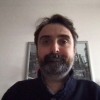 |
Luca LA ROCCA
Professore Associato Dipartimento di Scienze Fisiche, Informatiche e Matematiche sede ex-Matematica
|
Home |
Curriculum(pdf) |
Didattica |
Pubblicazioni
2024
- On the Detection of Significant Pairwise Interactions in Complex Systems
[Relazione in Atti di Convegno]
Fini, Giada; D’Addese, Gianluca; La Rocca, Luca; Villani, Marco
abstract
Many systems in nature, society and technology are complex systems, i.e., they are composed of numerous parts that interact in a non-linear way giving rise to positive and negative feedback. The dynamic organization of these systems often allows the emergence of intermediate structures that once formed profoundly influence the system and therefore play a key role in understanding its behavior. In the recent past our group has devised an effective method for identifying groups of interacting variables within a system, based on their observation. The result is a set of entities, each of which connects two or more nodes of the system: this result can therefore be represented by a hypergraph, which can be of considerable use for understanding the system under consideration. In particular, we use an index that allows us to evaluate the level of integration of a group of variables. In order for a group to be identified as significant, the value of this index must exceed a threshold that corresponds (under appropriate hypotheses) to a level of statistical significance decided by the user. In this work we propose a more elaborate approach to determining the significance threshold, which is (i) in itself theoretically interesting and (ii) of considerable practical utility. We use the new approach to determine collections of pairwise relationships in meaningful cases, such as relationships in gene regulatory networks.
2023
- Assessing skewness in financial markets
[Articolo su rivista]
Campisi, G.; La Rocca, L.; Muzzioli, S.
abstract
It is a matter of common observation that investors value substantial gains but are averse to heavy losses. Obvious as it may sound, this translates into an interesting preference for right-skewed return distributions, whose right tails are heavier than their left tails. Skewness is thus not only a way to describe the shape of a distribution, but also a tool for risk measurement. We review the statistical literature on skewness and provide a comprehensive framework for its assessment. Then, we present a new measure of skewness, based on the decomposition of variance in its upward and downward components. We argue that this measure fills a gap in the literature and show in a simulation study that it strikes a good balance between robustness and sensitivity.
2022
- Discussion to: Bayesian graphical models for modern biological applications by Y. Ni, V. Baladandayuthapani, M. Vannucci and F.C. Stingo
[Articolo su rivista]
Castelletti, F.; Consonni, G.; La Rocca, L.
abstract
We contribute to the discussion of the paper by Ni et al. (Stat Methods Appl, 2021. https://doi.org/10.1007/s10260-021-00572-8) by focusing on two aspects: (i) ordering of the variables for directed acyclic graphical models, and (ii) heterogeneity of the data in the presence of covariates. With regard to (i) we claim that an ordering should be assumed only when strongly reliable prior information is available; otherwise one should proceed with an unspecified ordering to guard against order misspecification. Alternatively, one can carry out Bayesian inference on the space of Markov equivalence classes or use a blend of observational and interventional data to alleviate the lack of identification. With regard to (ii) we complement the Authors’ analysis by enlarging the scope to mixed graphs as well as nonparametric Bayesian models.
2022
- Multiple arrows in the Bayesian quiver: Bayesian learning of partially directed structures from heterogeneous data.
[Relazione in Atti di Convegno]
La Rocca, Luca; Castelletti, Federico; Peluso, Stefano; Stingo, Francesco Claudio; Consonni, Guido
abstract
Motivated by the identification of complex dependencies in biological networks, we present a Bayesian method for structural learning of graphical models that exhibits two distinctive features: i) it does not assume a priori an ordering of the variables, but it learns arrows when possible and lines otherwise; ii) it assumes that the observations form subgroups having different but similar structures.
2022
- Two-Level Detection of Dynamic Organization in Cancer Evolution Models
[Relazione in Atti di Convegno]
D'Addese, Gianluca; Graudenzi, Alex; LA ROCCA, Luca; Villani, Marco
abstract
Many systems in nature, society and technology are composed of numerous nonlinearly interacting parts. The dynamic organization of these systems often allows the emergence of intermediate structures that once formed deeply affect the system, and therefore play a key role in understanding its behavior. An interesting hypothesis is that the simultaneous analysis of a system at both levels of description (the microlevel of the relationships between single entities, and the mesolevel constituted by their dynamically organized groups) can allow a better understanding of the phenomenon under examination. In this work we apply this idea to a cancer evolution model, of which each individual patient represents a particular instance. Specifically, in order to validate the idea we analyze the same synthetic dataset – whose ground truth is known – with two methods of analysis, and we merge the results in an innovative way. In doing this, we also evaluate the effectiveness of a new method of reconstructing networks of relationships.
