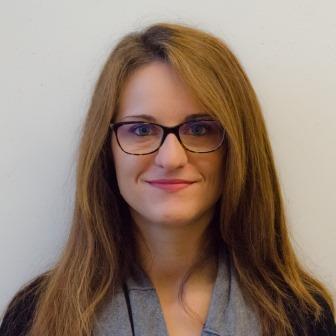 |
ROSALBA CALVINI
Ricercatore Legge 240/10 - t.det. Dipartimento di Scienze della Vita sede ex-Agraria
|
Home |
Curriculum(pdf) |
Didattica |
Pubblicazioni
2024
- Coupling randomisation and sparse modelling for the exploratory analysis of large hyperspectral datasets
[Articolo su rivista]
Calvini, R.; Amigo, J. M.
abstract
2023
- Design and application of a smartphone-based device for in vineyard determination of anthocyanins content in red grapes
[Articolo su rivista]
Menozzi, C; Calvini, R; Nigro, G; Tessarin, P; Bossio, D; Calderisi, M; Ferrari, V; Foca, G; Ulrici, A
abstract
The choice of the proper moment for harvesting is a crucial aspect in winemaking process, since the chemical attributes of grape berries strongly influence red wine quality. In particular, phenolic composition of red grapes plays a significant role in many sensory properties of wine related to color and taste. Anthocyanins are the most important phenolic compounds for red grapes: they accumulate in the grape skin during ripening, and they are responsible for the purple color of ripe berries. Routine analysis for the determination of grapes phenolic maturity includes chromatographic and spectroscopic techniques, that are time-consuming and expensive. In this work, we propose an innovative device conceived for the determination of grape phenolic maturity based on RGB images of grape berries acquired with a smartphone. The device has been designed to be used directly in the vineyard thanks to its small size and to the possibility of acquiring geolocated images of the berries under controlled lighting conditions. In this study, grape samples of three different varieties (Ancellotta, Lambrusco Salamino and Sangiovese) were collected at different harvest times from veraison to maturity and imaged by means of a common smartphone using the device. The RGB images were then converted into one-dimensional signals, named colourgrams, which codify the color properties of the images. The dataset of colourgrams was then used to calculate calibration models using Partial Least Squares (PLS) regression in order to relate color information with chemical parameters generally employed to evaluate grape phenolic maturity, such as total anthocyanins content and extractable anthocyanins content. The calibration models were implemented in a software interface that allows to acquire geolocated images of the grape samples, visualize the outcomes of the analysis, visualize maps and plots related to phenolic maturity, store data and share relevant information.
2023
- Evaluation of the potential of near infrared hyperspectral imaging for monitoring the invasive brown marmorated stink bug
[Articolo su rivista]
Ferrari, V.; Calvini, R.; Boom, B.; Menozzi, C.; Rangarajan, A. K.; Maistrello, L.; Offermans, P.; Ulrici, A.
abstract
The brown marmorated stink bug (BMSB), Halyomorpha halys, is an invasive insect pest of global importance that damages several crops, compromising agri-food production. Field monitoring procedures are fundamental to perform risk assessment operations, in order to promptly face crop infestations and avoid economical losses. To improve pest management, spectral cameras mounted on Unmanned Aerial Vehicles (UAVs) and other Internet of Things (IoT) devices, such as smart traps or unmanned ground vehicles, could be used as an innovative technology allowing fast, efficient and real-time monitoring of insect infestations.
The present study consists in a preliminary evaluation at the laboratory level of Near Infrared Hyperspectral Imaging (NIR-HSI) as a possible technology to detect BMSB specimens on different vegetal backgrounds, overcoming the problem of BMSB mimicry. Hyperspectral images of BMSB were acquired in the 980–1660 nm range, considering different vegetal backgrounds selected to mimic a real field application scene. Classification models were obtained following two different chemometric approaches. The first approach was focused on modelling spectral information and selecting relevant spectral regions for discrimination by means of sparse-based variable selection coupled with Soft Partial Least Squares Discriminant Analysis (s-Soft PLS-DA) classification algorithm. The second approach was based on modelling spatial and spectral features contained in the hyperspectral images using Convolutional Neural Networks (CNN). Finally, to further improve BMSB detection ability, the two strategies were merged, considering only the spectral regions selected by s-Soft PLS-DA for CNN modelling.
2023
- Mixture design and multivariate image analysis to monitor the colour of strawberry yoghurt purée
[Articolo su rivista]
Rolando, P. L.; Calvini, R.; Foca, G.; Ulrici, A.
abstract
Food colour is a commercial added value, since it represents the first appealing factor for consumers. In this context, this study was aimed at evaluating the effect of strawberry yoghurt purée (SYP) formulation on the corresponding colour and on its variation over time, which is mainly due to degradation and browning phenomena. To this aim, a combined approach was used that included mixture design and multivariate analysis of RGB images. Strawberry purée, sugar, lemon juice and two types of thickener were mixed in different proportions by I-optimal mixture design to obtain 44 SYP formulations. The samples were subjected to light and temperature stress conditions for five weeks; during this time the RGB images of the samples were acquired using a flatbed scanner, along with the images of the corresponding control samples. The dimensionality of the acquired images was reduced by two different approaches: i) the conversion of images into signals, namely colourgrams, which can be seen as the colour fingerprint of the imaged samples, and ii) the calculation of the median values of various colour-related parameters. The colourgrams dataset was then subjected to exploratory data analysis using Principal Component Analysis, while the median values of colour-related parameters were analysed using Response Surface Methodology and Partial Least Squares-Discriminant Analysis. The aim of data analysis was both to find the best colour parameters to describe colour variability over time, and to investigate the cause-effect relationship between mixture proportions and colour response. The results highlighted that, among the considered colour parameters, relative green (i.e., the ratio of green to lightness) and red could be used to monitor colour changes. Colour variation due to stress conditions was more pronounced for samples with a high percentage of strawberry purée, and the type of thickener also affected the colour degradation kinetics.
2022
- Data Fusion Approach to Simultaneously Evaluate the Degradation Process Caused by Ozone and Humidity on Modern Paint Materials
[Articolo su rivista]
Pagnin, L.; Calvini, R.; Sterflinger, K.; Izzo, F. C.
abstract
The knowledge of the atmospheric degradation reactions affecting the stability of modern materials is still of current interest. In fact, environmental parameters, such as relative humidity (RH), temperature, and pollutant agents, often fluctuate due to natural or anthropogenic climatic changes. This study focuses on evaluating analytical and statistical strategies to investigate the degradation processes of acrylic and styrene-acrylic paints after exposure to ozone (O3) and RH. A first comparison of FTIR and Py-GC/MS results allowed to obtain qualitative information on the degradation products and the influence of the pigments on the paints’ stability. The combination of these results represents a significant potential for the use of data fusion methods. Specifically, the datasets obtained by FTIR and Py-GC/MS were combined using a low-level data fusion approach and subsequently processed by principal component analysis (PCA). It allowed to evaluate the different chemical impact of the variables for the characterization of unaged and aged samples, understanding which paint is more prone to ozone degradation, and which aging variables most compromise their stability. The advantage of this method consists in simultaneously evaluating all the FTIR and Py-GC/MS variables and describing common degradation patterns. From these combined results, specific information was obtained for further suitable conservation practices for modern and contemporary painted films.
