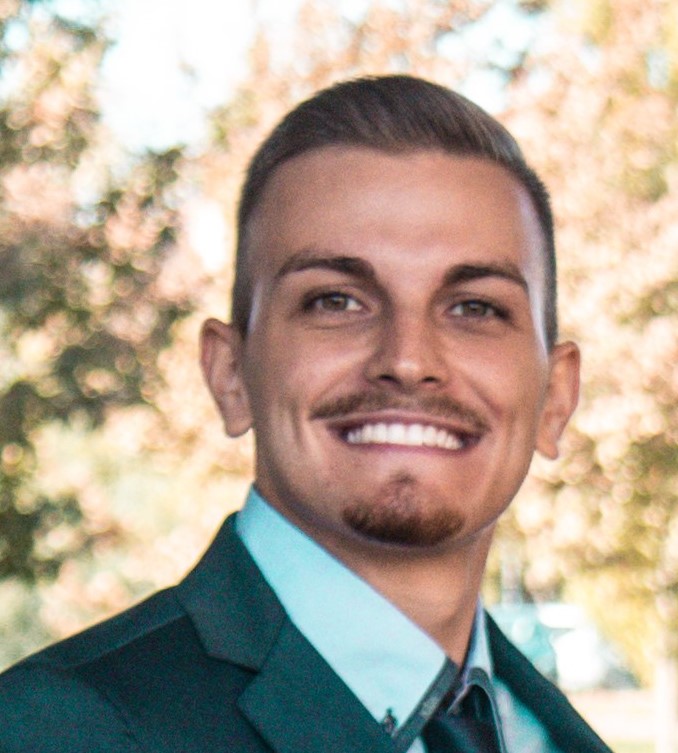 |
ROBERTO AMOROSO
Dottorando Dipartimento di Ingegneria "Enzo Ferrari"
|
Home |
Pubblicazioni
2024
- FOSSIL: Free Open-Vocabulary Semantic Segmentation through Synthetic References Retrieval
[Relazione in Atti di Convegno]
Barsellotti, Luca; Amoroso, Roberto; Baraldi, Lorenzo; Cucchiara, Rita
abstract
2024
- Parents and Children: Distinguishing Multimodal DeepFakes from Natural Images
[Articolo su rivista]
Amoroso, Roberto; Morelli, Davide; Cornia, Marcella; Baraldi, Lorenzo; Del Bimbo, Alberto; Cucchiara, Rita
abstract
2024
- Training-Free Open-Vocabulary Segmentation with Offline Diffusion-Augmented Prototype Generation
[Relazione in Atti di Convegno]
Barsellotti, Luca; Amoroso, Roberto; Cornia, Marcella; Baraldi, Lorenzo; Cucchiara, Rita
abstract
2024
- What’s Outside the Intersection? Fine-grained Error Analysis for Semantic Segmentation Beyond IoU
[Relazione in Atti di Convegno]
Bernhard, Maximilian; Amoroso, Roberto; Kindermann, Yannic; Baraldi, Lorenzo; Cucchiara, Rita; Tresp, Volker; Schubert, Matthias
abstract
2023
- Enhancing Open-Vocabulary Semantic Segmentation with Prototype Retrieval
[Relazione in Atti di Convegno]
Barsellotti, Luca; Amoroso, Roberto; Baraldi, Lorenzo; Cucchiara, Rita
abstract
2023
- Superpixel Positional Encoding to Improve ViT-based Semantic Segmentation Models
[Relazione in Atti di Convegno]
Amoroso, Roberto; Tomei, Matteo; Baraldi, Lorenzo; Cucchiara, Rita
abstract
2022
- Investigating Bidimensional Downsampling in Vision Transformer Models
[Relazione in Atti di Convegno]
Bruno, Paolo; Amoroso, Roberto; Cornia, Marcella; Cascianelli, Silvia; Baraldi, Lorenzo; Cucchiara, Rita
abstract
Vision Transformers (ViT) and other Transformer-based architectures for image classification have achieved promising performances in the last two years. However, ViT-based models require large datasets, memory, and computational power to obtain state-of-the-art results compared to more traditional architectures. The generic ViT model, indeed, maintains a full-length patch sequence during inference, which is redundant and lacks hierarchical representation. With the goal of increasing the efficiency of Transformer-based models, we explore the application of a 2D max-pooling operator on the outputs of Transformer encoders. We conduct extensive experiments on the CIFAR-100 dataset and the large ImageNet dataset and consider both accuracy and efficiency metrics, with the final goal of reducing the token sequence length without affecting the classification performance. Experimental results show that bidimensional downsampling can outperform previous classification approaches while requiring relatively limited computation resources.
2021
- Assessing the Role of Boundary-level Objectives in Indoor Semantic Segmentation
[Relazione in Atti di Convegno]
Amoroso, Roberto; Baraldi, Lorenzo; Cucchiara, Rita
abstract
Providing fine-grained and accurate segmentation maps of indoor scenes is a challenging task with relevant applications in the fields of augmented reality, image retrieval, and personalized robotics. While most of the recent literature on semantic segmentation has focused on outdoor scenarios, the generation of accurate indoor segmentation maps has been partially under-investigated. With the goal of increasing the accuracy of semantic segmentation in indoor scenarios, we focus on the analysis of boundary-level objectives, which foster the generation of fine-grained boundaries between different semantic classes and which have never been explored in the case of indoor segmentation. In particular, we test and devise variants of both the Boundary and Active Boundary losses, two recent proposals which deal with the prediction of semantic boundaries. Through experiments on the NYUDv2 dataset, we quantify the role of such losses in terms of accuracy and quality of boundary prediction and demonstrate the accuracy gain of the proposed variants.
2021
- Improving Indoor Semantic Segmentation with Boundary-level Objectives
[Relazione in Atti di Convegno]
Amoroso, Roberto; Baraldi, Lorenzo; Cucchiara, Rita
abstract
While most of the recent literature on semantic segmentation has focused on outdoor scenarios, the generation of accurate indoor segmentation maps has been partially under-investigated, although being a relevant task with applications in augmented reality, image retrieval, and personalized robotics.
With the goal of increasing the accuracy of semantic segmentation in indoor scenarios, we develop and propose two novel boundary-level training objectives, which foster the generation of accurate boundaries between different semantic classes.
In particular, we take inspiration from the Boundary and Active Boundary losses, two recent proposals which deal with the prediction of semantic boundaries, and propose modified geometric distance functions that improve predictions at the boundary level.
Through experiments on the NYUDv2 dataset, we assess the appropriateness of our proposal in terms of accuracy and quality of boundary prediction and demonstrate its accuracy gain.
2020
- Estimation of Traffic Matrices via Super-resolution and Federated Learning
[Poster]
Amoroso, Roberto; Esposito, Flavio; Merani, Maria Luisa
abstract
Network measurement and telemetry techniques are central to the management of today’s computer networks. One popular technique with several applications is the estimation of traffic matrices. Existing traffic matrix inference approaches that use statistical methods, often make assumptions on the structure of the matrix that may
be invalid. Data-driven methods, instead, often use detailed information about the network topology that may be unavailable or impractical to collect.
Inspired by the field of image processing, we propose a superresolution technique for traffic matrix inference that does not require any knowledge on the structural properties of the matrix elements to infer, nor a large data collection. Our experiments with anonymized Internet traces demonstrate that the proposed
approach can infer fine-grained network traffic with high precision outperforming existing data interpolation techniques, such as bicubic interpolation.