti, S.; Caraveo, P.; Perotti, F.; Chen, A.; Argan, A.; Costa, E.; Del Monte, E.; Evangelista, Y.; Feroci, M.; Lazzarotto, F.; Lapshov, I.; Pacciani, L.; Soffitta, P.; Vittorini, V.; Di Cocco, G.; Fuschino, F.; Galli, M.; Labanti, C.; Marisaldi, M.; Pellizzoni, A.; Pilia, M.; Trois, A.; Barbiellini, G.; Vallazza, E.; Antonelli, L. A.; Longo, F.; Morselli, A.; Picozza, P.; Prest, M.; Lipari, P.; Zanello, D.; Cattaneo, P. W.; Rappoldi, A.; Ferrari, A.; Paoletti, F.; Giommi, P.; Salotti, L.; Valentini, G.; D'Amico, F.
abstract
AGILE confirms intense gamma-ray activity above 100 MeV from the blazars PKS 0514-459 and TXS 1700+685, as previously reported by Fermi-LAT team with ATel #14633....
2021
- AGILE detection of enhanced gamma-ray activity from the blazar BL Lac
[Altro]
Piano, G.; Pittori, C.; Tavani, M.; Vercellone, S.; Donnarumma, I.; Bulgarelli, A.; Fioretti, V.; Parmiggiani, N.; Addis, A.; Baroncelli, L.; Di Piano, A.; Verrecchia, F.; Lucarelli, F.; Cardillo, M.; Ursi, A.; Casentini, C.; Gianotti, F.; Trifoglio, M.; Giuliani, A.; Mereghetti, S.; Caraveo, P.; Perotti, F.; Chen, A.; Argan, A.; Costa, E.; Del Monte, E.; Evangelista, Y.; Feroci, M.; Foffano, L.; Lapshov, I.; Menegoni, E.; Pacciani, L.; Soffitta, P.; Vittorini, V.; Lazzarotto, F.; Di Cocco, G.; Fuschino, F.; Galli, M.; Labanti, C.; Marisaldi, M.; Pellizzoni, A.; Pilia, M.; Trois, A.; Barbiellini, G.; Longo, F.; Vallazza, E.; Morselli, A.; Picozza, P.; Prest, M.; Lipari, P.; Zanello, D.; Cattaneo, P. W.; Rappoldi, A.; Ferrari, A.; Paoletti, F.; Antonelli, L. A.; Giommi, P.; Salotti, L.; Valentini, G.; D'Amico, F.
abstract
AGILE is detecting enhanced gamma-ray activity above 100 MeV from the blazar BL Lacertae. Integrating from 2021-08-06 UT 18:00:00 to 2021-08-08 UT 18:00:00 (MJD: 59432.75 - 59434.75), a preliminary multi-source maximum likelihood analysis yields a detection significance greater than 7 sigma and a flux F( > 100 MeV) = (6 +/- 1) x 10^-6 photons/cm^2/s....
2021
- AGILE detection of gamma-ray flaring activity from the FSRQ TXS 0646-176
[Altro]
Pittori, C.; Lucarelli, F.; Bulgarelli, A.; Tavani, M.; Fioretti, V.; Parmiggiani, N.; Addis, A.; Baroncelli, L.; Di Piano, A.; Verrecchia, F.; Piano, G.; Ursi, A.; Cardillo, M.; Casentini, C.; Menegoni, E.; Foffano, L.; Vercellone, S.; Donnarumma, I.; Gianotti, F.; Trifoglio, M.; Giuliani, A.; Mereghetti, S.; Caraveo, P.; Perotti, F.; Chen, A.; Argan, A.; Costa, E.; Del Monte, E.; Evangelista, Y.; Feroci, M.; Foffano, L.; Lapshov, I.; Menegoni, E.; Pacciani, L.; Soffitta, P.; Vittorini, V.; Lazzarotto, F.; Di Cocco, G.; Fuschino, F.; Galli, M.; Labanti, C.; Marisaldi, M.; Pellizzoni, A.; Pilia, M.; Trois, A.; Barbiellini, G.; Longo, F.; Vallazza, E.; Morselli, A.; Picozza, P.; Prest, M.; Lipari, P.; Zanello, D.; Cattaneo, P. W.; Rappoldi, A.; Ferrari, A.; Paoletti, F.; Antonelli, L. A.; Giommi, P.; Salotti, L.; Valentini, G.; D'Amico, F.
abstract
AGILE is detecting enhanced gamma-ray activity above 100 MeV from the FSRQ TXS 0646-176 (also known as 5BZQJ0648-1744, 4FGL J0648.4-1743) with radio coordinates R.A. = 102.1187437 deg, Decl....