2021
- Asymptotic Information-Theoretic Detection of Dynamical Organization in Complex Systems
[Articolo su rivista]
D'Addese, Gianluca; Sani, Laura; LA ROCCA, Luca; Serra, Roberto; Villani, Marco
abstract
The identification of emergent structures in complex dynamical systems is a formidable challenge. We propose a computationally efficient methodology to address such a challenge, based on modeling the state of the system as a set of random variables. Specifically, we present a sieving algorithm to navigate the huge space of all subsets of variables and compare them in terms of a simple index that can be computed without resorting to simulations. We obtain such a simple index by studying the asymptotic distribution of an information-theoretic measure of coordination among variables, when there is no coordination at all, which allows us to fairly compare subsets of variables having different cardinalities. We show that increasing the number of observations allows the identification of larger and larger subsets. As an example of relevant application, we make use of a paradigmatic case regarding the identification of groups in autocatalytic sets of reactions, a chemical situation related to the origin of life problem.
2020
- Assessing skewness in financial markets
[Working paper]
Campisi, G.; La Rocca, L.; Muzzioli, S.
abstract
It is common knowledge that investors like large gains and dislike large losses.
This translates into a preference for right-skewed return distributions, with right tails heavier than left tails.
Skewness is thus interesting not only as a way to describe the shape of a distribution, but also for risk measurement.
We review the statistical literature on skewness and provide a comprehensive framework for its assessment.
We present a new measure of skewness, based on a relative comparison between above average and below average returns.
We show that this measure represents a valid complement to the state of the art.
2020
- Bayesian learning of multiple directed networks from observational data
[Articolo su rivista]
Castelletti, F.; La Rocca, L.; Peluso, S.; Stingo, F. C.; Consonni, G.
abstract
Graphical modeling represents an established methodology for identifying complex dependencies in biological networks, as exemplified in the study of co-expression, gene regulatory, and protein interaction networks. The available observations often exhibit an intrinsic heterogeneity, which impacts on the network structure through the modification of specific pathways for distinct groups, such as disease subtypes. We propose to infer the resulting multiple graphs jointly in order to benefit from potential similarities across groups; on the other hand our modeling framework is able to accommodate group idiosyncrasies. We consider directed acyclic graphs (DAGs) as network structures, and develop a Bayesian method for structural learning of multiple DAGs. We explicitly account for Markov equivalence of DAGs, and propose a suitable prior on the collection of graph spaces that induces selective borrowing strength across groups. The resulting inference allows in particular to compute the posterior probability of edge inclusion, a useful summary for representing flow directions within the network. Finally, we detail a simulation study addressing the comparative performance of our method, and present an analysis of two protein networks together with a substantive interpretation of our findings.
2020
- Bayesian learning of multiple essential graphs
[Capitolo/Saggio]
La Rocca, Luca; Castelletti, Federico; Peluso, Stefano; Stingo, Francesco Claudio; Consonni, Guido
abstract
Structural learning of graphical models is a well-established approach to the identification of complex dependencies in biological networks. We here present a Bayesian methodology for learning directed networks from observational data when distinct subgroups of a population are observed.
2019
- Discrete graphical models and their parameterization
[Capitolo/Saggio]
La Rocca, Luca; Roverato, Alberto
abstract
This chapter is devoted to graphical models in which the observed variables are categorical, that is, whose state space consists of a finite number of values. The focus is on regression graph models, because this family of models allows us to approach discrete graphical models with a sufficient degree of generality. In particular, we cover as special cases both undirected and bidirected graphical models, as well as acyclic directed graphical models. Special attention is given to the problem of specifying suitable parameterizations and, from this viewpoint, we provide a unified approach to the parameterization of all models considered. This relies on a few general properties of conditional independence and Moebius inversion which are applied, almost identically, to all the models considered to derive suitable parameterizations and show their properties.