2022
- Former Foodstuff Products (FFPs) as Circular Feed: Types of Packaging Remnants and Methods for Their Detection
[Articolo su rivista]
Luciano, A; Mazzoleni, S; Ottoboni, M; Tretola, M; Calvini, R; Ulrici, A; Manoni, M; Bernardi, Cem; Pinotti, L
abstract
Alternative feed ingredients in farm animal diets are a sustainable option from several perspectives. Former food products (FFPs) provide an interesting case study, as they represent a way of converting food industry losses into ingredients for the feed industry. A key concern regarding FFPs is the possible packaging residues that can become part of the product, leading to potential contamination of the feed. Although the level of contamination has been reported as negligible, to ensure a good risk evaluation and assessment of the presence of packaging remnants in FFPs, several techniques have been proposed or are currently being studied, of which the main ones are summarized in this review. Accordingly visual inspections, computer vision (CV), multivariate image analysis (MIA), and electric nose (e-nose) are discussed. All the proposed methods work mainly by providing qualitative results, while further research is needed to quantify FFP-derived packaging remnants in feed and to evaluate feed safety as required by the food industries.
2022
- Sensory evaluation and mixture design assessment of coffee-flavored liquor obtained from spent coffee grounds
[Articolo su rivista]
Masino, Francesca; Montevecchi, Giuseppe; Calvini, Rosalba; Foca, Giorgia; Antonelli, Andrea
abstract
Spent coffee grounds are a source of bioactive compounds, including caffeine and other valuable substances, such as volatiles, fats, and alkaloids, which are currently not exploited. In this research, the water content was separated through absolute ethanol extraction yielding a hydroalcoholic solution of volatiles that could be an optimal base for a coffee-flavored liquor. This work was aimed at assessing the potential of coffee-flavored liquor formulations with the use of sensory techniques and mixture design.
Based on a mixture experimental design, a total of 17 coffee-flavored liquors, including replicates, were obtained at lab-scale by adding variable amounts of water, glucose syrup, and caramel to a fixed amount of hydroalcoholic extract flavored with vanillin.
First, a total of 328 consumers were asked to answer a questionnaire about the attributes of an ideal coffeeflavored liquor and their intensity in order to draw an ideal profile from a consumer point of view. Then, judges trained on the attributes of industrially-produced coffee liquors were involved in two descriptive sensory evaluation sessions of the 17 samples. Data analysis pointed to the coffee-flavored liquors with a sensory profile close to the ideal one. They showed
an intense black color (mean score 7) related to a higher caramel concentration in comparison with the other samples. In addition, they scored higher in flavor and body (mean score 7) due to the relative amount of syrup and water. It is noteworthy to mention that the judges regarded these samples as more balanced than the others in terms of alcohol strength, bitterness, and sweetness.
2022
- Toward the Development of Combined Artificial Sensing Systems for Food Quality Evaluation: A Review on the Application of Data Fusion of Electronic Noses, Electronic Tongues and Electronic Eyes
[Articolo su rivista]
Calvini, Rosalba; Pigani, Laura
abstract
2021
- Chemometrics, imaging and spectroscopy laboratory – Department of Life Sciences, University of Modena and Reggio Emilia
[Articolo su rivista]
Calvini, Rosalba; Foca, Giorgia; Ulrici, Alessandro
abstract
2021
- Evaluation of the effect of factors related to preparation and composition of grated Parmigiano Reggiano cheese using NIR hyperspectral imaging
[Articolo su rivista]
Calvini, R.; Michelini, S.; Pizzamiglio, V.; Foca, G.; Ulrici, A.
abstract
The present study is focused on the evaluation of the effect of grater type and fat content of the pulp on the spectral response obtained by near infrared hyperspectral imaging (NIR-HSI), when this technique is used to determine the rind percentage in Parmigiano Reggiano (P-R) cheese. To this aim, grated P-R cheese samples were prepared considering all the possible combinations between three levels of rind amount (8%, 18% and 28%), two levels of fat content of the pulp and two different grater types, and the corresponding hyperspectral images were acquired in the 900–1700 nm spectral range. In a first step, the average spectrum (AS) was calculated from each hyperspectral image, and the corresponding dataset was analysed by means of Analysis of Variance Simultaneous Component Analysis (ASCA) to assess the effect of the three considered factors and their two-way interactions on the spectral response. Then, the hyperspectral images were converted into Common Space Hyperspectrograms (CSH), which are signals obtained by merging in sequence the frequency distribution curves of quantities calculated from a Principal Component Analysis (PCA) model common to the whole hyperspectral image dataset. ASCA was also applied to the CSH dataset, in order to evaluate the effect of the considered factors on this kind of signals. Generally, all the three factors resulted to have a significant effect, but with a different extent according to the method used to analyse the hyperspectral images. Indeed, while fat content of the pulp and rind percentage showed a comparable effect on the spectral response of AS dataset, in the case of CSH signals rind percentage had a greater effect compared to the other main factors. However, CSH were also more sensitive to differences ascribable to the natural variability between diverse Parmigiano Reggiano cheese samples.
2021
- Multivariate Analysis in Microbiome Description: Correlation of Human Gut Protein Degraders, Metabolites, and Predicted Metabolic Functions
[Articolo su rivista]
Raimondi, S.; Calvini, R.; Candeliere, F.; Leonardi, A.; Ulrici, A.; Rossi, M.; Amaretti, A.
abstract
Protein catabolism by intestinal bacteria is infamous for releasing many harmful compounds, negatively affecting the health status, both locally and systemically. In a previous study, we enriched in protein degraders the fecal microbiota of five subjects, utilizing a medium containing protein and peptides as sole fermentable substrates and we monitored their evolution by 16S rRNA gene profiling. In the present study, we fused the microbiome data and the data obtained by the analysis of the volatile organic compounds (VOCs) in the headspace of the cultures. Then, we utilized ANOVA simultaneous component analysis (ASCA) to establish a relationship between metabolites and bacteria. In particular, ASCA allowed to separately assess the effect of subject, time, inoculum concentration, and their binary interactions on both microbiome and volatilome data. All the ASCA submodels pointed out a consistent association between indole and Escherichia–Shigella, and the relationship of butyric, 3-methyl butanoic, and benzenepropanoic acids with some bacterial taxa that were major determinants of cultures at 6 h, such as Lachnoclostridiaceae (Lachnoclostridium), Clostridiaceae (Clostridium sensu stricto), and Sutterellaceae (Sutterella and Parasutterella). The metagenome reconstruction with PICRUSt2 and its functional annotation indicated that enrichment in a protein-based medium affected the richness and diversity of functional profiles, in the face of a decrease of richness and evenness of the microbial community. Linear discriminant analysis (LDA) effect size indicated a positive differential abundance (p < 0.05) for the modules of amino acid catabolism that may be at the basis of the changes of VOC profile. In particular, predicted genes encoding functions belonging to the superpathways of ornithine, arginine, and putrescine transformation to GABA and eventually to succinyl-CoA, of methionine degradation, and various routes of breakdown of aromatic compounds yielding succinyl-CoA or acetyl-CoA became significantly more abundant in the metagenome of the bacterial community.
