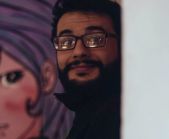 |
LEONARDO SANNA
|
Home |
Pubblicazioni
2023
- THE COVID-19 INFODEMIC ON TWITTER: Dialogic contraction within the echo chambers
[Capitolo/Saggio]
Bondi, M.; Sanna, L.
abstract
Fake news and misinformation are a key topic when discussing social media analysis research. Special attention has been paid to how social media discourse, rather than focusing on the correct identification of sources and voices, can end up constructing trust and credibility by emphasising shared identities and positions, usually in opposition to other views. Studies on “echo chambers” look at how the views of others are systematically rejected and used instrumentally to support one’s own beliefs. Twitter discourse is often a case in point. The focus of our analysis is on the language that manifests the writer’s position, starting from the concept of engagement as defined in Martin and White’s (2005) appraisal framework. This indicates the speaker’s degree of commitment to what is being expressed and manifests the speaker’s attitudes to opening and closing the dialogic space for external views. Using a corpus of tweets and one of journalistic texts on the pandemic, we test the hypothesis that the space given to dialogic contraction on Twitter may be wider than that provided by traditional journalism. The study - based on frequency analysis, concordance analysis, and word embedding - centres on a predefined list of appraisal markers indicating contraction or expansion. We look at the relative frequency of these markers and at their role in the ongoing debate. The results show that there are specific markers that dominate Twitter discourse: adversative “but”, negative “no”/“not”, and cognitive verbs like “know” and “think”. A closer analysis of concordances of negatives and cognitive verbs shows that it is possible to identify patterns that are clear signals of explicit denials, whether in representing a position or rejecting it, and that the verbs are used as markers of ideological positioning. Twitter thus turns out to be characterised by positioning that emphasises contrasting views and denial of other positions. (302 words).
2023
- The Origins of the Alleged Correlation between Vaccines and Autism. A Semiotic Approach
[Articolo su rivista]
Cosenza, Giovanna; Sanna, Leonardo
abstract
Our approach to the epistemology of post-truth is based on the idea that to fully comprehend any post-truth, going back to its origins (i.e., to the moment in which some faulty interpretations start to spread) can be not only relevant but illuminating.
One of the most renowned cases of post-truth concerns vaccines and their alleged relationship with autism. It all started in 1998, when The Lancet published a study suggesting a link between the measles, mumps, and rubella vaccine and some symptoms of autism. The case is relevant both because it is at the origins of contemporary anti-vaccinism and because it took twelve years to fully disprove what in 1998 was presented as a scientific truth: this means that the boundary between truth and falsehood has been blurred for a long period of time.
For this purpose, we have applied the semiotic methodology to 20 articles from The Independent, 20 from The Telegraph, 20 from The Guardian, and 20 from The Daily Mail, published between 1998 and 2010. Unexpectedly, many elements that can be seen as ‘post-truth seeds’, such as conspiracy theories and the joint presentation of multiple truths, have been found even in the most scientifically accurate newspapers
2022
- Exploring the echo chamber concept: A linguistic perspective
[Capitolo/Saggio]
Bondi, Marina; Sanna, Leonardo
abstract
Fake news and misinformation are a key topic when discussing social media analysis research. Special attention has been paid to how social media discourse, rather than focusing on the correct identification of sources and voices, can end up constructing trust and credibility by emphasizing shared identities and positions, usually in opposition to other views. Studies on “echo chambers” look at how the views of others are systematically rejected and used instrumentally to support one’s own beliefs. Twitter discourse is often a case in point. The focus of our analysis is on the language that manifests the writer’s position, starting from the concept of engagement as defined in Martin and White’s (2005) appraisal framework. This indicates the speaker’s degree of commitment to what is being expressed and manifests the speaker’s attitudes to opening and closing the dialogic space for external views. Using a corpus of tweets and one of journalistic texts on the pandemic, we test the hypothesis that the space given to dialogic contraction on Twitter may be wider than that provided by traditional journalism. The study - based on frequency analysis, concordance analysis and word embedding - centers on a predefined list of appraisal markers indicating contraction or expansion. We look at the relative frequency of these markers and at their role in the ongoing debate. The results show that there are specific markers that dominate Twitter discourse: adversative “but”, negative “no”/”not” and cognitive verbs like “know” and “think”. A closer analysis of concordances of negatives and cognitive verbs shows that, it is possible to identify patterns that are clear signals of explicit denials, whether in representing a position or rejecting it, and that the verbs are used as markers of ideological positioning. Twitter thus turns out to be characterized by positioning that emphasizes contrasting views and denial of other positions.