2021
- AGILE detection of gamma-ray flaring activity from the FSRQ Ton 0599
[Altro]
Verrecchia, F.; Piano, G.; Pittori, C.; Vercellone, S.; Donnarumma, I.; Tavani, M.; Bulgarelli, A.; Fioretti, V.; Parmiggiani, N.; Addis, A.; Baroncelli, L.; Di Piano, A.; Lucarelli, F.; Cardillo, M.; Ursi, A.; Casentini, C.; Gianotti, F.; Trifoglio, M.; Giuliani, A.; Mereghetti, S.; Caraveo, P.; Perotti, F.; Chen, A.; Argan, A.; Costa, E.; Del Monte, E.; Evangelista, Y.; Feroci, M.; Foffano, L.; Lapshov, I.; Menegoni, E.; Pacciani, L.; Soffitta, P.; Vittorini, V.; Lazzarotto, F.; Di Cocco, G.; Fuschino, F.; Galli, M.; Labanti, C.; Marisaldi, M.; Pellizzoni, A.; Pilia, M.; Trois, A.; Barbiellini, G.; Longo, F.; Vallazza, E.; Morselli, A.; Picozza, P.; Prest, M.; Lipari, P.; Zanello, D.; Cattaneo, P. W.; Rappoldi, A.; Ferrari, A.; Paoletti, F.; Antonelli, L. A.; Giommi, P.; Salotti, L.; Valentini, G.; D'Amico, F.
abstract
AGILE is detecting enhanced gamma-ray activity above 100 MeV from the FSRQ Ton 0599 (also known as 4C 29.45, PKS 1156+295; ICRS coords. (ep=J2000): RA 11 59 31.834 Dec +29 14 43.827, z=0.7)....
2021
- AGILE detection of intense transient gamma-ray activity from Cygnus X-3 emerging from a prolonged quenching state
[Altro]
Piano, G.; Verrecchia, F.; Pittori, C.; Tavani, M.; Bulgarelli, A.; Fioretti, V.; Parmiggiani, N.; Addis, A.; Baroncelli, L.; Di Piano, A.; Lucarelli, F.; Vercellone, S.; Cardillo, M.; Ursi, A.; Casentini, C.; Donnarumma, I.; Gianotti, F.; Trifoglio, M.; Giuliani, A.; Mereghetti, S.; Caraveo, P.; Perotti, F.; Chen, A.; Argan, A.; Costa, E.; Del Monte, E.; Evangelista, Y.; Feroci, M.; Foffano, L.; Lapshov, I.; Menegoni, E.; Pacciani, L.; Soffitta, P.; Vittorini, V.; Lazzarotto, F.; Di Cocco, G.; Fuschino, F.; Galli, M.; Labanti, C.; Marisaldi, M.; Pellizzoni, A.; Pilia, M.; Trois, A.; Barbiellini, G.; Longo, F.; Vallazza, E.; Morselli, A.; Picozza, P.; Prest, M.; Lipari, P.; Zanello, D.; Cattaneo, P. W.; Rappoldi, A.; Ferrari, A.; Paoletti, F.; Antonelli, L. A.; Giommi, P.; Salotti, L.; Valentini, G.; D'Amico, F.
abstract
The microquasar Cyg X-3 recently emerged from a deep and prolonged quenched state, which lasted about 5 months, on October 18, 2021 (Swift/BAT, 15-50 keV; http://swift.gsfc.nasa.gov/results/transients/CygX-3/)....
2021
- AGILE detection of transient gamma-ray activity from Cygnus X-3 during a prolonged quenched state
[Altro]
Piano, G.; Bulgarelli, A.; Verrecchia, F.; Pittori, C.; Tavani, M.; Fioretti, V.; Parmiggiani, N.; Addis, A.; Baroncelli, L.; Di Piano, A.; Lucarelli, F.; Vercellone, S.; Cardillo, M.; Ursi, A.; Casentini, C.; Donnarumma, I.; Gianotti, F.; Trifoglio, M.; Giuliani, A.; Mereghetti, S.; Caraveo, P.; Perotti, F.; Chen, A.; Argan, A.; Costa, E.; Del Monte, E.; Evangelista, Y.; Feroci, M.; Foffano, L.; Lapshov, I.; Menegoni, E.; Pacciani, L.; Soffitta, P.; Vittorini, V.; Lazzarotto, F.; Di Cocco, G.; Fuschino, F.; Galli, M.; Labanti, C.; Marisaldi, M.; Pellizzoni, A.; Pilia, M.; Trois, A.; Barbiellini, G.; Longo, F.; Vallazza, E.; Morselli, A.; Picozza, P.; Prest, M.; Lipari, P.; Zanello, D.; Cattaneo, P. W.; Rappoldi, A.; Ferrari, A.; Paoletti, F.; Antonelli, L. A.; Giommi, P.; Salotti, L.; Valentini, G.; D'Amico, F.
abstract
The microquasar Cyg X-3 is currently in a deep and prolonged quenched state. The hard X-ray emission is currently not detected (Swift/BAT, 15-50 keV; http://swift.gsfc.nasa.gov/results/transients/CygX-3/)....