2021
- SO2- and NOx- initiated atmospheric degradation of polymeric films: Morphological and chemical changes, influence of relative humidity and inorganic pigments
[Articolo su rivista]
Pagnin, L.; Calvini, R.; Wiesinger, R.; Schreiner, M.
abstract
The influence of polluting gases on the stability of polymeric films has not been studied extensively. In fact, the chemical interactions of such materials in contact with the ambient atmosphere depend on different factors such as the environmental conditions, the manufacturing process of the product, the presence of additives or various pigments. In this study, accelerated artificial gas ageing was carried out. The experiments were performed in a gas chamber exposing the samples to sulphur dioxide (SO2) and nitrogen oxide (NOx) with a concentration of 15 ppm. The relative humidity (RH) content chosen was 50% and 80% for a total of 168 h of gas exposure. The paint samples under investigation were composed of three different binding media (acrylic, alkyd, and styrene-acrylic) with various inorganic pigments. The morphological changes on the sample surface due to the different impact of the pollutant gases were investigated using a 3D microscope. Moreover, qualitative and semi-quantitative analyses were performed by Fourier-Transform Infrared (ATR-FTIR) spectroscopy, focusing on the degradation reactions and surface mapping evaluation. In order to fully exploit the chemical information on these materials contained in the ATR-FTIR spectra, multivariate analysis was carried out. In particular, Principal Component Analysis (PCA) enabled verifying the main spectral differences between the unaged and aged samples. Moreover, Analysis-of-Variance-Simultaneous Component Analysis (ASCA) was carried out to understand the influence of gas type, relative humidity, and inorganic pigment type on the deterioration process of the binders.
2020
- Colourgrams GUI: A graphical user-friendly interface for the analysis of large datasets of RGB images
[Articolo su rivista]
Calvini, R.; Orlandi, G.; Foca, G.; Ulrici, A.
abstract
Colourgrams GUI is a graphical user-friendly interface developed in order to facilitate the analysis of large datasets of RGB images through the colourgrams approach. Briefly, the colourgrams approach consists in converting a dataset of RGB images into a matrix of one-dimensional signals, the colourgrams, each one codifying the colour content of the corresponding original image. This matrix of signals can be in turn analysed by means of common multivariate statistical methods, such as Principal Component Analysis (PCA) for exploratory analysis of the image dataset, or Partial Least Squares (PLS) regression for the quantification of colour-related properties of interest. Colourgrams GUI allows to easily convert the dataset of RGB images into the colourgrams matrix, to interactively visualize the signals coloured according to qualitative and/or quantitative properties of the corresponding samples and to visualize the colour features corresponding to selected colourgram regions into the image domain. In addition, the software also allows to analyse the colourgrams matrix by means of PCA and PLS.
2020
- Dual Stage Image Analysis for a complex pattern classification task: Ham veining defect detection
[Articolo su rivista]
Lopes, J. F.; Barbon, A. P. A. C.; Orlandi, G.; Calvini, R.; Lo Fiego, D. P.; Ulrici, A.; Barbon, S.
abstract
Veins in pork thigh carcass are directly related to the quality of dry-cured ham, and consequently to its market value. Some veining defects over the surface of raw ham are easily detected by humans and precisely assessed by a specialist. However, the automatic evaluation of raw ham quality by image analysis poses some challenges to the traditional Computer Vision Systems (CVS), many of them grounded on the complex image pattern related to each defect level. To improve the CVS classification performance without overburdening feature extraction, as well as the common machine learning modelling, we propose Dual Stage Image Analysis (DSIA). DSIA is an additional step in a CVS, that was built in two stages based on the “divide and conquer” strategy. The first stage consists of splitting the region of interest into sub-regions to predict the presence of veining. In the second stage, the algorithm computes the number of veining sub-regions to assess the final defect level classification. A total of 194 raw ham samples were used to evaluate the DSIA performance in the experiments. Support Vector Machine and Random Forest algorithms were compared for inducing the classification model using 92 image features. Random Forest model was the best, capable of predicting defect level with 88.10% accuracy using DSIA. Without DSIA, the CVS with RF achieved an accuracy of 63.10%.
2020
- Exploring the potential of NIR hyperspectral imaging for automated quantification of rind amount in grated Parmigiano Reggiano cheese
[Articolo su rivista]
Calvini, R.; Michelini, S.; Pizzamiglio, V.; Foca, G.; Ulrici, A.
abstract
Parmigiano Reggiano (P-R) is one of the most important Italian food products labelled with Protected Designation of Origin (PDO). The PDO denomination is applied also to grated P-R cheese products meeting the requirements regulated by the Specifications of Parmigiano Reggiano Cheese. Different quality parameters are monitored, including the percentage of rind, which is edible and should not exceed the limit of 18% (w/w). The present study aims at evaluating the possibility of using near infrared hyperspectral imaging (NIR-HSI) to quantify the rind percentage in grated Parmigiano Reggiano cheese samples in a fast and non-destructive manner. Indeed, NIR-HSI allows the simultaneous acquisition of both spatial and spectral information from a sample, which is more suitable than classical single-point spectroscopy for the analysis of heterogeneous samples like grated cheese. Hyperspectral images of grated P-R cheese samples containing increasing levels of rind were acquired in the 900–1700 nm spectral range. Each hyperspectral image was firstly converted into a one-dimensional signal, named hyperspectrogram, which codifies the relevant information contained in the image. Then, the matrix of hyperspectrograms was used to calculate a calibration model for the prediction of the rind percentage using Partial Least Squares (PLS) regression. The calibration model was validated considering two external test sets of samples, confirming the effectiveness of the proposed approach.
2020
- Growing applications of hyperspectral and multispectral imaging
[Capitolo/Saggio]
Calvini, R.; Ulrici, A.; Amigo, J. M.
abstract
Hyperspectral and multispectral imaging (HSI and MSI, respectively) are well-known imaging techniques that have their origin in remote sensing. Nevertheless, the versatility of having a camera with the integration of a spectrometer for each pixel has profited many other branches of science and industry. Nowadays they are used in precision agriculture, pharmaceutical production, artworks, biochemistry, etc. There are several fields in which their implementation is still growing and being adapted to the requirements of the field. This chapter will review some of them, such as their implementation in waste sorting, forensic sciences, archaeology, or entomology. Of course, there are many other fields of expertise taking benefits from HSI and MSI. These are just some examples of unusual applications of HSI and MSI where we believe that there is still a vast potential for growth.