2018
- Dynamical Criticality in Gene Regulatory Networks
[Articolo su rivista]
Villani, Marco; La Rocca, Luca; Kauffman, Stuart Alan; Serra, Roberto
abstract
A well-known hypothesis, with far-reaching implications, is that biological evolution should preferentially lead to states that are dynamically critical. In previous papers, we showed that a well-known model of genetic regulatory networks, namely, that of random Boolean networks, allows one to study in depth the relationship between the dynamical regime of a living being's gene network and its response to permanent perturbations. In this paper, we analyze a huge set of new experimental data on single gene knockouts in S. cerevisiae, laying down a statistical framework to determine its dynamical regime. We find that the S. cerevisiae network appears to be slightly ordered, but close to the critical region. Since our analysis relies on dichotomizing continuous data, we carefully consider the issue of an optimal threshold choice.
2017
- Objective Bayes Covariate-Adjusted Sparse Graphical Model Selection
[Articolo su rivista]
Consonni, Guido; LA ROCCA, Luca; Peluso, Stefano
abstract
We present an objective Bayes method for covariance selection in Gaussian multivariate regression models having a sparse regression and covariance structure, the latter being Markov with respect to a directed acyclic graph (DAG). Our procedure can be easily complemented with a variable selection step, so that variable and graphical model selection can be performed jointly. In this way, we offer a solution to a problem of growing importance especially in the area of genetical genomics (eQTL analysis). The input of our method is a single default prior, essentially involving no subjective elicitation, while its output is a closed form marginal likelihood for every covariate-adjusted DAG model, which is constant over each class of Markov equivalent DAGs; our procedure thus naturally encompasses covariate-adjusted decomposable graphical models. In realistic experimental studies, our method is highly competitive, especially when the number of responses is large relative to the sample size.
2016
- Dynamically critical systems and power-law distributions: Avalanches revisited
[Relazione in Atti di Convegno]
Di Stefano, Marina L.; Villani, Marco; LA ROCCA, Luca; Kauffman, Stuart A.; Serra, Roberto
abstract
In this paper we show that a well-known model of genetic regulatory networks, namely that of Random Boolean Networks (RBNs), allows one to study in depth the relationship between two important properties of complex systems, i.e. dynamical criticality and power-law distributions. The study is based upon an analysis of the response of a RBN to permanent perturbations, that may lead to avalanches of changes in activation levels, whose statistical properties are determined by the same parameter that characterizes the dynamical state of the network (ordered, critical or disordered). Under suitable approximations, in the case of large sparse random networks an analytical expression for the probability density of avalanches of different sizes is proposed, and it is shown that for not-too-small avalanches of critical systems it may be approximated by a power law. In the case of small networks the above-mentioned formula does not maintain its validity, because of the phenomenon of self-interference of avalanches, which is also explored by numerical simulations.
2014
- Comments on “Robust Bayesian graphical modeling using Dirichlet t-distributions” by Finegold & Drton
[Articolo su rivista]
G., Consonni; LA ROCCA, Luca
abstract
Discussion contribution.
2013
- A Comparison of Objective Bayes Factors for Variable Selection in Linear Regression Models
[Relazione in Atti di Convegno]
LA ROCCA, Luca
abstract
This paper deals with the variable selection problem in linear regression models and its solution by means of Bayes factors. If substantive prior information is lacking or impractical to elicit, which is often the case in applications, objective Bayes factors come into play. These can be obtained by means of different methods, featuring Zellner-Siow priors, fractional Bayes factors and intrinsic priors. The paper reviews such methods and investigates their finite-sample ability to identify the simplest model supported by the data, introducing the notion of full discrimination power. The results obtained are relevant to structural learning of Gaussian DAG models, where large spaces of sets of recursive linear regressions are to be explored.
2013
- Log-Mean Linear Parameterizations for Smooth Independence Models
[Relazione in Atti di Convegno]
M., Lupparelli; LA ROCCA, Luca; A., Roverato
abstract
This contribution aims to show how the log-mean linear parameterization for a set of categorical data can be used to specify models of marginal independence including also independence constraints in partial tables. The collection of these independencies can be jointly specified in a single log-mean linear model preserving the smoothness property. In the graphical modelling area, this approach allows us to define a class of parsimonious bi-directed graph models where all the constraints have a clear interpretation in terms of independencies. Moreover, whenever the data at hand seem to satisfy both marginal and conditional independencies that jointly result in a non-smooth model, the log-mean linear parameterization provides a partial solution which allows us to preserve smoothness by specifying the conditional independence statements only in partial tables. Finally, we suggest a criterion for variable coding such that these independencies are tested in partial tables with many observations. A simulation study illustrates how this method increases the efficiency of inferential procedures especially for sparse tables.