2021
- AGILE detects a gamma-ray rebrightening of the blazar PKS 0903-57
[Altro]
Pittori, C.; Bulgarelli, A.; Tavani, M.; Verrecchia, F.; Lucarelli, F.; Vercellone, S.; Donnarumma, I.; Fioretti, V.; Parmiggiani, N.; Addis, A.; Baroncelli, L.; Di Piano, A.; Piano, G.; Cardillo, M.; Ursi, A.; Casentini, C.; Gianotti, F.; Trifoglio, M.; Giuliani, A.; Mereghetti, S.; Caraveo, P.; Perotti, F.; Chen, A.; Argan, A.; Costa, E.; Del Monte, E.; Evangelista, Y.; Feroci, M.; Foffano, L.; Lapshov, I.; Menegoni, E.; Pacciani, L.; Soffitta, P.; Vittorini, V.; Lazzarotto, F.; Di Cocco, G.; Fuschino, F.; Galli, M.; Labanti, C.; Marisaldi, M.; Pellizzoni, A.; Pilia, M.; Trois, A.; Barbiellini, G.; Longo, F.; Vallazza, E.; Morselli, A.; Picozza, P.; Prest, M.; Lipari, P.; Zanello, D.; Cattaneo, P. W.; Rappoldi, A.; Ferrari, A.; Paoletti, F.; Antonelli, L. A.; Giommi, P.; Salotti, L.; Valentini, G.; D'Amico, F.
abstract
AGILE is detecting intense gamma-ray activity above 100 MeV from the FSRQ PKS 0903-57 (also known as 5BZU J0904-5735). Integrating from 2021-12-03 08:00 UT until 2021-12-05 08:00 a preliminary multi-source maximum likelihood analysis yields a detection at the level of 6.2 sigma and a flux F( > 100 MeV) = (3.4 +/- 0.9) x 10^-6 photons/cm^2/s....
2021
- Agilepy: A Python framework for scientific analysis of AGILE data
[Relazione in Atti di Convegno]
Bulgarelli, A.; Baroncelli, L.; Addis, A.; Parmiggiani, N.; Aboudan, A.; Di Piano, A.; Fioretti, V.; Tavani, M.; Pittori, C.; Lucarelli, F.; Verrecchia, F.
abstract
The Italian AGILE space mission, with its Gamma-Ray Imaging Detector (GRID) instrument sensitive in the 30 MeV-50 GeV gamma-ray energy band, has been operating since 2007. Agilepy is an open-source Python package to analyse AGILE/GRID data. The package is built on top of the command-line version of the AGILE Science Tools, developed by the AGILE Team, publicly available and released by ASI/SSDC. The primary purpose of the package is to provide an easy to use high-level interface to analyse AGILE/GRID data by simplifying the configuration of the tasks and ensuring straightforward access to the data. The current features are the generation and display of sky maps and light curves, the access to gamma-ray sources catalogues, the analysis to perform spectral model and position fitting, the wavelet analysis. Agilepy also includes an interface tool providing the time evolution of the AGILE off-axis viewing angle for a chosen sky region. The Flare Advocate team also uses the tool to analyse the data during the daily monitoring of the gamma-ray sky. Agilepy (and its dependencies) can be easily installed using Anaconda....
2021
- GRB 210410A: AGILE detection of a burst
[Altro]
Ursi, A.; Verrecchia, F.; Pittori, C.; Tavani, M.; Argan, A.; Cardillo, M.; Casentini, C.; Evangelista, Y.; Piano, G.; Lucarelli, F.; Bulgarelli, A.; di Piano, A.; Fioretti, V.; Fuschino, F.; Parmiggiani, N.; Marisaldi, M.; Pilia, M.; Trois, A.; Donnarumma, I.; Longo, F.; Giuliani, A.; Agile, Team
abstract
The AGILE satellite detected the long GRB 210410A at T0 = 2021-04-10 00:53:16.64 +/- 0.01 s (UTC), reported by Fermi/GBM (GCN #29777), Swift/XRT (GCN #29778), and Fermi/LAT (GCN #29781). The burst is visible in the AGILE scientific ratemeters of the SuperAGILE (SA; 20-60 keV), MiniCALorimeter (MCAL; 0.4-100 MeV), and AntiCoincidence (AC; 50-200 keV) detectors. The event lasted ~15 s and released a total number of 1050 counts in the SA detector (above a background rate of 60 Hz), 29400 counts in the MCAL detector (above a background rate of 1200 Hz), and 72250 counts in the AC detector (above a background rate of 3320 Hz). The AGILE ratemeter light curves can be found at http://www.agilescienceapp.it/notices/GRB210410_AGILE_RM.png . The event also triggered a partial high time resolution MCAL data acquisition, whose light curve can be found at: http://www.agilescienceapp.it/notices/072541_GRB_545100796.643050.png . Additional analysis of AGILE data is in progress. Automatic MCAL GRB alert Notices can be found at: https://gcn.gsfc.nasa.gov/agile_mcal.html....