2020
- Hyperspectral imaging in crop fields: precision agriculture
[Capitolo/Saggio]
Caballero, D.; Calvini, R.; Amigo, J. M.
abstract
Precision agriculture is starting to play a key role in the new generation of modern agricultural revolution. Farmers are increasingly aware of the importance of maintaining direct control of several fundamental aspects such as the state of health of crops, the amount of water or fertilizer, and possible infections that can develop in the field. Hyperspectral imaging (HSI) and multispectral imaging (MSI) have been applied for these matters for some decades. Nevertheless, there are still several technological and practical barriers to face. This chapter provides an overview of some of the relevant scientific literature related to the application of HSI and MSI on crop fields. Some of the applications are related to the detection of contaminants and heavy metals, the control and assessment of water sources, and the detection of the health status of the fields. The main advantages and constraints and the methods to acquire the images (by using satellites or aerial vehicles) are also shown and discussed.
2020
- Image data fusion applied to pictorial layers recognition
[Abstract in Atti di Convegno]
Bonifazi, G.; Capobianco, G.; Serranti, S.; Calvini, R.
abstract
Hyper-Spectral Imaging (HSI) is gaining, as a diagnostic tool in the field of cultural heritage, an increasing interest and it has been largely utilized in the last decade tanks to its ability to obtain both spatial and spectral information from a sample. Furthermore, it is a consolidated practice, to perform a better sample characterization, to acquire multiple imaging data coming from different devices covering different spectral ranges. In the present study, we present an analytical approach based on data fusion strategies to classify layers of different pigments using hyperspectral images acquired in two spectral ranges: Visible near infrared (Vis-NIR: 400-1000 nm) and short infrared wavelength infrared (SWIR: 1000-2500 nm). The main aim of the study was to combine the data acquired by the two HSI sensors following a multivariate approach to classify pigments, thanks to the complementary information collected in the different spectral regions.
2020
- Multi-target prediction of wheat flour quality parameters with near infrared spectroscopy
[Articolo su rivista]
Barbon Junior, S.; Mastelini, S. M.; Barbon, A. P. A. C.; Barbin, D. F.; Calvini, R.; Lopes, J. F.; Ulrici, A.
abstract
Near Infrared (NIR) spectroscopy is an analytical technology widely used for the non-destructive characterisation of organic samples, considering both qualitative and quantitative attributes. In the present study, the combination of Multi-target (MT) prediction approaches and Machine Learning algorithms has been evaluated as an effective strategy to improve prediction performances of NIR data from wheat flour samples. Three different Multi-target approaches have been tested: Multi-target Regressor Stacking (MTRS), Ensemble of Regressor Chains (ERC) and Deep Structure for Tracking Asynchronous Regressor Stack (DSTARS). Each one of these techniques has been tested with different regression methods: Support Vector Machine (SVM), Random Forest (RF) and Linear Regression (LR), on a dataset composed of NIR spectra of bread wheat flours for the prediction of quality-related parameters. By combining all MT techniques and predictors, we obtained an improvement up to 7% in predictive performance, compared with the corresponding Single-target (ST) approaches. The results support the potential advantage of MT techniques over ST techniques for analysing NIR spectra.
2020
- Multivariate image analysis for the rapid detection of residues from packaging remnants in former foodstuff products (FFPs)–a feasibility study
[Articolo su rivista]
Calvini, R.; Luciano, A.; Ottoboni, M.; Ulrici, A.; Tretola, M.; Pinotti, L.
abstract
From a circular economy perspective, feeding livestock with food leftovers or former foodstuff products (FFPs) could be an effective option aimed at exploiting food leftover resources and reducing food losses. FFPs are valuable energy sources, characterised by a beneficial starch/sugar content, and also fats. However, besides these nutritional aspects, safety is a key concern given that FFPs are generally derived from packaged food. Packaging materials, such as plastics and paper, are not accepted as a feed ingredient which means that residues should be rigorously avoided. A sensitive and objective detection method is thus essential for an accurate risk evaluation throughout the former food production chain. To this end, former food samples were collected in processing plants of two different European countries and subjected to multivariate analysis of red, green, and blue (RGB) microscopic images, in order to evaluate the possible application of this non-destructive technique for the rapid detection of residual particles from packaging materials. Multivariate Image Analysis (MIA) was performed on single images at the pixel level, which essentially consisted in an exploratory analysis of the image data by means of Principal Component Analysis, which highlighted the differences between packaging and foodstuff particles, based on their colour. The whole dataset of images was then analysed by means of a multivariate data dimensionality reduction method known as the colourgrams approach, which identified clusters of images sharing similar features and also highlighted outlier images due to the presence of packaging particles. The results obtained in this feasibility study demonstrated that MIA is a promising tool for a rapid automated method for detecting particles of packaging materials in FFPs.
2020
- Photodegradation Kinetics of Alkyd Paints: The Influence of Varying Amounts of Inorganic Pigments on the Stability of the Synthetic Binder
[Articolo su rivista]
Pagnin, L.; Calvini, R.; Wiesinger, R.; Weber, J.; Schreiner, M.
abstract
As the effects of climate change pose an increasing risk of damaging outdoor modern and contemporary artworks’ aesthetic appearance by affecting their mechanical properties and chemical-physical stability, understanding the degradation processes attacking these objects is becoming more and more essential to their conservation. For this purpose, the kinetics of photo-oxidation processes occurring in alkyd paints and their stability in mixtures with different inorganic pigments were investigated. The aim was to characterize the different degradation reactions over time and study the photodegradation kinetics according to different pigments and pigment/binder ratios. This paper describes the degradation behavior of artificial ultramarine blue, hydrated chromium oxide green, and cadmium sulfate yellow pigments mixed with alkyd resin and aged under simulated sunlight exposure for a total of 1,008 h. The analytical techniques used offer complementary information on the characterization of the samples and their aging. Specifically, 3D Optical Microscopy allowed studying morphological and color changes. These results were supported by Scanning Electron Microscopy and Colorimetry analyses, also focused on studying the physical and granulometric characteristics of the pigments in relation to the binder degradation. Finally, qualitative and quantitative analysis was performed by Attenuated Total Reflection Infrared Spectroscopy. To support the obtained results, Multivariate Analysis of microscopic images was carried out with the aim of studying the degradation effects linked to color and texture changes. The obtained results demonstrate that the degradation processes of alkyd resin are influenced by the presence of the different inorganic pigments used and their concentration in the mixtures. This study should contribute as support to the field of conservation-restoration to find suitable protection strategies for paint surfaces against degradation agents.
2020
- Sustainable innovation: sensory study of coffee-flavoured liqueur from spent coffee grounds
[Poster]
Masino, F.; Montevecchi, G.; Calvini, R.; Foca, G.; Antonelli, A.
abstract
2019
- Data fusion of electronic eye and electronic tongue signals to monitor grape ripening
[Articolo su rivista]
Orlandi, Giorgia; Calvini, Rosalba; Foca, Giorgia; Pigani, Laura; Vasile Simone, Giuseppe; Ulrici, Alessandro
abstract
Two separate artificial sensors, an electronic eye (EE) and an electronic tongue (ET), were recently developed to monitor grape ripening based on the analysis of must. The aim of this research is to exploit the complementary information obtained by means of EE and ET sensing systems using different data fusion strategies, in order to develop an integrated device able to quickly and easily quantify the physico-chemical parameters that are used to assess phenolic ripeness. To this purpose, both low-level and mid-level data fusion approaches were investigated. Partial Least Squares (PLS) regression was applied to the fused data, with the aim of relating the information brought by the two sensors with twelve physico-chemical parameters measured on the must samples by standard analytical methods. The results achieved with mid-level data fusion outperformed those obtained using EE and ET separately, and highlighted that both the artificial sensors have made a significant contribution to the prediction of each one of the considered physico-chemical parameters.
