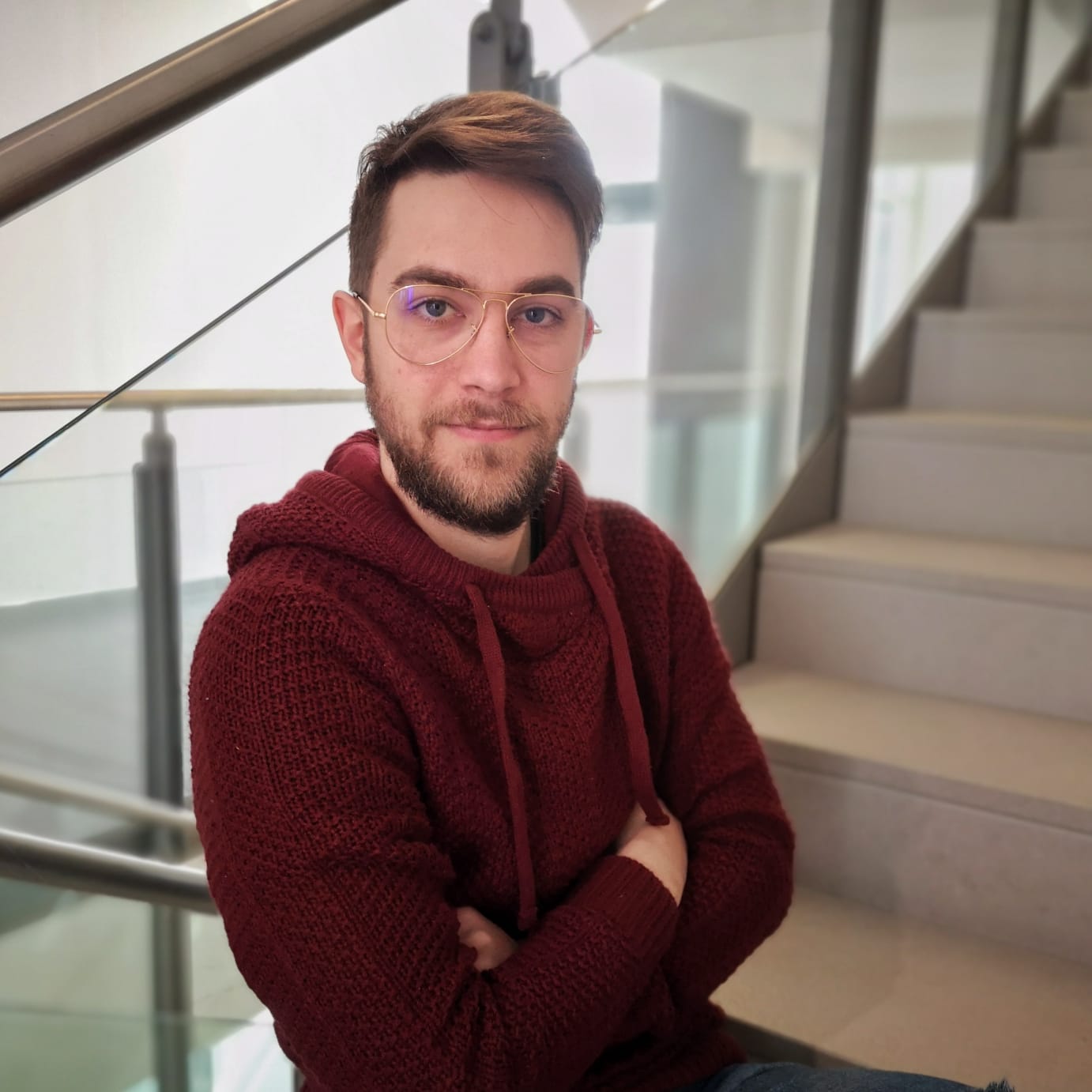 |
SAMUELE POPPI
Dottorando Dipartimento di Ingegneria "Enzo Ferrari"
|
Home |
Pubblicazioni
2024
- Multi-Class Unlearning for Image Classification via Weight Filtering
[Articolo su rivista]
Poppi, Samuele; Sarto, Sara; Cornia, Marcella; Baraldi, Lorenzo; Cucchiara, Rita
abstract
Machine Unlearning is an emerging paradigm for selectively removing the impact of training datapoints from a network. Unlike existing methods that target a limited subset or a single class, our framework unlearns all classes in a single round. We achieve this by modulating the network's components using memory matrices, enabling the network to demonstrate selective unlearning behavior for any class after training. By discovering weights that are specific to each class, our approach also recovers a representation of the classes which is explainable by design. We test the proposed framework on small- and medium-scale image classification datasets, with both convolution- and Transformer-based backbones, showcasing the potential for explainable solutions through unlearning.