2013
- Log-mean linear models for binary data
[Articolo su rivista]
A., Roverato; M., Lupparelli; LA ROCCA, Luca
abstract
This paper introduces a novel class of models for binary data, which we call log-mean linear models. They are specified by linear constraints on the log-mean linear parameter, defined as a log-linear expansion of the mean parameter of the multivariate Bernoulli distribution. We show that marginal independence relationships between variables can be specified by setting certain log-mean linear interactions to zero and, more specifically, that graphical models of marginal independence are log-mean linear models. Our approach overcomes some drawbacks of the existing parameterizations of graphical models of marginal independence.
2013
- Objective Bayesian Search of Gaussian Directed Acyclic Graphical Models for Ordered Variables with Non-Local Priors
[Articolo su rivista]
D., Altomare; G., Consonni; LA ROCCA, Luca
abstract
Directed acyclic graphical (DAG) models are increasingly employed in the study of physical and biological systems to model direct influences between variables. Identifying the graph from data is a challenging endeavor, which can be more reasonably tackled if the variables are assumed to satisfy a given ordering; in this case we simply have to estimate the presence or absence of each potential edge. Working under this assumption, we propose an objective Bayesian method for searching the space of Gaussian DAG models, which provides a rich output from minimal input. We base our analysis on non-local parameter priors, which are especially suited for learning sparse graphs, because they allow a faster learning rate, relative to ordinary local parameter priors, when the true unknown sampling distribution belongs to a simple model. We implement an efficient stochastic search algorithm, which deals effectively with data sets having sample size smaller than the number of variables, and apply our method to a variety of simulated and real data sets. Our approach compares favorably, in terms of the ROC curve for edge hit rate versus false alarm rate, to current state-of-the-art frequentist methods relying on the assumption of ordered variables; under this assumption it exhibits a competitive advantage over the PC-algorithm, which can be considered as a frequentist benchmark for unordered variables. Importantly, we find that our method is still at an advantage for learning the skeleton of the DAG, when the ordering of the variables is only moderately mis-specified. Prospectively, our method could be coupled with a strategy to learn the order of the variables, thus dropping the known ordering assumption.
2013
- The Whetstone and the Alum Block: Balanced Objective Bayesian Comparison of Nested Models for Discrete Data
[Articolo su rivista]
G., Consonni; J. J., Forster; LA ROCCA, Luca
abstract
When two nested models are compared, using a Bayes factor, from an objective standpoint, two seemingly conflicting issues emerge at the time of choosing parameter priors under the two models. On the one hand, for moderate sample sizes, the evidence in favor of the smaller model can be inflated by diffuseness of the prior under the larger model. On the other hand, asymptotically, the evidence in favor of the smaller model typically accumulates at a slower rate. With reference to finitely discrete data models, we show that these two issues can be dealt with jointly, by combining intrinsic priors and nonlocal priors in a new unified class of priors. We illustrate our ideas in a running Bernoulli example, then we apply them to test the equality of two proportions, and finally we deal with the more general case of logistic regression models.
2012
- Binary models of marginal independence: a comparison of different approaches
[Relazione in Atti di Convegno]
M., Lupparelli; LA ROCCA, Luca
abstract
This work compares the performances of three parameterizations for defining parsimonious submodels of binary bidirected graph models. In particular, it shows that the log-mean linear parameterization, recently developed by Roverato and coauthors, provides a useful tool to include in the model additional linear constraints with a clear interpretation in terms of independencies in special subpopulations. The features of the three parameterizations are illustrated through an example based on a data set with seven binary variables.
