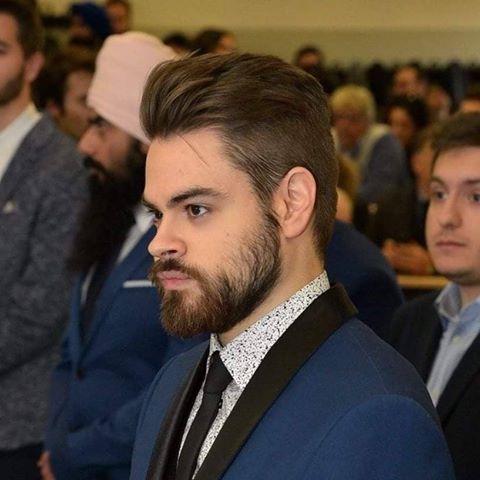 |
MATTEO FINCATO
Dottorando Dipartimento di Ingegneria "Enzo Ferrari"
|
Home |
Pubblicazioni
2022
- Dress Code: High-Resolution Multi-Category Virtual Try-On
[Relazione in Atti di Convegno]
Morelli, Davide; Fincato, Matteo; Cornia, Marcella; Landi, Federico; Cesari, Fabio; Cucchiara, Rita
abstract
2022
- Dress Code: High-Resolution Multi-Category Virtual Try-On
[Relazione in Atti di Convegno]
Morelli, Davide; Fincato, Matteo; Cornia, Marcella; Landi, Federico; Cesari, Fabio; Cucchiara, Rita
abstract
Image-based virtual try-on strives to transfer the appearance of a clothing item onto the image of a target person. Existing literature focuses mainly on upper-body clothes (e.g. t-shirts, shirts, and tops) and neglects full-body or lower-body items. This shortcoming arises from a main factor: current publicly available datasets for image-based virtual try-on do not account for this variety, thus limiting progress in the field. In this research activity, we introduce Dress Code, a novel dataset which contains images of multi-category clothes. Dress Code is more than 3x larger than publicly available datasets for image-based virtual try-on and features high-resolution paired images (1024 x 768) with front-view, full-body reference models. To generate HD try-on images with high visual quality and rich in details, we propose to learn fine-grained discriminating features. Specifically, we leverage a semantic-aware discriminator that makes predictions at pixel-level instead of image- or patch-level. The Dress Code dataset is publicly available at https://github.com/aimagelab/dress-code.
2022
- Transform, Warp, and Dress: A New Transformation-Guided Model for Virtual Try-On
[Articolo su rivista]
Fincato, Matteo; Cornia, Marcella; Landi, Federico; Cesari, Fabio; Cucchiara, Rita
abstract
Virtual try-on has recently emerged in computer vision and multimedia communities with the development of architectures that can generate realistic images of a target person wearing a custom garment. This research interest is motivated by the large role played by e-commerce and online shopping in our society. Indeed, the virtual try-on task can offer many opportunities to improve the efficiency of preparing fashion catalogs and to enhance the online user experience. The problem is far to be solved: current architectures do not reach sufficient accuracy with respect to manually generated images and can only be trained on image pairs with a limited variety. Existing virtual try-on datasets have two main limits: they contain only female models, and all the images are available only in low resolution. This not only affects the generalization capabilities of the trained architectures but makes the deployment to real applications impractical. To overcome these issues, we present Dress Code, a new dataset for virtual try-on that contains high-resolution images of a large variety of upper-body clothes and both male and female models. Leveraging this enriched dataset, we propose a new model for virtual try-on capable of generating high-quality and photo-realistic images using a three-stage pipeline. The first two stages perform two different geometric transformations to warp the desired garment and make it fit into the target person's body pose and shape. Then, we generate the new image of that same person wearing the try-on garment using a generative network. We test the proposed solution on the most widely used dataset for this task as well as on our newly collected dataset and demonstrate its effectiveness when compared to current state-of-the-art methods. Through extensive analyses on our Dress Code dataset, we show the adaptability of our model, which can generate try-on images even with a higher resolution.
2021
- VITON-GT: An Image-based Virtual Try-On Model with Geometric Transformations
[Relazione in Atti di Convegno]
Fincato, Matteo; Landi, Federico; Cornia, Marcella; Cesari, Fabio; Cucchiara, Rita
abstract