2021
- GRB 210410A: AGILE/MCAL analysis
[Altro]
Ursi, A.; Tavani, M.; Verrecchia, F.; Pittori, C.; Argan, A.; Cardillo, M.; Casentini, C.; Evangelista, Y.; Piano, G.; Lucarelli, F.; Bulgarelli, A.; di Piano, A.; Fioretti, V.; Fuschino, F.; Parmiggiani, N.; Marisaldi, M.; Pilia, M.; Trois, A.; Donnarumma, I.; Longo, F.; Giuliani, A.; Agile, Team
abstract
We carried out further analysis of the AGILE/MCAL data of GRB 210410A (GCNs #29777, #29778, #29779, #29780, #29781, #29782, #29786, #29787, #29788, #29790, #29793, #29797). The first 4 s of the burst released 5700 counts in the detector, above a background rate of 560 Hz. The spectral analysis shows a clear component up to 100 MeV. The time-integrated spectrum of the first ~4 s of the burst can be fitted in the energy range 0.4-100 MeV with a CPL model with alpha = -0.6 -0.25/+0.14 and cutoff energy Ec = 500 keV, plus an extra PL component with ph.ind. -1.31 -0.04/+0.04. The fit results in a reduced chi-squared of 1.48 (81 d.o.f.) and a fluence of 1.3e-04 ergs/cm^2 (90% confidence level), in the same energy range. We divided the data acquisition into three time intervals: interval a [T0-0.16s - T0+0.70s], interval b [T0+0.70s - T0+2.85s], and interval c [T0+2.85s - T0+4.00s]. The spectral analysis in the energy range 0.4-100 MeV shows an evolution of the PL high-energy spectral component, evolving from alpha = -1.91 to alpha = -0.92. Details are reported below: | model | CPL alpha | Ec | PL ph.ind. | redX^2 (dof) a | CPL+PL | -0.57 -1.46/+3.84 | 438 keV | -1.91 -0.96/+0.78 | 0.97 (81) b | CPL+PL | -0.57 -1.68/+0.67 | 365 keV | -0.98 -0.61/+0.39 | 1.38 (81) c | PL | ----- | --- | -0.92 -0.98/+0.40 | 1.05 (84) MCAL light curve and spectra in the different time intervals can be found at: http://www.agilescienceapp.it/notices/GRB210410A_AGILE-MCAL_intervals.png . Additional analysis of AGILE data is in progress. Automatic MCAL GRB alert Notices can be found at: https://gcn.gsfc.nasa.gov/agile_mcal.html....
2021
- GRB 210511B: AGILE detection
[Altro]
Ursi, A.; Fioretti, V.; Tavani, M.; Argan, A.; Cardillo, M.; Casentini, C.; Evangelista, Y.; Piano, G.; Lucarelli, F.; Pittori, C.; Verrecchia, F.; Bulgarelli, A.; di Piano, A.; Fuschino, F.; Parmiggiani, N.; Marisaldi, M.; Pilia, M.; Trois, A.; Donnarumma, I.; Longo, F.; Giuliani, A.; Agile, Team
abstract
The AGILE satellite detected the long GRB 210511B at T0 = 2021-05-11 11:26:37 s (UTC), reported by Fermi/GBM (GCN #29988) and GECAM (GCN #29990). The burst is visible in the AGILE scientific ratemeters of the SuperAGILE (SA; 20-60 keV), MiniCALorimeter (MCAL; 0.4-100 MeV), and AntiCoincidence (AC; 50-200 keV) detectors. The event lasted ~12 s and released a total number of 2120 counts in the SA detector (above a background rate of 64 Hz), 15630 counts in the MCAL detector (above a background rate of 1260 Hz), and 48375 counts in the AC detector (above a background rate of 3865 Hz). The AGILE ratemeter light curves can be found at http://www.agilescienceapp.it/notices/GRB210511_AGILE_RM.png . Additional analysis of AGILE data is in progress....
2021
- GRB 210517A: AGILE detection
[Altro]
Ursi, A.; Pittori, C.; Piano, G.; Tavani, M.; Argan, A.; Cardillo, M.; Casentini, C.; Evangelista, Y.; Lucarelli, F.; Verrecchia, F.; Bulgarelli, A.; di Piano, A.; Fioretti, V.; Fuschino, F.; Parmiggiani, N.; Marisaldi, M.; Pilia, M.; Trois, A.; Donnarumma, I.; Longo, F.; Giuliani, A.; Agile, Team
abstract
The AGILE satellite detected the long GRB 210517A at T0 = 2021-05-17 05:28:01 s (UTC), reported by Swift (GCNs #30032, #30034). The burst is visible in the AGILE scientific ratemeters of the SuperAGILE (SA; 20-60 keV) and MiniCALorimeter (MCAL; 0.4-100 MeV) detectors. The event lasted ~6 s and released a total number of 663 counts in the SA detector (above a background rate of 77 Hz) and 8759 counts in the MCAL detector (above a background rate of 1200 Hz). The AGILE ratemeter light curves can be found at http://www.agilescienceapp.it/notices/GRB210517A_AGILE_RM.png . Additional analysis of AGILE data is in progress....
