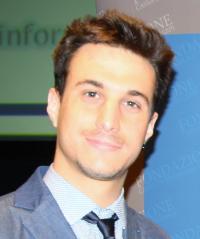 |
FEDERICO LANDI
|
Home |
Pubblicazioni
2022
- Dress Code: High-Resolution Multi-Category Virtual Try-On
[Relazione in Atti di Convegno]
Morelli, Davide; Fincato, Matteo; Cornia, Marcella; Landi, Federico; Cesari, Fabio; Cucchiara, Rita
abstract
Image-based virtual try-on strives to transfer the appearance of a clothing item onto the image of a target person. Existing literature focuses mainly on upper-body clothes (e.g. t-shirts, shirts, and tops) and neglects full-body or lower-body items. This shortcoming arises from a main factor: current publicly available datasets for image-based virtual try-on do not account for this variety, thus limiting progress in the field. In this research activity, we introduce Dress Code, a novel dataset which contains images of multi-category clothes. Dress Code is more than 3x larger than publicly available datasets for image-based virtual try-on and features high-resolution paired images (1024 x 768) with front-view, full-body reference models. To generate HD try-on images with high visual quality and rich in details, we propose to learn fine-grained discriminating features. Specifically, we leverage a semantic-aware discriminator that makes predictions at pixel-level instead of image- or patch-level. The Dress Code dataset is publicly available at https://github.com/aimagelab/dress-code.
2022
- Dress Code: High-Resolution Multi-Category Virtual Try-On
[Relazione in Atti di Convegno]
Morelli, Davide; Fincato, Matteo; Cornia, Marcella; Landi, Federico; Cesari, Fabio; Cucchiara, Rita
abstract
2022
- Embodied Navigation at the Art Gallery
[Relazione in Atti di Convegno]
Bigazzi, Roberto; Landi, Federico; Cascianelli, Silvia; Cornia, Marcella; Baraldi, Lorenzo; Cucchiara, Rita
abstract
Embodied agents, trained to explore and navigate indoor photorealistic environments, have achieved impressive results on standard datasets and benchmarks. So far, experiments and evaluations have involved domestic and working scenes like offices, flats, and houses. In this paper, we build and release a new 3D space with unique characteristics: the one of a complete art museum. We name this environment ArtGallery3D (AG3D). Compared with existing 3D scenes, the collected space is ampler, richer in visual features, and provides very sparse occupancy information. This feature is challenging for occupancy-based agents which are usually trained in crowded domestic environments with plenty of occupancy information. Additionally, we annotate the coordinates of the main points of interest inside the museum, such as paintings, statues, and other items. Thanks to this manual process, we deliver a new benchmark for PointGoal navigation inside this new space. Trajectories in this dataset are far more complex and lengthy than existing ground-truth paths for navigation in Gibson and Matterport3D. We carry on extensive experimental evaluation using our new space for evaluation and prove that existing methods hardly adapt to this scenario. As such, we believe that the availability of this 3D model will foster future research and help improve existing solutions.
2022
- Focus on Impact: Indoor Exploration with Intrinsic Motivation
[Articolo su rivista]
Bigazzi, Roberto; Landi, Federico; Cascianelli, Silvia; Baraldi, Lorenzo; Cornia, Marcella; Cucchiara, Rita
abstract
2022
- Spot the Difference: A Novel Task for Embodied Agents in Changing Environments
[Relazione in Atti di Convegno]
Landi, Federico; Bigazzi, Roberto; Cornia, Marcella; Cascianelli, Silvia; Baraldi, Lorenzo; Cucchiara, Rita
abstract
2022
- Transform, Warp, and Dress: A New Transformation-Guided Model for Virtual Try-On
[Articolo su rivista]
Fincato, Matteo; Cornia, Marcella; Landi, Federico; Cesari, Fabio; Cucchiara, Rita
abstract
Virtual try-on has recently emerged in computer vision and multimedia communities with the development of architectures that can generate realistic images of a target person wearing a custom garment. This research interest is motivated by the large role played by e-commerce and online shopping in our society. Indeed, the virtual try-on task can offer many opportunities to improve the efficiency of preparing fashion catalogs and to enhance the online user experience. The problem is far to be solved: current architectures do not reach sufficient accuracy with respect to manually generated images and can only be trained on image pairs with a limited variety. Existing virtual try-on datasets have two main limits: they contain only female models, and all the images are available only in low resolution. This not only affects the generalization capabilities of the trained architectures but makes the deployment to real applications impractical. To overcome these issues, we present Dress Code, a new dataset for virtual try-on that contains high-resolution images of a large variety of upper-body clothes and both male and female models. Leveraging this enriched dataset, we propose a new model for virtual try-on capable of generating high-quality and photo-realistic images using a three-stage pipeline. The first two stages perform two different geometric transformations to warp the desired garment and make it fit into the target person's body pose and shape. Then, we generate the new image of that same person wearing the try-on garment using a generative network. We test the proposed solution on the most widely used dataset for this task as well as on our newly collected dataset and demonstrate its effectiveness when compared to current state-of-the-art methods. Through extensive analyses on our Dress Code dataset, we show the adaptability of our model, which can generate try-on images even with a higher resolution.