2019
- Land Snails as a Valuable Source of Fatty Acids: A Multivariate Statistical Approach
[Articolo su rivista]
Galluzzo, Francesco Giuseppe; Cammilleri, Gaetano; Ulrici, Alessandro; Calvini, Rosalba; Pulvirenti, Andrea; Lo Cascio, Giovanni; Macaluso, Andrea; Vella, Antonio; Cicero, Nicola; Amato, Andtonella; Ferrantelli, Vincenzo
abstract
The fatty acid (FA) profile of wild Theba pisana, Cornu aspersum, and Eobania vermiculata land snail samples, collected in Sicily (Southern Italy), before and after heat treatment at +100 °C were examined by gas chromatography with a flame ionization detector (GC‐FID). The results show a higher content of polyunsaturated fatty acids (PUFAs) in all of the examined raw snails samples, representing up to 48.10% of the total fatty acids contents, followed by monounsaturated fatty acids (MUFAs). The thermal processing of the snail samples examined determined an overall reduction of PUFA levels (8.13%, 7.75%, and 4.62% for T. pisana, C. aspersum and E. vermiculata samples, respectively) and a species‐specific variation of saturated fatty acid (SFA) contents. Oleic acid remained the most abundant FA of all of the snails species examined, accounting for up to 29.95% of the total FA content. A relevant decrease of ɷ3/ɷ6 ratio was found only for T. pisana samples. The principal component analysis (PCA) showed a separation of the snail samples in terms of species and heat treatment. The results of this work suggest land snails as a valuable source of MUFA and PUFA contents and boiling as appropriate treatment, according to the maintenance of healthy properties.
2019
- Occhio e lingua elettronici ci dicono se l’uva è matura
[Articolo su rivista]
Foca, G.; Calvini, R.; Orlandi, G.; Pigani, L.; Masino, F.; Ulrici, A.
abstract
2018
- Apparato e metodo per determinare parametri fisici e chimici di un campione disomogeneo tramite acquisizione ed elaborazione di immagini a colori del campione
[Brevetto]
Ulrici, Alessandro; Calvini, Rosalba; Foca, Giorgia; Orlandi, Giorgia; Antonelli, Andrea
abstract
L’invenzione consiste in un dispositivo portatile compatto, economico e di semplice utilizzo per il monitoraggio in campo del grado di maturazione fenolica dell’uva mediante l'analisi di immagini acquisite utilizzando uno smartphone.
2018
- Automated quantification of defective maize kernels by means of Multivariate Image Analysis
[Articolo su rivista]
Orlandi, Giorgia; Calvini, Rosalba; Foca, Giorgia; Ulrici, Alessandro
abstract
This article describes the development of a fast and inexpensive method based on digital image analysis for the automated quantification of the percentage of defective maize (%DM). Defective kernels tend to foster high levels of mycotoxins like Deoxynivalenol (DON), which represents a risk for the health of humans and of farm animals. In this work, 332 RGB images of 83 mixtures containing different amounts of defective maize kernels were acquired using a digital camera. The mixtures were also analysed with a commercial ELISA test kit to determine their concentration of DON, that resulted highly correlated with the amount of defective kernels. Each image was then converted into a signal, named colourgram, which codifies its colour-related information content. The colourgrams were firstly explored using Principal Component Analysis. Then, calibration models of the %DM values were developed using Partial Least Squares (PLS) and interval PLS. The best interval PLS model allowed to predict the %DM values of external test set samples with a root mean square error value equal to 2.6%. Based on the output of this model it was also possible to highlight the defective-maize areas within the images, confirming the significance of the proposed approach. (C) 2017 Elsevier Ltd. All rights reserved.
2018
- Development of an automated method for the identification of defective hazelnuts based on RGB image analysis and colourgrams
[Articolo su rivista]
Giraudo, A.; Calvini, R.; Orlandi, G.; Ulrici, A.; Geobaldo, F.; Savorani, F.
abstract
Over the past decades, Red-Green-Blue (RGB) image analysis has gained increasing importance in industrial applications, since it has widely proved to be a suitable tool for food quality and process control. This article describes the development of a fast and objective method for the automated identification of defective hazelnut kernels based on multivariate analysis of RGB images. To this aim, an overall sample set of 2000 half-cut hazelnut kernels, previously assigned by industrial expert assessors as sound or defective (i.e. rotten or pest-affected), was collected and imaged using a digital camera. The colour-related information of the images was converted into one-dimensional signals, named colourgrams, which were firstly explored through the Principal Component Analysis and subsequently used to build classification models, based on both Partial Least Square-Discriminant Analysis (PLS-DA) and interval-PLS-DA (iPLS-DA) algorithms. A tree-structure hierarchical classification approach has been considered, i.e. the discrimination between sound and defective kernels as a first rule, and the discrimination between the two types of defect as a second rule. The best sound vs defective classification model was able to correctly recognize approximately the 97% of the test set defective samples, while the best rotten vs pest-affected model allowed classifying correctly more than 92% of the test set samples. Moreover, the image reconstruction performed using the selected colourgram features led to an exhaustive interpretation of the decision-making criteria adopted by the classification algorithms and further confirmed the reliability of the proposed method.
2018
- Developmentof a classification algorithm for efficient handling of multiple classes in sorting systems based on hyperspectral imaging
[Articolo su rivista]
Calvini, Rosalba; Orlandi, Giorgia; Foca, Giorgia; Ulrici, Alessandro
abstract
When dealing with practical applications of hyperspectral imaging, the development of efficient, fast and flexible classification algorithms is of the utmost importance. Indeed, the optimal classification method should be able, in a reasonable time, to maximise the separation between the classes of interest and, at the same time, to correctly reject possible outlier samples. To this aim, a new extension of Partial Least Squares Discriminant Analysis (PLS-DA), namely Soft PLS-DA, has been implemented. The basic engine of Soft PLS-DA is the same as PLS-DA, but class assignment is subjected to some additional criteria which allow samples not belonging to the target classes to be identified and rejected. The proposed approach was tested on a real case study of plastic waste sorting based on near infrared hyperspectral imaging. Household plastic waste objects made of the six recyclable plastic polymers commonly used for packaging were collected and imaged using a hyperspectral camera mounted on an industrial sorting system. In addition, paper and not recyclable plastics were also considered as potential foreign materials that are commonly found in plastic waste. For classification purposes, the Soft PLS-DA algorithm was integrated into a hierarchical classification tree for the discrimination of the different plastic polymers. Furthermore, Soft PLS-DA was also coupled with sparse-based variable selection to identify the relevant variables involved in the classification and to speed up the sorting process. The tree-structured classification model was successfully validated both on a test set of representative spectra of each material for a quantitative evaluation, and at the pixel level on a set of hyperspectral images for a qualitative assessment.