2021
- GRB 210531A: AGILE/MCAL detection (Fermi Trigger 644155206 / GRB 210531500)
[Altro]
Ursi, A.; Longo, F.; Tavani, M.; Argan, A.; Cardillo, M.; Casentini, C.; Evangelista, Y.; Piano, G.; Lucarelli, F.; Pittori, C.; Verrecchia, F.; Bulgarelli, A.; di Piano, A.; Fioretti, V.; Fuschino, F.; Parmiggiani, N.; Marisaldi, M.; Pilia, M.; Trois, A.; Donnarumma, I.; Giuliani, A.; Agile, Team
abstract
The AGILE satellite detected the long GRB 210531A at T0 = 2021-05-31 12:00:02.66 +/- 0.01 s (UTC), reported by Fermi/GBM (Trigger no. 644155206) and BALROG (GCN #30105). The burst is clearly visible in the AGILE scientific ratemeters of the SuperAGILE (SA; 20-60 keV), MiniCALorimeter (MCAL; 0.4-100 MeV), and AntiCoincidence (AC; 50-200 keV) detectors. The event lasted about 35 s and it released a total number of 9650 counts in the SA detector (above a background rate of 90 Hz), 53900 counts in the MCAL detector (above a background rate of 1400 Hz), and 151900 counts in the AC detector (above a background rate of 3780 Hz). The AGILE ratemeter light curves can be found at http://www.agilescienceapp.it/notices/GRB210531A_AGILE_RM.png . The event also triggered a partial high time resolution MCAL data acquisition. Additional analysis of AGILE data is in progress. Automatic MCAL GRB alert Notices can be found at: https://gcn.gsfc.nasa.gov/agile_mcal.html....
2021
- GRB 210606B: AGILE detection
[Altro]
Ursi, A.; Verrecchia, F.; Pittori, C.; Tavani, M.; Argan, A.; Cardillo, M.; Casentini, C.; Evangelista, Y.; Piano, G.; Lucarelli, F.; Bulgarelli, A.; di Piano, A.; Fioretti, V.; Fuschino, F.; Parmiggiani, N.; Marisaldi, M.; Pilia, M.; Trois, A.; Donnarumma, I.; Longo, F.; Giuliani, A.; Agile, Team
abstract
The AGILE satellite detected the long GRB 210606B at T0 = UTC 2021-06-06 22:41:03 s (UTC), reported by Fermi/GBM (Trigger no. 644712030), GECAM (GCN #39138), and BALROG (GCN #39139). The burst is visible in the AGILE scientific ratemeters of the SuperAGILE (SA; 20-60 keV), MiniCALorimeter (MCAL; 0.4-100 MeV), and AntiCoincidence (AC; 50-200 keV) detectors. The event lasted ~35 s and released a total number of 3120 counts in the SA detector (above a background rate of 66 Hz), 43110 counts in the MCAL detector (above a background rate of 1250 Hz), and 125100 counts in the AC detector (above a background rate of 3660 Hz). The AGILE ratemeter light curves can be found at http://www.agilescienceapp.it/notices/GRB210606B_AGILE_RM.png . The event also triggered a partial high time resolution MCAL data acquisition at T = UTC 2021-06-06 22:41:13.18 +/- 0.01 s, whose light curve can be found at: http://www.agilescienceapp.it/notices/073374_GRB_550104073.181294.png . Additional analysis of AGILE data is in progress. Automatic MCAL GRB alert Notices can be found at: https://gcn.gsfc.nasa.gov/agile_mcal.html....
2021
- GRB 211023A: AGILE detection
[Altro]
Ursi, A.; Cardillo, M.; Tavani, M.; Argan, A.; Casentini, C.; Evangelista, Y.; Menegoni, E.; Foffano, L.; Piano, G.; Lucarelli, F.; Pittori, C.; Verrecchia, F.; Bulgarelli, A.; di Piano, A.; Fioretti, V.; Fuschino, F.; Parmiggiani, N.; Marisaldi, M.; Pilia, M.; Trois, A.; Donnarumma, I.; Longo, F.; Giuliani, A.; Agile, Team
abstract
The AGILE satellite detected the long GRB 211023A at T0 = 2021-10-23 13:07:58 s (UTC), reported by Fermi GBM (GCNs #30958, #30965) and Fermi LAT (GCN #30961). The burst is clearly visible in the AGILE scientific ratemeters of the SuperAGILE (SA; 20-60 keV), MiniCALorimeter (MCAL; 0.4-100 MeV), and AntiCoincidence (AC; 50-200 keV) detectors. The event lasted about 45 s and it released a total number of 5300 counts in the SA detector (above a background rate of 90 Hz), 64325 counts in the MCAL detector (above a background rate of 1200 Hz), and 233020 counts in the AC detector (above a background rate of 2810 Hz). The AGILE ratemeter light curves can be found at http://www.agilescienceapp.it/notices/GRB211023A_AGILE_RM.png . The event also triggered a partial high time resolution MCAL data acquisition, from T1 = 2021-10-23 13:07:32.91 s +/- 0.01 (UTC) to T2 = 2021-10-23 13:08:05.20 +/- 0.01 s (UTC), and released 12480 counts in the detector, above a background rate of 620 Hz. The time-integrated spectrum of the first 17 s of the burst can be fitted in the energy range 0.4-50 MeV with a single power-law with ph. ind. = -2.45 (-0.27/+0.34), resulting in a reduced chi-squared of 0.89 (75 d.o.f.) and a fluence of 1.3e-05 ergs/cm^2 (90% confidence level), in the same energy range. The MCAL light curve can be found at: http://www.agilescienceapp.it/notices/075364_GRB211023A_562079268.000000.png . Additional analysis of AGILE data is in progress. Automatic MCAL GRB alert Notices can be found at: https://gcn.gsfc.nasa.gov/agile_mcal.html....