2024
- Safe-CLIP: Removing NSFW Concepts from Vision-and-Language Models
[Relazione in Atti di Convegno]
Poppi, Samuele; Poppi, Tobia; Cocchi, Federico; Cornia, Marcella; Baraldi, Lorenzo; Cucchiara, Rita
abstract
Large-scale vision-and-language models, such as CLIP, are typically trained on web-scale data, which can introduce inappropriate content and lead to the development of unsafe and biased behavior. This, in turn, hampers their applicability in sensitive and trustworthy contexts and could raise significant concerns in their adoption. Our research introduces a novel approach to enhancing the safety of vision-and-language models by diminishing their sensitivity to NSFW (not safe for work) inputs. In particular, our methodology seeks to sever "toxic" linguistic and visual concepts, unlearning the linkage between unsafe linguistic or visual items and unsafe regions of the embedding space. We show how this can be done by fine-tuning a CLIP model on synthetic data obtained from a large language model trained to convert between safe and unsafe sentences, and a text-to-image generator. We conduct extensive experiments on the resulting embedding space for cross-modal retrieval, text-to-image, and image-to-text generation, where we show that our model can be remarkably employed with pre-trained generative models. Our source code and trained models are available at: https://github.com/aimagelab/safe-clip.
2023
- Towards Explainable Navigation and Recounting
[Relazione in Atti di Convegno]
Poppi, Samuele; Rawal, Niyati; Bigazzi, Roberto; Cornia, Marcella; Cascianelli, Silvia; Baraldi, Lorenzo; Cucchiara, Rita
abstract
Explainability and interpretability of deep neural networks have become of crucial importance over the years in Computer Vision, concurrently with the need to understand increasingly complex models. This necessity has fostered research on approaches that facilitate human comprehension of neural methods. In this work, we propose an explainable setting for visual navigation, in which an autonomous agent needs to explore an unseen indoor environment while portraying and explaining interesting scenes with natural language descriptions. We combine recent advances in ongoing research fields, employing an explainability method on images generated through agent-environment interaction. Our approach uses explainable maps to visualize model predictions and highlight the correlation between the observed entities and the generated words, to focus on prominent objects encountered during the environment exploration. The experimental section demonstrates that our approach can identify the regions of the images that the agent concentrates on to describe its point of view, improving explainability.
2023
- Unveiling the Impact of Image Transformations on Deepfake Detection: An Experimental Analysis
[Relazione in Atti di Convegno]
Cocchi, Federico; Baraldi, Lorenzo; Poppi, Samuele; Cornia, Marcella; Baraldi, Lorenzo; Cucchiara, Rita
abstract
With the recent explosion of interest in visual Generative AI, the field of deepfake detection has gained a lot of attention. In fact, deepfake detection might be the only measure to counter the potential proliferation of generated media in support of fake news and its consequences. While many of the available works limit the detection to a pure and direct classification of fake versus real, this does not translate well to a real-world scenario. Indeed, malevolent users can easily apply post-processing techniques to generated content, changing the underlying distribution of fake data. In this work, we provide an in-depth analysis of the robustness of a deepfake detection pipeline, considering different image augmentations, transformations, and other pre-processing steps. These transformations are only applied in the evaluation phase, thus simulating a practical situation in which the detector is not trained on all the possible augmentations that can be used by the attacker. In particular, we analyze the performance of a k-NN and a linear probe detector on the COCOFake dataset, using image features extracted from pre-trained models, like CLIP and DINO. Our results demonstrate that while the CLIP visual backbone outperforms DINO in deepfake detection with no augmentation, its performance varies significantly in presence of any transformation, favoring the robustness of DINO.
2021
- Revisiting The Evaluation of Class Activation Mapping for Explainability: A Novel Metric and Experimental Analysis
[Relazione in Atti di Convegno]
Poppi, Samuele; Cornia, Marcella; Baraldi, Lorenzo; Cucchiara, Rita
abstract