2012
- Objective Bayes factors for Gaussian directed acyclic graphical models
[Articolo su rivista]
G., Consonni; LA ROCCA, Luca
abstract
We propose an objective Bayesian method for the comparison of all Gaussian directed acyclic graphical models defined on a given set of variables. The method, which is based on the notion of fractional Bayes factor (BF), requires a single default (typically improper) prior on the space of unconstrained covariance matrices, together with a prior sample size hyper-parameter, which can be set to its minimal value. We show that our approach produces genuine BFs. The implied prior on the concentration matrix of any complete graph is a data-dependent Wishart distribution, and this in turn guarantees that Markov equivalent graphs are scored with the same marginal likelihood. We specialize our results to the smaller class of Gaussian decomposable undirected graphical models and show that in this case they coincide with those recently obtained using limiting versions of hyper-inverse Wishart distributions as priors on the graph-constrained covariance matrices.
2011
- Comments on “Nonparametric Bayesian networks” by Ickstadt et al.
[Capitolo/Saggio]
G., Consonni; LA ROCCA, Luca
abstract
Discussion contribution.
2011
- Moment priors for Bayesian model choice with applications to directed acyclic graphs
[Capitolo/Saggio]
G., Consonni; LA ROCCA, Luca
abstract
We propose a new method for the objective comparison of two nested models based on non-local priors. More specifically, starting with a default prior under each of the two models, we construct a moment prior under the larger model, and then use the fractional Bayes factor for a comparison. Non-local priors have been recently introduced to obtain a better separation between nested models, thus accelerating the learning behaviour, relative to currently used local priors, when the smaller model holds. Although the argument showing the superior performance of non-local priors is asymptotic, the improvement they produce is already apparent for small to moderate samples sizes, which makes them a useful and practical tool. As a by-product, it turns out that routinely used objective methods, such as ordinary fractional Bayes factors, are alarmingly slow in learning that the smaller model holds. On the downside, when the larger model holds, non-local priors exhibit a weaker discriminatory power against sampling distributions close to the smaller model. However, this drawback becomes rapidly negligible as the sample size grows, because the learning rate of the Bayes factor under the larger model is exponentially fast, whether one uses local or non-local priors. We apply our methodology to directed acyclic graph models having a Gaussian distribution. Because of the recursive nature of the joint density, and the assumption of global parameter independence embodied in our prior, calculations need only be performed for individual vertices admitting a distinct parent structure under the two graphs; additionally we obtain closed-form expressions as in the ordinary conjugate case. We provide illustrations of our method for a simple three-variable case, as well as for a more elaborate seven-variable situation. Although we concentrate on pairwise comparisons of nested models, our procedure can be implemented to carry-out a search over the space of all models.
2011
- Objective Bayesian comparison of linear regression models
[Relazione in Atti di Convegno]
LA ROCCA, Luca
abstract
In this short paper, I consider the variable selection problem in linear regression models and review two objective Bayesian methods for which I have been developing R code. These two methods, namely, fractional Bayes factors and intrinsic priors, are useful when models are to be compared in lack of substantive prior information. In particular, they are useful when many variables are available for selection, and thus exponentially many models are to be compared, so that subjective prior elicitation under each model is virtually impossible. A case of special interest, which ultimately motivates my work on this topic, is when the structure of an acyclic directed graph is to be learned from data; in this case the model space is even larger, because each graph corresponds to a family of linear regression models.
2010
- Enhanced Objective Bayesian Testing for the Equality of two Proportions
[Working paper]
G., Consonni; J. J., Forster; LA ROCCA, Luca
abstract
WORKING PAPER M10/13, SOUTHAMPTON STATISTICAL SCIENCES RESEARCH INSTITUTE, UNIVERSITY OF SOUTHAMPTON, UK.We develop a new class of prior distributions for Bayesian comparison of nested models, which we call intrinsic moment priors. We aim at testing the equality of two proportions, based on independent samples, and thus focus on discrete data models. We illustrate our methodology in a running Bernoulli example, where we test a sharp null hypothesis, then we implement our procedure to test the equality of two proportions. A detailed analysis of the properties of our method is presented together with an application to a collection of real-world 2*2 tables involving a sensitivity analysis and a crossvalidation study.