2021
- GRB 211105A: AGILE detection of a burst
[Altro]
Ursi, A.; Menegoni, E.; Pittori, C.; Verrecchia, F.; Tavani, M.; Argan, A.; Cardillo, M.; Casentini, C.; Evangelista, Y.; Foffano, L.; Piano, G.; Lucarelli, F.; Bulgarelli, A.; di Piano, A.; Fioretti, V.; Fuschino, F.; Parmiggiani, N.; Marisaldi, M.; Pilia, M.; Trois, A.; Donnarumma, I.; Longo, F.; Giuliani, A.; Agile, Team
abstract
The AGILE satellite detected the long GRB 211105A at T0 = 2021-11-05 04:36:04 s (UTC). The burst is clearly visible in the AGILE scientific ratemeters of the SuperAGILE (SA; 20-60 keV) and MiniCALorimeter (MCAL; 0.4-100 MeV) detectors. The event lasted about 100 s and it released a total number of 14900 counts in the SA detector (above a background rate of 105 Hz) and 133500 counts in the MCAL detector (above a background rate of 1240 Hz). The AGILE ratemeter light curves can be found at http://www.agilescienceapp.it/notices/GRB211105A_AGILE_RM.png Additional analysis of AGILE data is in progress....
2021
- GRB 211120A: AGILE detection
[Altro]
Ursi, A.; Pittori, C.; Trois, A.; Tavani, M.; Argan, A.; Cardillo, M.; Casentini, C.; Evangelista, Y.; Menegoni, E.; Foffano, L.; Piano, G.; Lucarelli, F.; Verrecchia, F.; Bulgarelli, A.; di Piano, A.; Fioretti, V.; Fuschino, F.; Parmiggiani, N.; Marisaldi, M.; Pilia, M.; Donnarumma, I.; Longo, F.; Giuliani, A.; Agile, Team
abstract
The AGILE satellite detected the GRB 211120A at T0 = 2021-11-20 23:05:20 (UTC), reported by GECAM (GCN #31100) and AstroSat CZTI (GCN #31104). The burst is clearly visible in the AGILE scientific ratemeters of the SuperAGILE (SA; 20-60 keV), MiniCALorimeter (MCAL; 0.4-100 MeV), and AntiCoincidence (AC; 50-200 keV) detectors. The event lasted about 6 s and it released a total number of 821 counts in the SA detector (above a background rate of 96 Hz), 9275 counts in the MCAL detector (above a background rate of 1210 Hz), and 23969 counts in the AC detector (above a background rate of 3440 Hz). The AGILE ratemeter light curves can be found at http://www.agilescienceapp.it/notices/GRB211120A_AGILE_RM.png . The event also triggered a partial high time resolution MCAL data acquisition, covering the main peak of the burst, from T1 = 2021-11-20 23:05:20.0 s +/- 0.5 (UTC) to T2 = 2021-11-20 23:05:26.0 +/- 0.5 s (UTC), and released 2291 counts in the detector, above a background rate of 710 Hz. The time-integrated spectrum of the burst between T1 and T1+2.43 s can be fitted in the energy range 0.4-20 MeV with a single power-law with ph. ind. = -2.43 (-0.34/+0.44), resulting in a reduced chi-squared of 1.09 (62 d.o.f.) and a fluence of 5.9e-06 ergs/cm^2 (90% confidence level), in the same energy range. The MCAL light curve can be found at: http://www.agilescienceapp.it/notices/GRB211120A_MCAL.png . Additional analysis of AGILE data is in progress. Automatic MCAL GRB alert Notices can be found at: https://gcn.gsfc.nasa.gov/agile_mcal.html. Please note that in the MCAL notice #564534599 the T0 was affected by a temporal shift of about +279 sec due to a temporary ground software problem now solved....
