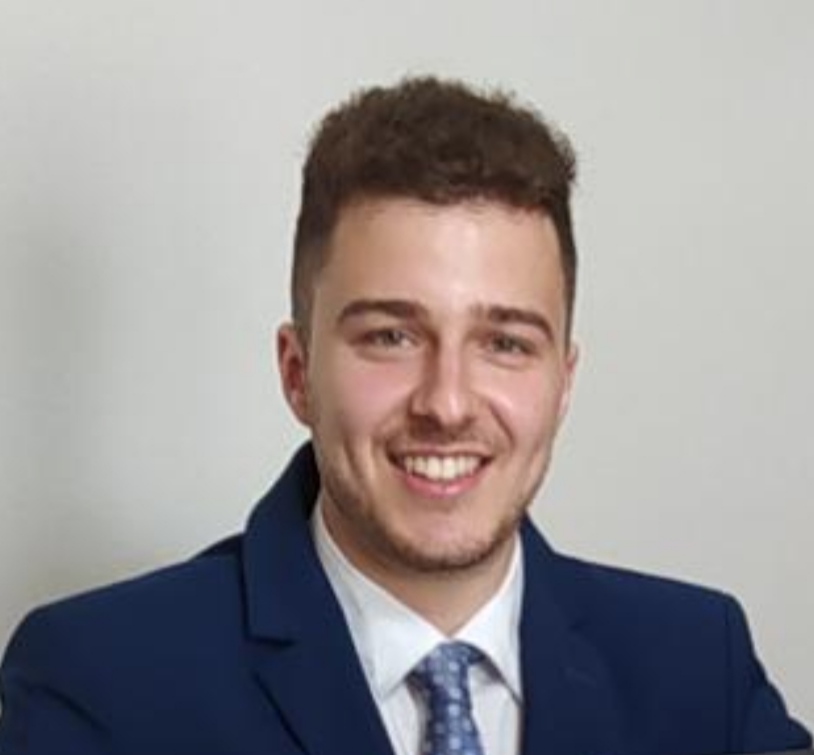 |
GIUSEPPE CARTELLA
Dottorando Dipartimento di Ingegneria "Enzo Ferrari"
|
Home |
Pubblicazioni
2024
- Trends, Applications, and Challenges in Human Attention Modelling
[Relazione in Atti di Convegno]
Cartella, Giuseppe; Cornia, Marcella; Cuculo, Vittorio; D'Amelio, Alessandro; Zanca, Dario; Boccignone, Giuseppe; Cucchiara, Rita
abstract
Human attention modelling has proven, in recent years, to be particularly useful not only for understanding the cognitive processes underlying visual exploration, but also for providing support to artificial intelligence models that aim to solve problems in various domains, including image and video processing, vision-and-language applications, and language modelling. This survey offers a reasoned overview of recent efforts to integrate human attention mechanisms into contemporary deep learning models and discusses future research directions and challenges.
2024
- Unveiling the Truth: Exploring Human Gaze Patterns in Fake Images
[Articolo su rivista]
Cartella, Giuseppe; Cuculo, Vittorio; Cornia, Marcella; Cucchiara, Rita
abstract
Creating high-quality and realistic images is now possible thanks to the impressive advancements in image generation. A description in natural language of your desired output is all you need to obtain breathtaking results. However, as the use of generative models grows, so do concerns about the propagation of malicious content and misinformation. Consequently, the research community is actively working on the development of novel fake detection techniques, primarily focusing on low-level features and possible fingerprints left by generative models during the image generation process. In a different vein, in our work, we leverage human semantic knowledge to investigate the possibility of being included in frameworks of fake image detection. To achieve this, we collect a novel dataset of partially manipulated images using diffusion models and conduct an eye-tracking experiment to record the eye movements of different observers while viewing real and fake stimuli. A preliminary statistical analysis is conducted to explore the distinctive patterns in how humans perceive genuine and altered images. Statistical findings reveal that, when perceiving counterfeit samples, humans tend to focus on more confined regions of the image, in contrast to the more dispersed observational pattern observed when viewing genuine images. Our dataset is publicly available at: https://github.com/aimagelab/unveiling-the-truth.
2023
- LaDI-VTON: Latent Diffusion Textual-Inversion Enhanced Virtual Try-On
[Relazione in Atti di Convegno]
Morelli, Davide; Baldrati, Alberto; Cartella, Giuseppe; Cornia, Marcella; Bertini, Marco; Cucchiara, Rita
abstract
The rapidly evolving fields of e-commerce and metaverse continue to seek innovative approaches to enhance the consumer experience. At the same time, recent advancements in the development of diffusion models have enabled generative networks to create remarkably realistic images. In this context, image-based virtual try-on, which consists in generating a novel image of a target model wearing a given in-shop garment, has yet to capitalize on the potential of these powerful generative solutions. This work introduces LaDI-VTON, the first Latent Diffusion textual Inversion-enhanced model for the Virtual Try-ON task. The proposed architecture relies on a latent diffusion model extended with a novel additional autoencoder module that exploits learnable skip connections to enhance the generation process preserving the model's characteristics. To effectively maintain the texture and details of the in-shop garment, we propose a textual inversion component that can map the visual features of the garment to the CLIP token embedding space and thus generate a set of pseudo-word token embeddings capable of conditioning the generation process. Experimental results on Dress Code and VITON-HD datasets demonstrate that our approach outperforms the competitors by a consistent margin, achieving a significant milestone for the task.
2023
- Multimodal Garment Designer: Human-Centric Latent Diffusion Models for Fashion Image Editing
[Relazione in Atti di Convegno]
Baldrati, Alberto; Morelli, Davide; Cartella, Giuseppe; Cornia, Marcella; Bertini, Marco; Cucchiara, Rita
abstract
2023
- OpenFashionCLIP: Vision-and-Language Contrastive Learning with Open-Source Fashion Data
[Relazione in Atti di Convegno]
Cartella, Giuseppe; Baldrati, Alberto; Morelli, Davide; Cornia, Marcella; Bertini, Marco; Cucchiara, Rita
abstract
The inexorable growth of online shopping and e-commerce demands scalable and robust machine learning-based solutions to accommodate customer requirements. In the context of automatic tagging classification and multimodal retrieval, prior works either defined a low generalizable supervised learning approach or more reusable CLIP-based techniques while, however, training on closed source data. In this work, we propose OpenFashionCLIP, a vision-and-language contrastive learning method that only adopts open-source fashion data stemming from diverse domains, and characterized by varying degrees of specificity. Our approach is extensively validated across several tasks and benchmarks, and experimental results highlight a significant out-of-domain generalization capability and consistent improvements over state-of-the-art methods both in terms of accuracy and recall. Source code and trained models are publicly available at: https://github.com/aimagelab/open-fashion-clip.