2010
- Innovation and performance: some evidence from Italian firms
[Relazione in Atti di Convegno]
Ferrari, Mascia; LA ROCCA, Luca
abstract
PURPOSE - This paper aims to explain through a statistical model the link between innovation and performance. The data taken into consideration is from Unicredit Group survey for the period 2004-2006 on Italian manufacturing firms.METHODOLOGY - We consider a broad concept of innovation: investments in R&D and technology, new processes, new products, innovation in terms of marketing and organization, investments in training of human resources. Performance is measured in terms of ROA without considering extraordinary items and taxes, to eliminate exceptional events and fiscal aspects.FINDINGS - With respect to innovation strategies, we find a weak, but significant, relationship between ROA and innovation. In addition, the influence of innovation on ROA does depend on innovation types and industry structures. Conversely, the amount of innovation expenditures does not have an influence on performance.LIMITATIONS - The main limitations of our analysis are represented by the missing values coming from the financial reporting in short form and by the consideration of a short time period (the year 2006), with reference to the innovation expenditures and the measurement of performance.IMPLICATIONS - From a managerial point of view, our model describing the relationship between innovation drivers and financial performance might represent a useful tool for managers aiming to introduce or implement innovation strategies in their organization. ORIGINALITY - Innovation is a common topic in econometric studies but not so much in managerial and accounting literature. The goal of the paper is to link macro and micro perspectives in a combined framework based on managerial and financial accounting.
2009
- Non local alternative priors for Gaussian directed acyclic graphical models
[Relazione in Atti di Convegno]
LA ROCCA, Luca; G., Consonni
abstract
Prior choice for Bayesian model comparison can be problematic for several reasons. In particular, for the comparison of two nested models, it was recently pointed out that typical prior choices may produce an unsatisfactory learning behavior of the Bayes factor. More in detail, if the sub-model is not true the accumulation of evidence is exponentially fast in favor of the encompassing model, whereas it is only sub-linear in favor of the sub-model under the assumption that the latter is true. To alleviate this imbalance, it was suggested that the prior under the encompassing model be modified so that it vanishes over the sub-space corresponding to the sub-model, thus obtaining a Non Local Alternative Prior (NLAP). In this work, we develop NLAPs for the comparison of Gaussian directed acyclic graphical models, and contrast their performance with that of traditional priors.
2008
- Bayesian non-parametric estimation of smooth hazard rates for seismic hazard assessment
[Articolo su rivista]
LA ROCCA, Luca
abstract
Hazard rate estimation is an alternative to density estimation for positive variables that is of interest when variables are times to event. In particular, it is here shown that hazard rate estimation is useful for seismic hazard assessment. This paper suggests a simple, but flexible, Bayesian method for non-parametric hazard rate estimation, based on building the prior hazard rate as the convolution mixture of a Gaussian kernel with an exponential jump-size compound Poisson process. Conditions are given for a compound Poisson process prior to be well-defined and to select smooth hazard rates, an elicitation procedure is devised to assign a constant prior expected hazard rate while controlling prior variability, and a Markov chain Monte Carlo approximation of the posterior distribution is obtained. Finally, the suggested method is validated in a simulation study, and some Italian seismic event data are analysed.
2008
- Chain graph models: topological sorting of meta-arrows and efficient construction of B-essential graphs
[Articolo su rivista]
LA ROCCA, Luca; A., Roverato
abstract
Essential graphs and largest chain graphs are well-established graphical representations of equivalence classes of directed acyclic graphs and chain graphs respectively, especially useful in the context of model selection. Recently, the notion of a labelled block ordering of vertices B was introduced as a flexible tool for specifying subfamilies of chain graphs. In particular, both the family of directed acyclic graphs and the family of “unconstrained” chain graphs can be specified in this way, for the appropriate choice of B. The family of chain graphs identified by a labelled block ordering of vertices B is partitioned into equivalence classes each represented by means of a B-essential graph. In this paper, we introduce a topological ordering of meta-arrows and use this concept to devise an efficient procedure for the construction of B-essential graphs. In this way we also provide an efficient procedure for the construction of both largest chain graphs and essential graphs. The key feature of the proposed procedure is that every meta-arrow needs to be processed only once.