2018
- Electronic eye for the prediction of parameters related to grape ripening
[Articolo su rivista]
Orlandi, G.; Calvini, R.; Pigani, L.; Foca, G.; Vasile Simone, G.; Antonelli, A.; Ulrici, A.
abstract
An electronic eye (EE) for fast and easy evaluation of grape phenolic ripening has been developed. For this purpose, berries of different grape varieties were collected at different harvest times from veraison to maturity, then an amount of the derived must was deposited on a white sheet of absorbent paper to obtain a sort of paper chromatography. Thus, RGB images of the must spots were collected using a flatbed scanner and converted into one-dimensional signals, named colourgrams, which codify the colour properties of the images. The dataset of colourgrams was used to build calibration models to relate the colour of the images with the phenolic composition of the samples – determined by reference analytical methods – and therefore to follow the ripening trend. Satisfactory calibration models were obtained for the prediction of the most important parameters related to phenolic ripening of grapes, such as colour index, tonality, total anthocyanins content, malvidin-3-O-glucoside and petunidin-3-O-glucoside.
2018
- Prediction of parameters related to grape ripening by multivariate calibration of voltammetric signals acquired by an electronic tongue
[Articolo su rivista]
Pigani, Laura; VASILE SIMONE, Giuseppe; Foca, Giorgia; Ulrici, Alessandro; Masino, Francesca; Cubillana Aguilera, L.; Calvini, Rosalba; Seeber, Renato
abstract
An electronic tongue (ET) consisting of two voltammetric sensors, namely a poly-ethylendioxythiophene modified
Pt electrode and a sonogel carbon electrode, has been developed aiming at monitoring grape ripening. To
test the effectiveness of device and measurement procedures developed, samples of three varieties of grapes have
been collected from veraison to harvest of the mature grape bunches. The derived musts have been then submitted
to electrochemical investigation using Differential Pulse Voltammetry technique. At the same time,
quantitative determination of specific analytical parameters for the evaluation of technological and phenolic
maturity of each sample has been performed by means of conventional analytical techniques. After a preliminary
inspection by principal component analysis, calibration models were calculated both by partial least squares
(PLS) on the whole signals and by the interval partial least squares (iPLS) variable selection algorithm, in order
to estimate physico-chemical parameters. Calibration models have been obtained both considering separately
the signals of each sensor of the ET, and by proper fusion of the voltammetric data selected from the two sensors
by iPLS. The latter procedure allowed us to check the possible complementarity of the information brought by
the different electrodes. Good predictive models have been obtained for estimation of pH, total acidity, sugar
content, and anthocyanins content. The application of the ET for fast evaluation of grape ripening and of most
suitable harvesting time is proposed.
2017
- Transferring results from NIR-hyperspectral to NIR-multispectral imaging systems: A filter-based simulation applied to the classification of Arabica and Robusta green coffee
[Articolo su rivista]
Calvini, Rosalba; Amigo, Jose Manuel; Ulrici, Alessandro
abstract
Due to the differences in terms of both price and quality, the availability of effective instrumentation to discriminate between Arabica and Robusta coffee is extremely important. To this aim, the use of multispectral imaging systems could provide reliable and accurate real-time monitoring at relatively low costs. However, in practice the implementation of multispectral imaging systems is not straightforward: the present work investigates this issue, starting from the outcome of variable selection performed using a hyperspectral system. Multispectral data were simulated considering four commercially available filters matching the selected spectral regions, and used to calculate multivariate classification models with Partial Least Squares-Discriminant Analysis (PLS-DA) and sparse PLS-DA. Proper strategies for the definition of the training set and the selection of the most effective combinations of spectral channels led to satisfactory classification performances (100% classification efficiency in prediction of the test set).
2016
- Data dimensionality reduction and data fusion for fast characterization of green coffee samples using hyperspectral sensors
[Articolo su rivista]
Calvini, Rosalba; Foca, Giorgia; Ulrici, Alessandro
abstract
Hyperspectral sensors represent a powerful tool for chemical mapping of solid-state samples, since they provide spectral information localized in the image domain in very short times and without the need of sample pretreatment. However, due to the large data size of each hyperspectral image, data dimensionality reduction (DR) is necessary in order to develop hyperspectral sensors for real-time monitoring of large sets of samples with different characteristics. In particular, in this work, we focused on DR methods to convert the three-dimensional data array corresponding to each hyperspectral image into a one-dimensional signal (1D-DR), which retains spectral and/or spatial information. In this way, large datasets of hyperspectral images can be converted into matrices of signals, which in turn can be easily processed using suitable multivariate statistical methods. Obviously, different 1D-DR methods highlight different aspects of the hyperspectral image dataset. Therefore, in order to investigate their advantages and disadvantages, in this work, we compared three different 1D-DR methods: average spectrum (AS), single space hyperspectrogram (SSH) and common space hyperspectrogram (CSH). In particular, we have considered 370 NIR-hyperspectral images of a set of green coffee samples, and the three 1D-DR methods were tested for their effectiveness in sensor fault detection, data structure exploration and sample classification according to coffee variety and to coffee processing method. Principal component analysis and partial least squares-discriminant analysis were used to compare the three separate DR methods. Furthermore, low-level and mid-level data fusion was also employed to test the advantages of using AS, SSH and CSH altogether. [Figure not available: see fulltext.]
2016
- Preliminary analysis of RGB images for the identification of defective maize kernels
[Relazione in Atti di Convegno]
Orlandi, Giorgia; Calvini, Rosalba; Foca, Giorgia; Ulrici, Alessandro
abstract
In order to investigate the effectiveness of multivariate image analysis for the evaluation of maize defects, RGB images of maize samples containing different percentages of defective kernels were acquired and then converted into colourgrams, i.e., signals codifying colour-related features. Multivariate analysis of the colourgrams matrix showed a distribution of the acquired samples according to the amount of defective kernels.
2016
- Sparse-Based Modeling of Hyperspectral Data
[Capitolo/Saggio]
Calvini, Rosalba; Ulrici, Alessandro; Amigo, J. M.
abstract
One of the main issues of hyperspectral imaging data is to unravel the relevant, yet overlapped, huge amount of information contained in the spatial and spectral dimensions. When dealing with the application of multivariate models in such high-dimensional data, sparsity can improve the interpretability and the performance of the model. In this chapter, we will introduce the exploration of hyperspectral images using a sparse version of the well-known principal component analysis method to demonstrate how the derived models can reveal very useful spectral zones. In particular, we will present two practical applications related to different issues: the separation among groups of homogeneous samples and the identification of outlier pixels in the spatial domain. For both case studies, guidance to the identification of the proper level of sparsity will be provided and, furthermore, we will show how sparsity can improve the chemical interpretation of the results.