2020
- Data–driven Semiotics and Semiotics–driven Machine Learning
[Articolo su rivista]
Sanna, Leonardo
abstract
Nowadays there is a huge and growing variety of digital data. Despite
the obvious relevance for the humanities and the social sciences, these massive
quantities of data, usually defined as “big data”, are mainly selected and ana-
lyzed using computer science and statistics. The paper proposes a theoretical and
practical approach to the analysis of large quantities of data within the field of
semiotic analysis. The main claim is that semiotics should dialogue with IT and
statistics, that are essential to deal with the vastness and continuous variability of
data. In particular, machine learning might become really useful from a semiotic
perspective. In this work, we use a machine learning technique that is used in
Natural Language Processing (NLP), to create a vector space based on probabilities
of co–occurrences of words. In a distributional semantics perspective, this space is
interpreted as a representation of semantic relations among words. We present
then two directions in which we could intend the joint effort of semiotics and
machine learning. In the first case, we propose a case study of semiotics–driven
machine learning, in which we create a dataset starting from a semiotic analysis.
In the second case, we present an example of data–driven semiotics, were the
semiotic tools are used on an existing dataset, that was not build with semiotic
scopes. The two directions have not to be intended as a dichotomy but instead
as a part of a joint effort where semiotics interacts with machine learning and
machine learning interacts with qualitative analysis.
2020
- Implementing Eco’s Model Reader with Word Embeddings. An Experiment on Facebook Ideological Bots
[Relazione in Atti di Convegno]
Sanna, Leonardo; Compagno, Dario
abstract
In semiotics, the concept of model reader is used to describe the felicity conditions of a text, that is, the information and pragmatic competence needed to interpret the text with reference to a hypothesis on its producer's intention. The model reader permits to formulate inferences about the implicit content of sentences and of the entire text. In this paper we propose to formalize the model reader as a function that takes as inputs a text and a larger context and produces as output what is needed to complete the text's implicit information, filling up its “blank spaces”. One possible technique to implement this function is word embedding. We performed an experiment in this sense, using the data collected and analyzed by the Tracking Exposed group (TREX) during the Italian 2018 elections. For their study, six blank Facebook profiles were created, each characterized by a political orientation: all profiles followed the same common set of 30 pages, representative of the entire Italian political spectrum at the time, but each profile interacted only with content linked to its distinctive political orientation. TREX's study of the profiles' newsfeeds demonstrated that each profile was prompted with an uneven distribution of information sources, biased by its political orientation. For our study, we created six different word spaces, one for each profile. Then we identified a certain number of politically neutral terms and observed the semantic associations of these terms in each word space. To identify the terms, we performed a classification of the entire corpus with the software Iramuteq and selected the most significant terms associated with each cluster. Finally, by performing some operations within each word space, we observed some differences in semantic associations that are coherent with the political orientation of the corresponding profile. These results appear to show that word embedding is a valuable approach for computational text pragmatics, as they can help to model the inferences performed by a certain reader. Also, these results suggest the pertinence of such analyses for the study of filter bubbles resulting from algorithmic personalization.
2020
- YTTREX: crowdsourced analysis of YouTube’s recommender system during COVID-19 pandemic
[Relazione in Atti di Convegno]
Sanna, Leonardo; Romano, Salvatore; Corona, Giulia; Agosti, Claudio
abstract
Algorithmic personalization is difficult to approach because it entails studying many different user experiences, with a lot of variables outside of our control. Two common biases are frequent in experiments: relying on corporate service API and using synthetic profiles with small regards of regional and individualized profiling and personalization. In this work, we present the result
of the first crowdsourced data collections of YouTube's recommended videos via YouTube Tracking Exposed (YTTREX). Our tool collects evidence of algorithmic personalization via an HTML parser, anonymizing the users. In our experiment we used a BBC video about COVID-19, taking into account 5 regional BBC channels in 5 different languages and we saved the recommended videos that were shown during each session. Each user watched the first five second of the videos, while the extension captured the recommended videos.
We took into account the top-20 recommended videos for each completed session, looking for evidence of algorithmic personalization. Our results showed that the vast majority of videos were recommended only once in our experiment. Moreover, we collected evidence that there is a significant difference between the videos we could retrieve using the official API and what we collected with our extension. These findings show that filter bubbles exist and that they need to be investigated with a crowdsourced approach.