2021
- GRB 211229B: AGILE detection
[Altro]
Ursi, A.; Pittori, C.; Verrecchia, F.; Tavani, M.; Argan, A.; Cardillo, M.; Casentini, C.; Evangelista, Y.; Menegoni, E.; Foffano, L.; Piano, G.; Lucarelli, F.; Bulgarelli, A.; di Piano, A.; Fioretti, V.; Fuschino, F.; Parmiggiani, N.; Marisaldi, M.; Pilia, M.; Trois, A.; Donnarumma, I.; Longo, F.; Giuliani, A.; Agile, Team
abstract
The AGILE satellite detected the long GRB 211229B at T0 = 2021-12-29 22:18:43 (UTC), reported by Fermi GBM (GCN #31336) and GECAM (GCN #31339). The burst is clearly visible in the AGILE scientific ratemeters of the SuperAGILE (SA; 20-60 keV), MiniCALorimeter (MCAL; 0.4-100 MeV), and AntiCoincidence (AC; 50-200 keV) detectors. The event lasted about 40 s and it released a total number of 5440 counts in the SA detector (above a background rate of 120 Hz), 48230 counts in the MCAL detector (above a background rate of 1170 Hz), and 141030 counts in the AC detector (above a background rate of 3440 Hz). The AGILE ratemeters light curves can be found at http://www.agilescienceapp.it/notices/GRB211229B_AGILE_RM.png . The event also triggered a partial high time resolution MCAL data acquisition, covering the main peak of the burst, from T1 = 2021-12-29 22:18:45.12 s +/- 0.01 (UTC) to T2 = 2021-12-29 22:18:48.83 +/- 0.01 s (UTC), and released 2354 counts in the detector, above a background rate of 620 Hz. The time-integrated spectrum of the burst in this time interval can be fitted in the energy range 0.4-20 MeV with a power-law with ph. ind. = -2.45 (-0.82/+1.53), resulting in a reduced chi-squared of 1.40 (34 d.o.f.) and a fluence of 1.8e-06 ergs/cm^2 (90% confidence level), in the same energy range. The MCAL light curve can be found at: http://www.agilescienceapp.it/notices/GRB211229B_AGILE_MCAL.png . Additional analysis of AGILE data is in progress. Automatic MCAL GRB alert Notices can be found at: https://gcn.gsfc.nasa.gov/agile_mcal.html....
2021
- Multi-messenger and transient astrophysics with the Cherenkov Telescope Array
[Relazione in Atti di Convegno]
Bošnjak, Ž.; Brown, A. M.; Carosi, A.; Chernyakova, M.; Cristofari, P.; Longo, F.; López-Oramas, A.; Santander, M.; Satalecka, K.; Schüssler, F.; Sergijenko, O.; Stamerra, A.; Agudo, I.; Alves Batista, R.; Amato, E.; Anguner, E. O.; Antonelli, L. A.; Backes, M.; Balazs, Csaba; Baroncelli, L.; Becker Tjus, J.; Bigongiari, C.; Bissaldi, E.; Boisson, C.; Bolmont, J.; Böttcher, M.; Bordas, P.; Braiding, C.; Bregeon, J.; Bucciantini, N.; Bulgarelli, A.; Burton, M.; Cangemi, F.; Caraveo, P.; Cardillo, M.; Caroff, S.; Casanova, S.; Chaty, S.; Coelho, J. G.; Cotter, G.; D'Aì, A.; D'Ammando, F.; de Gouveia Dal Pino, E. M.; della Volpe, D.; de Martino, D.; Di Girolamo, T.; Di Piano, A.; Djannati-Ataï, A.; Dwarkadas, V.; de Ona Wilhelmi, E.; Dos Anjos, R. C.; Emery, G.; Fedorova, E.; Fegan, S.; Fiasson, A.; Fioretti, V.; Filipovic, M. D.; Gaggero, D.; Galanti, G.; Gasparrini, D.; Ghirlanda, G.; Goldoni, P.; Granot, J.; Green, J. G.; Heller, M.; Hnatyk, B.; Hnatyk, R.; Horan, D.; Hovatta, T.; Inoue, S.; Jamrozy, M.; Kantzas, D.; Khélifi, B.; Komin, N.; Lamastra, A.; La Palombara, N.; Lenain, J. P.; Lindfors, E.; Liodakis, I.; Lombardi, S.; Lucarelli, F.; Luque-Escamilla, P. L.; Majumdar, P.; Marcowith, A.; Markoff, S.; Marti, J.; Martinez, M.; Mazin, D.; Mckeague, S.; Mereghetti, S.; Mestre, E.; Montaruli, T.; Morlino, G.; Morselli, A.; Mundell, C.; Murach, T.; Nava, L.; Nayerhoda, A.; Nicastro, L.; Niemiec, J.; Nikolajuk, M.; Olmi, B.; Ong, R.; Orienti, M.; Osborne, J. P.; Paredes, Josep M.; Pareschi, G.; Parmiggiani, N.; Patricelli, B.; Pe'Er, A.; Piano, G.; Pühlhofer, G.; Punch, M.; Reimer, O.; Ribó, M.; Rodriguez, G.; Rodriguez, J.; Romano, P.; Romeo, G.; Roncadelli, M.; Rowell, G.; Rudak, B.; Sadeh, I.; Salesa Greus, F.; Saturni, F. G.; Sawangwit, U.; Seglar-Arroyo, M.; Shellard, R. C.; Sol, H.; Starling, R.; Stolarczyk, T.; Tagliaferri, G.; Tavecchio, F.; Tibaldo, L.; Testa, V.; Vercellone, S.; Viana, A.; Vink, J.; Vitale, V.; Vergani, S. D.; Vorobiov, S.; Wierzcholska, A.; Yang, L.; Zavrtanik, D.; Zhdanov, V.