2015
- Fast exploration and classification of large hyperspectral image datasets for early bruise detection on apples
[Articolo su rivista]
Ferrari, Carlotta; Foca, Giorgia; Calvini, Rosalba; Ulrici, Alessandro
abstract
Hyperspectral imaging allows to easily acquire tens of thousands of spectra for a single sample in few seconds; though valuable, this data-richness poses many problems due to the difficulty of handling a representative amount of samples altogether. For this reason, we recently proposed an approach based on the idea of reducing each image into a one-dimensional signal, named hyperspectrogram, which accounts both for spatial and for spectral information. In this manner, a dataset of hyperspectral images can be easily and quickly converted into a set of signals (2D data matrix), which in turn can be analyzed using classical chemometric techniques. In this work, the hyperspectrograms obtained from a dataset of 800 NIR-hyperspectral images of two different apple varieties were used to discriminate bruised from sound apples using iPLS-DA as variable selection algorithm, which allowed to efficiently detect the presence of bruises. Moreover, the reconstruction as images of the selected variables confirmed that the automated procedure led to the exact identification of the spatial features related to the onset and to the subsequent evolution with time of the bruise defect.
2015
- Practical comparison of sparse methods for classification of Arabica and Robusta coffee species using near infrared hyperspectral imaging
[Articolo su rivista]
Calvini, Rosalba; Ulrici, Alessandro; Amigo, Jose Manuel
abstract
In the present work sparse-based methods are applied to the analysis of hyperspectral images with the aim at studying their capability of being adequate methods for variable selection in a classification framework. The key aspect of sparse methods is the possibility of performing variable selection by forcing the model coefficients related to irrelevant variables to zero. In particular, two different sparse classification approaches, i.e. sPCA+kNN and sPLS-DA, were compared with the corresponding classical methods (PCA + kNN and PLS-DA) to classify Arabica and Robusta coffee species. Green coffee samples were analyzed using near infrared hyperspectral imaging and the average spectra from each hyperspectral image were used to build training and test sets; furthermore a test image was used to evaluate the performances of the considered methods at pixel-level. In our case, sparse methods led to similar results as classical methods, with the advantage of obtaining more interpretable and parsimonious models. An important result to highlight is that variable selection performed with two different sparse classification approaches converged to the selection of same spectral regions, which implies the chemical relevance of those regions in the discrimination of Arabica and Robusta coffee species.
2014
- Applicazione della spettroscopia FT-NIR per la determinazione degli acidi grassi e del numero di iodio in campioni di grasso suino prelevati da diversi strati sottocutanei
[Abstract in Atti di Convegno]
Foca, Giorgia; Ulrici, Alessandro; Ferrari, Carlotta; Calvini, Rosalba; Ielo, Maria Cristina; Minelli, Giovanna; LO FIEGO, Domenico Pietro
abstract
La qualità tecnologica e organolettica del grasso suino può essere stimata mediante analisi
chimiche quali il numero di iodio e la determinazione gascromatografica della composizione in
acidi grassi. Tali metodi, tuttavia, sono lunghi, costosi, dannosi per l’ambiente e non sono adatti per
seguire un processo in tempo reale.
In questo lavoro, abbiamo valutato la potenzialità della spettroscopia NIR, accoppiata a
tecniche chemiometriche appropriate, per predire il numero di iodio e la composizione in acidi
grassi di campioni di grasso suino estratti da due diversi strati sottocutanei1, partendo da misure di
riferimento acquisite con i metodi tradizionali, analisi di Wijs e analisi gascromatografica. Gli
spettri NIR sono stati acquisiti utilizzando due diversi accessori: sfera integratrice e sonda a fibre
ottiche. Riguardo alle tecniche chemiometriche, abbiamo utilizzato PCA come metodo di analisi
esplorativa, che ha consentito di eliminare i campioni outlier, quindi abbiamo costruito diversi
modelli di calibrazione mediante PLS. Successivamente, abbiamo applicato agli spettri anche un
metodo di calibrazione con selezione di variabili, iPLS. Poiché non è possibile sapere a priori quale
sia il miglior pretrattamento per estrarre l’informazione utile dagli spettri, sono state confrontate
diverse combinazioni di pretrattamenti per ottenere il miglior modello.
Concludendo, abbiamo discusso i modelli ottenuti al fine di: i) individuare le condizioni
operative (considerando sia le condizioni strumentali che le procedure chemiometriche utilizzate)
che hanno portato all’ottenimento dei modelli migliori; ii) individuare le regioni spettrali più
informative ai fini della calibrazione; iii) ottenere una migliore comprensione delle caratteristiche
chimiche del grasso proveniente dai due diversi strati sottocutanei; iv) comprendere quali acidi
grassi possano effettivamente essere quantificati con la spettroscopia NIR in un processo reale.
2014
- Classification of Arabica and Robusta coffee samples subjected to different technological treatments using various image analysis methods
[Abstract in Atti di Convegno]
Calvini, Rosalba; Foca, Giorgia; Bellucci, L.; Ulrici, Alessandro
abstract
Coffee varietal differentiation based on NIR spectroscopy has been widely investigated in the last
20 years [1-3]. In this work, we have applied hyperspectral imaging in the NIR range (900-1700
nm) for the classification of Arabica and Robusta coffee varieties, considering coffee beans
subjected to different processing methods, i.e., the so-called dry method (to produce natural coffee),
wet method (to produce washed coffee) and a somewhat intermediate processing method, referred to
as polishing method (to produce polished coffee).
PCA has been used as an exploratory technique both on the image mean spectra and on the
hyperspectrograms obtained from the images. The hyperspectrograms are built by compressing the
useful information contained in each hyperspectral image into a signal composed by the frequency
distribution curves of quantities calculated by PCA [4]. This procedure allows to compress the
information conveyed by the hyperspectral images, maintaining at the same time both spatial- and
spectral-related features.
The PCA models obtained showed a clear clustering of Arabica and Robusta samples, whereas,
considering the technological treatment, the polished coffee samples are clearly distinguishable
from the others, while natural and washed coffee samples are quite superimposed.
Image mean spectra and hyperspectrograms were then subjected to PLS-DA classification after
preprocessing using SNV followed by meancentering or meancentering only. Concerning the
discrimination of coffee samples between Arabica and Robusta categories, the same value of
classification efficiency in prediction (EFFPRED = 86.3%) has been obtained considering both the
mean spectra and the hyperspectrograms. After forward iPLS-DA variable selection, EFFPRED
increased up to 98.6% for models calculated using the mean spectra and up to 100% for the models
calculated using the hyperspectrograms.
As for the discrimination of the coffee samples into the three natural, polished and washed
processing categories, the PLS-DA models calculated using mean spectra led to EFFPRED values
equal to 81.1%, 95.7% and 49.8%, respectively, while the PLS-DA models calculated using
hyperspectrograms led to EFFPRED values equal to 94.7%, 100% and 92.4%, respectively. In this
case, iPLS-DA variable selection led to an increase of the performances of the model calculated on
mean spectra (EFFPRED equal to 82.9%, 98.6% and 86.5%, respectively) and to a decrease of the
performances of the model calculated using hyperspectrograms (EFFPRED equal to 82.9%, 89.3%
and 86.5%, respectively).
2014
- Data reduction di immagini iperspettrali: applicazione a problemi di classificazione
[Abstract in Atti di Convegno]
Calvini, Rosalba; Ferrari, Carlotta; Foca, Giorgia; Ulrici, Alessandro
abstract
L'imaging iperspettrale (HSI) consente di acquisire in pochi secondi ipercubi di grandi
dimensioni, composti da milioni di spettri, che corrispondono a file spesso più grandi di 50 MB.