2008
- Counting the languages we could speak
[Relazione in Atti di Convegno]
La Rocca, Luca; Guardiano, Cristina
abstract
The Principles and Parameters (P&P) approach to the study of the human language faculty, stemming from Chomsky’s investigations in the 1980s, is based on the idea that syntactic knowledge is determined by the interaction of at least two sets of abstract entities: a) Principles, i.e., unvarying linguistic universals; b) Parameters, i.e., open choices between binary values innately predefined by Universal Grammar (UG), that must be closed (set) by language learners on the basis of environmental evidence. Parameters are supposed to be finite in number and to form a list of universal options that define the whole space of variation of biologically acquirable human grammars; thus, the apparently enormous amount of language variation seems reducible to the possible combinations of parameter values. This reduction represents one of the major contributions of P&P theories to the biolinguistic framework, in order to explain cultural variation and to provide a reliable model of language acquisition. Furthermore, recent developments suggest that parametric analyses have the potential to renovate the evolutionary and historical study of cognitive domains.Discovering the whole list of UG parameters is a challenging task which is far from completion. A feasible strategy to systematically analyse parameter variation is to adopt heuristic models which reduce the magnitude of grammatical diversity, though preserving its complexity. The analysis of the (ideally) whole list of parameters concerning a single, independent and internally coherent module of the grammar (the nominal domain) proposed by Longobardi and coauthors is a first contribution in this direction that hints at interesting theoretical and historical results. In particular, partial interactions between parameters and their impact on the downsizing of grammatical variation have been explored; a partial interaction occurs when the setting of a certain (set of) parameter(s) induces the setting of a specific value of another parameter. Focusing on a list of 50 parameters, Longobardi and coauthors show that the phenomenon is quite pervasive: 38 out of 50 parameters undergo the effect of at least one other parameter; 28 out of 50 parameters induce an effect on at least one other parameter. As a consequence, the total number of possible languages comes to be considerably reduced. Assessing the amount of this reduction is the goal of this work.
2008
- Package giRaph for graph representation in R
[Relazione in Atti di Convegno]
LA ROCCA, Luca; C., Dethlefsen; J. H., Badsberg
abstract
This paper presents the giRaph package for R, providing formal classes and methods to handle a broad family of graphs, including graphs with loops, multiple edges and hyperedges (i.e. edges involving more than two vertices) both directed and undirected. Since there is no unique way to represent a graph that is optimal for all computations, four different representations are considered: incidence list, incidence matrix, adjacency list and adjacency matrix. Simple methods to set and retrieve information using these representationsare made available.
2008
- Tests based on intrinsic priors for the equality of two correlated proportions
[Articolo su rivista]
G., Consonni; LA ROCCA, Luca
abstract
Correlated proportions arise in longitudinal (panel) studies. A typical example is the “opinion swing” problem: “Has the proportion of people favoring a politician changed after his recent speech to the nation on TV?” Because the same group of individuals is interviewed before and after the speech, the two proportions are correlated. A natural null hypothesis to be tested is whether the corresponding population proportions are equal. A standard Bayesian approach to this problem has already been considered in the literature, based on a Dirichlet prior for the cell probabilities of the underlying 2 × 2 table under the alternative hypothesis, together with an induced prior under the null. With a lack of specific prior information, a diffuse (e.g., uniform) distribution may be used. We claim that this approach is not satisfactory, because in a testing problem one should make sure that the prior under the alternative is adequately centered around the region specified by the null, in order to obtain a fairer comparison between the two hypotheses, especially when the data are in reasonable agreement with the null. Following an intrinsic prior methodology, we develop two strategies for the construction of a collection of objective priors increasingly peaked around the null. We provide a simple interpretation of their structure in terms of weighted imaginary sample scenarios. We illustrate our method by means of three examples, carrying out sensitivity analysis and providing comparison with existing results.
2006
- On block ordering of variables in graphical modelling
[Articolo su rivista]
A., Roverato; LA ROCCA, Luca
abstract
In graphical modelling, the existence of substantive background knowledge on block ordering of variables is used to perform structural learning within the family of chain graphs (CGs) in which every block corresponds to an undirected graph and edges joining vertices in different blocks are directed in accordance with the ordering. We show that this practice may lead to an inappropriate restriction of the search space and introduce the concept of labelled block ordering B corresponding to a family of B-consistent CGs in which every block may be either an undirected graph or a directed acyclic graph or, more generally, a CG. In this way we provide a flexible tool for specifying subsets of chain graphs, and we observe that the most relevant subsets of CGs considered in the literature are families of B-consistent CGs for the appropriate choice of B. Structural learning within a family of B-consistent CGs requires to deal with Markov equivalence. We provide a graphical characterization of equivalence classes of B-consistent CGs, namely the B-essential graphs, as well as a procedure to construct the S-essential graph for any given equivalence class of B-consistent chain graphs. Both largest CGs and essential graphs turn out to be special cases of B-essential graphs.