2021
- Explore and Explain: Self-supervised Navigation and Recounting
[Relazione in Atti di Convegno]
Bigazzi, Roberto; Landi, Federico; Cornia, Marcella; Cascianelli, Silvia; Baraldi, Lorenzo; Cucchiara, Rita
abstract
Embodied AI has been recently gaining attention as it aims to foster the development of autonomous and intelligent agents. In this paper, we devise a novel embodied setting in which an agent needs to explore a previously unknown environment while recounting what it sees during the path. In this context, the agent needs to navigate the environment driven by an exploration goal, select proper moments for description, and output natural language descriptions of relevant objects and scenes. Our model integrates a novel self-supervised exploration module with penalty, and a fully-attentive captioning model for explanation. Also, we investigate different policies for selecting proper moments for explanation, driven by information coming from both the environment and the navigation. Experiments are conducted on photorealistic environments from the Matterport3D dataset and investigate the navigation and explanation capabilities of the agent as well as the role of their interactions.
2021
- Multimodal Attention Networks for Low-Level Vision-and-Language Navigation
[Articolo su rivista]
Landi, Federico; Baraldi, Lorenzo; Cornia, Marcella; Corsini, Massimiliano; Cucchiara, Rita
abstract
Vision-and-Language Navigation (VLN) is a challenging task in which an agent needs to follow a language-specified path to reach a target destination. The goal gets even harder as the actions available to the agent get simpler and move towards low-level, atomic interactions with the environment. This setting takes the name of low-level VLN. In this paper, we strive for the creation of an agent able to tackle three key issues: multi-modality, long-term dependencies, and adaptability towards different locomotive settings. To that end, we devise "Perceive, Transform, and Act" (PTA): a fully-attentive VLN architecture that leaves the recurrent approach behind and the first Transformer-like architecture incorporating three different modalities -- natural language, images, and low-level actions for the agent control. In particular, we adopt an early fusion strategy to merge lingual and visual information efficiently in our encoder. We then propose to refine the decoding phase with a late fusion extension between the agent's history of actions and the perceptual modalities. We experimentally validate our model on two datasets: PTA achieves promising results in low-level VLN on R2R and achieves good performance in the recently proposed R4R benchmark. Our code is publicly available at https://github.com/aimagelab/perceive-transform-and-act.
2021
- Out of the Box: Embodied Navigation in the Real World
[Relazione in Atti di Convegno]
Bigazzi, Roberto; Landi, Federico; Cornia, Marcella; Cascianelli, Silvia; Baraldi, Lorenzo; Cucchiara, Rita
abstract
The research field of Embodied AI has witnessed substantial progress in visual navigation and exploration thanks to powerful simulating platforms and the availability of 3D data of indoor and photorealistic environments. These two factors have opened the doors to a new generation of intelligent agents capable of achieving nearly perfect PointGoal Navigation. However, such architectures are commonly trained with millions, if not billions, of frames and tested in simulation. Together with great enthusiasm, these results yield a question: how many researchers will effectively benefit from these advances?
In this work, we detail how to transfer the knowledge acquired in simulation into the real world. To that end, we describe the architectural discrepancies that damage the Sim2Real adaptation ability of models trained on the Habitat simulator and propose a novel solution tailored towards the deployment in real-world scenarios. We then deploy our models on a LoCoBot, a Low-Cost Robot equipped with a single Intel RealSense camera. Different from previous work, our testing scene is unavailable to the agent in simulation. The environment is also inaccessible to the agent beforehand, so it cannot count on scene-specific semantic priors. In this way, we reproduce a setting in which a research group (potentially from other fields) needs to employ the agent visual navigation capabilities as-a-Service. Our experiments indicate that it is possible to achieve satisfying results when deploying the obtained model in the real world.
2021
- VITON-GT: An Image-based Virtual Try-On Model with Geometric Transformations
[Relazione in Atti di Convegno]
Fincato, Matteo; Landi, Federico; Cornia, Marcella; Cesari, Fabio; Cucchiara, Rita
abstract
2021
- Working Memory Connections for LSTM
[Articolo su rivista]
Landi, Federico; Baraldi, Lorenzo; Cornia, Marcella; Cucchiara, Rita
abstract
2019
- Embodied Vision-and-Language Navigation with Dynamic Convolutional Filters
[Relazione in Atti di Convegno]
Landi, Federico; Baraldi, Lorenzo; Corsini, Massimiliano; Cucchiara, Rita
abstract
In Vision-and-Language Navigation (VLN), an embodied agent needs to reach a target destination with the only guidance of a natural language instruction. To explore the environment and progress towards the target location, the agent must perform a series of low-level actions, such as rotate, before stepping ahead. In this paper, we propose to exploit dynamic convolutional filters to encode the visual information and the lingual description in an efficient way. Differently from some previous works that abstract from the agent perspective and use high-level navigation spaces, we design a policy which decodes the information provided by dynamic convolution into a series of low-level, agent friendly actions. Results show that our model exploiting dynamic filters performs better than other architectures with traditional convolution, being the new state of the art for embodied VLN in the low-level action space. Additionally, we attempt to categorize recent work on VLN depending on their architectural choices and distinguish two main groups: we call them low-level actions and high-level actions models. To the best of our knowledge, we are the first to propose this analysis and categorization for VLN.