abstract
The discovery of gravitational waves, high-energy neutrinos or the very-high-energy counterpart of gamma-ray bursts has revolutionized the high-energy and transient astrophysics community. The development of new instruments and analysis techniques will allow the discovery and/or follow-up of new transient sources. We describe the prospects for the Cherenkov Telescope Array (CTA), the next-generation ground-based gamma-ray observatory, for multi-messenger and transient astrophysics in the decade ahead. CTA will explore the most extreme environments via very-high-energy observations of compact objects, stellar collapse events, mergers and cosmic-ray accelerators....
2021
- Transient gamma-ray emission from Cygnus X-3 detected by AGILE during the current quenched/hypersoft state
[Altro]
Piano, G.; Pittori, C.; Verrecchia, F.; Tavani, M.; Bulgarelli, A.; Fioretti, V.; Parmiggiani, N.; Addis, A.; Baroncelli, L.; Di Piano, A.; Lucarelli, F.; Vercellone, S.; Cardillo, M.; Ursi, A.; Casentini, C.; Donnarumma, I.; Gianotti, F.; Trifoglio, M.; Giuliani, A.; Mereghetti, S.; Caraveo, P.; Perotti, F.; Chen, A.; Argan, A.; Costa, E.; Del Monte, E.; Evangelista, Y.; Feroci, M.; Lazzarotto, F.; Lapshov, I.; Menegoni, E.; Pacciani, L.; Soffitta, P.; Vittorini, V.; Di Cocco, G.; Fuschino, F.; Galli, M.; Labanti, C.; Marisaldi, M.; Pellizzoni, A.; Pilia, M.; Trois, A.; Barbiellini, G.; Longo, F.; Vallazza, E.; Morselli, A.; Picozza, P.; Prest, M.; Lipari, P.; Zanello, D.; Cattaneo, P. W.; Rappoldi, A.; Ferrari, A.; Paoletti, F.; Antonelli, L. A.; Giommi, P.; Salotti, L.; Valentini, G.; D'Amico, F.
abstract
The microquasar Cyg X-3 is currently in a deep quenched state, with a very low hard X-ray emission (Swift/BAT, 15-50 keV; http://swift.gsfc.nasa.gov/results/transients/CygX-3/)....
2021
- rta-dq-lib: a software library to perform online data quality analysis of scientific data
[Relazione in Atti di Convegno]
Baroncelli, Leonardo; Bulgarelli, Andrea; Parmiggiani, Nicolo; Fioretti, Valentina; Addis, Antonio; De Cesare, Giovanni; Di Piano, Ambra; Conforti, Vito; Gianotti, Fulvio; Russo, Federico; Maurin, Gilles; Vuillaume, Thomas; Aubert, Pierre; Garcia, Emilio; Zoccoli, Antonio
abstract
The Cherenkov Telescope Array (CTA) is an initiative that is currently building the largest gamma-ray ground Observatory that ever existed. A Science Alert Generation (SAG) system, part of the Array Control and Data Acquisition (ACADA) system of the CTA Observatory, analyses online the telescope data - arriving at an event rate of tens of kHz - to detect transient gamma-ray events. The SAG system also performs an online data quality analysis to assess the instruments' health during the data acquisition: this analysis is crucial to confirm good detections. A Python and a C++ software library to perform the online data quality analysis of CTA data, called rta-dq-lib, has been proposed for CTA. The Python version is dedicated to the rapid prototyping of data quality use cases. The C++ version is optimized for maximum performance. The library allows the user to define, through XML configuration files, the format of the input data and, for each data field, which quality checks must be performed and which types of aggregations and transformations must be applied. It internally translates the XML configuration into a direct acyclic computational graph that encodes the dependencies of the computational tasks to be performed. This model allows the library to easily take advantage of parallelization at the thread level and the overall flexibility allow us to develop generic data quality analysis pipelines that could also be reused in other applications....
2020
- Detection of short Gamma-Ray Bursts with CTA through real-time analysis
[Curatela]
DI PIANO, Ambra
abstract
2016
- Ottica geometrica e ondulatoria, e applicazioni astrofisiche
[Altro]
DI PIANO, Ambra
abstract