2005
- On Bayesian Nonparametric Estimation of Smooth Hazard Rates with a View to Seismic Hazard Assessment
[Working paper]
LA ROCCA, Luca
abstract
WORKING PAPER R 38-05, DIPARTIMENTO DI SCIENZE SOCIALI, COGNITIVE E QUANTITATIVE, UNIVERSITA' DI MODENA E REGGIO EMILIA, REGGIO EMILIA, ITALY.Nonparametric inference on the hazard rate is an alternative to density estimation for positive variables which naturally deals with right censored observations. It is a classic topic of survival analysis which is here shown to be of interest in the applied context of seismic hazard assessment. This paper puts forth a new Bayesian approach to hazard rate estimation, based on building the prior hazard rate as a convolution mixture of a probability density with a compound Poisson process. The resulting new class of nonparametric priors is studied in view of its use for Bayesian inference: first, conditions are given for the prior to be well defined and to select smooth distributions; then, a procedure is developed to choose the hyperparameters so as to assign a constant expected prior hazard rate, while controlling prior variability; finally, an MCMC approximation of the posterior distribution is found. The proposed algorithm is implemented for the analysis of some Italian seismic event data and a possible adjustment to a well established class of prior hazard rates is discussed in some detail.
2005
- Stima bayesiana non parametrica della funzione di intensità con applicazione alla valutazione della pericolosità sismica di alcune zone sismogenetiche italiane
[Articolo su rivista]
LA ROCCA, Luca
abstract
Nota di presentazione della tesi di dottorato.
2004
- A Bayesian hierarchical model for the evaluation of a Website
[Articolo su rivista]
L., DI SCALA; LA ROCCA, Luca; G., Consonni
abstract
Consider a website and the surfers visiting its pages. A typical issue of interest, for example while monitoring an advertising campaign, concerns whether a specific page has been designed successfully, i.e. is able to attract surfers or address them to other pages within the site. We assume that the surfing behaviour is fully described by the transition probabilities from one page to another, so that a clickstream (sequence of consecutively visited pages) can be viewed as a finite-state-space Markov chain. We then implement a variety of hierarchical prior distributions on the multivariate logits of the transition probabilities and define, for each page, a content effect and a link effect. The former measures the attractiveness of the page due to its contents, while the latter signals its ability to suggest further interesting links within the site. Moreover, we define an additional effect, representing overall page success, which incorporates both effects previously described. Using WinBUGS, we provide estimates and credible intervals for each of the above effects and rank pages accordingly.
2004
- On Bayesian analysis of the proportional hazards model
[Relazione in Atti di Convegno]
LA ROCCA, Luca
abstract
This work deals with Bayesian inference for Cox's proportional hazards model. After a brief introduction to the problem, from a historical perspective, an MCMC solution is suggested, based on a novel Bayesian nonparametric approach to hazard rate estimation. The suggested method, allowing for right censored as well as exact observations, is then applied to the well-known leukemia remission times in Cox's landmark paper.
2002
- A Markovian mixture model for Web data
[Articolo su rivista]
L., DI SCALA; LA ROCCA, Luca
abstract
In this paper we carry out a site-centric clickstream analysis by fitting a probabilistic model to the click sequences of surfers browsing an e-commerce Web site. In particular, surfers’ paths are modeled as observations originating from a finite mixture of Markov chains which takes values in the site’s page-space. We preliminarily tackle the problem of making inference on the number of distinct visits in which each surfers’ sequence of clicks can be divided. We then deal with goodness-of-fit testing of the model to real data, exploring the heterogeneity of surfers. Finally, by using a simple model with only two components, we shed light on the relationship between surfing behavior and tendency to on-line purchasing.
2002
- Probabilistic modelling for clickstream analysis
[Relazione in Atti di Convegno]
L., DI SCALA; LA ROCCA, Luca
abstract
Abstract. Our aim is to explore the possibility of performing site-centric clickstream analysis by means of probabilistic modelling. We consider the clickstream originating from a given Web site as a Markovian sequence taking values in the site's page-space. An extra page is added which represents the rest of the Web and is used to determine clickstream fractures (i.e. multiple visits). Different models for the memory of Web surfers and for their heterogeneity are investigated. As an example, the methodology is then applied to data originating from an e-commerce site.