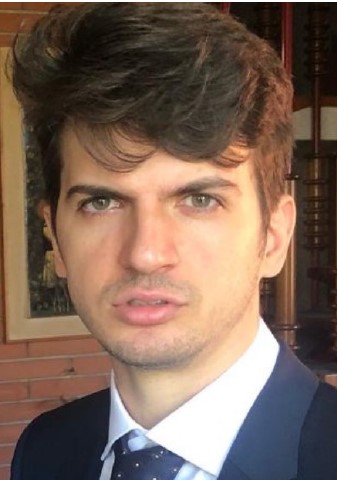 |
GIULIO DE SABBATA
Dottorando Dipartimento di Ingegneria "Enzo Ferrari"
|
Home |
Pubblicazioni
2022
- Big Data Integration for Data-Centric AI
[Abstract in Atti di Convegno]
Bergamaschi, Sonia; Beneventano, Domenico; Simonini, Giovanni; Gagliardelli, Luca; Aslam, Adeel; De Sabbata, Giulio; Zecchini, Luca
abstract
Big data integration represents one of the main challenges for the use of techniques and tools based on Artificial Intelligence (AI) in several crucial areas: eHealth, energy management, enterprise data, etc. In this context, Data-Centric AI plays a primary role in guaranteeing the quality of the data on which these tools and techniques operate. Thus, the activities of the Database Research Group (DBGroup) of the “Enzo Ferrari” Engineering Department of the University of Modena and Reggio Emilia are moving in this direction. Therefore, we present the main research projects of the DBGroup, which are part of collaborations in various application sectors.
2022
- High Levels of Circulating Tumor Plasma Cells as a Key Hallmark of Aggressive Disease in Transplant-Eligible Patients With Newly Diagnosed Multiple Myeloma
[Articolo su rivista]
Bertamini, L.; Oliva, S.; Rota-Scalabrini, D.; Paris, L.; More, S.; Corradini, P.; Ledda, A.; Gentile, M.; De Sabbata, G.; Pietrantuono, G.; Pascarella, A.; Tosi, P.; Curci, P.; Gilestro, M.; Capra, A.; Galieni, P.; Pisani, F.; Annibali, O.; Monaco, F.; Liberati, A. M.; Palmieri, S.; Luppi, M.; Zambello, R.; Fazio, F.; Belotti, A.; Tacchetti, P.; Musto, P.; Boccadoro, M.; Gay, F.
abstract
PURPOSE High levels of circulating tumor plasma cells (CTC-high) in patients with multiple myeloma are a marker of aggressive disease. We aimed to confirm the prognostic impact and identify a possible cutoff value of CTC-high for the prediction of progression-free survival (PFS) and overall survival (OS), in the context of concomitant risk features and minimal residual disease (MRD) achievement.METHODS. CTC were analyzed at diagnosis with two-tube single-platform flow cytometry (sensitivity 4 x 10(-5)) in patients enrolled in the multicenter randomized FORTE clinical trial (ClinicalTrials.gov identifier: NCT02203643). MRD was assessed by second-generation multipara meter flow cytometry (sensitivity 10(-5)). We tested different cutoff values in series of multivariate (MV) Cox proportional hazards regression analyses on PFS outcome and selected the value that maximized the Harrell's C-statistic. We analyzed the impact of CTC on PFS and OS in a MV analysis including baseline features and MRD negativity.RESULTS CTC analysis was performed in 401 patients; the median follow-up was 50 months (interquartile range, 45-54 months). There was a modest correlation between the percentage of CTC and bone marrow plasma cells (r = 0.38). We identified an optimal CTC cutoff of 0.07% (approximately 5 cells/mu L, C-index 0.64). In MV analysis, CTC-high versus CTC-low patients had significantly shorter PFS (hazard ratio, 2.61; 95% CI, 1.49 to 2.97, P < .001; 4-year PFS 38% v69%) and OS (hazard ratio, 2.61; 95% CI, 1.49 to 4.56; P < .001; 4-year OS 68% v92%). The CTC levels, but not the bone marrow plasma cell levels, affected the outcome. The only factor that reduced the negative impact of CTC-high was the achievement of MRD negativity (interaction P = .039).CONCLUSION In multiple myeloma, increasing levels of CTC above an optimal cutoff represent an easy-to-assess, robust, and independent high-risk factor. The achievement of MRD negativity is the most important factor that modulates their negative prognostic impact. (C) 2022 by American Society of Clinical Oncology
2022
- Progressive Entity Resolution with Node Embeddings
[Relazione in Atti di Convegno]
Simonini, Giovanni; Gagliardelli, Luca; Rinaldi, Michele; Zecchini, Luca; De Sabbata, Giulio; Aslam, Adeel; Beneventano, Domenico; Bergamaschi, Sonia
abstract
Entity Resolution (ER) is the task of finding records that refer to the same real-world entity, which are called matches. ER is a fundamental pre-processing step when dealing with dirty and/or heterogeneous datasets; however, it can be very time-consuming when employing complex machine learning models to detect matches, as state-of-the-art ER methods do. Thus, when time is a critical component and having a partial ER result is better than having no result at all, progressive ER methods are employed to try to maximize the number of detected matches as a function of time.
In this paper, we study how to perform progressive ER by exploiting graph embeddings. The basic idea is to represent candidate matches in a graph: each node is a record and each edge is a possible comparison to check—we build that on top of a well-known, established graph-based ER framework. We experimentally show that our method performs better than existing state-of-the-art progressive ER methods on real-world benchmark datasets.