Questa ricchezza di dati rappresenta il principale vantaggio dell’HSI, sebbene causi seri problemi
per la gestione dei dati, tali da complicare lo sviluppo di applicazioni industriali efficienti per il
controllo in linea. Il nostro gruppo di ricerca ha recentemente proposto un’alternativa1 per trattare
dataset composti da decine o centinaia di immagini iperspettrali, che consiste nel convertire ogni
immagine iperspettrale in un segnale, chiamato iperspettrogramma, costruito in modo da
considerare sia l’informazione di natura spaziale che spettrale. Risulta così possibile trasformare
dataset composti da un elevato numero di immagini iperspettrali in matrici bidimensionali di
iperspettrogrammi, che a loro volta possono essere analizzate utilizzando i più comuni metodi
chemiometrici quali PCA, PLS o PLS-DA.
In questo contesto, presentiamo due applicazioni degli iperspettrogrammi per la soluzione di
problemi di classificazione. Una prima applicazione riguarda l'individuazione precoce di difetti
superficiali in diverse varietà di mele, con particolare attenzione ai campioni in cui il difetto non
risulta apprezzabile ad occhio nudo. Le 800 immagini iperspettrali acquisite sono state convertite in
iperspettrogrammi permettendo così la riduzione delle dimensioni del dataset da 18.6 GB a 4.7 MB.
Inoltre la selezione di variabili mediante iPLS-DA ha permesso di ridurre ulteriormente le
dimensioni del dataset e identificare le regioni più rilevanti nel segnale. Il migliore modello iPLSDA,
calcolato utilizzando solo 30 variabili delle 1200 iniziali, ha portato ad un valore di efficienza
in predizione sul test set esterno pari a 89.6%. Una seconda applicazione riguarda la classificazione
di caffè verde appartenente a diverse tipologie: Arabica e Robusta. Prove preliminari hanno
mostrato come la classificazione mediante PLS-DA effettuata sugli iperspettrogrammi ha portato ad
un valore di efficienza in predizione del 98.3%.
2014
- Detection of contamination by aflatoxins on apricot kernels using NIR-hyperspectral imaging
[Abstract in Atti di Convegno]
Calvini, Rosalba; Zivoli, R.; Piemontese, L.; Ferrari, Carlotta; Foca, Giorgia; Perrone, G.; Ulrici, Alessandro; Solfrizzo, M.
abstract
Aflatoxins can be found as contaminants in a wide range of foods, such as nuts, cereals, dried fruits
and milk. Due to their hepatotoxic and carcinogenic effects, the maximum allowed concentration of
aflatoxins is nowadays regulated in many countries, with levels up to 50 μg/kg.
In routine analysis, the main methods used to determine aflatoxins are based on high-performance
liquid chromatography (HPLC) and enzyme-linked immunosorbent assay (ELISA) [1]. Despite the
very high sensitivity of these methods, they are destructive, expensive, time consuming and not
appropriate for real time control, e.g., online.
Consequently, the development of fast, non destructive and economic methods for aflatoxins
detection and monitoring in food industry is becoming more and more important.
Our studies showed that manual sorting of dark or spotted apricot kernels removed 97.3-99.5% of
total aflatoxins [2]. However, discolored seeds could be visually identified only after removing the
skins from each seed by means of a time-consuming operation.
For these reasons, in this work we investigated the possibility to use NIR–HSI for the fast and nondestructive
automated identification of aflatoxin contaminated unpeeled apricot kernels.
On the whole 9 hyperspectral images, each one containing 48 kernels, were acquired in the 900-
1700 nm range. After image acquisition, the kernels were peeled to identify the dark or spotted
kernels and subjected to HPLC analysis for AFB1 quantification.
Classification models were then calculated on a training set of NIR spectra extracted from a
representative number of non-contaminated and dark seeds, selected on 5 images on the basis of
HPLC analysis results as well as of the visual evaluation of the peeled kernels. The remaining 4
images were instead used as independent test set for model validation. Since dark seeds were found
to have a higher concentration of AFB1 than spotted seeds, the latter ones were not included in the
training set.
Different iPLS-DA classification models, built using different signal preprocessing methods and
different interval size values, were then evaluated in terms of classification efficiency in cross
validation of the training set pixels, in order to select the optimal conditions. The results were
reported under the form of predicted probability maps, and for each single kernel the contamination
was estimated as the percentage of pixels assigned to the “contaminated” class by the iPLS-DA
model.
2014
- Exploration of datasets of hyperspectral images
[Abstract in Atti di Convegno]
Ferrari, Carlotta; Calvini, Rosalba; Foca, Giorgia; Ulrici, Alessandro
abstract
Hyperspectral images of size usually greater than 50 MB can be easily acquired in very short times,
generally without the need of sample pretreatment. While Multivariate Image Analysis (MIA) tools
can be efficiently used for the exploration of single hyperspectral images or of groups composed by
a limited number (say up to 10) of merged images, the exploration of datasets composed by a large
number (>10) of images is less straightforward. However, a representative sampling of a large
number of specimens is frequently required to correctly estimate both intra- and inter-sample
variability. This implies the acquisition of datasets composed by a large number of hyperspectral
images and of several GB in size, especially in those cases where only one or a few samples can be
included in a single image scene. In this context, the exploration of the dataset by applying MIA to
single images or to subgroups of merged images does not allow to gain a global overview of the
entire dataset variability and to properly highlight the possible presence of outliers, clusters and/or
trends. A fast procedure which can be adopted to deal with this issue consists in computing the
average spectrum of each image, to build a matrix of average spectra of the analyzed hyperspectral
images. Although this approach leads sometimes to satisfactory results (especially when dealing
with homogeneous materials), the information related to spatial variability is lost, and the
hyperspectral image data are turned into “common” (i.e., not spatially resolved) spectral data. By
averaging spectra, for example, the useful information related to the presence of a defect localized
in a relatively narrow image area could be diluted within the massive amount of other “well
behaving” pixels, becoming no longer detectable.
Aiming to develop a fast and easy-to-use tool able to facilitate the exploration of large datasets of
hyperspectral images while maintaining both spectral- and spatial-related information of the
original images, we have proposed an approach which consists in automatically converting each
hyperspectral image into a signal named hyperspectrogram [1]. Essentially, the hyperspectrogram
can be viewed as a fingerprint containing the relevant information brought by the original
hyperspectral image, and is composed by a first part accounting for the spatial information and by a
second part accounting for the spectral information. By representing each image with a vector of
few hundreds of points, this procedure enables to compare simultaneously up to hundreds of images
by means of common multivariate analysis methods, such as PCA.
In order to facilitate the exploration of datasets of hyperspectral images through hyperspectrograms,
we have recently developed a Matlab Graphical User Interface (GUI), which easily allows
calculation and visualization of hyperspectrograms, exploration of the dataset and visualization of
the features of interest contained within each single sample directly in the original image domain.