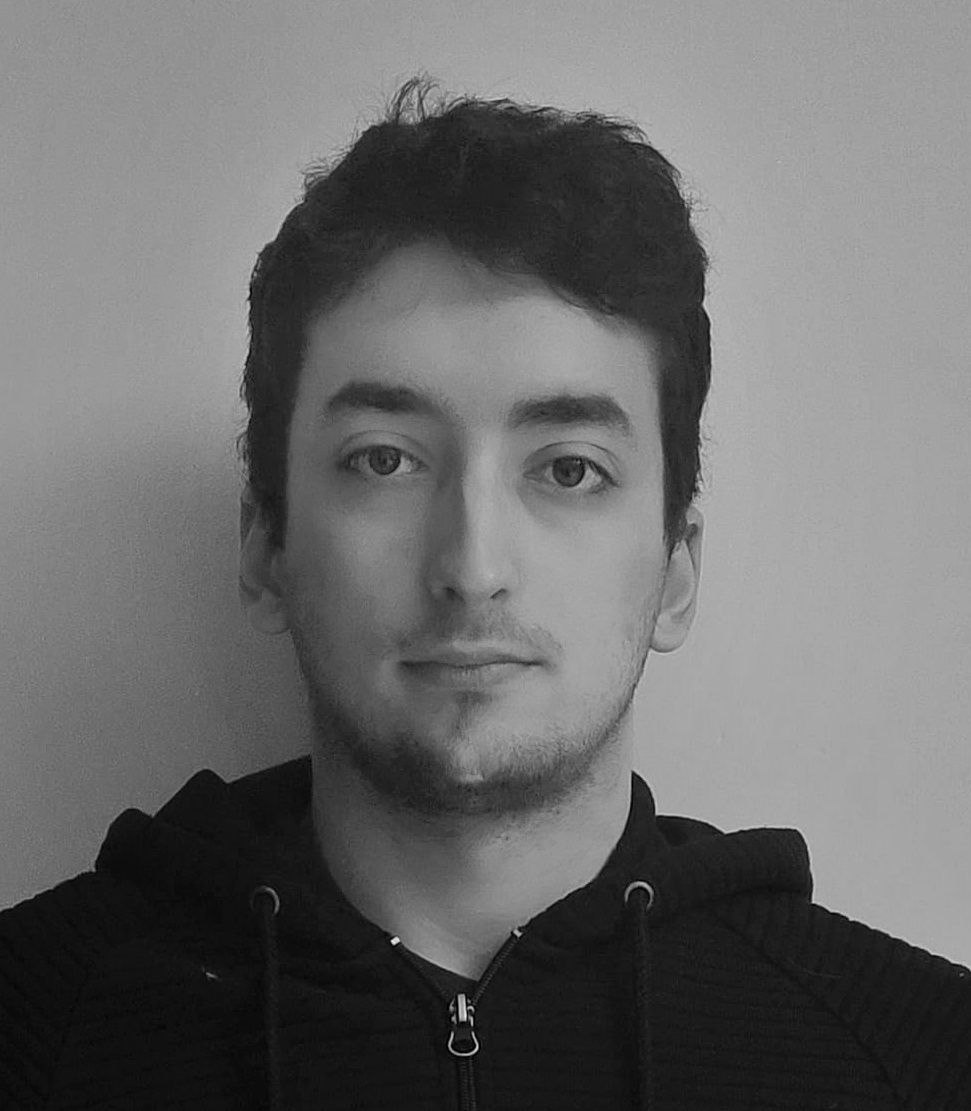 |
LUCA LUMETTI
Dottorando Dipartimento di Ingegneria "Enzo Ferrari"
|
Home |
Curriculum(pdf) |
Pubblicazioni
2024
- A State-of-the-Art Review with Code about Connected Components Labeling on GPUs
[Articolo su rivista]
Bolelli, Federico; Allegretti, Stefano; Lumetti, Luca; Grana, Costantino
abstract
This article is about Connected Components Labeling (CCL) algorithms developed for GPU accelerators. The task itself is employed in many modern image-processing pipelines and represents a fundamental step in different scenarios, whenever object recognition is required. For this reason, a strong effort in the development of many different proposals devoted to improving algorithm performance using different kinds of hardware accelerators has been made. This paper focuses on GPU-based algorithmic solutions published in the last two decades, highlighting their distinctive traits and the improvements they leverage. The state-of-the-art review proposed is equipped with the source code, which allows to straightforwardly reproduce all the algorithms in different experimental settings. A comprehensive evaluation on multiple environments is also provided, including different operating systems, compilers, and GPUs.
Our assessments are performed by means of several tests, including real-case images and synthetically generated ones, highlighting the strengths and weaknesses of each proposal. Overall, the experimental results revealed that block-based oriented algorithms outperform all the other algorithmic solutions on both 2D images and 3D volumes, regardless of the selected environment.
2024
- Enhancing Patch-Based Learning for the Segmentation of the Mandibular Canal
[Articolo su rivista]
Lumetti, Luca; Pipoli, Vittorio; Bolelli, Federico; Ficarra, Elisa; Grana, Costantino
abstract
Segmentation of the Inferior Alveolar Canal (IAC) is a critical aspect of dentistry and maxillofacial imaging, garnering considerable attention in recent research endeavors. Deep learning techniques have shown promising results in this domain, yet their efficacy is still significantly hindered by the limited availability of 3D maxillofacial datasets. An inherent challenge is posed by the size of input volumes, which necessitates a patch-based processing approach that compromises the neural network performance due to the absence of global contextual information. This study introduces a novel approach that harnesses the spatial information within the extracted patches and incorporates it into a Transformer architecture, thereby enhancing the segmentation process through the use of prior knowledge about the patch location. Our method significantly improves the Dice score by a factor of 4 points, with respect to the previous work proposed by Cipriano et al., while also reducing the training steps required by the entire pipeline. By integrating spatial information and leveraging the power of Transformer architectures, this research not only advances the accuracy of IAC segmentation, but also streamlines the training process, offering a promising direction for improving dental and maxillofacial image analysis.
2023
- Annotating the Inferior Alveolar Canal: the Ultimate Tool
[Relazione in Atti di Convegno]
Lumetti, Luca; Pipoli, Vittorio; Bolelli, Federico; Grana, Costantino
abstract
The Inferior Alveolar Nerve (IAN) is of main interest in the maxillofacial field, as an accurate localization of such nerve reduces the risks of injury during surgical procedures. Although recent literature has focused on developing novel deep learning techniques to produce accurate segmentation masks of the canal containing the IAN, there are still strong limitations due to the scarce amount of publicly available 3D maxillofacial datasets. In this paper, we present an improved version of a previously released tool, IACAT (Inferior Alveolar Canal Annotation Tool), today used by medical experts to produce 3D ground truth annotation. In addition, we release a new dataset, ToothFairy, which is part of the homonymous MICCAI2023 challenge hosted by the Grand-Challenge platform, as an extension of the previously released Maxillo dataset, which was the only publicly available. With ToothFairy, the number of annotations has been increased as well as the quality of existing data.
2023
- Buffer-MIL: Robust Multi-instance Learning with a Buffer-Based Approach
[Relazione in Atti di Convegno]
Bontempo, G.; Lumetti, L.; Porrello, A.; Bolelli, F.; Calderara, S.; Ficarra, E.
abstract
Histopathological image analysis is a critical area of research with the potential to aid pathologists in faster and more accurate diagnoses. However, Whole-Slide Images (WSIs) present challenges for deep learning frameworks due to their large size and lack of pixel-level annotations. Multi-Instance Learning (MIL) is a popular approach that can be employed for handling WSIs, treating each slide as a bag composed of multiple patches or instances. In this work we propose Buffer-MIL, which aims at tackling the covariate shift and class imbalance characterizing most of the existing histopathological datasets.
With this goal, a buffer containing the most representative instances of each disease-positive slide of the training set is incorporated into our model. An attention mechanism is then used to compare all the instances against the buffer, to find the most critical ones in a given slide. We evaluate Buffer-MIL on two publicly available WSI datasets, Camelyon16 and TCGA lung cancer, outperforming current state-of-the-art models by 2.2% of accuracy on Camelyon16.
2023
- Inferior Alveolar Canal Automatic Detection with Deep Learning CNNs on CBCTs: Development of a Novel Model and Release of Open-Source Dataset and Algorithm
[Articolo su rivista]
Di Bartolomeo, Mattia; Pellacani, Arrigo; Bolelli, Federico; Cipriano, Marco; Lumetti, Luca; Negrello, Sara; Allegretti, Stefano; Minafra, Paolo; Pollastri, Federico; Nocini, Riccardo; Colletti, Giacomo; Chiarini, Luigi; Grana, Costantino; Anesi, Alexandre
abstract
Introduction: The need of accurate three-dimensional data of anatomical structures is increasing in the surgical field. The development of convolutional neural networks (CNNs) has been helping to fill this gap by trying to provide efficient tools to clinicians. Nonetheless, the lack of a fully accessible datasets and open-source algorithms is slowing the improvements in this field. In this paper, we focus on the fully automatic segmentation of the Inferior Alveolar Canal (IAC), which is of immense interest in the dental and maxillo-facial surgeries. Conventionally, only a bidimensional annotation of the IAC is used in common clinical practice. A reliable convolutional neural network (CNNs) might be timesaving in daily practice and improve the quality of assistance. Materials and methods: Cone Beam Computed Tomography (CBCT) volumes obtained from a single radiological center using the same machine were gathered and annotated. The course of the IAC was annotated on the CBCT volumes. A secondary dataset with sparse annotations and a primary dataset with both dense and sparse annotations were generated. Three separate experiments were conducted in order to evaluate the CNN. The IoU and Dice scores of every experiment were recorded as the primary endpoint, while the time needed to achieve the annotation was assessed as the secondary end-point. Results: A total of 347 CBCT volumes were collected, then divided into primary and secondary datasets. Among the three experiments, an IoU score of 0.64 and a Dice score of 0.79 were obtained thanks to the pre-training of the CNN on the secondary dataset and the creation of a novel deep label propagation model, followed by proper training on the primary dataset. To the best of our knowledge, these results are the best ever published in the segmentation of the IAC. The datasets is publicly available and algorithm is published as open-source software. On average, the CNN could produce a 3D annotation of the IAC in 6.33 s, compared to 87.3 s needed by the radiology technician to produce a bidimensional annotation. Conclusions: To resume, the following achievements have been reached. A new state of the art in terms of Dice score was achieved, overcoming the threshold commonly considered of 0.75 for the use in clinical practice. The CNN could fully automatically produce accurate three-dimensional segmentation of the IAC in a rapid setting, compared to the bidimensional annotations commonly used in the clinical practice and generated in a time-consuming manner. We introduced our innovative deep label propagation method to optimize the performance of the CNN in the segmentation of the IAC. For the first time in this field, the datasets and the source codes used were publicly released, granting reproducibility of the experiments and helping in the improvement of IAC segmentation.